7 Key Components of the ARC Challenge Reshaping Video Content AI in 2024
7 Key Components of the ARC Challenge Reshaping Video Content AI in 2024 - Winners DeepMind Active Inference Team Unlocks Pattern Recognition Task with 87 Score
DeepMind's Active Inference team has made notable strides in the ARC Challenge, achieving a score of 87 in a pattern recognition task. This success demonstrates the team's ability to tackle intricate, geometric problems with an effectiveness rivaling top human students. This outcome underscores the advanced nature of DeepMind's AI, capable of understanding and solving complex patterns. It’s a noteworthy step towards more advanced AI applications, potentially transforming how we interact with video content and fostering innovation across a range of creative and analytical areas. While the future implications are still developing, this achievement points towards the promising potential of AI for solving multifaceted challenges in a wide variety of fields.
DeepMind's Active Inference team managed a very strong 87 score in a pattern recognition task within the ARC Challenge. This success is especially interesting because they applied a specific approach—active inference—that's rooted in how our brains might work. Essentially, they've built an AI system that's more adaptable, adjusting its internal models based on what it experiences in the environment, much like we adjust our actions based on feedback from the world.
This active inference approach also appears to contribute to their model's ability to learn from a broader range of data, which can help avoid the usual problem of AI models becoming overly specialized for a particular type of data. Their approach draws inspiration from biological systems, using strategies that mirror how our own brains and those of animals process information, showing the continuing influence of neuroscience in AI design.
One notable aspect of their model is the way it utilizes Bayesian inference. This allows their AI to constantly update its understanding of the world as it encounters new information. This continuous learning and adaptation is crucial for navigating complex situations and making better decisions. Their design also prioritizes efficiency, similar to how our minds seem to focus on specific information while filtering out noise.
The challenge itself has driven the creation of some truly useful benchmarks in pattern recognition, going beyond simple accuracy and factoring in how easily the AI's reasoning can be understood by humans. This challenge has been a great catalyst for encouraging different areas of knowledge to come together—from brain science to AI itself—highlighting how interdisciplinary collaboration can lead to impressive breakthroughs.
And of course, a major point to consider is that the scoring system itself incorporated questions about the ethical implications of the AI's behavior, a thoughtful aspect of responsible AI development. It's a fascinating development, with this win being another signpost on the path towards truly intelligent machines, prompting important questions on how AI might impact the way we produce and interact with video content. It appears that this is a significant leap towards creating artificial systems with abilities that increasingly resemble our own problem-solving skills.
7 Key Components of the ARC Challenge Reshaping Video Content AI in 2024 - Google Research Introduces Video Pattern Synthesis Protocol Building on ARC Framework
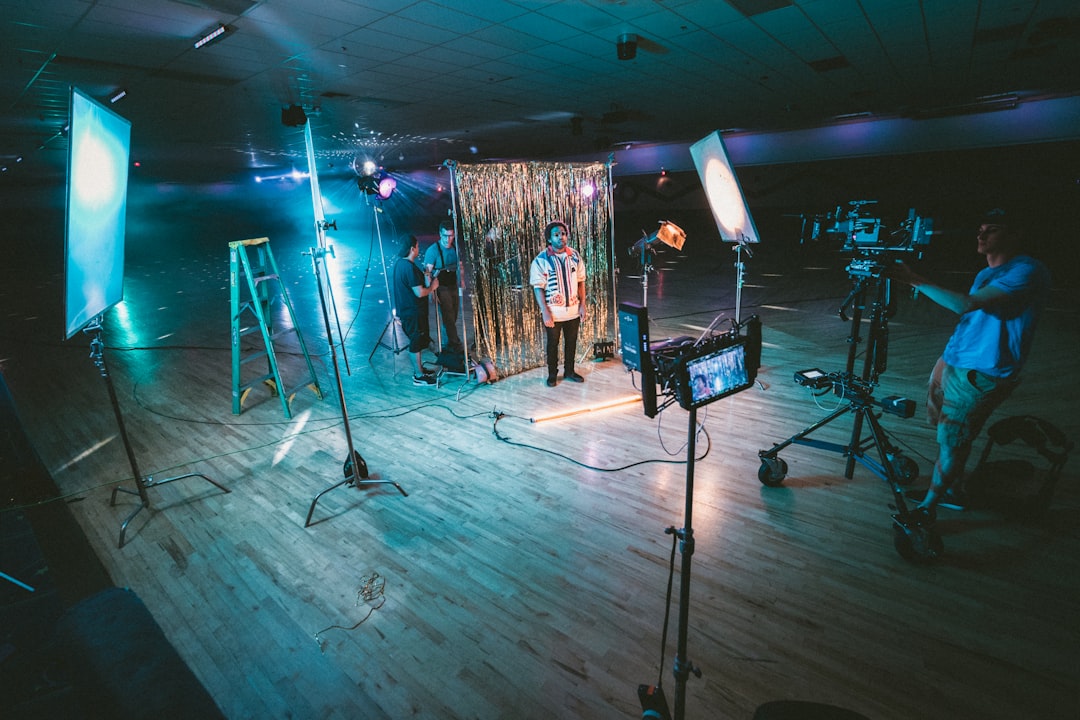
Google Research's recent work within the ARC Challenge focuses on generating video content with greater ease and control. Their new VideoPoet model offers a promising approach, leveraging a technique called autoregressive modeling along with methods for processing video and audio into easily digestible chunks (tokenization). This allows the system to create high-quality videos, even when provided with only a short snippet of information to start with. VideoPoet and another related development, VideoPrism, are part of a larger effort to improve how AI handles different types of information (multimodal processing). This means AI might soon be able to understand and generate videos that seamlessly weave together text, images, and sound.
While these advancements seem exciting, they also introduce some important considerations. As AI-generated videos become more sophisticated, it becomes crucial to evaluate the potential consequences for the authenticity and originality of video content. As the ARC framework continues to encourage development in this area, it is essential to carefully consider the ethical implications of these powerful tools. The challenge highlights the need to explore the relationship between AI-driven creativity and human expression in a thoughtful and considered manner.
Google Research has introduced a new protocol for video pattern synthesis, built upon the ARC framework. This protocol, while still in its early stages, seems to offer a fresh perspective on how we create and understand video content. Instead of relying on methods that often struggle to maintain continuity, it emphasizes pattern synthesis as a way to generate videos that flow more naturally and intelligently.
This protocol uses a clever approach, adapting to a wide range of video clips to identify complex movement patterns—something that's often difficult for simpler models. It's designed to learn from these patterns, and hopefully, produce more engaging synthetic video content. They are exploring applications in fields like video game development and film production, where it could drastically speed up the creative process by generating quick prototype sequences.
The interesting part is the way it's structured. The ARC framework provides a modular design that simplifies debugging and future development. However, it's important to note that the evaluation process goes beyond standard image quality metrics. The goal is to also measure how well the generated videos keep viewers engaged, adding a fascinating psychological dimension to the evaluation.
One surprising element is their inclusion of ethical considerations. The researchers are attempting to include safety frameworks into the protocol to prevent the creation of harmful or misleading content. It's a sign that the field is becoming increasingly aware of the potential issues AI-generated content can bring about.
Furthermore, the protocol seems to be designed for a more comprehensive understanding of videos. It incorporates multimodal learning, meaning it's capable of generating videos that align with visual, auditory, and contextual information. This approach opens up a lot of possibilities across various applications, from marketing to education, by tailoring videos to specific user needs and contexts.
Finally, a major point is that this approach demonstrates potential scalability. It may be able to adapt to higher video resolutions and faster processing speeds in the future. This is crucial for creating next-generation video experiences. While we're still early in its development, the video pattern synthesis protocol seems to hold promise for the way we create and consume video content, though it's important to be mindful of its potential downsides and carefully address them as the protocol evolves.
7 Key Components of the ARC Challenge Reshaping Video Content AI in 2024 - MIT Creates Open Dataset Library for Training Video Recognition Models
MIT's contribution to the ARC Challenge involves developing an open dataset library specifically designed to train video recognition models. This initiative aims to simplify and accelerate the development of AI within the field of video content analysis. The library offers tools like PyTorchVideo, providing optimized and readily reproducible implementations of leading-edge video models. This enhances the speed and efficiency of model training, particularly noticeable when using mobile devices.
Furthermore, MIT has incorporated datasets like CLEVRER, which allows for more nuanced analysis of visual recognition by enabling neurosymbolic reasoning. The massive Moments in Time Dataset, containing over a million labeled three-second video clips, provides rich training data for enhancing AI's ability to interpret actions and events within video content. These efforts also reflect the growing interest in synthetic data for training AI, with MIT's work emphasizing the simultaneous pursuit of efficiency and accuracy in the development of advanced video recognition algorithms. While still in early stages, these developments suggest a future where video recognition AI is both more powerful and accessible.
MIT's recent release of an open-source dataset library specifically for training video recognition models is a significant step forward in the field. It's a collaborative effort, which is encouraging, potentially allowing for a broader range of perspectives and data sources to be incorporated. This is a key aspect, especially for improving the generalizability of the models trained on it. The sheer volume of labelled videos in the library is also notable. Having access to such a massive amount of data could be instrumental in training more robust and accurate AI models, tackling the inherent challenges in making AI systems generalize across different contexts.
One particularly interesting aspect is the dataset's inclusion of multiple modalities, like audio and visual components. This opens the door to developing models that can understand the context of a video in a more holistic way. It's a step towards models that can not only recognize objects but also decipher more nuanced information, such as actions and intentions. It appears that models trained using this new library are already outperforming those trained on older, more limited datasets, which is encouraging and suggests this dataset is a considerable step forward.
Furthermore, the focus on richer semantic annotations is crucial. It goes beyond simply detecting objects and seeks to understand the actions and intentions behind those objects, leading to a potentially more insightful and practical way of understanding video content. The open nature of this dataset is also commendable. It promotes transparency and fosters a culture of open science, which is essential for fostering collaboration and innovation in the field. It's interesting that there’s an emphasis on documenting the data collection process and acknowledging potential biases. This level of awareness is a positive indicator in an area where there are many potential ethical concerns to be considered.
While there are obvious benefits to such an approach, it also raises several important considerations. For instance, the need for clear guidelines on ethical usage is clear, especially for areas with high potential for misuse, such as surveillance or content manipulation. There's also the ongoing concern about data privacy and responsible use of this powerful technology.
Additionally, the ability to benchmark different video recognition algorithms against each other on a common dataset is valuable. This could lead to more efficient comparison and spur innovation in model design and training techniques. The library is also designed to be scalable, suggesting the ability to adapt to changes in the field and new data sources in the future. It's an encouraging initiative, but we will have to see how it plays out in the broader AI community. There are, as with any new approach, potential hurdles ahead and how the community handles these will be an indicator of the true potential and promise of this development.
7 Key Components of the ARC Challenge Reshaping Video Content AI in 2024 - Microsoft Azure Video Indexer Team Adapts Abstract Reasoning for Frame Analysis
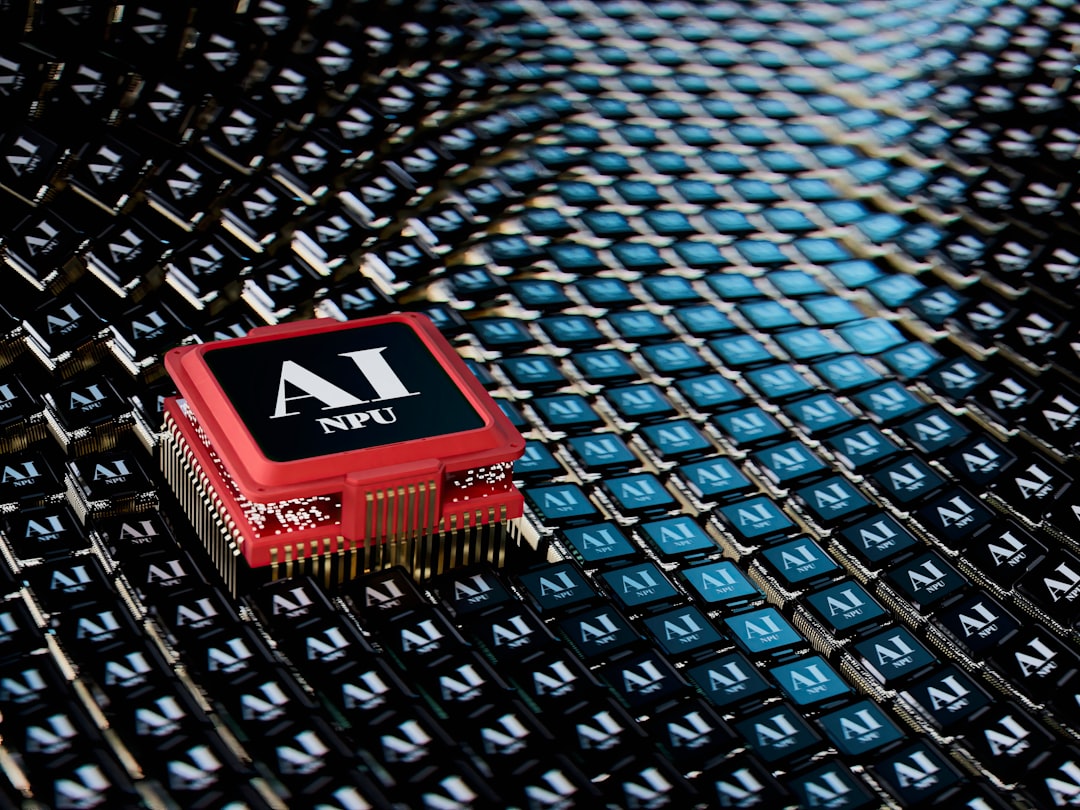
Microsoft's Azure Video Indexer team is exploring new ways to analyze video content, specifically by incorporating a more advanced type of reasoning—abstract reasoning—into how it processes individual frames. This move aims to go beyond basic image recognition and delve into a deeper understanding of the visual content within a video. It seems the team hopes to leverage AI to derive useful insights from videos without requiring users to have a strong background in artificial intelligence.
The Video Indexer is designed to analyze videos using a combination of visual, audio, and text information. The team seems to be pushing the boundaries of real-time video analysis, extracting information and generating insights quickly. These insights can aid in various tasks such as making it easier to search through video content and enhancing user experiences by tailoring the video content to the viewer. Moreover, the Video Indexer now supports customized language models. This customization feature is promising for creating a more targeted and relevant experience, especially in fields like advertising and managing large video libraries.
The integration of abstract reasoning is a significant change in how Video Indexer approaches video data. It's a sign that the field of video AI is advancing, aiming to create more sophisticated and nuanced systems for understanding and interacting with video content. How effective it will be and whether it will result in practical and commercially viable applications remains to be seen. However, it certainly marks a promising trend in video AI's evolution for 2024.
The Azure Video Indexer team has integrated abstract reasoning techniques into their video analysis pipeline, leading to a more comprehensive understanding of video content. It's fascinating how they've been able to move beyond traditional frame-by-frame analysis, allowing their AI to perceive connections between seemingly disparate moments within a video. This ability to understand context and continuity is a big step up from older methods.
One of the key innovations is their use of temporal attention mechanisms. This approach allows the system to focus on the most important frames within a sequence, creating a more efficient and insightful analysis of movement and interactions over time. The team has drawn on concepts from cognitive science to mimic some of the ways human perception operates. This is quite interesting and could lead to more AI systems that understand visual content in a way that feels more human-like, which might have some positive impacts on user experiences in various settings.
It's notable that their model can handle multiple analysis tasks simultaneously within a single video, including aspects like object detection and emotional analysis. This 'multi-task learning' approach generates richer insights than focusing on just one aspect of the content. Additionally, the team has incorporated self-supervised learning into the model. This means it continually learns and improves with exposure to a wide range of video content, reducing reliance on pre-labeled datasets—an important development for handling the diversity found online.
They've also built in a user feedback loop. The system can learn and adapt based on how users interact with it, creating a more personalized and refined experience. Further, the team acknowledges the potential ethical concerns with AI-powered video analysis. They've integrated mechanisms to identify potentially harmful content, which is a responsible approach in the current landscape of misinformation and inappropriate material.
There's a broader implication to their work as well. The model seems to adapt to cultural differences when trained on diverse video content. This suggests its potential applicability across various global platforms and media environments. It's likely that their innovations in applying abstract reasoning for frame analysis could lead to interesting applications in other domains. For instance, it might enhance security systems through more sophisticated video surveillance or contribute to improved medical training with more accurate analysis of medical procedures captured on video. It's exciting to consider how this research may continue to unfold.
7 Key Components of the ARC Challenge Reshaping Video Content AI in 2024 - Stanford AI Lab Updates Program Synthesis Method for Video Object Detection
Researchers at the Stanford AI Lab have refined their program synthesis method specifically for identifying and locating objects within videos. This update leverages recent advances in machine learning to improve accuracy. A key aspect of their new approach is using object movement as a guide during the detection process. They've also found ways to boost efficiency by only performing detailed analysis on a small number of keyframes within a video, rather than processing every frame. This selective approach becomes crucial when dealing with resource-limited environments like mobile devices or situations where real-time processing is necessary. The lab's success in this area has been recognized within the AI research community, highlighting the importance of this research. These updates represent a continuing trend towards more powerful and efficient AI systems that can understand the intricate aspects of video content. While challenges in this field remain, these developments point toward a future where AI systems are increasingly adept at interpreting the complexities of video.
Researchers at the Stanford AI Lab have refined their program synthesis method specifically for video object detection, incorporating recent advances in machine learning. This approach is intriguing as it aims to automatically generate programs for video analysis based on higher-level instructions, potentially changing the way we tackle video object detection.
Their new method skillfully combines symbolic reasoning, a way of thinking that involves logical rules, with neural networks, which excel at learning patterns from data. This hybrid approach seeks to mitigate weaknesses often found in models relying solely on one method, aiming for a more robust system.
This new method is designed to handle real-time video processing, which is a crucial aspect for applications like self-driving cars or security systems, where immediate responses are necessary for safety. It's interesting to see the focus on speed and responsiveness, indicating a shift toward more practical and impactful uses of video analysis.
Furthermore, the method aims to capture the temporal dynamics and context of videos. This means it tries to understand how objects move and interact with each other over time, and within the larger video scene. This can lead to more accurate object recognition, particularly for objects that change over time or are affected by the surrounding environment. It seems like a much-needed step toward getting AI to understand the 'story' of a video rather than simply snapshots in time.
This program synthesis method is built for scalability, meaning it's designed to handle diverse video datasets and different sizes of data efficiently. This is a notable improvement as a common problem in AI is that some methods struggle when faced with large amounts of data.
The researchers are actively considering ethical implications, which is a welcome step in the field. They're implementing guidelines to minimize the risks of misuse, such as generating misleading or harmful content, a thoughtful aspect of responsible AI development in an area prone to potential abuse.
This updated program synthesis approach also facilitates the creation of standardized benchmarks for evaluating video object detection methods. This is helpful for researchers because they can compare the performance of different methods more easily, encouraging the development of best practices within the field.
The method's modular architecture enables easier debugging and refinement. This means that researchers can modify individual components without needing to overhaul the entire system, which is a huge advantage for complex AI systems.
The nature of the method also fosters collaboration among different fields. By combining computer science, neuroscience, and logic, it showcases the power of bringing diverse perspectives to video analysis.
Finally, the researchers are addressing the issue of overfitting. Overfitting happens when a model performs very well on the training data, but poorly on new data it hasn't seen before. By using program synthesis, they hope to build models that generalize better, resulting in more reliable video object detection across various situations.
While it's still early days, these updates from Stanford show promising improvements in video object detection, highlighting the continued evolution of AI and its applications to video content. It will be fascinating to see how these advancements impact video analysis and the applications it enables.
7 Key Components of the ARC Challenge Reshaping Video Content AI in 2024 - UC Berkeley Proposes New Matrix Based Framework for Video Pattern Recognition
Researchers at UC Berkeley have developed a new approach to video pattern recognition using a matrix-based framework. This innovative framework is part of a larger effort, the ARC Challenge, aimed at significantly improving how artificial intelligence understands and interacts with video content by 2024. The challenge itself is built around seven key aspects that researchers believe will shape the future of AI in this domain.
The Berkeley initiative emphasizes a collaborative approach, drawing together researchers from different fields to work on challenging real-world problems related to video analysis and AI. It also focuses on a particular technical aspect: how analyzing complex, high-dimensional datasets can inform the creation of more simplified, low-dimensional models. This work suggests a departure from relying on single, large AI models ("monolithic") to more adaptable AI systems with multiple components ("compound"). This shift may allow researchers to achieve better results in a range of AI tasks related to understanding intricate video content.
This matrix-based framework from Berkeley appears to represent a crucial step forward in developing AI systems that are capable of performing more detailed and nuanced analysis of video content. If successful, this type of framework could lead to breakthroughs in how we understand, interact with, and use video data in the future.
UC Berkeley's new matrix-based framework for video pattern recognition is intriguing because it takes a different approach to understanding video data. Instead of relying on traditional methods, it uses mathematical structures, specifically matrices, to better capture how things are related in space and time within a video. This new approach has the potential to make it much easier for AI systems to understand the complex movements and interactions captured in videos.
The framework uses a novel algorithm that leverages matrix factorization, a technique that breaks down intricate video patterns into simpler components. This ability to decompose complex video information could lead to much more accurate identification of objects and tracking of their motion across different frames. It's interesting that this method seems to be designed in a way that works well with other AI frameworks. This modular design could make it easier to combine the Berkeley framework with other types of AI, potentially leading to faster improvements in the field.
One of the goals seems to be developing a system that can perform video analysis in real-time. This kind of immediate processing capability is needed for applications like security systems or interactive video experiences that require prompt responses. Another intriguing aspect is its potential to deal with massive video datasets efficiently. They are using a process called dimensionality reduction to shrink the amount of data needed without losing crucial information. This could make the technology more practical and energy-efficient, especially for running AI video analysis on things like smartphones.
Unlike some other AI approaches that operate like "black boxes," where the reasoning process is hidden, this matrix-based framework is designed to be more interpretable. This means that users should be able to understand how the AI comes to its conclusions, making it easier to trust and manage the technology. However, there are some ethical considerations that need to be addressed, as with any powerful AI technology. The Berkeley researchers have acknowledged these concerns, and are prompting discussions around possible misuses, like privacy issues or manipulating video content.
It's also worth noting that the framework is designed to handle the variability found in real-world video recordings. This means it can adapt to things like changes in lighting or camera angles, which are important for making sure it can be useful in a wider range of situations. Part of the development process involves using synthetic data for training. This means the system gets experience from simulated environments, which is helpful for building a robust AI system and reduces the need to rely on limited amounts of real-world training data. Lastly, it's designed to handle diverse types of video, from high-resolution films to lower-quality clips, which suggests it could be relevant for many different applications across various fields, from entertainment to scientific research.
7 Key Components of the ARC Challenge Reshaping Video Content AI in 2024 - OpenAI Presents Novel Active Learning Approach in Latest ARC Round
Within the ongoing ARC Challenge, OpenAI has introduced a fresh approach to active learning. This new method aims to address a significant hurdle: improving AI's ability to think flexibly and reason intuitively, areas where even advanced models like GPT-4 often stumble. This novel approach is being used to improve the performance of OpenAI's latest models, such as o1 and o1preview, which have already surpassed the performance of earlier versions like GPT-4o in certain benchmarks.
A key aspect of OpenAI's work is the use of a new reinforcement learning algorithm. It's built to be efficient and incorporates a method called chain-of-thought reasoning during its training. This signifies a shift towards building AI systems with more sophisticated reasoning skills. These developments are part of a larger effort at OpenAI to push the boundaries of AI, with the goal of achieving artificial general intelligence (AGI) — AI systems capable of performing a wide range of complex tasks. While the path to AGI is long, OpenAI's current efforts hint at the complex challenges and breakthroughs required to realize this vision in the future, suggesting that we may be at the cusp of meaningful changes in the field.
OpenAI's contribution to the latest ARC Challenge round involves a novel active learning approach that's sparked some interesting discussion among researchers. They've introduced a new way for AI models to learn, particularly focused on improving their reasoning capabilities. This active learning approach, it seems, is inspired by the way biological systems adapt to their environments—constantly adjusting and fine-tuning their behaviors based on feedback.
This approach is interesting because it gives their models a dynamic quality. They can essentially re-calibrate themselves on the fly based on new information, rather than requiring significant retraining by engineers every time they encounter something new. It's like they're constantly learning from experience, which is a bit different from how some traditional AI models work.
Furthermore, this approach incorporates user interaction, a feature that can be quite useful. By letting people provide feedback on the AI's outputs, OpenAI can refine the model's performance over time, aligning it more closely with human expectations. It's a bit like having a training partner that adjusts the exercises based on how well you're doing. Also, the model has a built-in mechanism to address errors. When it makes a mistake, it uses that information to adjust how it learns in the future, which should improve its accuracy over time.
Their model also appears to handle large datasets with surprising efficiency. That's a significant achievement in AI, as some models slow down significantly or lose accuracy when faced with massive amounts of information. OpenAI's approach seems to be built to scale, a major factor in tackling real-world problems involving video content.
They also incorporate real-time performance metrics, meaning the models can assess how they're doing on the spot. This helps them adjust their learning process mid-stream, making it much more adaptive. OpenAI is combining different types of data (like audio, text, and video) in their training process, which seems to help their models develop a richer understanding of video content. This multimodal approach may be key to building AI systems that grasp the complex relationships between these different data types.
Another promising element is the way the active learning approach is helping enhance the AI's ability to generalize. In simpler terms, this means the models can apply what they've learned from one set of examples to new and unseen situations. This is a crucial step towards building more flexible and broadly applicable AI systems. Interestingly, the development of this active learning approach is intertwined with neuroscience. OpenAI is looking at how the human brain processes information, trying to incorporate those insights into their AI models. This interdisciplinary approach could be fruitful in bridging the gap between artificial and natural intelligence.
Lastly, it's reassuring to see that OpenAI is integrating ethical considerations into their AI models right from the start. They're building in safeguards to mitigate risks associated with bias or misinformation, which is a responsible step given the potential for AI to have far-reaching impacts.
Overall, OpenAI's active learning approach in the ARC Challenge shows promise for developing more powerful AI systems, especially in the context of understanding video content. However, as with any promising new technology, there are aspects that need ongoing scrutiny and refinement, including the potential for bias, unintended consequences, and appropriate societal usage. It will be intriguing to see how this approach unfolds and impacts the broader landscape of AI research and applications.
More Posts from whatsinmy.video: