Analyze any video with AI. Uncover insights, transcripts, and more in seconds. (Get started for free)
7 Underutilized Video Datasets for AI-Driven Content Analysis Projects
7 Underutilized Video Datasets for AI-Driven Content Analysis Projects - VSPW Dataset Offers 3,536 Videos for Scene Parsing
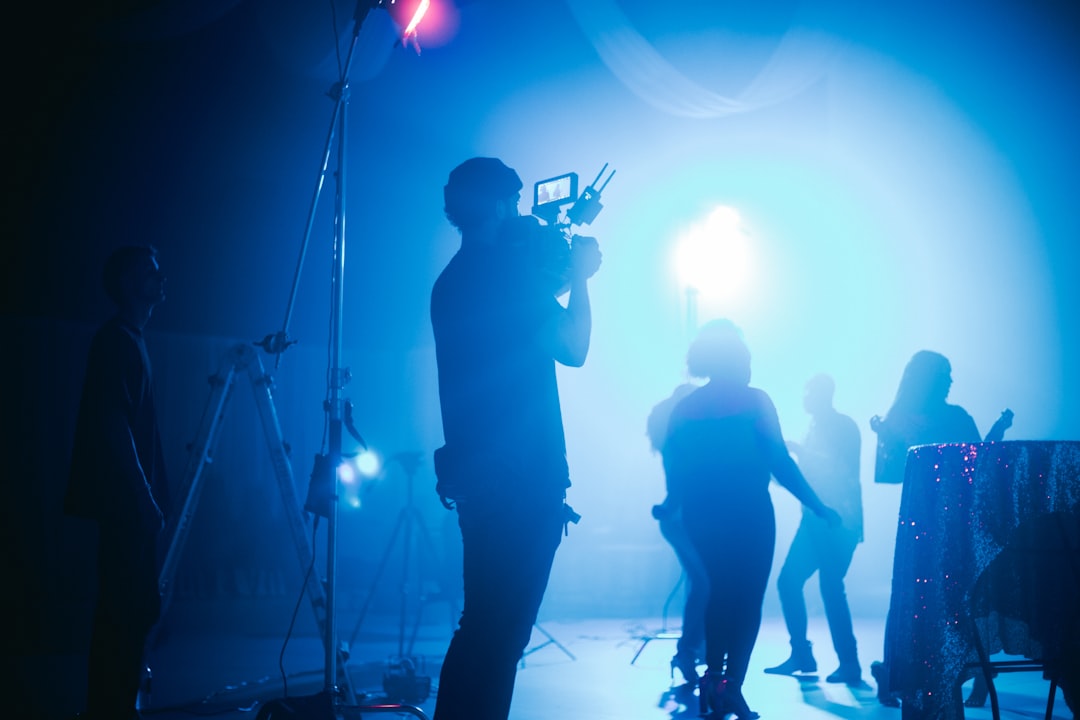
The VSPW dataset provides a substantial set of 3,536 videos specifically designed for scene parsing tasks. These videos, each lasting about five seconds on average, are captured at 15 frames per second. Furthermore, the dataset includes annotations for 124 distinct categories, enabling detailed semantic segmentation analysis. The videos are curated with carefully trimmed clips, ensuring each one encompasses a complete shot. The dataset's primary goal is to advance scene parsing beyond still images and into the realm of video, offering a diverse range of real-world settings suitable for various AI content analysis projects. The inherent challenge within the VSPW dataset lies in the precise labeling of every frame with specific semantic tags, a process aimed at improving the accuracy of video semantic segmentation methods. This dataset's comprehensive nature promises to help propel advancements in this field.
The VSPW dataset, introduced in a 2021 CVPR paper, offers a collection of 3,536 videos specifically designed for scene parsing in video data. These videos, typically around 5 seconds in length, are captured at 15 frames per second, giving us a glimpse into the dynamics of the scenes. Each video snippet is carefully chosen to capture complete shots, effectively presenting the temporal context of the scene. The dataset boasts annotations for 124 categories, making it valuable for tasks like semantic segmentation. The primary motivation behind creating VSPW was to push the field beyond static image scene parsing and explore how to apply these techniques in a more dynamic, video-based context.
This dataset is interesting as it covers a variety of real-world situations, which could be beneficial for exploring scene understanding across varied conditions. However, the core challenge with VSPW lies in its task: automatically associating predefined labels to each pixel in every video frame. This task can be computationally complex, especially given the need for accurate label assignments.
While VSPW provides a large annotated dataset for video scene understanding, its size and the diversity of included scenes could be seen as a limitation for certain research areas. The extensive manual annotation is commendable for providing high-quality data, but there are naturally questions surrounding its scalability for larger-scale video projects. Its strengths are in its emphasis on challenging scene understanding tasks involving movement, such as the identification of people or vehicles in motion within different environments. Still, its value as a benchmark dataset needs to be weighed against the limitations of the data itself, and thus necessitates exploring other datasets for more complete understanding.
7 Underutilized Video Datasets for AI-Driven Content Analysis Projects - HowTo100M Project Analyzes 100 Million Narrated Video Clips
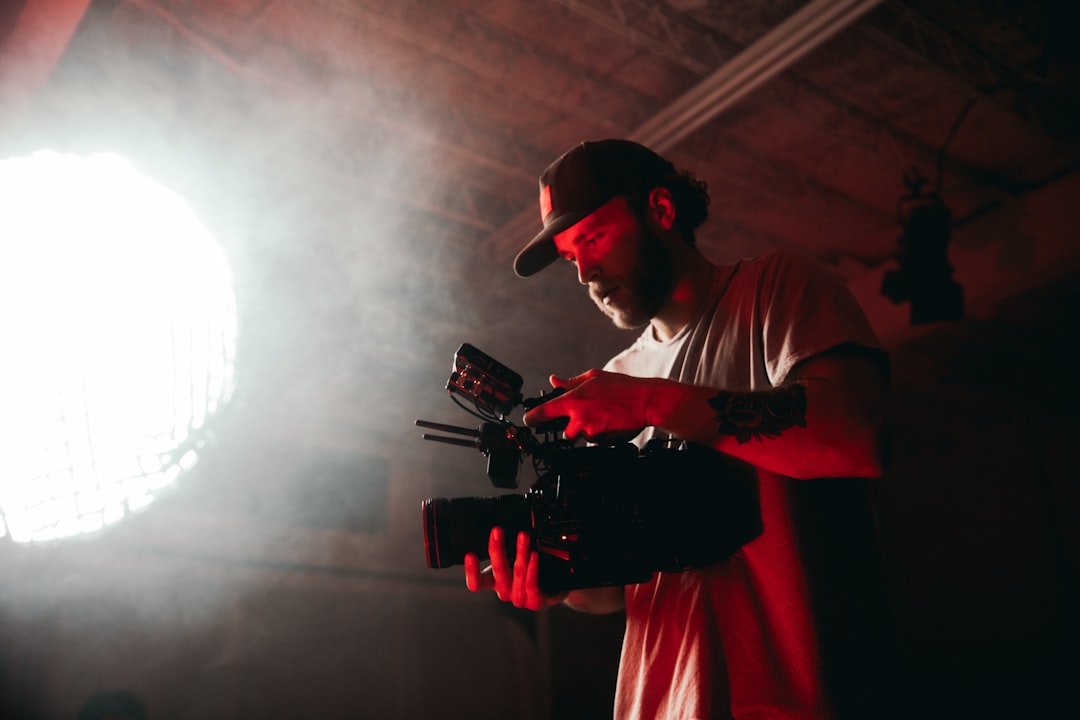
The HowTo100M project delves into a massive collection of 136 million video clips extracted from 122 million instructional videos, each featuring a narrator. This dataset covers a wide variety of visual tasks, exceeding 23,000, with people demonstrating and explaining how to do things across various areas of life. A key feature is its use of readily available, automatically generated YouTube captions to gather a large amount of narrated video content. This approach, in turn, allows researchers to develop a unique joint text-video representation that can enhance the understanding of visual actions in videos. This method also tries to overcome the issue of mismatches between what is seen and heard in narrated videos. Notably, the models created using this dataset have achieved top performance in tasks like finding relevant videos based on text queries and identifying specific actions within videos. The project's success highlights the potential of using large, imperfect datasets to train models for content analysis. Containing roughly 15 years' worth of video content, HowTo100M provides a significant resource for studying how people teach and learn through video, while simultaneously offering an alternative to the traditional, expensive practice of having people manually create captions.
The HowTo100M project delves into a massive collection of 136 million video clips, culled from 122 million instructional videos found online. This scale provides a potentially rich source for studying how people explain things visually. The project's focus is on videos where the creator's aim is to visually communicate a task, often a complex one.
These videos cover a remarkably diverse range of tasks, over 23,000, across many different areas. This breadth makes it a potentially valuable dataset for researchers seeking to create general purpose AI models capable of understanding a variety of human actions. It is interesting that this dataset is built using automatically generated captions from YouTube, allowing for a massive dataset that otherwise might be prohibitively expensive or time-consuming to create.
The HowTo100M team uses this massive dataset to create a joint text-video embedding. In essence, they build a model that can learn from the connection between the spoken words and the visual information within these videos. Notably, this joint embedding shows promise in transferring knowledge to other datasets, suggesting that the learned patterns are not limited to instructional videos.
One intriguing aspect of this work is tackling the challenge of misaligned narration in these instructional videos. Because these are often from a variety of creators, and are not professionally produced, there's a chance that the spoken words might not perfectly match the visuals. The HowTo100M model is designed to handle this, demonstrating its potential in robustly learning from noisy, large-scale data.
The model built using this dataset has produced state-of-the-art results in tasks like text-to-video retrieval and action recognition within instructional video datasets. Its performance is notable on benchmarks like YouCook2 and CrossTask. This suggests that the HowTo100M project's methods are well-suited to the kinds of challenges faced when building AI models that work with human instructions captured in videos.
The sheer scale of this dataset offers a crucial advantage over more traditional text-video embedding datasets. Typically, these traditional datasets require painstaking manual captioning, a very time-consuming and expensive process. The approach used with HowTo100M shows a way to get around this, enabling potentially faster and more scalable development of AI models.
HowTo100M covers 15 years of video content, which is a significant amount of information for researchers to leverage. The sheer length of time spanned in the dataset likely exposes it to changes in video production styles, lighting, and general video quality, a potential advantage for creating more robust AI systems. However, the need to analyze the nature of change over the years within the dataset is important to understand if trends in visual representations of tasks and associated narrations have changed.
Naturally, the size and complexity of the dataset also introduce challenges. There are questions about how to best process the data, how to evaluate its quality, and what kinds of computational resources are needed to effectively use it for research purposes.
The HowTo100M project shows the promise of large-scale datasets for AI. However, as with any large-scale project, there are hurdles to be addressed before the full potential of the dataset can be realized. While this dataset is useful as a benchmark, research needs to ensure that AI systems created using such datasets are not only effective but also understandable, allowing us to understand the nature of their predictions and the underlying logic behind them.
7 Underutilized Video Datasets for AI-Driven Content Analysis Projects - AVA Dataset Provides Finely Labeled Data for Human Actions
The AVA dataset offers a detailed look at human actions, providing finely labeled data for 80 different basic visual actions. It's built from 430 movie clips, totaling 162 million action labels, making it a substantial dataset. Researchers can use AVA to explore the complexities of human activity in a variety of settings because it breaks each clip into 3-second intervals, offering a granular view of actions over time. Notably, actions are labeled for each person in a video clip, allowing researchers to understand both the spatial and temporal aspects of human movement. This feature also allows for studying actions that occur together, like "standing while talking," providing a more complete view of human interactions. The videos are drawn from a range of movie genres and countries, suggesting a level of real-world diversity. While AVA provides a valuable foundation for studying action recognition in video, the scale of the dataset and the challenges inherent in analyzing human behavior in real videos raise questions about its overall limitations.
The AVA dataset is primarily focused on recognizing human actions, offering a substantial collection of over 57,000 video clips covering a wide range of activities. This makes it a useful resource for training models to understand dynamic human behavior, which is often complex and varied. Interestingly, AVA doesn't just provide basic labels for actions, but also incorporates detailed temporal annotations. These annotations pinpoint the exact start and end times of each action within a clip, offering crucial context for analysis. The dataset tackles 80 different action categories, spanning from straightforward actions like walking and running to more intricate ones like hugging or dancing. This breadth highlights the inherent challenges in accurately labeling human interactions and behaviors.
AVA leverages a weakly supervised learning strategy, relying on video-level labels for training instead of relying on every single frame's annotation. This approach is practical when dealing with massive datasets and helps reduce the annotation burden. The dataset's temporal resolution is quite impressive, with most actions annotated at a 30 frames per second rate. This level of detail allows researchers to capture the fluidity of human movement accurately. One of the noteworthy challenges AVA presents is the common presence of obstructions. Many actions unfold within busy environments where people or objects frequently block the view of the action itself. This feature makes the dataset valuable for developing models that can handle the kinds of complexities found in real-world settings.
The AVA dataset includes videos captured from various perspectives, using cameras ranging from personal devices to surveillance systems. This broad diversity of camera perspectives enriches the training data by offering different visual contexts for action recognition algorithms to learn from. Researchers have observed that AVA's use of real-world scenarios pushes the limits of action detection beyond what was traditionally found in datasets where many actions were often captured in isolated, controlled environments. The dataset has become a cornerstone for various action recognition competitions and academic challenges, solidifying its position as a standard benchmark for progress in the field.
While AVA has significant strengths, there are limitations to consider. For instance, it predominantly focuses on frequently occurring actions, potentially leading to skewed performance when encountering less common or novel behaviors in practical applications. This bias could be a factor to keep in mind when trying to leverage AVA's dataset for certain research tasks.
7 Underutilized Video Datasets for AI-Driven Content Analysis Projects - YouTube8M Dataset Surpasses Existing Video Collections
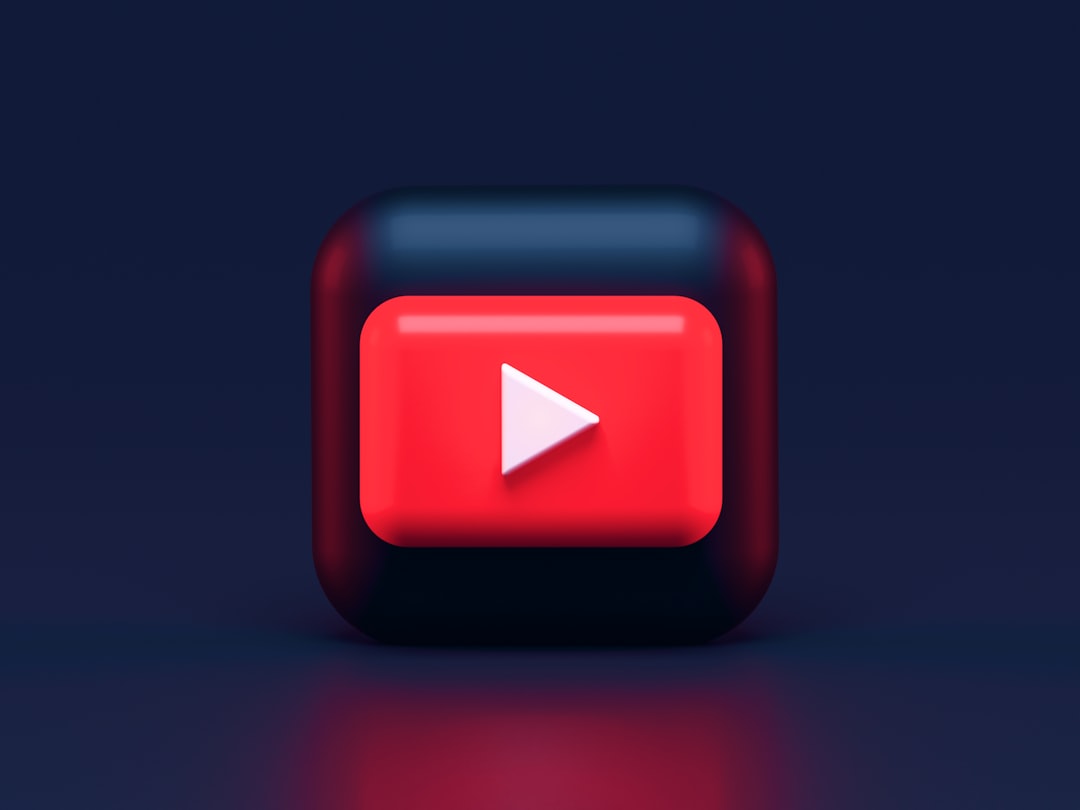
The YouTube8M dataset stands out as a remarkably large collection of labeled video data, containing a staggering 8 million YouTube video IDs and encompassing a vast amount of content, around 500,000 hours. This scale surpasses previous video datasets like Sports1M, which only included a million videos primarily focused on sporting events. YouTube8M's annotations are comprehensive, covering a broad range of 4,800 visual entities. Notably, the dataset has been refined, leading to a smaller but higher-quality version with 5.6 million videos and improved annotations. It's specifically designed to be a useful benchmark for multilabel video classification, a task that was previously lacking a suitably large dataset comparable to ImageNet for images. This dataset, organized into training, validation, and test sets, enables the rapid training of machine learning models on a vast scale, making it valuable for AI research focusing on video content understanding. Given the limited availability of similar comprehensive video datasets, YouTube8M provides a significant resource for advancing research in this area.
YouTube8M is a truly massive dataset, encompassing over 8 million YouTube video IDs, which provides a remarkably diverse look at the breadth of content on the platform. It's more than just a dataset; it's a repository of countless videos, spanning a wide range of topics and genres, making it ideal for studying the nuances of YouTube's content landscape.
This dataset stands out due to its use of a weakly supervised learning approach. Instead of manually labeling each frame, which would be incredibly labor-intensive, it assigns labels at the video level. This allows for efficient processing of the massive dataset without needing an immense workforce to annotate each and every frame.
Each video in the dataset has associated metadata, like titles, descriptions, and tags. This supplementary data can help enhance the performance of models built for tasks like classifying videos or even helping with content moderation efforts.
One fascinating area of research with YouTube8M is understanding the temporal relationships within the videos. Because you have so many videos over time, you can study how themes and topics evolve and change within the YouTube universe itself.
Researchers leverage 3D convolutional neural networks to extract spatial-temporal features within the videos. This approach allows for a more in-depth understanding of the video content compared to older approaches that treated videos as a collection of static images.
The wide array of video genres within YouTube8M presents an excellent chance to do very detailed analyses. You can isolate features of specific types of content, which can facilitate studies on topics like recognizing emotions within videos or understanding how narrative structure differs in varying genres.
However, YouTube8M's size, while offering many advantages, can also present some hurdles. Training models on such a huge dataset can require immense computational resources, making it essential to consider the trade-offs involved in terms of efficiency and resource allocation.
The YouTube8M dataset has sparked various academic challenges and competitions, leading to a collaborative space where researchers can compare approaches and techniques to improve video classification methods. This open, shared approach helps advance the entire field.
Because YouTube is always evolving and changing, there's an inherent possibility for researchers to incorporate updates into their models by incorporating new YouTube content. This dynamic aspect makes YouTube8M especially valuable for building models that remain relevant and effective over time.
While YouTube8M offers enormous potential, its diverse nature also introduces the risk of biases. The balance of various classes within the dataset might not be perfectly even, meaning that some video types might be overrepresented while others are underrepresented. This could lead to biases in the trained models that need to be considered carefully when applying these models to real-world scenarios.
7 Underutilized Video Datasets for AI-Driven Content Analysis Projects - AI-Powered Tagging Enhances Video Content Categorization
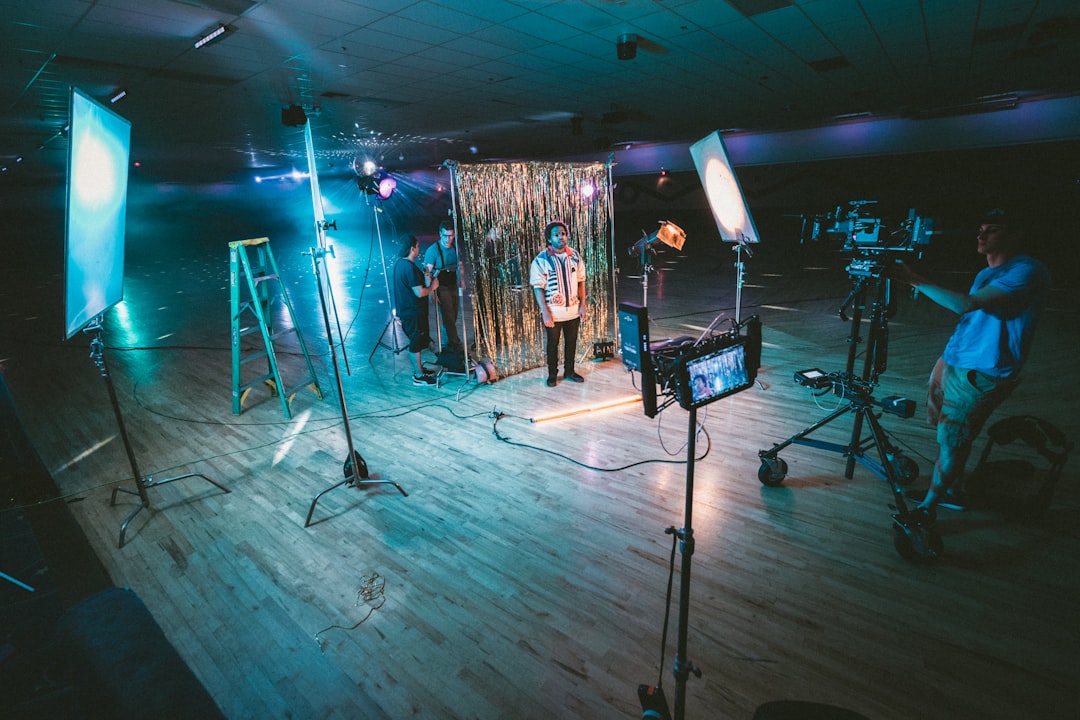
AI-powered tagging is transforming how we categorize video content, making it simpler to manage and discover relevant videos. These systems employ sophisticated algorithms that analyze a video's visual, audio, and textual components to generate highly specific tags. These tags can be based on various aspects, such as the objects shown, the scene depicted, or the overall theme of the video. The increasing need for effective video analysis is driving the adoption of AI-powered tagging, as it streamlines the management process while also boosting user engagement through improved search functionalities. However, while these systems offer significant progress, they also come with challenges regarding the precision and ability to scale to larger and more complex video collections. Real-world scenarios often pose difficulties, as the diversity and richness of content can exceed the limitations of basic tagging approaches. Nevertheless, the development of AI-powered tagging represents a major advancement in video content management, and its integration with underutilized video datasets is poised to further enhance the power of AI-driven content analysis projects.
AI-powered tagging is transforming how we categorize and understand video content. It's essentially automating the process of assigning metadata, making video libraries more organized and easier to search. These AI systems are able to analyze a wide range of video elements, including the visual scene, the audio, and any associated text, allowing for the identification of objects, scenes, and thematic elements with impressive accuracy. This ability to automatically categorize content is particularly important as the demand for video analysis explodes. Experts predict that the video analytics industry could be worth a staggering $50.7 billion by 2032, underscoring the growing significance of these tools. Furthermore, the broader generative AI field, which often relies on these video analysis techniques, is also poised for significant growth, with projections of reaching $11.8 trillion by 2032.
These AI-powered tagging systems often rely on object recognition, creating and assigning relevant metadata tags to videos, which drastically improves search functionality. The algorithms behind these systems are complex, analyzing the context of the multimedia and generating tags that reflect the content. This automated approach streamlines video management, making it easier to organize and retrieve specific video segments. The adoption of AI in video management is increasingly widespread, with many organizations recognizing its benefits in streamlining workflows and ultimately, improving user engagement.
This wave of innovation has led to remarkable advancements, such as Adobe's automated video autotagger, which relies on machine learning to automate the tagging process. It represents a notable step forward, demonstrating how these techniques are becoming integrated into familiar software. In essence, these AI-powered tagging systems are simplifying video content management, becoming essential tools for industries that rely heavily on video content.
The evolution of AI tagging has been fascinating, going from systems that relied heavily on human input to sophisticated systems that convert video into searchable mathematical objects. This transition reveals a significant leap in the technology available for managing and analyzing video. It is an exciting time for video analysis, and we are likely to see further improvements in these systems as research and development continue.
7 Underutilized Video Datasets for AI-Driven Content Analysis Projects - Law Enforcement Utilizes Video Analysis for Crime Prevention
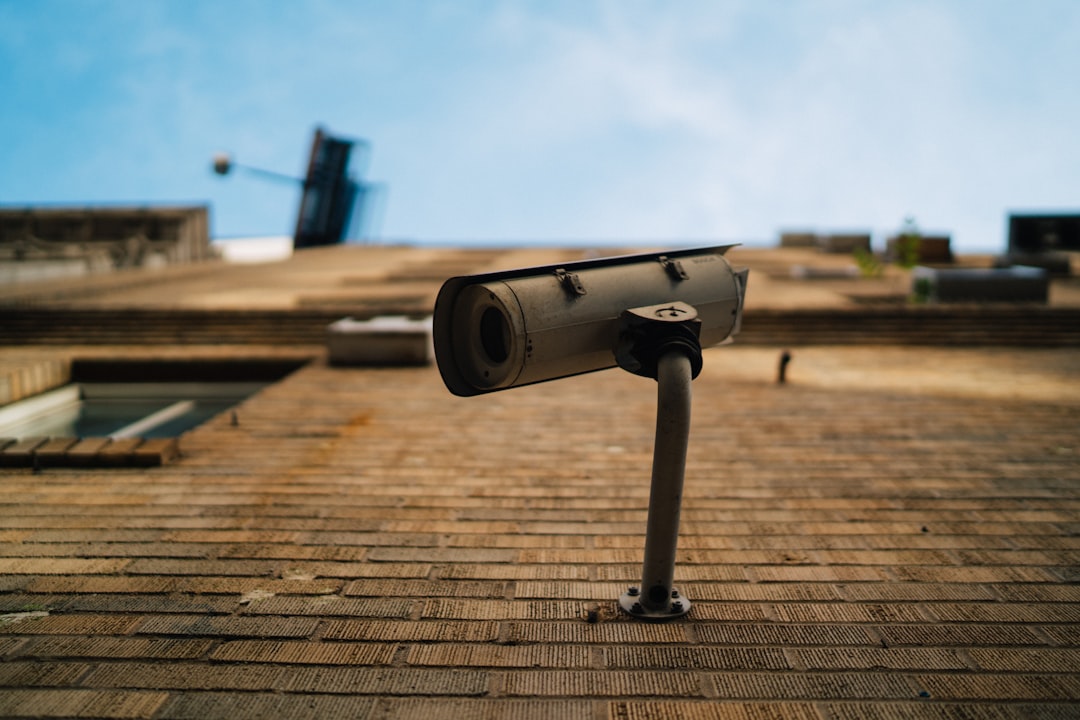
Law enforcement agencies are leveraging video analysis, powered by artificial intelligence, as a tool for crime prevention. This technology allows for the real-time review of various video sources, making it easier for officers to assess situations and identify potential risks. AI algorithms can detect anomalies, like unusual crowd behavior or changes in foot traffic, which may signal impending criminal activity. Furthermore, advanced video analytics, using deep learning, can identify intricate details previously missed by human observation, leading to improved investigation accuracy. The use of AI in Real-Time Crime Centers (RTCCs) is particularly notable, as it enables the rapid processing and analysis of data from multiple sources, allowing for more informed threat assessments and improved operational planning. However, concerns remain about the potential misuse of these technologies and the need to thoroughly train officers in the ethical considerations and limitations of AI-driven systems. Despite ongoing debates, video analysis presents a powerful tool with the potential to enhance public safety, but it must be implemented responsibly and thoughtfully.
Law enforcement is increasingly using video analysis to enhance crime prevention efforts, with some studies showing a potential reduction in crime rates in areas with advanced surveillance systems. This improvement is often attributed to the ability to monitor situations in real-time and respond quickly.
While there are benefits, the use of facial recognition based on video footage, while seemingly quick, can raise concerns due to the potential for inaccuracies. The reliability of such systems varies depending on factors like the quality of the video and even the demographic features of individuals in the recordings, highlighting potential bias issues.
The use of video analytics in areas with higher crime rates seems to be effective. Research suggests strategically placed cameras can act as a deterrent, potentially reducing crime in those areas by a significant amount because criminals may perceive a higher risk of being caught.
AI can further enhance video analysis by helping detect unusual behaviors within groups of people, which could be a way to identify potential threats before they escalate. This predictive capability has shown promise in large events and gatherings, and can be used to provide a better overall picture of the situation, which enhances safety.
The practice of recording and analyzing video data from public spaces brings up crucial ethical considerations. Without regulations and careful considerations of privacy, there’s a risk that the technology’s benefits will be outweighed by the potential for misuse or infringements on people's privacy.
While video analysis is often associated with urban areas, it’s also starting to be adopted in rural areas, often through community partnerships that encourage local businesses to install security systems that can benefit everyone in a region.
It’s surprising that a large portion of video evidence in investigations comes from ordinary individuals who share footage from their phones or home cameras. This growing practice highlights a possible shift toward a community-based approach to safety.
Using real-time video analysis tools allows law enforcement to allocate resources more efficiently. Data-driven approaches have been shown to lead to improved emergency response times, which can be significant during critical incidents.
These tools can also be configured to focus on specific actions or behaviors that are related to particular criminal activities, like drug trafficking or gang-related issues. This type of targeted analysis can increase the effectiveness of investigations and improve the outcomes of cases.
The recent use of drones equipped with video analysis is leading to innovative ways to address crime prevention. These aerial platforms are particularly useful for overseeing large groups or events and can provide a broader view of the situation, which can be essential for quick decision-making. While the use of drones for this purpose has potential benefits, we also need to be aware of the associated potential privacy issues.
7 Underutilized Video Datasets for AI-Driven Content Analysis Projects - Deep-Dive Analysis of Visual and Auditory Elements in Videos
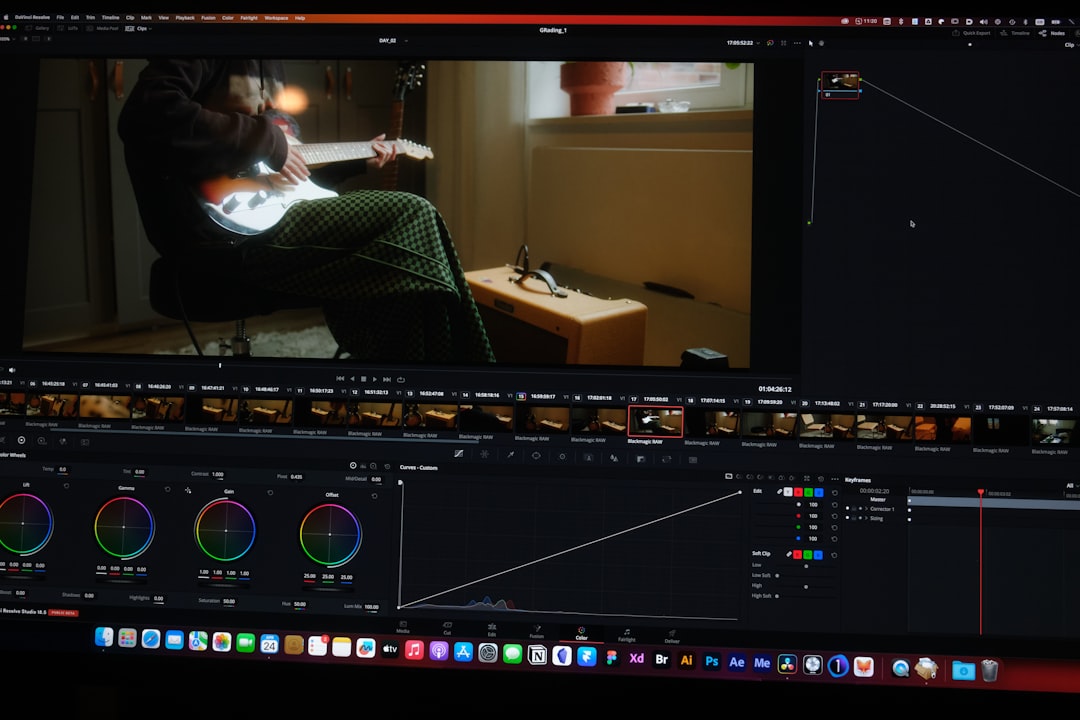
Analyzing the visual and audio components of videos is becoming increasingly sophisticated thanks to artificial intelligence. We can now examine both aspects simultaneously, providing a deeper and more efficient understanding of video content compared to older approaches. AI tools allow for automated tagging based on things like object recognition and scene identification, helping speed up video analysis. Researchers have developed structured ways to approach these tasks, such as with the Visual-Verbal Video Analysis (VVVA) method.
While impressive advancements have been made, challenges remain. Deep learning techniques for tasks like summarizing longer videos or identifying human activities are still somewhat limited. It's an area where further research is needed to refine existing models, allowing them to better manage complexity and variable length videos. Overall, this area of research is constantly evolving and presents opportunities to build AI systems capable of understanding video content in much greater detail.
AI's ability to delve into the intricacies of video content, encompassing both visual and auditory aspects, opens up exciting avenues for research. We can now analyze videos holistically, evaluating the combined impact of visuals and audio on viewers. This capability allows for automated tagging based on features like object and scene recognition, leading to more streamlined and effective analysis of video content.
The Visual-Verbal Video Analysis (VVVA) method provides a structured approach to extract and analyze video data, offering researchers a framework to organize and interpret the multifaceted information present in videos. This area has shown particular promise in the use of deep learning techniques that extract key visual features, allowing us to build more robust models.
While there's growing interest in summarizing videos, current deep learning techniques often struggle with longer videos, which presents a major research challenge. Unconstrained video analytics, on the other hand, seeks to address the challenges inherent in real-world videos, which can vary greatly in length and environment. Understanding human activity is crucial in video analysis, with the focus on improving accuracy in recognizing various actions across these diverse settings.
One area of focus has been on improving the foundational tools for video analysis. VideoPrism, for instance, is a visual encoder aimed at improving tasks like classification, retrieval, and localization in videos. Researchers have been actively developing deep learning libraries like PyTorchVideo, which provide foundational support for video-based research across a spectrum of projects.
These advancements in video analysis use deep learning to automate and refine the processes of extracting and evaluating data from videos, paving the way for more comprehensive insights. The use of AI in video processing offers a powerful approach to extract information from videos, but its effectiveness is tied to the nature of the models used to perform these analyses. We need to continue to research and refine techniques for understanding how different types of visual and audio information impact our ability to understand these increasingly sophisticated models. While there has been progress in automating these tasks, significant research remains on how to more efficiently address biases present in models and develop more interpretable models.
Analyze any video with AI. Uncover insights, transcripts, and more in seconds. (Get started for free)
More Posts from whatsinmy.video: