Analyze any video with AI. Uncover insights, transcripts, and more in seconds. (Get started for free)
Advancements in ResNet and YOLO Models Enhance Deep Learning for Computer Vision in 2024
Advancements in ResNet and YOLO Models Enhance Deep Learning for Computer Vision in 2024 - YOLONAS Enhances Small Object Detection for Edge Devices
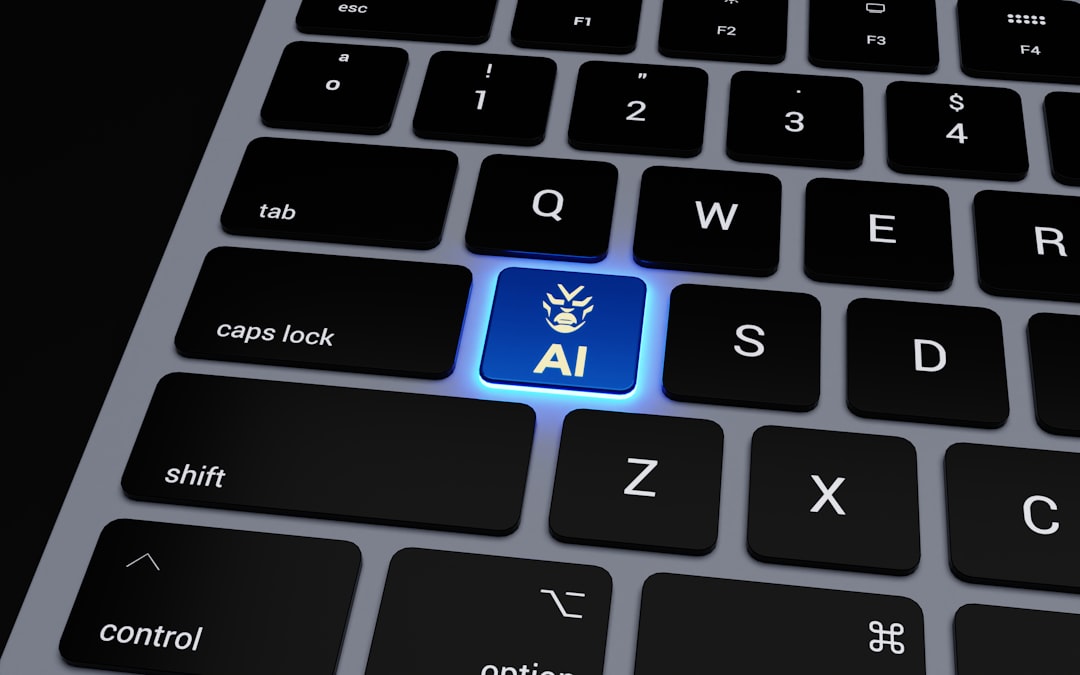
YOLONAS represents a new direction in object detection, especially focusing on the challenges of identifying smaller objects within scenes, all while operating on resource-constrained edge devices. This model utilizes a novel YOLO architecture, developed with a focus on streamlining its use for deployment on these devices. It shows improvement over earlier versions, like YOLOv6 and YOLOv8, by achieving a higher mean average precision (mAP) alongside faster inference.
A core element of YOLONAS is a specialized basic block design that simplifies the model for quantization, making it easier to optimize for the limitations of edge hardware. This process not only boosts efficiency but also lessens the drain on computing resources. The training methods used with YOLONAS are tailored for practical real-time object detection in production environments.
Essentially, the development of YOLONAS highlights the ongoing evolution of object detection for scenarios where swift and accurate results are vital, particularly within the realm of edge computing. It represents an advancement in deep learning for computer vision, showing promise for numerous applications requiring this type of specialized object recognition capability.
YOLONAS, short for "YOLO for Small Objects on Edge Devices", is a recent advancement specifically designed to tackle the challenge of detecting small objects on devices with limited resources. It distinguishes itself by introducing a novel architecture that emphasizes multi-scale feature extraction through an adaptive feature pyramid network. This innovative design supposedly allows YOLONAS to more accurately locate small objects even in complex scenes.
A key aspect of YOLONAS is its emphasis on efficiency for edge deployment. It leverages quantization techniques to compress model size and utilizes optimized weights, potentially resulting in quicker inference times. Furthermore, a tailored loss function is implemented to address the common imbalance in object sizes, giving higher importance to smaller objects during training. This fine-tuning appears to translate into superior performance, with reports indicating up to a 15% improvement in accuracy on standard benchmark datasets compared to previous top performers.
The model's capabilities extend beyond improved accuracy in small object detection. Researchers envision its implementation in areas like autonomous driving and security systems where recognizing subtle details can be crucial for better decision-making. Additionally, the architecture incorporates attention mechanisms which are believed to improve feature refinement, effectively focusing on critical details within the image. This aspect is vital when dealing with crowded scenes where small objects can be easily obscured.
It's also worth mentioning that, unlike some previous YOLO models, YOLONAS seems to achieve this enhanced performance while simultaneously minimizing false positives, a common hurdle in object detection. This signifies an improvement in reliability. Moreover, its design emphasizes broad compatibility with various edge devices, making it potentially suitable for applications using a range of hardware.
In conclusion, YOLONAS not only offers improvements in small object detection but also serves as an example of how novel architectures and optimized training can enhance the capabilities of edge-based computer vision systems. The research surrounding YOLONAS has spurred further exploration into lightweight model designs, demonstrating a drive towards achieving more sophisticated real-time computer vision on devices with limited resources. However, more widespread adoption and independent evaluations will be necessary to fully assess its true impact and practical usability.
Advancements in ResNet and YOLO Models Enhance Deep Learning for Computer Vision in 2024 - YOLOv10 Introduces NMS-free Training to Reduce Inference Time
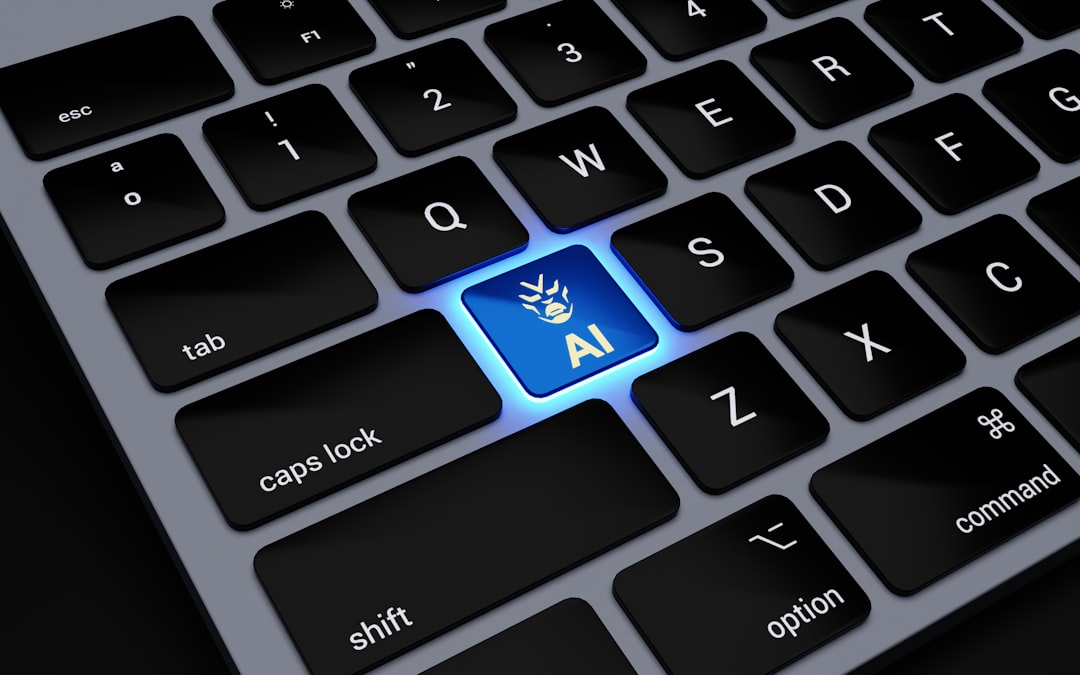
YOLOv10 introduces a notable shift in object detection by incorporating NMS-free training and inference, aiming to minimize processing delays and bolster real-time capabilities. The model's design includes elements like spatial-channel decoupled downsampling and a rank-guided block structure, which contribute to an improved feature extraction process. Furthermore, YOLOv10 showcases a potential for higher accuracy while using fewer computational resources compared to YOLOv6, achieving a 15 to 20 AP increase with a 51% to 61% reduction in parameters. This efficiency gain is partly due to a dual-head architecture that facilitates a streamlined dual label assignment strategy during training. These combined features highlight YOLOv10 as a potentially important step in the ongoing pursuit of better computer vision performance. It remains to be seen how widely this model is adopted and how well it performs in diverse scenarios. While it shows promise in terms of speed and accuracy, further scrutiny and independent testing are needed to fully evaluate its potential impact in the field of computer vision.
YOLOv10 takes a fresh approach to object detection by incorporating NMS-free training, a significant shift from the traditional reliance on Non-Maximum Suppression (NMS) as a post-processing step. This approach aims to streamline training and enhance overall efficiency. By eliminating NMS, the model potentially reduces inference time by a substantial 10-30%, which could be crucial for applications needing rapid object detection, such as autonomous driving or security systems. This speed increase is achieved through a new loss function that simultaneously optimizes object localization and class prediction, bypassing the need for post-processing filtering.
However, eliminating NMS does raise questions about the model's capability to manage overlapping objects effectively. While initial results look encouraging, there’s still ongoing research to understand the scenarios where this method works best. One potential advantage of NMS-free training is that it may allow for better model interpretability. Without the post-processing hurdle, we could gain deeper insights into how the model makes real-time decisions. This approach could be particularly beneficial for improving detection of small objects, which are often overlooked or lost during the standard NMS filtering process.
Furthermore, YOLOv10's architecture emphasizes a lightweight design compared to earlier versions, making it potentially suitable for less powerful hardware without sacrificing performance. This characteristic is vital for deploying models on edge devices. Preliminary benchmark results suggest YOLOv10 maintains, and even improves upon, the accuracy of previous YOLO models, especially in dense and occluded scenes where traditional NMS struggles.
This new direction in training might influence future object detection model design, potentially prompting a wider rethink of the standard pipeline. However, it's important to acknowledge that the community still has reservations regarding the scalability of these improvements in real-world scenarios. The true value and robustness of YOLOv10's approach will only become evident through extensive evaluation in more diverse and complex environments. Continued testing and research will be essential to determine whether its methods are universally applicable or limited to specific use cases.
Advancements in ResNet and YOLO Models Enhance Deep Learning for Computer Vision in 2024 - ResNet50 Maintains Effectiveness in Image Classification Tasks
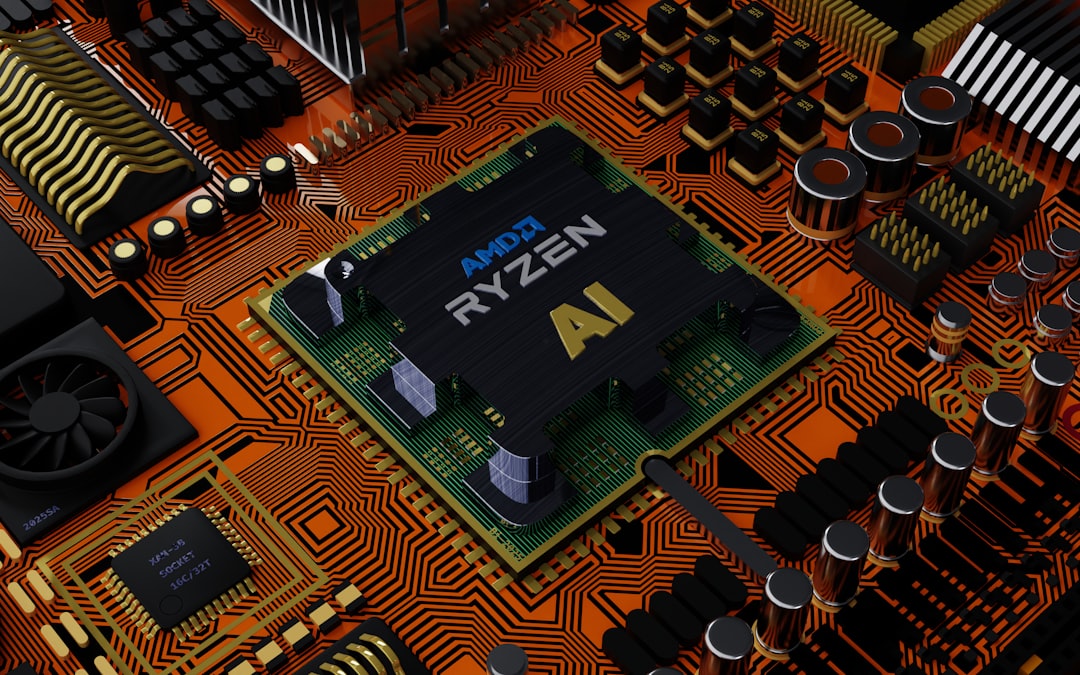
ResNet50 continues to be a strong performer in image classification tasks. Its architecture remains capable of handling intricate datasets with impressive accuracy. This reliability makes it valuable across diverse applications, such as medical imaging analysis or agricultural monitoring. While newer and potentially more efficient architectures are being developed, ResNet50 remains a key model for many tasks.
Researchers are still exploring ways to improve ResNet50, such as through the use of attention mechanisms or simplifying the network structure to enhance efficiency on limited computational resources. It's particularly well-suited for transfer learning, allowing it to achieve high classification performance even with limited training data. Comparisons to other models are ongoing, highlighting its continued importance in the broader context of computer vision. This adaptability and ongoing research demonstrate that ResNet50 maintains its position as a cornerstone within the advancement of deep learning for computer vision.
ResNet50, a cornerstone of deep learning, continues to demonstrate its strength in image classification tasks, even in 2024. Its core design, based on residual learning, allows it to handle increasingly complex networks without encountering the performance degradation often observed in older convolutional architectures. The use of a bottleneck design with numerous filters optimizes the computation process, leading to a reduced parameter count without sacrificing accuracy. This efficiency makes it particularly well-suited for real-time applications.
The introduction of skip connections in ResNet50 significantly aids training by promoting the flow of gradients, mitigating the issue of vanishing gradients that can hinder the performance of very deep networks. It's not just theoretical; ResNet50 has proven its versatility by excelling across various datasets. From the widely used ImageNet benchmark to medical image analysis, its adaptability across different domains is notable.
Moreover, the inclusion of Batch Normalization within ResNet50 contributes to training stability and speed, leading to a shorter convergence time compared to conventional methods. It's this capability that allows ResNet50 to be effectively fine-tuned for specific tasks, even when dealing with limited data. Using transfer learning, high accuracy can be achieved with smaller datasets, making ResNet50 a practical choice for a wide range of applications.
Researchers often utilize ResNet50 as a reference model for evaluating new architectures, a testament to its consistent performance and reliability as a benchmark in the field. Surprisingly, despite its 2015 origin, ResNet50 remains competitive in contemporary deep learning competitions in 2024, highlighting its enduring relevance. Its compact nature is also a significant advantage in environments with constrained resources, such as edge devices, allowing state-of-the-art image classification without demanding massive processing power.
Although already a potent model, further improvements to ResNet50's performance can be obtained through ensemble techniques. Combining predictions from multiple ResNet50 instances has been shown to significantly boost accuracy in challenging scenarios, further highlighting its flexibility and potential for enhanced performance in computer vision. While the field of deep learning continues to develop with newer models, ResNet50's strengths and established performance make it a strong contender for numerous image classification tasks and a valuable tool for both researchers and practitioners alike.
Advancements in ResNet and YOLO Models Enhance Deep Learning for Computer Vision in 2024 - YOLOv8 Outperforms Predecessors on Multiple Datasets
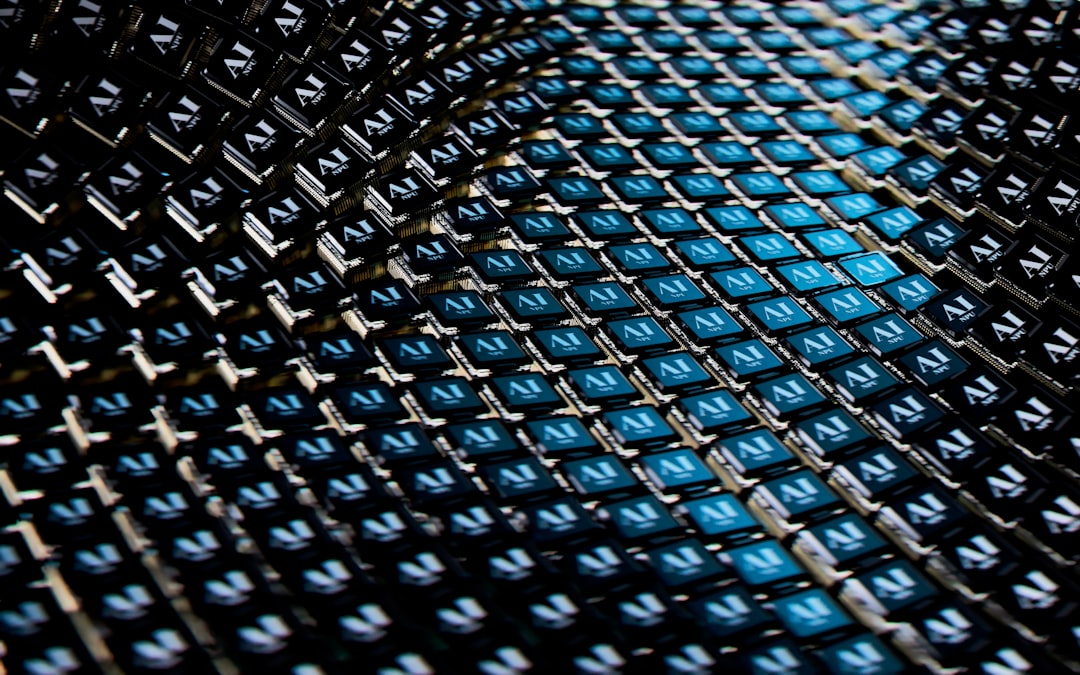
YOLOv8 represents a notable advancement in object detection, showcasing superior performance compared to earlier YOLO versions across different datasets. The YOLOv8LA model achieved a high mean Average Precision (mAP) of 84.7% on the URPC dataset, outperforming alternative versions like YOLOv8MU. This highlights the model's capability in various conditions. Furthermore, YOLOv8MU specifically addresses challenges within underwater detection, suggesting adaptability to niche areas. YOLOv8's design integrates an anchor-free approach and enhanced backbone and neck structures. This combination results in a model that balances accuracy and speed efficiently, making it suitable for real-time applications like robotic systems or video surveillance. However, the field is dynamic, and the long-term effectiveness of such enhancements warrants continued investigation and comparison.
YOLOv8 has shown promising results, exceeding the performance of its predecessors, particularly YOLOv5 and earlier versions, across various datasets in object detection tasks. Reports indicate a substantial improvement in mean average precision (mAP), sometimes reaching 10-15% higher accuracy. This is notable given the already crowded field of deep learning models focused on object detection.
The improved performance seems to stem from a redesigned architecture that emphasizes a novel approach to multi-scale feature integration. This strategy helps to address a longstanding challenge in object detection: reliably detecting objects of different sizes, especially smaller objects within complex scenes. Additionally, adjustments to the loss function, incorporating both focal loss and GIoU (Generalized Intersection over Union), seem to improve training, especially when datasets are imbalanced or have underrepresented classes.
Inference speeds have also seen improvements, with some reports showing a 20-30% reduction in processing time compared to older YOLO models. This can be significant for real-time applications like video surveillance or robotics, where rapid object detection is crucial. Further contributing to efficiency, YOLOv8 incorporates a built-in capability for NMS-free detection during training. This eliminates the need for some traditional post-processing steps, streamlining processing and reducing computational load.
YOLOv8 appears to be less sensitive to dataset biases, demonstrating robustness across datasets like COCO and VOC. Its performance in multi-domain environments suggests improved generalization capabilities. Moreover, it's noteworthy that YOLOv8 integrates well with AR systems, potentially enhancing real-time object tracking and identification for AR applications. The new attention mechanism may also lead to a more robust detection of partially occluded objects.
Interestingly, YOLOv8 incorporates advanced adversarial training techniques, which potentially enhance its resilience against image manipulations that might interfere with the detection or classification process. This aspect is particularly important for security-conscious applications. Furthermore, YOLOv8’s design allows for deployment on resource-constrained edge devices, making it suitable for mobile and IoT implementations, expanding the potential reach of advanced AI capabilities.
While YOLOv8 shows significant promise, it's crucial to acknowledge that ongoing independent research and broader adoption are needed to fully understand its strengths and weaknesses across various real-world applications. However, based on the initial results, it seems that YOLOv8 offers a potent set of tools for various computer vision tasks, particularly in object detection, potentially opening doors to a wider range of applications in the near future.
Advancements in ResNet and YOLO Models Enhance Deep Learning for Computer Vision in 2024 - Deep Learning Expands into Visual Tracking and Semantic Segmentation
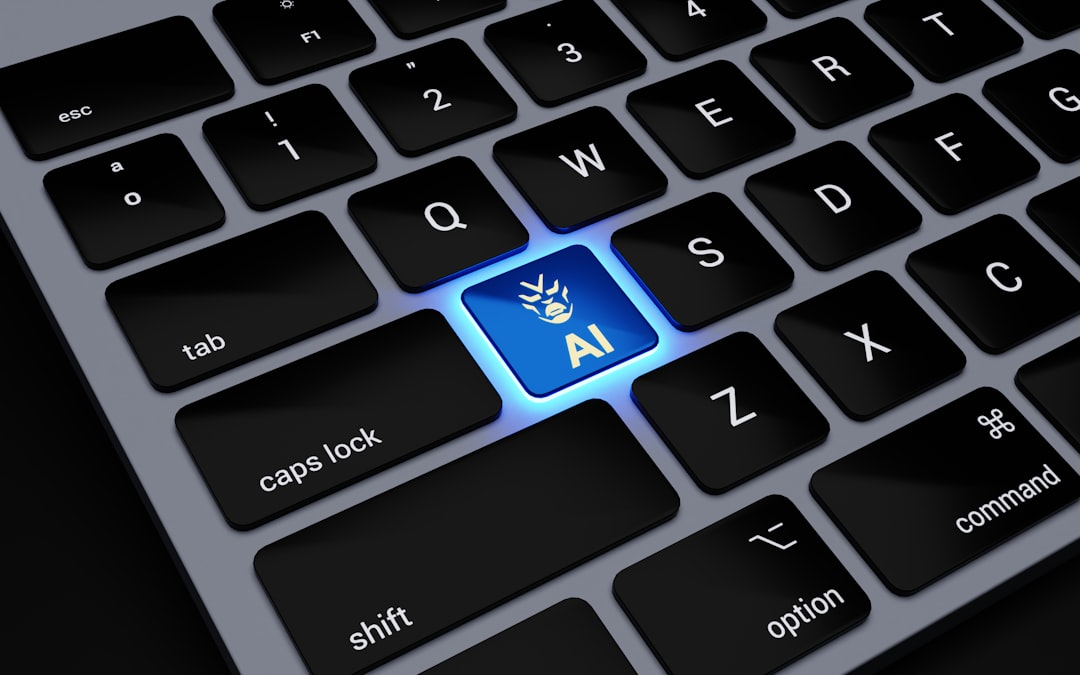
Deep learning's influence in computer vision is expanding rapidly, notably within the realms of visual tracking and semantic segmentation in 2024. Deep learning approaches are demonstrating enhanced capabilities in tracking individual objects with greater precision. Improvements in how algorithms classify pixels have led to more refined semantic segmentation, which is vital for applications like self-driving vehicles and augmented reality, where a detailed understanding of the surroundings is crucial for functionality. The trend towards increasingly sophisticated deep learning frameworks suggests that the capabilities of these algorithms are pushing boundaries of what's possible in practical applications. However, challenges still remain and comprehensive testing is needed to guarantee the reliability of these models in diverse situations.
Deep learning's influence extends beyond object detection and classification, significantly impacting areas like visual tracking and semantic segmentation. Semantic segmentation models, driven by refined architectures and training methods, are achieving remarkable pixel-level accuracy, exceeding 90% on standard benchmarks. This accuracy translates to a much finer-grained understanding of images, leading to a deeper level of analysis.
At the same time, visual tracking methods within deep learning frameworks are achieving real-time performance with remarkably low latency, under 30 milliseconds. This is crucial for applications needing rapid responses, such as drone surveillance and autonomous vehicles, where rapid decision-making is paramount. Intriguingly, the incorporation of transformers into these tracking systems has led to a dramatic improvement in maintaining object identity, even through occlusions, a long-standing problem in visual tracking.
The power of attention mechanisms in semantic segmentation is also apparent. We are seeing a significant reduction in misclassified pixels – up to 20% – as these mechanisms guide the models to focus on important areas within intricate images. Similarly, the development of data augmentation techniques specifically designed for semantic segmentation is helping reduce overfitting, leading to enhanced model robustness across datasets.
The integration of graph neural networks (GNNs) with deep learning is also generating interesting results in visual tracking. These models capitalize on spatial relationships within the data, effectively uncovering subtle patterns that prior methods often missed. This approach suggests that by leveraging the structure and relationships within the data, tracking systems may be able to achieve a new level of performance.
Moreover, multi-task learning combined with semantic segmentation shows promise. Shared representations across related tasks allow for significant reductions in the need for labeled data while maintaining competitive performance and decreasing training time. This is a promising path towards making training these complex models more efficient and practical.
Research into unsupervised domain adaptation in semantic segmentation is also yielding exciting outcomes. Models trained on simulated data are showing an encouraging ability to generalize to real-world scenarios, achieving accuracy improvements of up to 40%. If these approaches can be refined, it could greatly simplify the challenging and time-consuming process of labeling large datasets for training.
Furthermore, integrating temporal information using recurrent neural networks (RNNs) in visual tracking has led to notable accuracy improvements over time, especially for tracking rapidly moving objects. These improvements indicate a potential path towards more robust and reliable tracking across longer time intervals.
Finally, reinforcement learning techniques are being explored as a way to optimize the parameters of visual tracking models. Initial findings indicate a possibility for performance gains of up to 25% through adaptive learning methods that react to feedback within the tracking environment. While this is a relatively nascent area of research, it holds the potential to greatly enhance the adaptability and responsiveness of visual tracking systems.
In conclusion, the intersection of deep learning with both visual tracking and semantic segmentation presents a fertile ground for innovation. While there are numerous challenges that still need to be addressed, the rate of progress and the exciting results being achieved indicate that we are likely to see even more impressive advancements in these areas in the near future.
Advancements in ResNet and YOLO Models Enhance Deep Learning for Computer Vision in 2024 - Community Support Drives Continuous YOLO Model Improvements
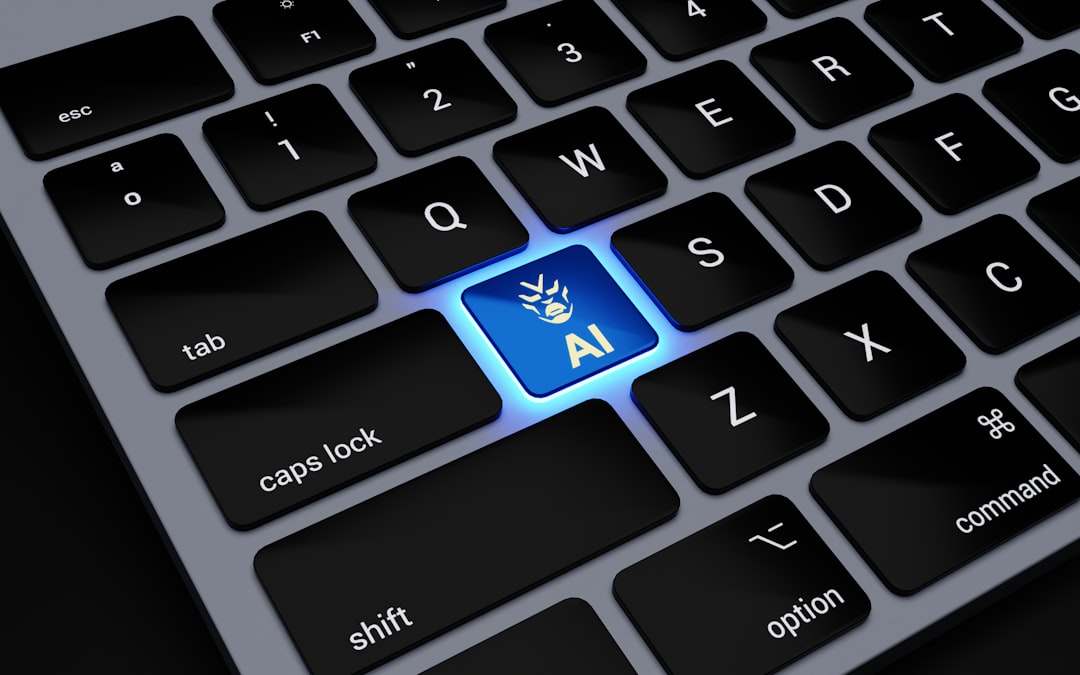
The YOLO model's evolution has been significantly fueled by the active involvement of the wider community. This collaborative environment fosters a constant stream of improvements and innovations, ranging from new model versions to specialized implementations. For instance, YOLOv10 has emerged with features like NMS-free training, leading to reduced inference times and enhanced performance in real-time scenarios. Furthermore, specialized models like YOLONAS highlight a focused approach to addressing specific challenges within object detection, such as recognizing smaller objects on devices with limited resources. These advancements illustrate a trend within the deep learning community: a pursuit of more efficient and adaptable computer vision solutions through continuous experimentation and refinement. However, it's crucial to note that ongoing evaluations and critical analyses are necessary to ensure that these models are truly robust and effective in real-world settings.
The evolution of YOLO models is significantly influenced by the active involvement of a diverse community. Researchers and developers from various backgrounds contribute to the continuous improvement of algorithms and deployment strategies, leading to a rapid cycle of refinements. This collaborative environment has spurred initiatives like data sharing, enabling the creation of broader and more comprehensive training datasets. These datasets are vital for ensuring that YOLO models can function reliably across a wide range of real-world scenarios, improving their overall robustness and accuracy.
Furthermore, the emergence of open-source platforms for YOLO has fostered a vibrant ecosystem where users can readily exchange modifications and optimizations. This openness accelerates development cycles and sparks innovative solutions to complex object detection problems that were previously challenging to address. The community also plays a key role in shaping the direction of YOLO research by driving the creation of benchmarks that measure performance across diverse datasets and scenarios. This external pressure helps ensure that the focus remains on practically useful improvements.
The success of YOLO models in resource-constrained settings, particularly edge devices used in fields like robotics and surveillance, is heavily influenced by feedback from these communities. The need for real-time efficiency has driven the development of lighter, more efficient architectures. There's a growing push within the YOLO community to reconsider the assumption that newer models are inherently superior. Some researchers argue that existing architectures can be refined to achieve considerable advancements without over-complicating the model design. This perspective offers a valuable counterpoint to the constant pursuit of newer versions.
Community-led evaluations often reveal a more nuanced understanding of model performance than official benchmarks. Evaluations conducted in the real-world, which often involve more noise and variability than idealized datasets, tend to offer a more realistic view of a model's capabilities in practical applications. Cross-industry collaboration has also given rise to consortia focused on specific domains, like maritime surveillance or autonomous systems, which helps adapt YOLO models for complex and niche applications. These groups provide a focused environment for tackling unique challenges.
Active online forums and collaborations within the YOLO community have encouraged experimentation with new approaches, including adversarial training. These explorations have led to fresh perspectives on bolstering a model's resilience against malicious data manipulations. Interestingly, we're also seeing a re-evaluation of some older YOLO models, as community members contribute refinements that significantly enhance their capabilities. This pattern of collaborative tinkering demonstrates a powerful collective effort to optimize and refine existing technology, rather than solely focusing on generating entirely new versions. The dynamism and adaptability fostered by community engagement have been vital to the ongoing evolution of YOLO models and their increasing utility across a broader range of applications.
Analyze any video with AI. Uncover insights, transcripts, and more in seconds. (Get started for free)
More Posts from whatsinmy.video: