AI-Generated Faces Analyzing Video Deepfakes Created by ThisPersonDoesNotExist
AI-Generated Faces Analyzing Video Deepfakes Created by ThisPersonDoesNotExist - AI-Generated Faces Blur Lines Between Real and Artificial
The proliferation of AI-generated faces is blurring the boundaries between authentic and fabricated identities, pushing the notion of truth in the digital realm to a new frontier. These synthetic faces are becoming remarkably lifelike, often fooling individuals into believing they are interacting with real people. This phenomenon has a substantial impact on online interactions, especially within social media platforms, where fabricated personas can readily blend into the crowd. The increasing sophistication of deepfakes, driven by advanced AI, not only poses a challenge to discerning real from artificial but also creates a crisis of trust. The ethical and legal landscapes are struggling to catch up with this technology, highlighting the need for a comprehensive understanding of its ramifications. The consequences of this artificially created reality extend beyond mere visual deception, questioning our fundamental notions of identity, interaction, and information credibility in the age of AI. The difficulty in distinguishing between real and AI-generated faces raises critical questions about the future of online interactions and how we navigate an increasingly blurred reality.
The emergence of AI-generated faces, often powered by Generative Adversarial Networks (GANs), has fundamentally altered our perception of authenticity. These algorithms continuously refine their creations, pushing the boundaries of realism until the difference between AI-generated and real faces becomes nearly imperceptible to the human eye. It's fascinating how these synthetic faces can sometimes trigger genuine emotional responses in viewers, suggesting that we might unknowingly form personal connections and biases towards these artificial personas, despite knowing they are not real.
Beyond just faces, AI can generate entire identities, complete with fabricated backgrounds, creating individuals who may never have existed. This raises questions for technologies that rely on facial recognition, as these AI-generated faces can potentially bypass security measures designed to identify human subjects, making them susceptible to manipulation. The implications for security are substantial.
The capacity to produce such realistic faces brings to the forefront a range of ethical concerns, primarily the potential for the creation of misleading content and the subsequent blurring of the lines between truthful information and deliberate deception in various media forms. It's intriguing how these algorithms incorporate intricate details like subtle skin textures and lighting nuances, often drawing upon vast datasets of real human faces, thereby increasing the authenticity of the synthetic output.
There's a curious psychological aspect related to this technology: the exploration of the "uncanny valley" phenomenon. As AI-generated faces reach a point of near-human realism, they can trigger discomfort or uneasiness in some observers. As the technology matures, it's not surprising that the concept of identity and representation is undergoing a transformation. These AI-created faces can be used in various fields, including art, gaming, and social media, raising issues of consent and personal rights, as these synthetic personas could potentially represent or replace real individuals without their permission.
The continual evolution of this technology suggests that future iterations will not only create increasingly realistic faces, but may also enable customization based on individual preferences. This opens up a new set of ethical considerations, particularly regarding representation and ensuring respect for individuals. The implications of these technologies are profound, and warrant careful consideration moving forward.
AI-Generated Faces Analyzing Video Deepfakes Created by ThisPersonDoesNotExist - ThisPersonDoesNotExist Pushes Boundaries of Synthetic Imagery
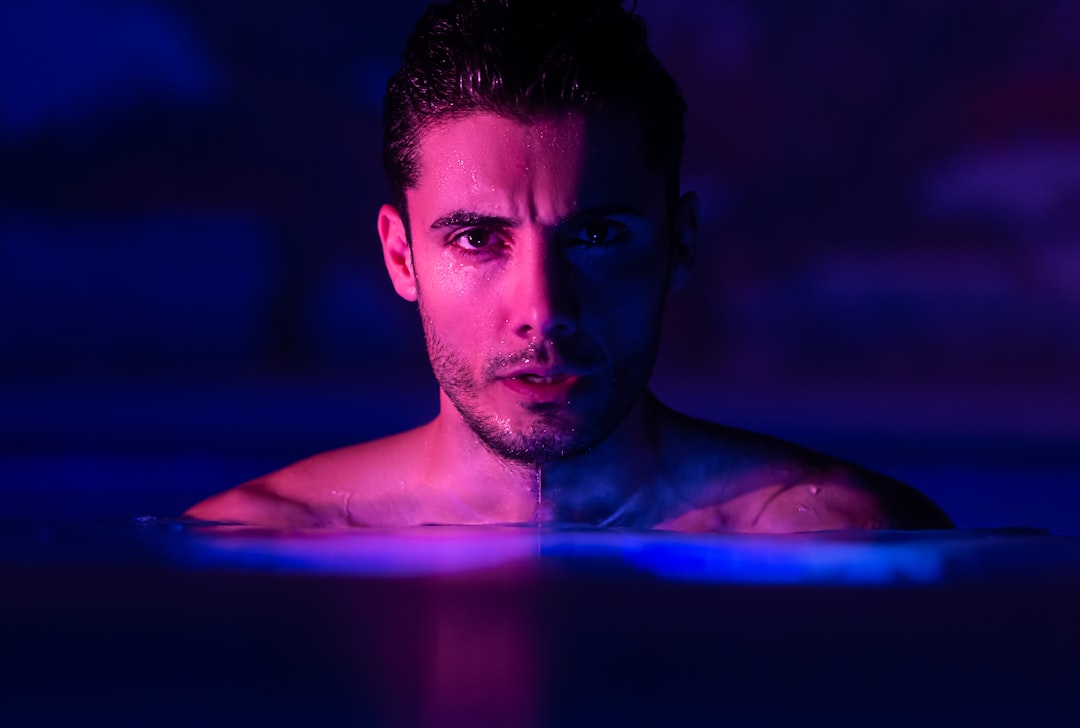
ThisPersonDoesNotExist exemplifies the cutting edge of synthetic imagery creation, leveraging powerful artificial intelligence techniques like generative adversarial networks (GANs). The website's core function is to generate entirely fictional, yet incredibly realistic, human faces. Each time the page is refreshed, a new, never-before-seen individual is displayed, showcasing the impressive power of AI in generating synthetic visuals. This technology pushes the boundaries of what's possible in creating artificial imagery, forcing a confrontation with the blurring line between real and artificial, especially when it comes to representing human faces.
While this technology offers exciting potential for creativity and expression, it also compels us to consider the implications for society. Questions about identity and our evolving understanding of representation in a digital landscape are becoming more urgent as AI-generated faces become increasingly convincing. As these synthetic creations grow more sophisticated, they could significantly impact how we interact and communicate online, raising important ethical and social discussions surrounding the responsible use of this technology. The future of synthetic faces and their impact on our perceptions of authenticity will be something to watch closely.
ThisPersonDoesNotExist, a website that generates remarkably realistic images of fictional people, exemplifies the rapid advancements in AI-generated imagery. It leverages the power of Generative Adversarial Networks (GANs), a type of AI architecture where two neural networks compete—one generating images and the other evaluating their authenticity. This constant back-and-forth refines the generated images until they become nearly indistinguishable from real photographs.
Beyond simple visual realism, these AI-created faces often carry inferred personality traits, subtly influenced by features like age, expression, and ethnicity. Viewers are likely to project characteristics onto these artificial individuals, creating a fascinating study in how human perception responds to synthesized faces. Research suggests that certain facial features, like symmetry and youthfulness, are intrinsically more appealing, and the GANs are designed to exploit these biases, making the generated faces more engaging.
The AI systems behind these faces are trained on vast datasets of human images, capturing a diverse spectrum of facial features and appearances, contributing to the impressive realism of the output. However, while the images are incredibly convincing, a keen eye might still detect subtle anomalies. Genuine skin possesses unique identifiers like pore and follicle patterns that are currently absent in the AI-generated images. These are becoming progressively harder to differentiate as the technology advances, presenting a fascinating challenge for researchers.
The accessibility of this technology has significant implications for the spread of misinformation. AI-generated faces could be seamlessly integrated into false news articles or fabricated social media profiles, complicating the task of verifying information and identifying authentic sources. Moreover, the psychological phenomenon of the "uncanny valley" comes into play. As these AI faces achieve near-human realism, they can trigger a sense of unease or discomfort in some viewers, particularly when inconsistencies in subtle details reveal their synthetic nature.
As the capability to customize synthetic faces progresses, engineers and developers face ethical quandaries regarding representation. There's a risk that the potential for manipulation could erase or unintentionally distort real identities. Further, the ability to produce convincing synthetic faces also presents substantial security concerns. These faces have the potential to bypass facial recognition systems, exposing vulnerabilities in security protocols that are not designed to differentiate between a real individual and an entirely fabricated one.
This constant evolution of AI-generated faces compels us to seek new ways to identify synthetic content and protect against the potential misuse of this technology. The development of robust detection methods is becoming increasingly crucial as the boundary between real and artificial continues to blur, presenting both a technical and regulatory challenge in the years to come.
AI-Generated Faces Analyzing Video Deepfakes Created by ThisPersonDoesNotExist - Deepfake Detection Challenges in the Age of Advanced AI
The continuous evolution of artificial intelligence has led to increasingly sophisticated deepfake technology, posing a significant challenge to our ability to distinguish between genuine and fabricated content. The creation of highly realistic deepfakes blurs the line between reality and deception, impacting the reliability of video and audio as evidence, particularly within sensitive domains like politics and national security where trust in information is crucial. The subtle alterations employed in generating deepfakes often exploit human cognitive biases, making it difficult to discern manipulation and potentially eroding our confidence in visual and auditory media. While deepfake detection methods are emerging, current techniques largely concentrate on identifying visual and video alterations, with the intricacies of manipulated audio often overlooked. This indicates a need for more comprehensive and adaptable detection strategies. To mitigate the potential risks associated with deepfakes, continued development of innovative detection methods paired with enhanced public awareness regarding the technology is paramount in navigating this new era of synthetic media and protecting against the potential for malicious intent and misinformation.
The rapid evolution of deepfake technology has created a constant back-and-forth between those creating these synthetic videos and researchers attempting to detect them. It's become an ongoing struggle, with every advancement in deepfake generation met with new efforts to counter it.
Researchers have found that audio deepfakes can often be harder to detect than their visual counterparts. The subtle nuances of human speech and voice modulation are difficult to replicate convincingly, adding another dimension to the challenge of synthetic identities.
The effectiveness of current deepfake detection methods depends greatly on the quality and source of the deepfake itself. Even the most advanced detection techniques can struggle with well-crafted synthetic media, highlighting the need for constant refinement and improvement.
The problem of detecting deepfakes is made more complex by the fact that some of the most sophisticated deepfake generation methods are specifically designed to hinder detection. Techniques like manipulating pixel-level details or mimicking subtle facial expressions can make it exceptionally difficult to pinpoint manipulation.
Deepfake detection research often utilizes machine learning, but this approach can be hindered by the limitations of the training data. If a detection model is trained primarily on images of one specific population, it may struggle with deepfakes generated from a different group.
Intriguingly, some deepfake detection methods leverage the same algorithms used to create synthetic images. By using adversarial training, these methods try to identify patterns specific to artificial generation.
The issue of "overfitting" in deepfake detection can lead to models that perform remarkably well on the datasets they're trained on but falter when encountering real-world scenarios. This often happens when the model faces variations it wasn't exposed to during the training phase.
Global concerns about misinformation are accelerating the development of new deepfake detection tools. Governments and academic institutions are collaborating to create standardized detection benchmarks in an attempt to minimize the potential for social disruption caused by deepfakes.
While deepfakes are often associated with malicious intent, they also have potential applications in fields like filmmaking, entertainment, and education. This creates a dilemma about their ethical use and necessitates a more complex approach to their development.
Exposure to deepfakes can have a negative effect on how we perceive visual media. This can lead to a general distrust of any visual information, as viewers may start to question the authenticity of what they see even in real, unaltered content.
AI-Generated Faces Analyzing Video Deepfakes Created by ThisPersonDoesNotExist - Analyzing Video Authenticity Using Machine Learning Algorithms
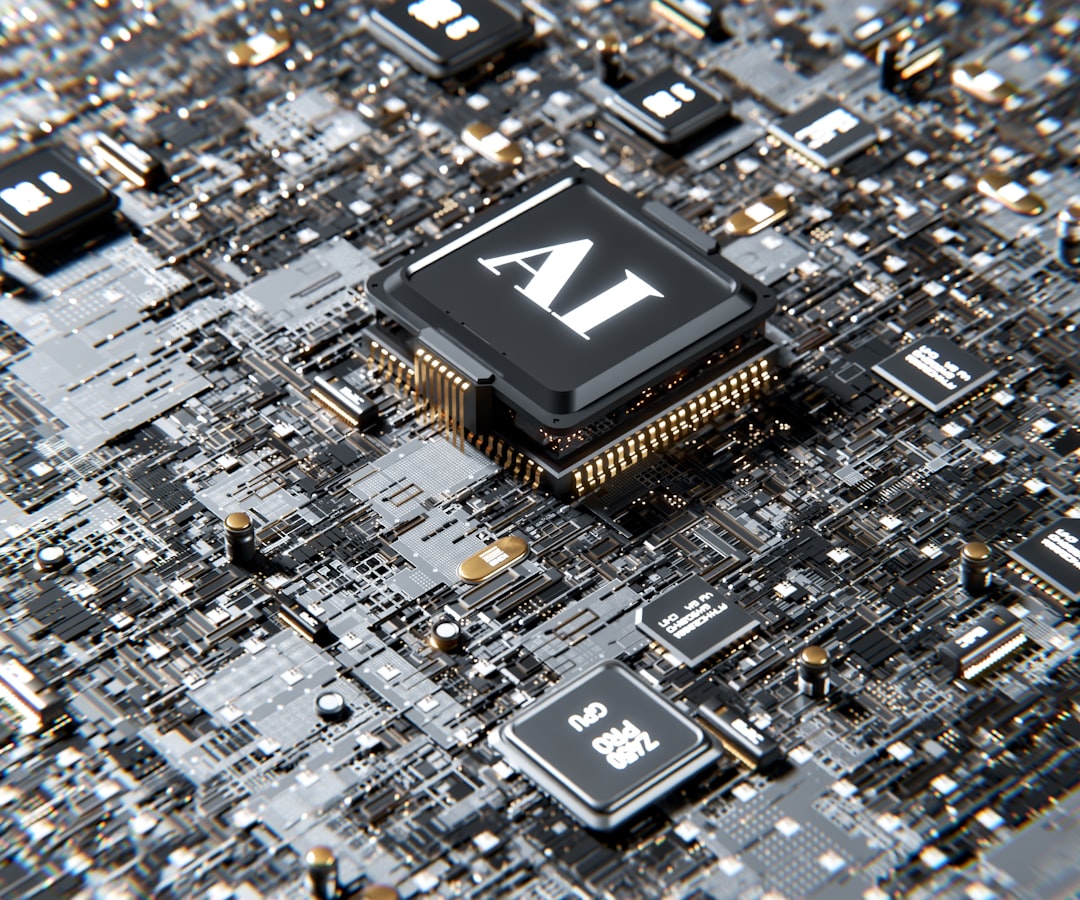
Analyzing video authenticity using machine learning algorithms has become increasingly important as AI-generated content, particularly deepfakes, becomes more sophisticated. The ability to seamlessly blend artificial content with real footage makes it harder to discern genuine from manipulated media. Machine learning algorithms are being employed to detect deepfakes by examining subtle cues like inconsistencies in blinking patterns or the synchronization of mouth movements with audio. These methods aim to identify telltale signs that might reveal the artificial nature of the content. However, as deepfake technology evolves, the challenge of distinguishing real from fake grows. The potential consequences of widespread misinformation and the erosion of trust in video content necessitate the development of robust and adaptable detection strategies. This ongoing arms race between those creating increasingly convincing deepfakes and researchers striving to detect them underscores the crucial need for innovative solutions to safeguard against the potential harm of manipulated media.
Analyzing video authenticity using machine learning algorithms is a continuously evolving field, driven by the increasing sophistication of deepfake technology. The effectiveness of these algorithms heavily relies on the breadth and quality of the training data they're exposed to. To accurately learn the subtle cues of manipulated videos, these algorithms need massive datasets covering a wide range of facial expressions, camera angles, and lighting conditions. This need for extensive and diverse data makes data collection a significant hurdle for researchers.
Despite the impressive advancements in deepfake generation, subtle imperfections can still slip through. These irregularities can manifest as inconsistencies in lip synchronization, blinking patterns, or inconsistencies in shadows. Detection algorithms are being developed and refined to pick up these seemingly minor details that may betray the artificial nature of a video.
Recently, machine learning has achieved remarkable progress in real-time deepfake detection during live streams. This is a major breakthrough, as it empowers platforms to immediately react to manipulations as they occur. This ability has the potential to dramatically curb the spread of misinformation by addressing the issue in real-time.
Transfer learning, a technique where models are trained on one type of data and then adapted for a different one, is becoming increasingly useful. It allows researchers to efficiently refine models originally trained on static images to be effective on video data. This helps streamline the process of creating more robust deepfake detection systems.
For optimal performance, some of the most promising methods analyze both audio and video streams simultaneously. They search for any discrepancies between what's heard and what's seen, as this can indicate artificial manipulation. This multi-modal approach of leveraging audio-visual cues is increasingly becoming a crucial aspect of verification.
Machine learning approaches often involve a combination of two model types: generative models that are trained to create content and discriminative models that specialize in identifying real from synthetic content. This interrelationship between these models helps strengthen the reliability of the detection systems.
However, the use of deepfake detection technology raises a critical ethical issue. The same underlying technology that creates deepfakes can be applied to detect them. This gives rise to concerns about access to these advanced detection tools, their potential for misuse, and the need for regulations to protect privacy.
Interestingly, the rise of deepfakes is having an impact on how people perceive visual media. Continued exposure to deepfakes can lead to a general increase in skepticism towards all visual content, with viewers questioning the truthfulness of even unaltered media.
The performance of deepfake detection algorithms can fluctuate greatly based on their architecture and fine-tuning. Models optimized for specific types of manipulations can falter when presented with new deepfake techniques. This underscores the ongoing need for ongoing research and development to enhance the ability of these algorithms to keep pace with evolving threats.
To ensure comparability and drive progress, there is a growing trend towards establishing standardized benchmarks for evaluating deepfake detection algorithms. By fostering consistency in the way algorithms are assessed, researchers can readily compare their methods against a shared set of performance metrics. This approach should facilitate the creation of more effective tools that can help tackle the growing issue of misinformation.
AI-Generated Faces Analyzing Video Deepfakes Created by ThisPersonDoesNotExist - Ethical Implications of AI-Created Media on Social Platforms
The ethical landscape surrounding AI-generated media, especially within the context of social platforms, is rapidly evolving. With deepfakes and AI-created content reaching new levels of realism, questions of identity, authenticity, and consent become paramount. The ease with which fabricated personas can blend into online interactions, particularly on social media, raises concerns about the spread of misinformation and its potential to erode trust. These concerns are further amplified by the lack of comprehensive regulations guiding the development and use of AI-generated content, leading to anxieties about privacy violations, biased representations, and potential copyright infringement. The continuous evolution of AI technologies demands a thoughtful and multi-faceted approach that navigates the ethical dilemmas they pose, while recognizing the creative possibilities they offer. Finding a balance between fostering innovation and mitigating the potential for harm is essential as we navigate this era of increasingly artificial content within our digital spaces.
The increasing realism of AI-generated media has introduced a curious dynamic where users can develop emotional connections with fabricated personas, even while recognizing their artificial nature. This effect muddies the waters of how we perceive relationships within digital spaces, raising questions about the impact on our understanding of genuine human interaction.
Legal frameworks are struggling to adapt to the rapid advancements in deepfake technology, often relying on outdated laws ill-equipped to handle the novel threats posed by synthetic identities and misinformation. This mismatch between technology and regulation creates a fertile ground for potential abuse.
Some research suggests that people find it more difficult to detect deepfakes featuring AI-generated faces compared to traditional deepfakes manipulating real people. It's as if our cognitive biases encourage a natural tendency to readily accept what appears authentic, even if it's entirely fabricated.
The wide availability of AI-generated faces has created an intriguing scenario where users might unwittingly amplify the spread of false information. Emotionally engaging but completely fabricated stories featuring these synthetic individuals can gain considerable traction on social media platforms, leading to rapid dissemination of misinformation.
The capacity of deepfakes to bypass traditional facial recognition systems raises substantial concerns about future privacy and security risks. The ability to create convincing synthetic faces has the potential to open pathways for identity theft or manipulation without a person's knowledge or consent, posing a serious threat to personal safety.
Cultural standards regarding consent and representation are being tested as AI-generated faces become integrated into marketing and entertainment, often without informing audiences of their synthetic nature. This presents complex questions about potential violations of individual rights, as these fabricated personas might substitute or represent real people without their awareness or permission.
The diversity of human perception across different demographic groups creates intriguing ethical considerations. The same AI-generated face can provoke vastly different responses depending on an individual's background, leading to potential exploitation through targeted content manipulation that might be based on gender, racial, or age-based biases.
Research suggests that the increasing prevalence of deepfake technology may lead to a broader desensitization towards visual media. Over time, we might find it progressively harder to differentiate real events from fabricated ones, potentially leading to pervasive skepticism about credible news sources. This erosion of trust in verifiable information could be a significant consequence of the technology.
Current deepfake detection methods tend to be reactive, frequently playing catch-up with newer methods for creating deepfakes. This suggests a crucial need for more proactive and preventative measures to safeguard against the potential harms of this technology. There's a gap between responding to new synthetic media and developing methods to address it before widespread distribution.
The potential for misuse of AI-generated facades extends beyond the spread of misinformation. For example, these synthetic faces can be leveraged for cyberbullying or harassment by creating convincingly real-looking fake accounts. This capability poses serious threats to online safety and could exacerbate issues related to mental health, as individuals are targeted with fake interactions.
AI-Generated Faces Analyzing Video Deepfakes Created by ThisPersonDoesNotExist - WhatsinmyVideo Tool Emerges as Deepfake Analysis Frontrunner
The WhatsinmyVideo tool has emerged as a leading contender in deepfake analysis, primarily due to its AI-powered video processing capabilities. Its ability to analyze most videos within a relatively short time frame—typically 5 to 10 minutes—is notable, although longer videos may require up to 30 minutes for processing. The tool offers detailed analysis outputs, such as transcriptions and summaries of the video content, streamlining the analytical process compared to traditional, manual methods. As deepfakes become increasingly realistic, tools like WhatsinmyVideo become crucial for combating the spread of misinformation and preserving the trustworthiness of digital media. However, the ongoing advancement of deepfake techniques could potentially challenge the effectiveness of current detection methods, making continued refinement and innovation essential. Furthermore, the potential for misuse of such tools highlights the need for careful consideration of ethical implications alongside technical advancements, aiming for responsible development and implementation.
1. **Navigating the Authenticity Crisis:** The rapid evolution of AI-generated faces, particularly within videos, is creating a complex landscape where discerning genuine from fabricated content becomes increasingly challenging. Even subtle imperfections in a deepfake might go unnoticed by viewers, making it difficult to establish the true nature of what we see in the digital realm.
2. **The Data Challenge in Deepfake Detection:** Machine learning algorithms are being employed to detect deepfakes by recognizing subtle anomalies, but their effectiveness is tightly linked to the diversity and quality of the training data. To truly improve these detection systems, researchers need access to massive, comprehensive datasets representing a wide range of facial expressions, demographics, and environmental conditions, which poses a considerable hurdle.
3. **Real-Time Deepfake Detection: A Promising Frontier:** Recent advancements in real-time deepfake detection during live streams are quite interesting, potentially enabling platforms to quickly identify manipulated content. However, this type of detection relies heavily on the simultaneous analysis of both audio and video streams, searching for any inconsistencies that might indicate artificial manipulation.
4. **The Uncanny Valley: A Psychological Hurdle:** The phenomenon of the uncanny valley highlights a curious human reaction to very realistic synthetic faces. While these AI-generated faces can be remarkably lifelike, they often contain subtle cues of artificiality that trigger an unsettling feeling in some viewers. This suggests a deep-seated need for authenticity in our interactions with visual media.
5. **The Auditory Deepfake Challenge:** While visual deepfakes have received much attention, the manipulation of audio presents a unique challenge. Replicating the intricate nuances of human speech, like vocal inflection and emotional cues, proves to be a complex task for AI, making audio deepfakes potentially even harder to detect.
6. **The Double-Edged Sword of Deepfake Detection:** It's interesting that some deepfake detection methods employ the very same algorithms used in the creation of deepfakes. While helpful for spotting signs of manipulation, this also raises ethical questions about who controls access to such powerful tools and how they might be misused.
7. **Cognitive Biases and Misinformation:** Research suggests that people may struggle to spot AI-generated faces in deepfakes compared to those that manipulate real people. This may be tied to our cognitive biases, making us more inclined to readily accept something as genuine, even if it's completely artificial. This factor raises serious concerns about the spread of misinformation in a world of increasingly realistic deepfakes.
8. **The Impact on Social Interactions:** The introduction of AI-generated faces into social media presents a unique challenge to understanding human connection and authenticity. Fabricated personas can, curiously, elicit genuine emotional responses from viewers, raising questions about the potential for emotional manipulation and how we perceive relationships in digital spaces.
9. **The Legal Landscape: Struggling to Keep Pace:** Existing legal frameworks haven't quite caught up with the rapid evolution of deepfake technology, creating a gap in regulations. This regulatory vacuum leaves the door open for potential abuse, lacking proper protections against identity theft, malicious impersonation, and the spread of misinformation.
10. **A Gradual Erosion of Trust:** As deepfakes become more common, there's a risk of people becoming gradually desensitized to visual media. This can lead to a general distrust of all visual information, where viewers begin to question the authenticity of everything they see—even real, unaltered content. This constant skepticism can be detrimental to society's ability to trust legitimate news sources and navigate a world of increasingly artificial imagery.
More Posts from whatsinmy.video: