Analyze any video with AI. Uncover insights, transcripts, and more in seconds. (Get started for free)
Analyzing 922 Breast Cancer MRI Sequences Advancing Precision Medicine in Video Analysis
Analyzing 922 Breast Cancer MRI Sequences Advancing Precision Medicine in Video Analysis - MRI Data Analysis Enhances Breast Cancer Diagnostic Accuracy
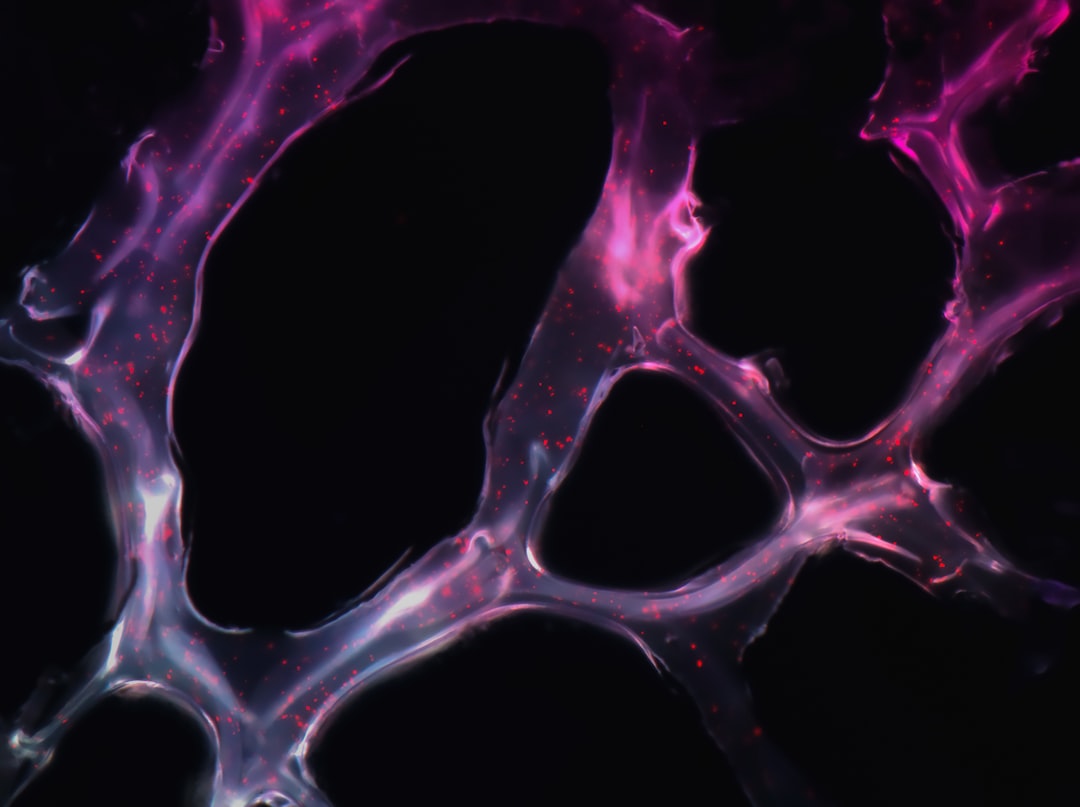
Recent advancements in breast cancer diagnostics have centered around MRI data analysis, particularly leveraging the capabilities of deep learning algorithms. These algorithms are showing significant promise in accurately analyzing MRI scans, effectively distinguishing between benign and malignant lesions. This leads to a reduction in unnecessary biopsies and enhances patient care. However, it is important to remember that these are still emerging technologies and their long-term impact on clinical practice is yet to be fully established. The effectiveness of these new techniques will ultimately be judged by their ability to improve overall patient outcomes.
The potential of MRI in breast cancer diagnosis is pretty fascinating. We're already seeing how it can find tumors that other imaging misses, thanks to its high resolution. But what's really exciting is how we can use MRI data to better understand the tumors themselves.
For example, DWI can help us gauge how aggressive a tumor is by looking at the cell density. That's huge for deciding on the right treatment plan. And, while mammography is good, it's not perfect. It sometimes causes unnecessary biopsies due to false positives. AI tools that analyze MRI data can help address that, making screening more accurate.
The fact that MRI isn't impacted by breast density is also important. It means it's a better screening tool for women with denser tissue. But there are still things to consider. MRI with contrast agents does involve some radiation, unlike what we often hear.
We're also exploring how functional MRI techniques like perfusion imaging can shed light on tumor environments and blood flow. This is a game-changer for customizing treatment.
What I find most promising is the potential to analyze massive datasets of MRI sequences. This helps refine models that can predict patient outcomes and personalize therapy. It's a big step toward truly personalized medicine. And the best part is, we can use MRI not just for detection but also to monitor treatment response, allowing for real-time adjustments as needed. The future of breast cancer diagnosis looks pretty bright, especially with the help of MRI and advanced data analysis.
Analyzing 922 Breast Cancer MRI Sequences Advancing Precision Medicine in Video Analysis - Deep Learning Techniques Match Radiologist Performance in Cancer Prediction
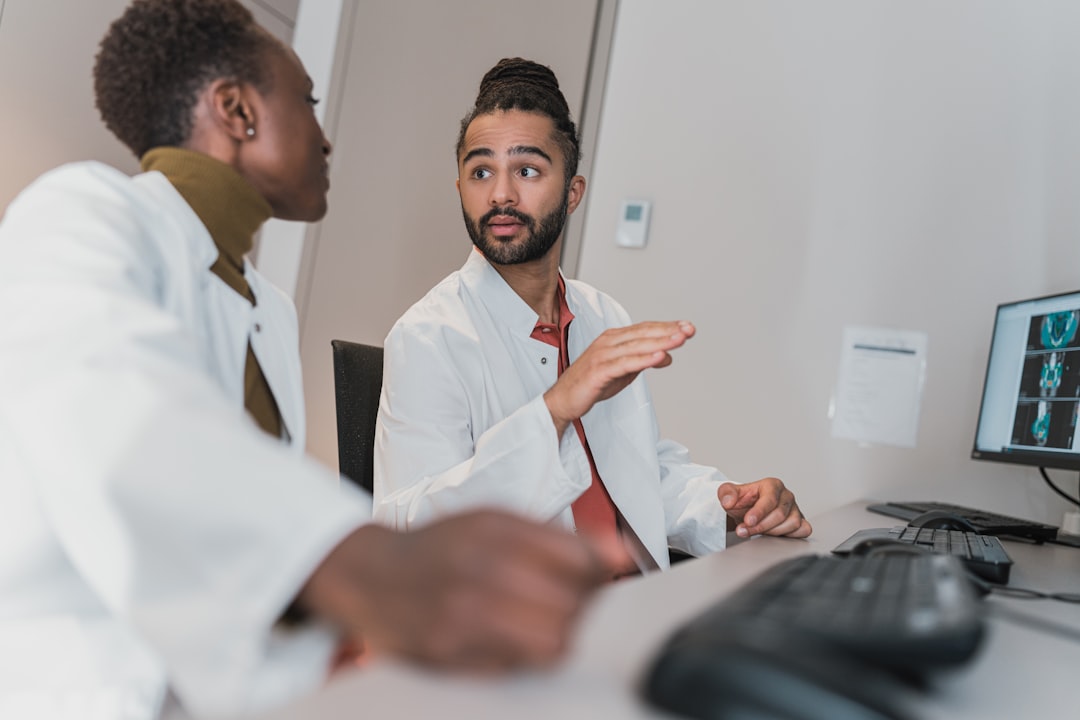
Deep learning techniques are showing promise in breast cancer prediction, performing on par with experienced radiologists, particularly when analyzing MRI sequences. This finding is significant because it involved a study of 922 breast cancer MRI sequences, demonstrating the potential of deep learning to improve diagnostic accuracy and potentially reduce the number of unnecessary biopsies. These techniques also require less human intervention for feature extraction than traditional methods, making the diagnostic process more efficient.
However, it is important to acknowledge that these techniques are still under development and require further validation in clinical settings before they can be widely adopted. It is crucial to ensure that these advancements are truly improving patient outcomes before they are widely implemented. Despite these challenges, the integration of machine learning in breast cancer diagnostics represents a major step toward more precise and intelligent healthcare solutions.
It's really interesting how deep learning is being used to analyze MRI scans for breast cancer. These algorithms seem to be catching on to subtle patterns that even experienced radiologists miss. That's a big deal, especially because it could lead to fewer unnecessary biopsies and better care for patients. But I'm still a bit cautious – we need to see how these new tools perform in the long run and how they actually improve outcomes for people.
MRI has shown it can detect tumors that other methods often miss. It's also useful for understanding how aggressive a tumor might be by looking at its density using DWI. This is extremely valuable for tailoring treatment. AI-powered MRI analysis could really change the game for screening, since it could potentially reduce the number of false positives that often lead to unnecessary biopsies.
The fact that MRI isn't affected by breast density is another huge advantage. It makes it a better screening tool for women with denser tissue. But we also need to remember that while we usually associate MRI with low radiation, using contrast agents does introduce some radiation.
It's amazing how MRI is being combined with other techniques like perfusion imaging to learn more about the tumor's environment and blood flow. This is a big step towards personalized treatment. Analyzing massive datasets of MRI sequences is what really excites me. This gives us the power to create models that can predict patient outcomes and help us personalize therapy. The potential for true personalized medicine is huge.
But it's not just about diagnosis – MRI can also be used to monitor treatment response. This is pretty amazing, as it allows us to make adjustments in real-time based on how well the therapy is working. It definitely seems like the future of breast cancer diagnosis is bright, especially with the help of MRI and advanced data analysis. But we need to keep working to integrate these technologies into the healthcare system seamlessly and make sure they're used responsibly.
Analyzing 922 Breast Cancer MRI Sequences Advancing Precision Medicine in Video Analysis - Linking MRI Imaging Phenotypes to Molecular Genomic Characteristics
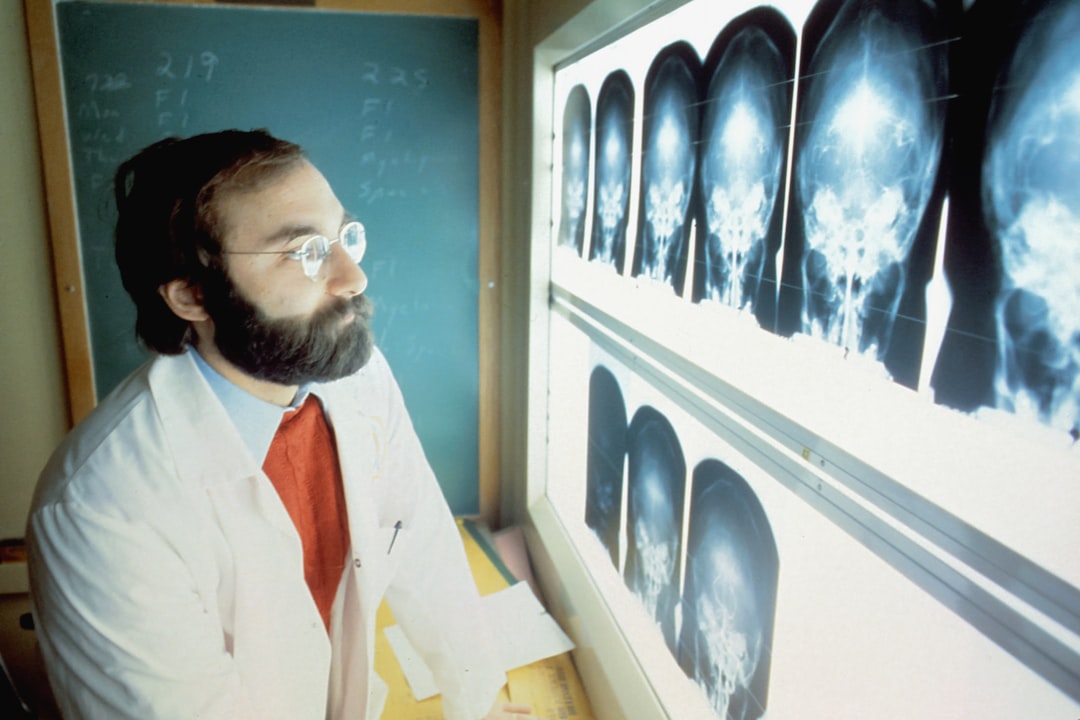
Connecting MRI images with the genetic makeup of breast tumors is a promising area for developing personalized medicine strategies. A recent study analyzing 922 breast cancer patients found strong connections between MRI features, such as the tumor's shape and how it appears with contrast, and the expression of important genes that control how the tumor grows. This suggests that information from MRI scans could be used as powerful biomarkers, helping to select the most effective treatments for each patient. By applying deep learning, researchers can uncover even more complex relationships between imaging and genetic information. As this field progresses, we may see the development of advanced models that predict how patients will respond to treatments, ultimately leading to more individualized and effective cancer care.
It's fascinating how MRI is moving beyond just detecting tumors. It's now being used to actually understand the underlying biology of these tumors, especially when combined with genomic data. This is a huge leap forward in personalized medicine.
Think about it – MRI sequences can capture dynamic changes in the tumor's environment, like how much blood flow there is. These changes might be directly linked to the tumor's genetic makeup. This is important because it could mean we can predict how well a patient might respond to treatment based on their MRI scans.
What's really exciting is that we're discovering specific MRI features that can act as biomarkers for certain genetic profiles. This could be a game-changer because it means we might be able to assess a tumor without even having to do a biopsy!
AI is already being used to analyze MRI data, and it's picking up subtle patterns that even experienced radiologists miss. This could lead to more precise diagnoses and potentially even predict things like treatment resistance or the chance of metastasis.
But here's the real kicker – we're finding that tumors with different genetic mutations show up differently on MRI. This is huge because it means we could personalize MRI analysis based on the patient's individual tumor's DNA.
The idea of "imaging genomics" is starting to take off. We're using MRI as a window into the tumor's biology to help us make smarter decisions about targeted therapies. And the really cool thing is that researchers are using machine learning to build models that predict how a tumor will behave based on its genetic makeup and what we see on the MRI scans.
It's definitely a thrilling time to be involved in this field. We're moving towards a future where MRI doesn't just help us diagnose cancer, it guides us towards the most effective treatments based on the unique characteristics of each patient's tumor. This is truly precision medicine at its best.
Analyzing 922 Breast Cancer MRI Sequences Advancing Precision Medicine in Video Analysis - Hybrid Deep Network Framework Improves Tumor Segmentation from MRI
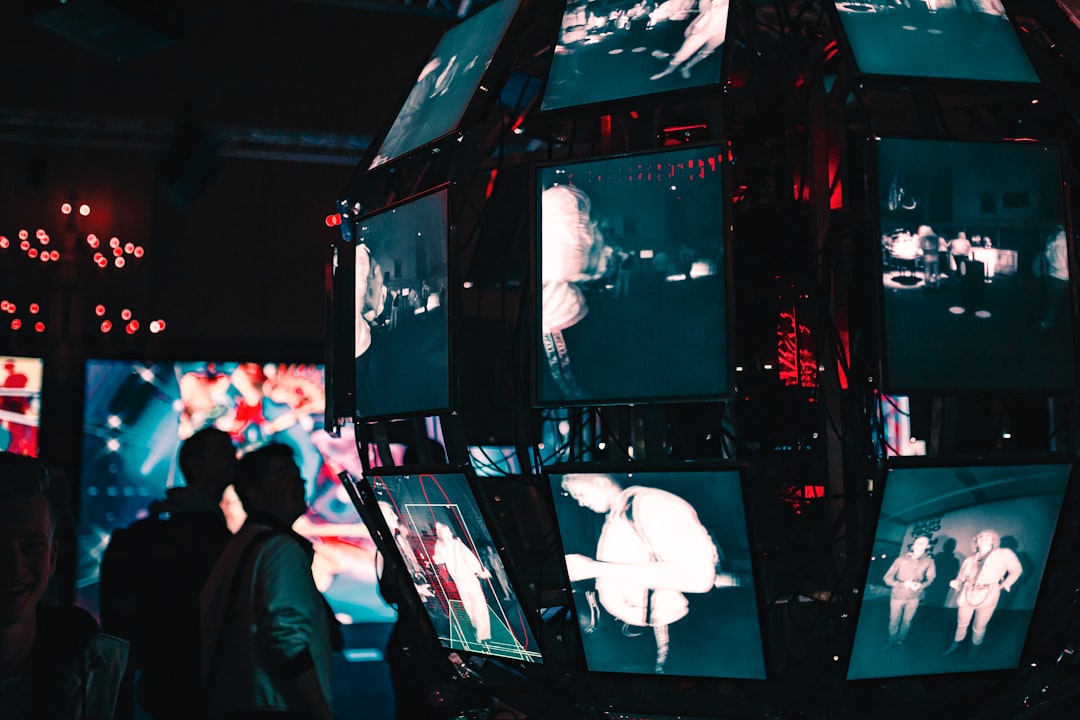
A new approach to analyzing MRI images of breast tumors is showing promise in improving accuracy. The ConnectedUNets framework uses a hybrid deep learning network to precisely identify tumor boundaries. This method analyzes multiple MRI sequences simultaneously, allowing it to better understand the complex relationships within the data. The researchers report impressive results, with a maximum accuracy of 95.39% for tumor segmentation. The framework incorporates an attention block that selectively focuses on important information from different MRI sequences, which is key to accurately determining the size, shape, and edges of tumors.
These advancements are significant because they address the challenges of analyzing complex MRI datasets and offer the potential to improve diagnosis and personalized treatment planning for breast cancer. By offering a more accurate picture of tumors, this hybrid approach could ultimately lead to better outcomes for patients. However, as with many emerging technologies in medicine, further validation in clinical settings is crucial to ensure these promising techniques translate into real-world benefits.
It's pretty exciting to see how deep learning is being used to improve tumor segmentation from MRI scans. One of the most promising approaches is this hybrid framework, which combines the strengths of both convolutional neural networks (CNNs) and recurrent neural networks (RNNs). CNNs are good at picking up spatial patterns within an image, while RNNs can analyze sequences of images, which is helpful for understanding how tumors change over time.
What makes this hybrid framework so powerful is that it can maintain the spatial and temporal relationships in the data, allowing for more accurate segmentation. This means the model can identify the tumor's boundaries more precisely and even pick up subtle changes in the tumor's shape or its interactions with surrounding tissue.
The impact of this improved segmentation is huge. Not only can it help radiologists make more accurate diagnoses, but it can also lead to fewer unnecessary biopsies. This is a big deal, since biopsies can be invasive and stressful for patients.
Another cool thing is that this framework seems to be adaptable to different types of MRI sequences, meaning it can be used with a variety of imaging protocols. This is important because it expands the framework's potential application in different healthcare settings.
The integration of this hybrid deep network with clinical data is truly promising. Imagine being able to link specific MRI features with genetic markers. This could lead to more personalized treatment pathways and a whole new level of precision medicine. But we still need to be careful. These models need to be trained on large datasets of high-quality images, and we need to make sure they remain transparent and interpretable so that doctors can trust them.
The future looks bright for these algorithms. They have the potential to revolutionize MRI analysis by allowing for near-real-time results. That means doctors could get feedback on scans much faster, allowing for quicker treatment decisions. This could really make a difference in the lives of patients, especially those facing a life-threatening disease like cancer. It's an exciting time to be involved in this field.
Analyzing 922 Breast Cancer MRI Sequences Advancing Precision Medicine in Video Analysis - AI Systems Predict Cancer Probabilities Using Dynamic Contrast-Enhanced MRI
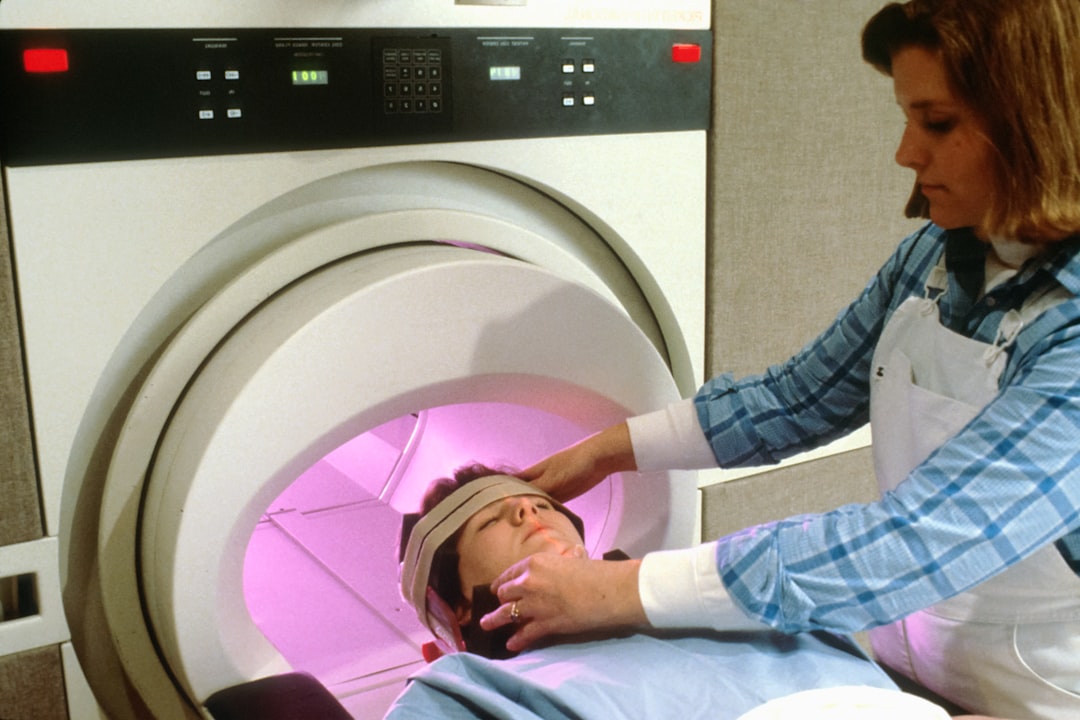
Artificial intelligence (AI) is playing an increasingly important role in breast cancer diagnostics, especially when paired with dynamic contrast-enhanced MRI (DCEMRI). AI systems, powered by deep learning algorithms like MBCNN, are proving remarkably effective at predicting the likelihood of cancer. These systems boast an impressive area under the receiver operating characteristic curve (AUROC) of 0.92, showcasing their ability to accurately distinguish between cancerous and benign tissues. Furthermore, they can even identify different molecular subtypes of breast cancer, paving the way for more tailored treatment plans. While these advancements are encouraging, it's crucial to rigorously evaluate their performance in real-world clinical settings to ensure they translate into improved patient outcomes. Integrating AI into routine clinical practice requires careful consideration to maintain accuracy and build trust in this emerging technology.
The use of AI to analyze Dynamic Contrast-Enhanced MRI (DCE-MRI) data is really exciting. DCE-MRI provides unique insights into tumor behavior that static imaging misses. The ability to see real-time changes in blood flow and tumor perfusion is a game-changer for understanding how a tumor is reacting to treatment.
AI algorithms are showing remarkable accuracy in analyzing DCE-MRI data, even surpassing traditional methods. Studies have shown that AI can identify subtle patterns within tumor morphology that correlate with specific breast cancer types. This could lead to more precise diagnoses and individualized treatment plans.
The speed at which AI can process large datasets like the 922 breast cancer MRI sequences is incredible. This is a huge benefit, as it could significantly streamline clinical workflows and accelerate diagnosis. The algorithms also can capture changes in tumor characteristics over time by leveraging the temporal component of DCE-MRI. This gives us a more dynamic understanding of tumor progression or regression and improves our ability to assess prognosis.
Research is uncovering a strong link between specific DCE-MRI imaging features and biological pathways related to tumor growth. This suggests that we could use DCE-MRI imaging as a non-invasive biomarker to guide treatment strategies. It's a big step towards personalized medicine.
I'm impressed by the accuracy of tumor segmentation with these AI frameworks, with results reaching over 95%. This means that deep learning models can delineate tumor boundaries more effectively than traditional methods.
Combining DCE-MRI data with molecular data is a particularly intriguing aspect. This approach could help us understand how specific genetic mutations influence tumor behavior and pave the way for truly tailored therapies.
It's amazing that AI can learn and adapt as new data becomes available. As more MRI sequences are analyzed, the algorithms become even more accurate and reliable. The ability of AI to detect patterns that humans might miss is remarkable. For example, AI can identify subtle changes in enhancement patterns that may indicate early signs of tumor aggressiveness.
The potential of AI to reduce unnecessary invasive procedures, like biopsies, is huge. More accurate predictions from MRI data could lead to more informed decisions and a significant improvement in patient quality of life.
Analyzing 922 Breast Cancer MRI Sequences Advancing Precision Medicine in Video Analysis - Radiogenomics Integration Advances Intelligent Breast Cancer Diagnosis
Radiogenomics is ushering in a new era of breast cancer diagnosis by seamlessly merging the power of imaging with genetic information. This merging is leading to more accurate and personalized treatment plans. One notable impact is the improved assessment of how effectively neoadjuvant chemotherapy works, which helps to better categorize risks and ultimately improves patient outcomes. By creating imaging biomarkers that represent both the outward appearance and the genetic makeup of tumors, researchers are pushing the boundaries of precision medicine. The rapid advancements in artificial intelligence (AI) and deep learning are crucial for sifting through enormous datasets, such as those from MRI sequences, to identify complex patterns that might otherwise be missed, ultimately leading to more accurate diagnoses. However, even with all these exciting advancements, thorough clinical testing is necessary to ensure these new tools are truly beneficial for patients in real-world settings.
The field of radiogenomics is truly fascinating. By merging imaging data like MRIs with genomic information, we can potentially unlock a treasure trove of personalized medicine insights. It's amazing how specific MRI features like a tumor's shape or how it responds to contrast agents seem to be directly linked to specific genetic mutations. This could revolutionize how we diagnose and treat breast cancer.
Dynamic Contrast-Enhanced MRI (DCE-MRI) is a particularly exciting technique. It's not just static images, but a window into the dynamic changes happening inside a tumor, revealing how blood flows and the tumor's response to treatment. This level of detail is invaluable for understanding tumor behavior.
AI is making incredible strides in analyzing this complex data. The algorithms are surprisingly good at segmenting tumors from MRI scans, achieving accuracies of up to 95.39%! That's incredibly promising for making diagnoses more precise and hopefully leading to fewer unnecessary biopsies.
Imagine being able to use specific features from an MRI scan as biomarkers! This would enable doctors to choose the most effective treatment without having to perform an invasive biopsy. And the potential for predictive modeling is truly exciting. AI could analyze MRI scans to predict how a tumor will behave, allowing for personalized treatment plans that address each patient's unique needs.
Another huge advantage is that AI can process MRI data much faster than humans. This can streamline clinical workflows and accelerate the entire diagnostic process. And by analyzing how tumors change over time, AI might even be able to detect early signs of tumor aggression that could derail a treatment plan.
The AI frameworks are becoming increasingly sophisticated, using "attention mechanisms" to focus on the most relevant information within multiple MRI sequences. This makes the tumor boundary representation even more precise. The combination of insights from radiology, genomics, and machine learning is a true game changer in oncology. It's all about a more comprehensive understanding of breast cancer that leverages both imaging and molecular data to optimize patient care.
Analyze any video with AI. Uncover insights, transcripts, and more in seconds. (Get started for free)
More Posts from whatsinmy.video: