Analyzing the Video Content of F1 World Champions' Iconic Races A Data-Driven Approach
Analyzing the Video Content of F1 World Champions' Iconic Races A Data-Driven Approach - Data Collection Techniques for F1 World Champions' Races
The pursuit of victory in Formula 1 has driven a dramatic shift towards data-centric approaches. Teams now employ a diverse array of data collection methods to dissect every aspect of a race. This includes compiling a wide range of data points, encompassing race outcomes, individual driver performances, and detailed information about each race track. The goal is to build a comprehensive picture of a race, enabling a granular analysis that goes beyond simple win/loss records.
Beyond collecting the raw data, organizing and refining it is equally crucial. Structured formats, such as relational databases, ensure that the vast quantities of information are readily accessible and can be efficiently utilized for performance optimization. However, the focus isn't solely on technical aspects. Understanding the preferences and behaviors of F1's global fanbase has become increasingly important. By analyzing viewer engagement across platforms, teams can gain insights into what fans find most compelling, enabling them to customize content in a way that resonates more deeply.
Furthermore, exploring how factors like starting positions and the wealth of data generated by wind tunnel testing affect race outcomes has become vital. The insights gleaned from these data points can profoundly influence team strategies and decisions, pushing the boundaries of what is possible in this highly competitive field. This data-driven methodology serves as a crucial instrument for extracting value from every aspect of a race, from the mechanics of a car to the psychology of a driver and the preferences of the viewers.
The world of Formula 1 has embraced a data-centric approach, with teams now leveraging highly sophisticated data collection techniques. Modern F1 cars are equipped with telemetry systems that capture a tremendous amount of data—up to 1,000 data points per second—providing incredibly detailed insights into every aspect of a car's performance, from engine function and tire wear to the intricacies of aerodynamic efficiency.
Analyzing this wealth of data requires advanced methods, including machine learning algorithms. These algorithms can effectively sift through past race data, tire performance patterns, and even opponent behaviors to predict optimal pit stop timings, a critical factor in race strategy. Furthermore, real-time monitoring of crucial aerodynamic factors like ground effect becomes essential. Changes in track conditions—from wind variations to fluctuating surface temperatures—can impact downforce and car stability, and engineers can adjust setups and strategies accordingly based on this ongoing data stream.
Each race generates a massive volume of information. Driver actions, like throttle position and speed, along with the nuances of suspension dynamics, contribute to approximately 1.5 terabytes of data per race. This vast repository serves as a foundation for analyzing race performance in hindsight, and also serves as a resource for future engineering developments and performance improvements.
The integration of video analysis with computer vision technology has significantly enhanced the level of insight F1 teams can achieve. Real-time tracking of competitors' positions and speed allows drivers and strategists to make informed decisions concerning overtaking opportunities and defensive driving tactics. Similarly, GPS technology provides extremely precise measurements of vehicle position—accurate down to centimeters— allowing for detailed comparison of driving styles and optimal racing lines taken by drivers throughout a track.
Beyond the performance of the car itself, teams are increasingly focusing on the driver. Integrating biometric sensors into driver suits facilitates the monitoring of vital physiological parameters such as heart rate and G-force exposure. This data allows them to understand how physical stress impacts a driver's ability to maintain performance under the extreme pressures of racing.
Furthermore, meticulous analysis of data from past races, specifically regarding tire performance at specific circuits, is proving invaluable in optimizing tire selection and car setup. Teams strive to balance lap times with minimizing tire degradation.
Augmented reality (AR) tools are also starting to play a role in enhancing engineers' comprehension of complex data sets. Through 3D visualizations of data, they gain a better grasp of vehicle dynamics and are able to respond more quickly to race conditions and the demands of real-time feedback.
The impact of data is not restricted to the race itself. Predictive analytics extends into the development of the cars themselves. Simulations powered by collected race data can guide engineers in making improvements and identifying aspects requiring further engineering refinement. The scope of F1 data analysis is constantly expanding, as historical data continues to provide new insights and is supplemented by real-time monitoring of race performance, contributing to the rapid advancement of the sport.
Analyzing the Video Content of F1 World Champions' Iconic Races A Data-Driven Approach - Machine Learning Algorithms Applied to Race Video Analysis
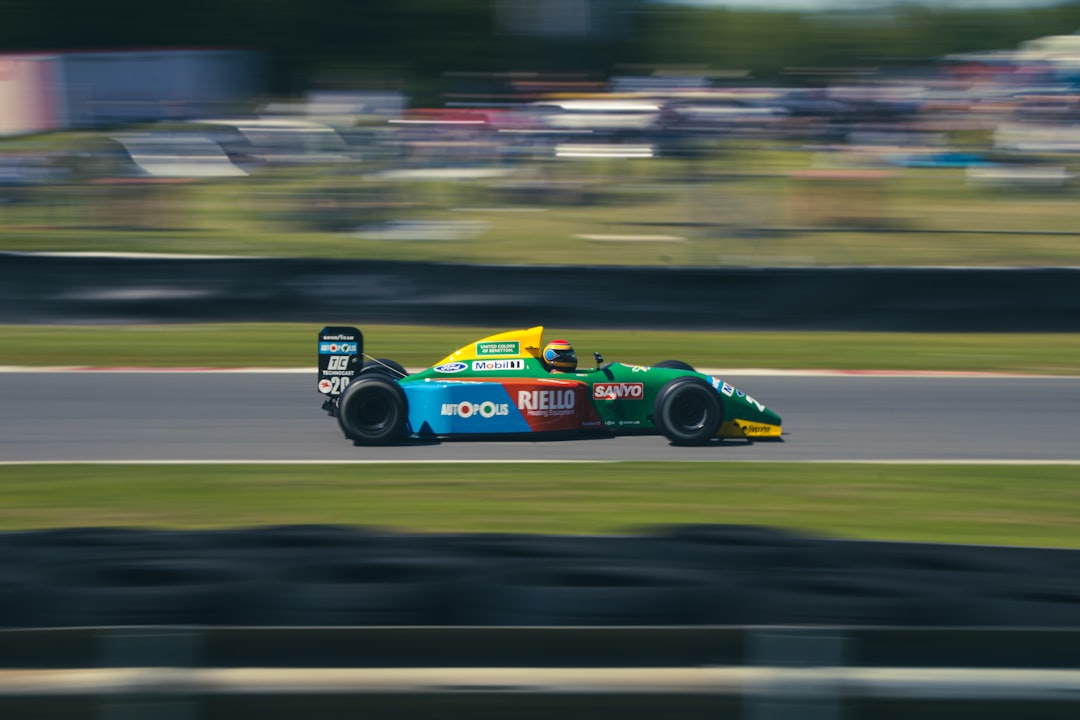
Machine learning algorithms are increasingly being used to analyze race videos in Formula 1, offering a more nuanced approach to understanding race dynamics. These algorithms are particularly valuable for processing the massive amounts of video data generated during a race, extracting meaningful insights that were previously difficult to obtain. By employing methods like supervised learning and deep learning, teams can automate the analysis of race footage, reducing the need for manual review and providing quicker access to information about car and driver performance.
Furthermore, machine learning enables the integration of data from diverse sources, such as telemetry and video, allowing for a more comprehensive understanding of a race. This capability, achieved through data fusion, is proving particularly useful in developing a more complete understanding of how a variety of factors influence race outcomes. These algorithms have far-reaching implications, not only impacting the technical aspects of the sport, but also shaping the way teams make strategic decisions and adapt to ever-changing track conditions in real-time. The reliance on sophisticated data analysis tools is becoming increasingly critical for gaining a competitive advantage in Formula 1, transforming how the sport is managed and ultimately influencing future advancements. While there are potential challenges associated with developing and deploying these complex systems, it is clear that machine learning is playing a pivotal role in shaping the future of Formula 1.
Machine learning algorithms, particularly those like XGBoost, K-Nearest Neighbors (KNN), Random Forest, Decision Trees, and Logistic Regression, are proving increasingly valuable in analyzing the complex data sets generated during Formula 1 races. These algorithms can often surpass traditional statistical methods because they possess the ability to adapt and refine their predictive models as new data becomes available. This adaptive nature allows them to become more accurate over time, leading to more reliable predictions about race outcomes, which, in the past, achieved a high 98% accuracy rate.
Deep learning, especially convolutional neural networks (CNNs), has introduced the potential for real-time video analysis within the realm of motorsport. This has enabled teams to not just track cars on the circuit, but also gain a more nuanced understanding of the events on-track by identifying aspects like tire slip, and crucial driver reactions, such as those during overtaking maneuvers. It's an intriguing avenue for potentially uncovering subtle aspects of the race not readily apparent from telemetry alone.
Techniques like data augmentation, commonly utilized in machine learning, offer a way to create simulated racing conditions within the training data. This is valuable because it allows algorithms to learn how to adjust to varying track conditions, like differing surface types and weather situations, without requiring extensive real-world racing. This approach could potentially minimize the risk for the drivers and cars.
Reinforcement learning presents the opportunity for models to simulate vast numbers of race scenarios in a virtual environment. This presents a strong argument for the use of virtual simulation. This approach can help refine strategies, like optimizing pit stops and tire usage before a real race, reducing risk. One interesting angle for future research could be applying this to simulate driver fatigue and its effects on performance and strategy.
The application of unsupervised learning could help uncover patterns in the massive historical data collected on past races. This could potentially unearth valuable insights into track characteristics and tire wear that might not be easily recognizable through standard analysis. However, this field is still in its early phases and the application in real-world settings is still being established.
The analysis of video streams provides a mechanism to directly compare driver actions against a collection of optimal driving practices. By cross-referencing telemetry against visual feedback on successful driving techniques, we can potentially identify performance gaps and provide feedback. This could help improve crucial areas of a driver's performance, like braking points and acceleration timing. This kind of detailed analysis is particularly helpful in the training of new drivers and could provide a quantifiable evaluation of their strengths and weaknesses.
Advanced machine learning approaches enable predictions of competitor actions based on their past performance. Examining the data from previous races, algorithms can assess the probability of aggressive tactics and defensive maneuvers based on a driver's tendencies. The predictive capabilities of these algorithms are of huge interest because they can provide insights into the dynamic interplay of racing and help with decision making on the track.
Combining video analysis with sensor data has enabled the creation of synthetic data sets that can model extreme race conditions. This is quite significant as it allows engineers and strategists to test and evaluate their strategies without having to risk human life and machine integrity. Testing extreme conditions virtually allows engineers to see how the cars will behave under a very large and diverse range of driving and environmental parameters.
Real-time machine learning analysis can vastly decrease the time required to reach decisions during a race. Teams can more quickly implement informed alterations to their race plans because of the dynamic nature of the race events. This suggests the value of rapid decisions to capitalizing on race-changing events that frequently occur in this racing series.
Training machine learning models with diversified data sets collected from various race tracks can enhance their ability to extrapolate across different environments. This will help in establishing more adaptable and stable strategies for future races. A question remains: are these algorithms ready to implement into real-time decision making by F1 teams in a robust and reliable manner?
Analyzing the Video Content of F1 World Champions' Iconic Races A Data-Driven Approach - Visual Recognition of Iconic Overtaking Maneuvers
Within the dynamic realm of Formula 1, the ability to execute iconic overtaking maneuvers stands as a testament to a driver's skill and strategic acumen. These maneuvers, often characterized by late braking or precise positioning at specific circuits like the Senna S at Interlagos, demand a high level of precision and split-second decision-making. Examining these maneuvers through a visual lens reveals the intricate interplay of technique and strategy that leads to a successful overtake. The application of sophisticated methods like machine learning and video analysis is further enhancing our understanding of these iconic moments, providing granular insights into how driver behavior and approach angles contribute to overtaking success. While these technologies offer exciting avenues for optimization, concerns remain about the increasing difficulty of overtaking in the sport, particularly as the performance gaps between teams narrow. As Formula 1 continues its relentless pursuit of performance and technological advancement, the application of these analytical techniques could significantly impact the way overtaking maneuvers are conceptualized, executed, and studied in the future. There's a risk that some of the core excitement of Formula 1 may be diminished if overtaking becomes too difficult.
Formula 1 overtaking maneuvers, especially the iconic ones, involve a complex interplay of driver skill, track conditions, and strategic decision-making. It's not just about raw speed; drivers need to be highly attuned to visual cues from other competitors, such as changes in acceleration or subtle body movements. These visual hints often signal upcoming changes in their positioning on the track. It's been observed that the most effective overtaking usually takes place within a relatively short window, perhaps 3 to 5 seconds, requiring drivers to quickly assess both the risk and potential benefits of the maneuver while simultaneously gauging their opponents' actions.
While seasoned race analysts can identify overtaking instances during post-race reviews, machine learning and particularly deep learning algorithms offer a novel approach. They enable real-time detection and analysis, revealing potentially critical insights that might escape human observation, even from experts. By employing machine vision techniques, we can delve deeper into how drivers are interacting with their environment. Using advanced algorithms on race video footage could reveal predictive patterns in driver behavior. For instance, we could perhaps forecast a more aggressive overtake or a defensive maneuver based on prior driver actions. This idea, while intriguing, is still an active area of research.
Maintaining precise positioning during a potential overtake is paramount. Research shows that a leading position, ideally at least a car width ahead of the car to overtake, significantly improves the odds of a successful maneuver. Even the slightest miscalculations can lead to a loss of control, potentially causing a dangerous incident. We're seeing a trend where drivers with access to real-time information, such as a competitor’s position and their tire status, tend to have more successful overtakes. This link between data access and competitive advantage is a noteworthy outcome from data analytics.
Track design has an impact on when and how drivers consider an overtake. Certain sequences of corners, especially those with more expansive views, may allow for better observation of overtaking possibilities, influencing tactical decisions. In addition, we are learning that a driver's mental state plays a significant role in these decisions. Research shows that stress can hinder visual recognition of critical details. This suggests that highly stressed drivers may be more prone to less controlled, more reactive racing maneuvers. Further, it has been speculated that accomplished drivers may have enhanced temporal visual memory, which allows them to quickly reconstruct recent events and predict where rivals might be headed in a high-speed environment.
There's a potential benefit to integrating simulation technology into driver training. Using high-fidelity simulations in combination with machine learning methods allows drivers to hone their overtaking skills in various conditions. These simulations can help drivers develop an advanced cognitive awareness to spot overtaking cues from their opponents and refine their reactions. While much of this research is still in its early stages, this approach has promise in creating more skilled and adaptable racers.
Overall, analyzing race videos through the lens of computer vision allows researchers to understand the nuanced aspects of iconic F1 overtaking maneuvers. While this technology is still developing, it may become increasingly helpful for teams to better understand a driver's strengths, weaknesses, and overall driving style. Further development of machine learning algorithms and visual recognition technology could bring unprecedented understanding to this aspect of racing, and possibly provide a new approach to developing both drivers and race strategy.
Analyzing the Video Content of F1 World Champions' Iconic Races A Data-Driven Approach - Telemetry Data Integration with Video Footage
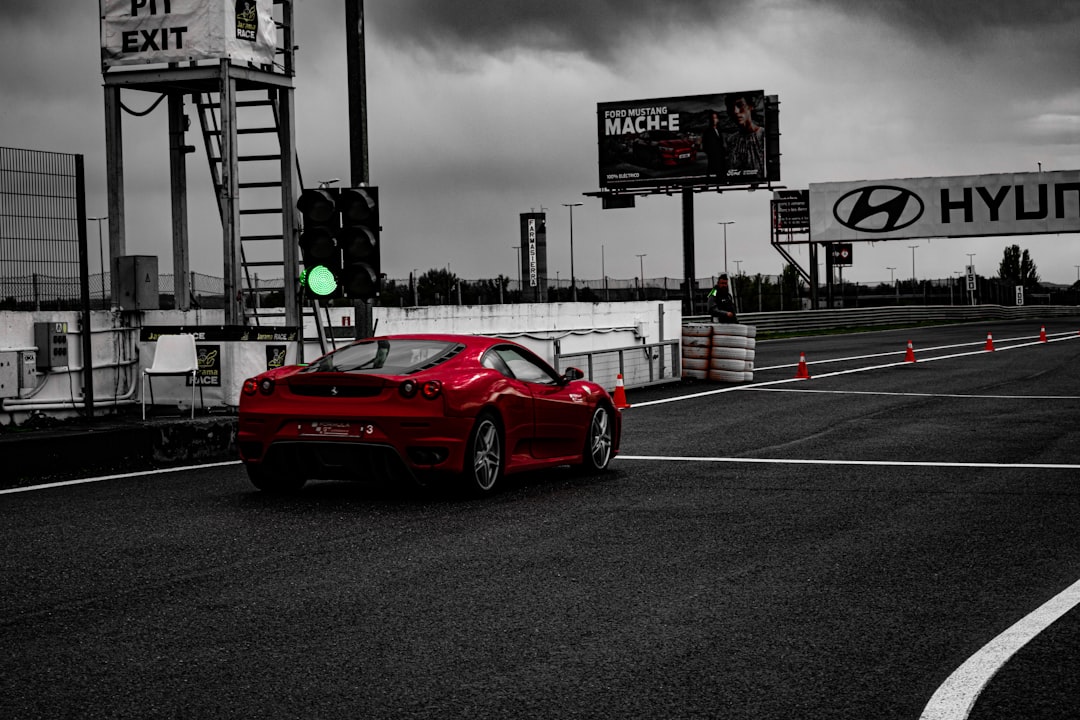
Integrating telemetry data with video footage has significantly advanced Formula 1's ability to analyze race performance. Combining the wealth of real-time car performance data from telemetry systems with video captures allows teams to gain a more comprehensive understanding of race events. This integration facilitates direct comparisons between what drivers are doing and the corresponding car data, leading to a multi-dimensional understanding of critical race moments. However, as Formula 1 leans more heavily on these technologically advanced methods, concerns arise about the balance between human instinct and automated analyses, especially in the intense and high-pressure racing environment. The continued development of this technology shows tremendous potential for progress, but also prompts questions about the fairness and implications of data-driven decision-making in such a fast-paced and competitive sport.
Formula 1's reliance on telemetry goes beyond just engine and tire data; it also offers a window into the driver's physiological state. Biometric sensors integrated into driver suits capture heart rate variability, giving us insights into how stress impacts performance and decision-making during crucial race moments. This connection between emotional states and lap times is a fascinating avenue for exploration.
Combining video footage with telemetry creates a powerful tool for analyzing a race. We can literally see the car's dynamics and the driver's actions simultaneously, thanks to video capture at high resolutions like 30 frames per second. This level of detail allows us to break down overtaking maneuvers with unprecedented precision, pinpointing exactly what actions lead to success.
When we synchronize video with the telemetry data streams, we can dive deep into specific race moments. Engineers can perform detailed performance reviews, studying braking patterns, throttle inputs in real-time, and analyzing how the car's aerodynamics behave in different track sections. It's like having a microscope for race analysis.
However, this wealth of data presents its own set of challenges. The sheer volume of data from a single race can easily reach 1.5 terabytes. Developing efficient data management and processing systems becomes essential for turning raw data into timely insights that can be acted upon.
We're seeing increasing potential in using advanced visual recognition algorithms. These algorithms can learn from historical race footage and telemetry data to recognize specific overtaking techniques. This could lead to skill-based recommendation systems within driver training programs—a way to objectively analyze and optimize driver performance.
Fusing telemetry and video analysis allows us to create better predictive models. By identifying patterns in past driver behavior, we can predict the likelihood of specific competitor actions or tactics. This predictive element can be a game changer for strategy, as teams can anticipate and adjust to different racing scenarios based on prior learned responses.
It's important to remember that not all telemetry data is equally useful. We often need sophisticated filtering techniques to sift through massive datasets and pinpoint the most relevant metrics. This task is crucial for isolating signals that truly correlate with improved performance.
Interestingly, combined telemetry and video analytics can be leveraged to build computational models that enhance real-time decision-making. These models, through algorithmic design, can predict optimal pit stop strategies based on projected changes in track conditions during a race.
Applying machine learning to video analysis also allows us to identify subtle tire wear patterns, which we can then correlate with telemetry data. This dual perspective leads to optimized pit strategies that account for both the car's mechanical condition and environmental factors like track temperature.
Augmented reality (AR) is becoming a powerful tool in this space. Overlays of telemetry data onto video feeds give engineers richer contextual information during a race. By visualizing optimal driving lines and techniques directly within the racing environment, they can make real-time adjustments and optimize strategies. This capability has the potential to change how engineers and teams approach strategy during a race.
Analyzing the Video Content of F1 World Champions' Iconic Races A Data-Driven Approach - Quantifying Driver Performance Through Video Metrics
Formula 1's pursuit of performance has led to a greater emphasis on quantifiable insights derived from video analysis, especially when it comes to understanding driver performance. By extracting data from video—like a driver's cornering speed or how they execute overtaking maneuvers—teams can get a much clearer picture of what's happening on the track. This has opened up new avenues for understanding how drivers affect race outcomes. The combined use of video analysis and the already vast array of telemetry data creates a more comprehensive understanding of key moments in a race. While these technological advancements offer ways to refine strategy and training, concerns remain about the role of human judgment amidst this push for automation in such a fast-paced and high-stakes sport. The future of Formula 1, it seems, will be increasingly shaped by how these insights are utilized, with potentially significant impacts on driver development and strategic decisions within the racing environment.
Formula 1 teams are increasingly leveraging the combination of video and telemetry data to gain a deeper understanding of race performance. By aligning high-definition video with the constant stream of telemetry data, engineers can meticulously examine key race moments, dissecting everything from the driver's throttle input to how the car's aerodynamics behave with exceptional detail.
A single F1 race generates a massive amount of data, including video, telemetry, and sensor readings—roughly 1.5 terabytes. This sheer volume of data requires highly advanced data management tools to efficiently extract usable insights from the raw information.
The integration of biometric sensors within driver suits allows teams to monitor a driver's physiological responses during the race, including heart rate variability. This helps us understand the interplay between stress and performance, particularly in the high-pressure moments of a race, which can inform more effective strategic decisions.
Machine learning algorithms are being used to review past race videos to identify distinct overtaking maneuvers. These insights can inform individualized driver training programs, which utilize real-time performance feedback to help drivers improve their skills.
Sophisticated visual recognition algorithms can pick up subtle patterns in driver behavior that might be missed by human analysts. This helps us understand how a driver's technique changes depending on a car's mechanical condition or the characteristics of the track.
Beyond purely mechanical data, telemetry can help us predict how rivals might behave in a race. By analyzing past races, teams can adjust strategies based on the anticipated probability of aggressive or defensive maneuvers by their competitors.
Predicting optimal pit stop strategies relies on both telemetry and video data. Computational models can provide projections of how track conditions might shift throughout a race, allowing teams to react quickly and perform pit stops at the most opportune times.
Combining telemetry sensors with video data offers a new perspective on tire wear. By analyzing both, teams can optimize tire management and ensure performance across the duration of a race.
Augmented reality (AR) tools are increasingly used to enhance engineers' understanding of race situations. AR overlays provide engineers with a broader context by combining live video with key telemetry data and visualizations of ideal driving lines. This approach allows teams to more rapidly adapt to changing conditions.
The ongoing investigation into overtaking maneuvers, informed by advanced technologies, suggests a link between driving psychology and race results. Drivers with exceptionally good temporal visual memory—the ability to quickly reconstruct events they've recently seen—seem to be able to make better decisions under pressure.
Analyzing the Video Content of F1 World Champions' Iconic Races A Data-Driven Approach - Comparative Analysis of Champions' Driving Styles Over Decades
Examining the driving styles of Formula 1 World Champions across decades unveils a captivating evolution tied to technological advancements, vehicle capabilities, and evolving race strategies. Drivers like Gilles Villeneuve and Michael Schumacher, icons of the past, exemplified a more aggressive, perhaps even riskier, driving approach. This contrasts sharply with the smoother and more calculated styles we observe in modern champions like Lewis Hamilton and Max Verstappen. The sport has shifted towards a greater emphasis on tire management and performance optimization, significantly influenced by the wealth of data available to contemporary drivers. This data-driven focus has contributed to a degree of homogenization in driving styles across the grid. However, individual drivers still retain distinct stylistic traits, demonstrating the persistent importance of personal preferences within the highly competitive and technologically demanding world of Formula 1. As the sport continues to progress, dissecting these driving styles through a data-centric lens could hold the key to understanding the characteristics of future champions and the path to sustained success.
Formula 1 has witnessed a significant evolution in driving styles across the decades, shifting from a reliance on intuition and inherent skill to a more data-driven approach. Drivers from the 1980s, like Gilles Villeneuve, often relied on their experience and instincts, in contrast to the modern champions, like Lewis Hamilton and Max Verstappen, who heavily utilize real-time telemetry and predictive analytics. This transformation highlights the growing influence of technology and strategic analysis in the sport.
A fascinating aspect is the use of biometric feedback from driver suits. Monitoring metrics like heart rate and G-force offers valuable insights into the impact of physical stress on driver performance and decision-making under the demanding conditions of a race. This detailed look at physiological influences has the potential to unearth patterns that shape a driver's style and decision-making during intense race moments.
The role of machine learning algorithms is becoming increasingly critical in analyzing historical driver behavior. These algorithms can now predict the likelihood of successful overtakes, which has become even more crucial as the competitive gap between teams narrows. This predictive capacity compels drivers to refine their approaches, adapting rapidly to new insights from the data.
Integrating telemetry with video footage provides a more holistic view of race performance. Teams can analyze not only performance numbers but also how drivers control vehicle dynamics during crucial phases like cornering, coupled with details like their throttle input. This layered analysis of both the mechanical and human elements leads to better insights to improve technical setups and driver skill development.
Advanced visual recognition is revolutionizing our understanding of driving behaviors. Algorithms can identify tire slip or optimal overtaking angles in real-time. This detailed perspective enables a deeper dive into iconic driving styles and allows for refining driver training protocols, promising even greater levels of refinement in skill.
There is evidence that top drivers might have superior temporal visual memory—the ability to retain and recall recent racing events. This enhanced cognitive skill allows them to anticipate rival moves more accurately, shaping their driving styles and influencing overtaking strategies.
The capacity of a driver to adjust their driving strategy, guided by real-time data about track conditions, has been shown to improve performance. The most successful champions are incorporating this data into their decision-making process, showcasing a shift toward a more analytical approach.
Analyzing archived footage of previous races through the lens of machine learning has the potential to reveal long-term trends and shifts in driving styles among champion drivers. This historical context offers valuable lessons that today's champions can leverage to fine-tune their own techniques, learning from past triumphs and missteps.
Overtaking maneuvers require a remarkable degree of precision. Data suggests a narrow window of 3-5 seconds exists where overtakes are most likely to be successful. This emphasizes the need for precise speed and positioning control. Champions often refine their style to seize these fleeting opportunities, highlighting the perfect balance between instinct and strategic decisions.
The expansion of data analysis in racing inevitably raises questions about the balance between automated decision-making and a driver's innate skill set. As teams incorporate more technology, concerns arise regarding the influence on traditional driving styles and the core aspects of racing. This delicate interplay between human instinct and technological enhancement shapes the future of this exhilarating sport.
More Posts from whatsinmy.video: