Data Annotation Training Unlocking AI Potential Through Precision Labeling
Data Annotation Training Unlocking AI Potential Through Precision Labeling - Raw data collection methods for AI training in video analysis
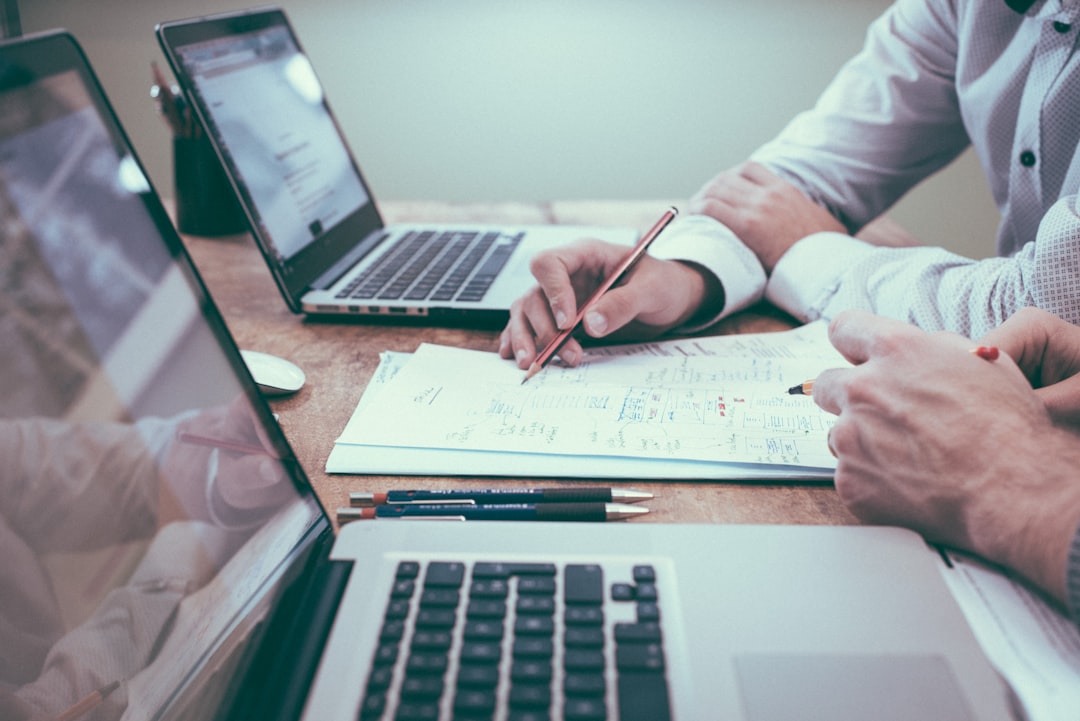
The foundation of AI training for video analysis rests upon the methods used to collect raw data. This involves a diverse range of strategies, such as utilizing readily available open-source video datasets, creating synthetic data to augment real-world examples, or leveraging the knowledge learned from pre-trained models through transfer learning. This process isn't simply a matter of gathering any video footage. It demands a well-defined structure encompassing stages like meticulously planning the dataset's purpose, selecting appropriate collection methods, and preparing the data for training. The quality of this raw data is inherently linked to the reliability and effectiveness of the resulting AI model. Poor quality input data leads to poor output, limiting the application's true potential.
However, the journey of collecting and preparing video data for AI isn't solely about technical aspects. It's vital to recognize that the methods employed can inadvertently introduce bias, which can severely undermine the integrity and trustworthiness of the final AI system. Therefore, ethical concerns are a critical component of data acquisition and curation, emphasizing the necessity for fair and unbiased practices. Furthermore, as AI technology progresses, the quest for refined and efficient annotation techniques remains a priority, driven by a desire to boost accuracy and expedite the labeling process – these processes play a vital role in making the information easily usable by the AI model.
1. Gathering raw video data for AI training involves innovative methods like crowdsourcing, where large numbers of individuals contribute to collecting and labeling vast video datasets. This approach can drastically accelerate data collection, potentially generating millions of data points in a shorter timeframe than traditional techniques.
2. Diverse motion sensors, including optical flow sensors, play a crucial role in extracting data from videos. These sensors offer valuable insights into object movement patterns, which are essential for training AI systems to analyze video in real-time.
3. Public spaces are often used as sources for raw video data, with surveillance cameras being a common method of acquisition. However, this practice raises crucial ethical concerns around privacy and data consent, significantly impacting how these datasets can be ethically used.
4. Recent advances in motion capture technology facilitate the collection of highly precise and detailed human movement data. This data can be synthesized for AI training, leading to more nuanced representations of human biomechanics and improved algorithm training processes.
5. Simulated environments are increasingly utilized to accelerate the raw data collection process. Generating synthetic video data lets engineers create various scenarios that might be rare or difficult to obtain in the real world. This technique enhances training datasets and boosts AI model performance.
6. Real-time data streaming is becoming more prevalent, enabling systems to collect and label data concurrently. This approach results in considerably faster iteration cycles for AI model optimization, but it introduces complexities in quality control due to the need for instantaneous annotations.
7. Integrating different data modalities, such as audio or environmental sensor data, can greatly enhance video analysis. This multimodal approach provides a richer context for model training, leading to better comprehension of intricate scenarios.
8. Data annotation tools can be implemented to enable direct data collection from end-users. This approach allows for the acquisition of localized and scenario-specific data that reflects users' actual experiences, resulting in improved model relevance.
9. Coding and annotating video data often relies on frameworks that permit extensive tagging and labeling. However, inconsistencies in human annotation can lead to noisy datasets, requiring the implementation of complex data cleaning and validation techniques.
10. The development of efficient annotation methods tailored for mobile devices is crucial given the limited computational resources available. Innovative approaches to real-time labeling and processing could enable on-device learning, posing unique challenges and opportunities for data collection in video analysis.
Data Annotation Training Unlocking AI Potential Through Precision Labeling - Key steps in data annotation process for video content
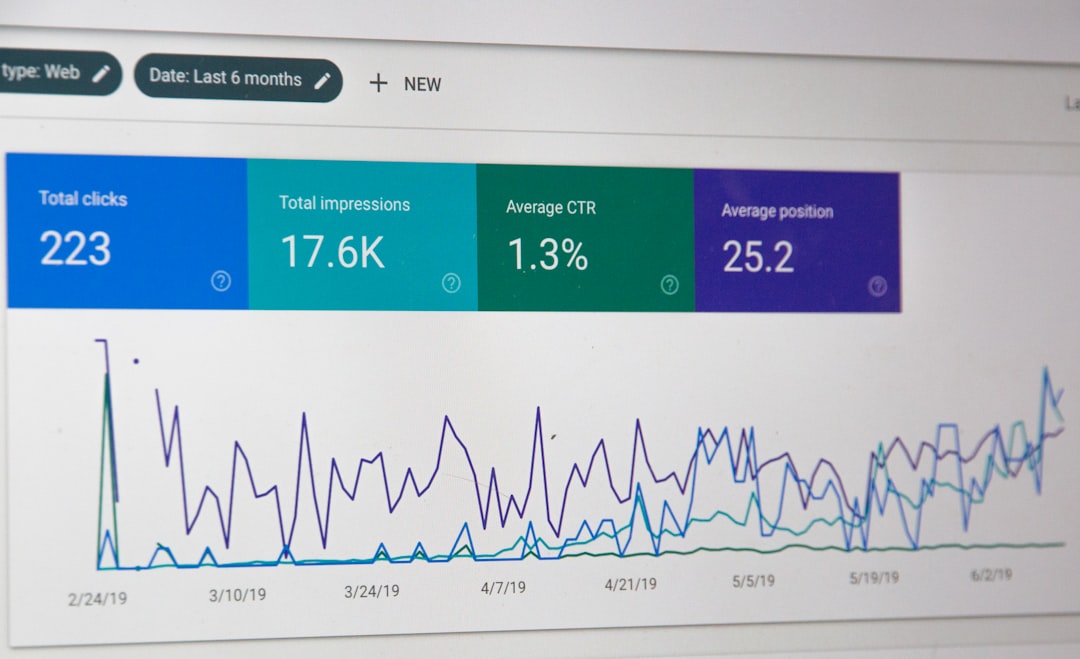
The core of training AI models to understand video content lies within the data annotation process. This process translates raw video footage into a structured format that AI can interpret. It begins with choosing the right software tools, which are essential for efficiently handling and labeling the videos. Next, the videos are uploaded into the selected software. The annotator then identifies the key elements or "targets" within each video – be it objects, actions, or events – and marks them with specific labels. Finally, the annotated data is saved or exported in a format compatible with the AI model being trained.
Maintaining consistency in labeling is paramount. Inconsistent annotations introduce "noise" into the data and make it less reliable for training. This careful approach is particularly crucial when it comes to training AI systems for tasks related to computer vision and machine learning.
Beyond basic identification, the annotation process can also involve more sophisticated labeling techniques. These include approaches like structural annotation, which describes the structure or organization of the video, and semantic annotation, which provides context and meaning to the content.
The importance of meticulous annotation is only increasing. The growing demand for AI capabilities across industries will require larger, cleaner, and more precisely annotated datasets. Understanding the core steps in this process allows for better leverage of AI in video analysis and related domains.
1. Improving the quantity of labeled data without needing to capture more video can be achieved using techniques like frame interpolation. This essentially involves predicting intermediate frames between existing ones, leading to a denser dataset of annotated frames. While seemingly helpful, this approach might introduce inaccuracies if the interpolation process isn't robust enough.
2. The choice of labeling approach for objects, actions, or scenes has a noticeable effect on how well a video analysis AI model learns. For instance, using bounding boxes compared to semantic segmentation will result in a different type of representation, influencing how well the model recognizes and interprets the content. It highlights the need for careful consideration when designing the labeling strategy.
3. It's been observed that even experienced human annotators can disagree on what constitutes a correct label, leading to inconsistency issues. This can be a significant problem, especially if we consider the fact that disagreement rates can be surprisingly high, up to 30%. It's a reminder that annotator training needs to be robust and clear labeling guidelines are crucial to minimize these issues.
4. Maintaining consistency of labels across the frames of a video is incredibly important. If there are inconsistencies, the AI model can become confused as it tries to understand how things move or interact over time. This requirement adds a layer of complexity to the annotation process, demanding precise attention to detail.
5. Leveraging computer vision tools to pre-annotate a video before a human annotator begins can greatly reduce the time required for labeling. Rather than manually annotating everything, humans can instead review and refine pre-existing annotations, potentially saving significant time and effort. However, we should carefully examine the accuracy of these pre-annotation algorithms.
6. Tracking not just static labels like object location but also dynamic qualities like when an object is partially hidden or interaction histories can significantly improve the detail of the annotation. This addition, however, creates a challenge in how these complex annotations are designed, and the impact of increased complexity needs further exploration.
7. Introducing elements of gamification during the annotation process is an interesting way to increase motivation and potentially enhance annotation accuracy. It is hypothesized that injecting some competitiveness into the labeling tasks might lead to a more engaged workforce and better quality data. However, it's unclear whether gamified approaches improve accuracy, and the potential trade-offs in quality need evaluation.
8. The resolution and frame rate of a video have a considerable effect on the quality of annotations. Higher resolution and frame rate videos can enable more granular analyses but come at the cost of larger file sizes and increased computational demands for processing and labeling. Researchers need to find the ideal balance between video quality and the ability to effectively process and annotate it.
9. Understanding not only what's happening in a video but also when it occurs adds a whole other level of complexity. Temporal annotations, which cover tasks like action segmentation or event detection, are becoming increasingly important for many applications. While this adds considerable sophistication to the process, the resulting model's performance might need further analysis to determine the exact impact of temporal understanding.
10. Collaborative platforms where multiple annotators work on the same video dataset can speed up the annotation process. However, challenges arise in coordinating tasks and maintaining consistency across multiple annotators. This setup offers an interesting opportunity, but the potential for introducing errors related to disagreement needs to be investigated and managed.
Data Annotation Training Unlocking AI Potential Through Precision Labeling - AI-powered tools streamlining video labeling workflows
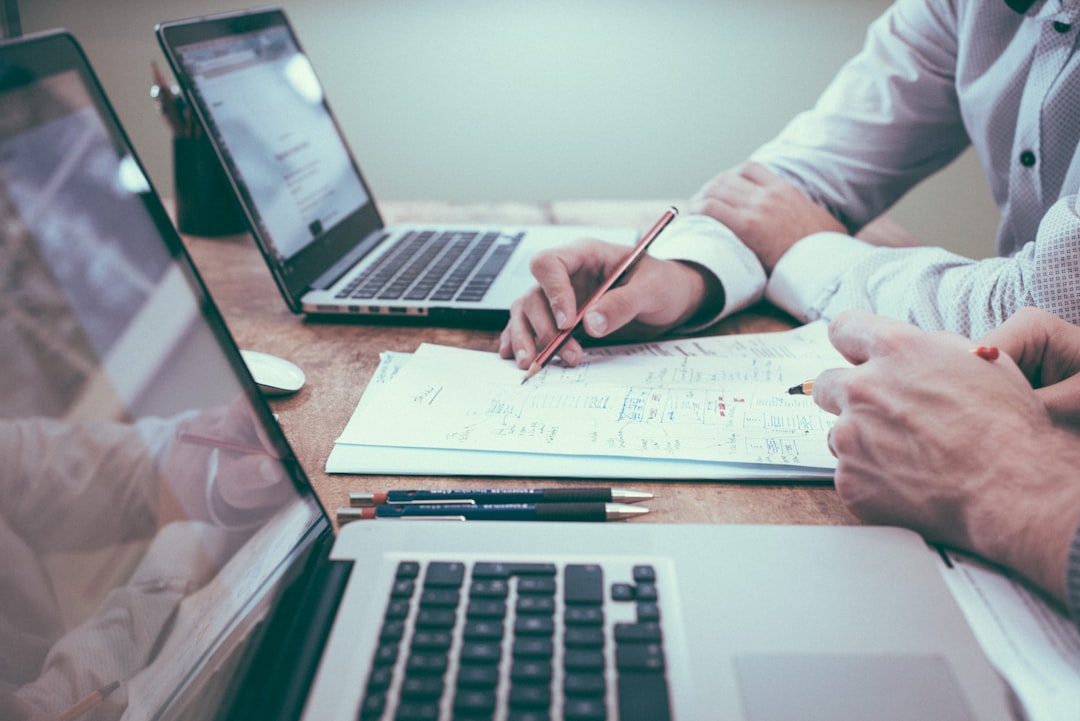
AI is increasingly automating and enhancing video labeling workflows. Tools now facilitate a range of annotation methods, including bounding boxes, segmentation, and lines, all of which are important for creating the high-quality training data needed by AI models. Platforms utilizing AI are helping streamline video labeling, which in turn speeds up the process and lowers costs compared to traditional manual methods. Beyond simply speeding things up, these AI-powered tools are also striving to improve the consistency and precision of the labels themselves, something crucial for building reliable AI systems. As this technology matures, it promises to further refine data annotation practices, ensuring the data used to train AI models is consistently accurate and of the highest quality. While there are gains, there are also risks, like the potential for biases or errors introduced by the automation itself, which must be continuously evaluated.
AI-powered tools are increasingly being used to streamline the process of labeling video data, offering significant improvements in efficiency compared to manual methods. For example, AI-driven labeling can process videos at speeds of up to 100 frames per second, significantly outpacing human annotators who typically work at a rate of 2-10 frames per second. Studies have shown that these tools can decrease the time needed to label large datasets by as much as 80%, freeing up human experts to focus on more complex tasks like model design and evaluation.
Some of these AI-powered tools employ active learning techniques. These techniques prioritize the most uncertain labels, ensuring that human annotators concentrate their efforts on areas where the model is less confident, thereby maximizing the effectiveness of the labeling process. However, it's crucial to acknowledge that not all video annotation tasks are suitable for automation. Tasks that necessitate subjective interpretation, like recognizing human emotions in a video, still present challenges for AI systems to achieve human-level accuracy.
Semi-supervised learning offers a promising path to enhance the value of limited labeled data. This approach leverages both labeled and unlabeled video data, enabling AI models to learn from a wider range of examples without requiring extensive manual annotation. Furthermore, employing heatmaps generated from AI analysis can guide human annotators towards the most critical parts of a video, leading to potential gains of up to 50% in labeling efficiency. This process helps ensure that key data points are not missed during the review.
Certain advanced labeling methods, like instance segmentation, provide higher resolution labeling compared to traditional approaches. For instance, instance segmentation can distinguish between individual objects within a complex scene, allowing for a more granular understanding of the data, which traditional bounding boxes struggle to achieve. However, it is important to recognize that errors in the annotation process can lead to issues in the machine learning pipeline. Mistakes early in the annotation process can skew the results of later evaluations, a phenomenon known as error propagation. This underscores the significance of annotation quality in ensuring reliable AI model outputs.
The integration of 3D data with video can offer richer insights into both spatial and temporal aspects of the data. This technique provides a more comprehensive picture of the scene's dynamics. However, this approach is computationally demanding and requires sophisticated methods for data interpretation. This presents a trade-off between the level of detail attainable and the feasibility of implementing such a labeling scheme. Lastly, it is notable that annotators with specialized domain knowledge, like medical imaging or autonomous vehicles, can significantly enhance labeling accuracy. This underscores the fact that expertise in the application domain is as crucial as technical proficiency when it comes to achieving precise annotations.
Data Annotation Training Unlocking AI Potential Through Precision Labeling - Human-in-the-loop approaches enhancing annotation accuracy
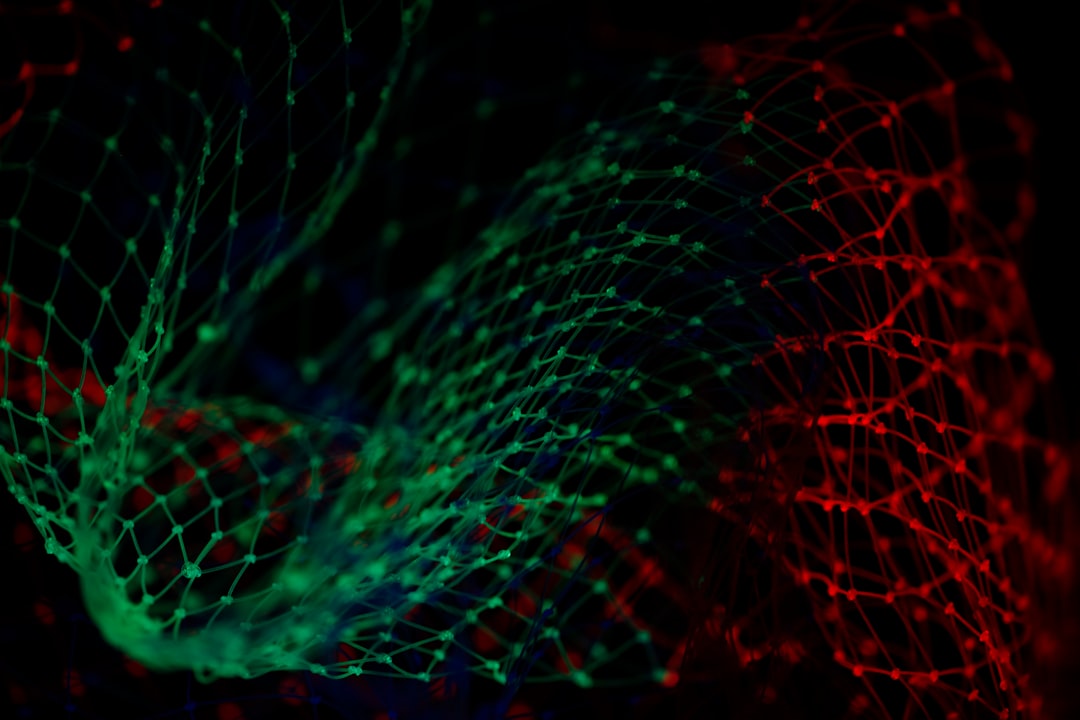
Human-in-the-loop (HITL) methods improve the accuracy of data annotation by integrating human expertise into the process. This involves incorporating human annotators into a feedback loop with AI algorithms, particularly when the AI's confidence in its annotations is low. By incorporating human judgment, especially in areas requiring subjective interpretation, HITL helps reduce errors and biases that can creep into fully automated systems.
The effectiveness of HITL depends heavily on the quality of the human annotators involved. Properly training annotators and providing them with clear guidelines is crucial for consistently high-quality annotations. While this approach can streamline annotation and potentially lower costs, it's important to recognize that maintaining human involvement adds complexity to the workflow.
Essentially, HITL approaches not only enhance the reliability of the training data but also reshape the typical AI development lifecycle by continuously integrating human knowledge throughout the training and refinement stages. This ensures AI systems are not just technically advanced, but also informed by human understanding.
1. Human-in-the-loop (HITL) methods can significantly improve annotation accuracy by allowing human experts to review and refine the labels generated by algorithms, leading to greater confidence in the quality of the training data for AI models. This combination of human understanding and machine learning can address potential biases that automated systems might introduce on their own.
2. Including human feedback in the labeling process isn't just about improving accuracy; it also creates opportunities for ongoing model refinement. As annotators interact with the systems, their insights help refine the algorithms, resulting in increasingly sophisticated models better equipped to handle complex visual situations.
3. Studies suggest a large percentage of machine learning projects fail due to poor data quality, highlighting the importance of having human annotators supervise the creation of datasets. Even small inconsistencies in the labeling process can affect the training process and compromise the quality of results.
4. When human annotators disagree on labels, it can reveal subtle aspects of video content that might otherwise be missed. This variability can lead to the discovery of new categories or details, making the iterative refinement of labels a valuable tool for gaining a deeper understanding of visual datasets.
5. HITL systems often incorporate "explanation interfaces" that guide annotators through the process of labeling complicated scenes. These interfaces can show the AI's confidence level for each label, helping annotators to concentrate on the most unclear areas, which directly contributes to improved data quality.
6. Continuously involving human experts helps uncover rare or unusual situations that might not be adequately represented in typical training datasets, thus leading to more robust AI models. By introducing algorithms to less common examples, annotators can help prevent overfitting and improve AI's ability to generalize across a wider range of situations.
7. Recognizing emotions and understanding context in video can be significantly aided by human annotators, who bring a nuanced perspective that AI currently struggles to replicate. This includes subtle aspects like sarcasm and body language, which automated systems often miss, highlighting the irreplaceable value of human insight in this area.
8. Research suggests improved communication among annotators can enhance consensus and minimize variations in labeling decisions. Tools designed for collaboration and discussion, where annotators can explain their reasoning, have been shown to lead to more consistent annotation.
9. Recent studies have shown that incorporating user feedback into active learning frameworks can improve labeling efficiency, emphasizing the crucial role of annotators as partners in the data labeling process rather than just labelers.
10. While HITL methods generally increase annotation accuracy, they're not without potential downsides. Over-reliance on specific human interpretations could introduce new biases, making careful monitoring and a balanced approach necessary to maintain an unbiased dataset.
Data Annotation Training Unlocking AI Potential Through Precision Labeling - Impact of precise labeling on AI model performance in video understanding
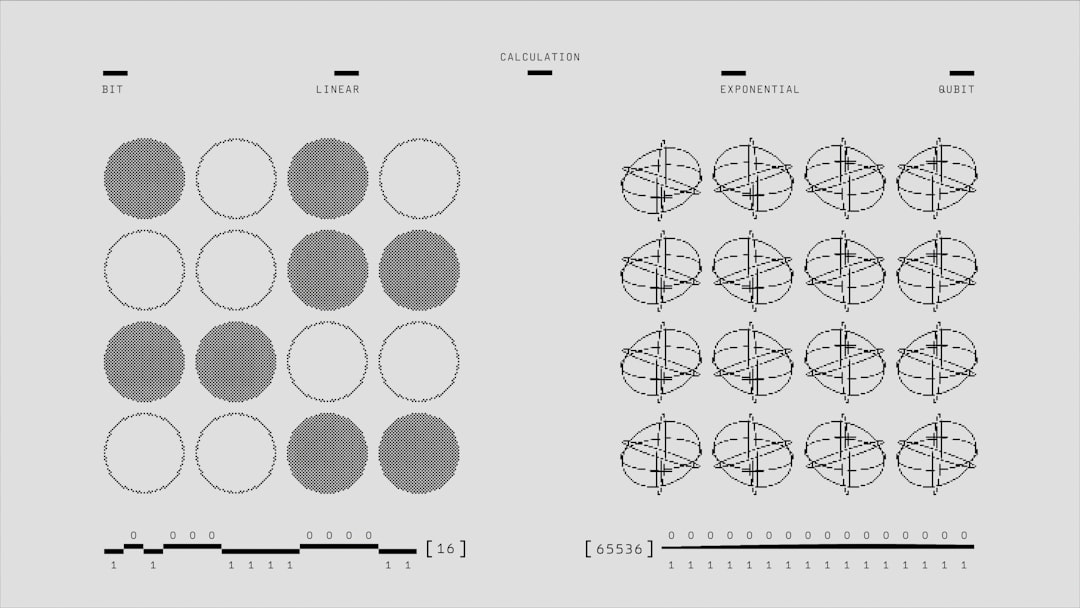
The effectiveness of AI models in understanding video content hinges heavily on the precision of the data annotation process. When training AI to grasp intricate visual patterns, accurate labels serve as the essential foundation, enabling algorithms to learn effectively. However, inconsistent or imprecise labeling introduces significant noise into the training dataset, potentially hindering the model's ability to learn and make sound decisions. The rising complexity and diversity of videos demand ever more meticulous annotation approaches. To build truly reliable AI for video analysis, it's essential to implement robust annotation techniques and leverage the latest tools to ensure the quality and consistency of the labeled data. As the need for sophisticated video understanding grows, precise labeling becomes a critical investment for creating AI models capable of interpreting and analyzing video data effectively.
1. The accuracy of AI models in video understanding is strongly tied to the precision of the labels used during training. Research indicates that even minor labeling errors can decrease model performance by up to 20%, emphasizing the importance of accuracy throughout the labeling process.
2. Maintaining consistent labeling across the dataset significantly impacts model performance. Variations in how elements are labeled can introduce "noise" that confuses the AI during training. Studies suggest that consistent annotation can lead to a 30% increase in the model's learning speed.
3. Including temporal annotations – information about when events occur in a video – can surprisingly improve model prediction accuracy, especially for intricate sequences. For instance, some studies have observed a 25% improvement in predictive accuracy when models are trained with temporal annotations.
4. The level of detail used in labeling can influence a model's performance, particularly in complex scenes. For example, the difference between using instance segmentation (which distinguishes individual objects) and traditional bounding boxes can impact how well a model identifies overlapping objects in a scene by as much as 50%.
5. The ability of human annotators to maintain consistent and precise labels is not unlimited. Cognitive factors like fatigue impact performance, with peak annotation quality typically lasting around an hour. After that point, the chances of errors and inconsistencies in labeling tend to rise.
6. Using AI-generated heatmaps can significantly enhance human annotation efficiency. These heatmaps help annotators focus on the most important or difficult portions of a video, resulting in a potential 40% improvement in overall speed. This approach strategically leverages the combined capabilities of AI and human insight.
7. There's a fascinating interplay between automated labeling and human understanding in video analysis. While automated labeling can be efficient, human annotators often offer subjective interpretations that help models generalize better, particularly in areas like understanding the emotional context of a video.
8. Hybrid systems, often called human-in-the-loop (HITL) systems, can provide significant benefits in the labeling process. These systems aim to leverage the strengths of both humans and AI, achieving a 50% reduction in annotation time while simultaneously improving the quality of the resulting labeled dataset due to human oversight.
9. As video resolutions increase, the challenge of accurate labeling also escalates. Models trained with higher resolution data tend to show up to 15% better accuracy than models trained on lower resolution data. However, this improvement comes at the cost of needing substantially more processing power.
10. The use of collaborative annotation platforms has shown promise in improving the quality of labeled datasets. By bringing together multiple annotators and fostering discussion, these platforms can lead to a 20% reduction in discrepancies and increase the overall agreement on label choices. This collaborative approach taps into the collective knowledge and experience of a diverse group, improving the reliability of the labeled data.
Data Annotation Training Unlocking AI Potential Through Precision Labeling - Future trends in data annotation for video-based AI applications
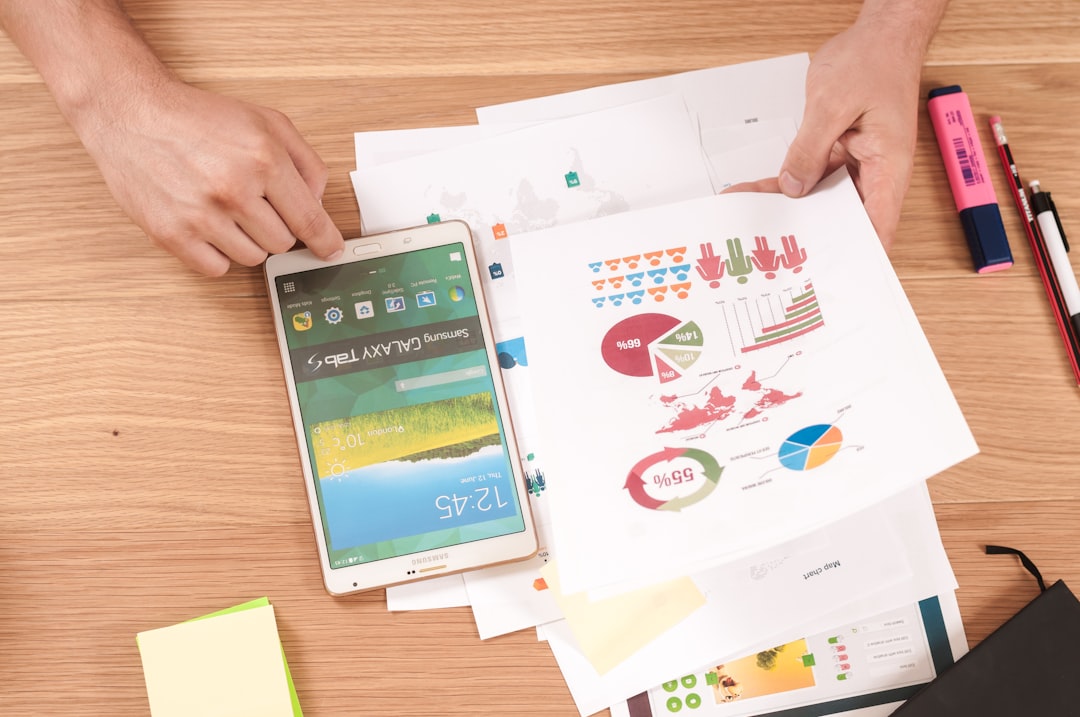
The landscape of data annotation for video-based AI applications is poised for significant changes. We can expect future trends to focus on more accurate and efficient labeling practices. A growing need to incorporate multiple data types, such as audio and sensor data, into the annotation process (multimodal annotation) will likely become commonplace. This shift is driven by a desire to build richer contexts for AI models and improve their overall comprehension of video content. Furthermore, we expect that humans will remain central to the annotation process, with human-in-the-loop techniques gaining prominence. This approach ensures that the human element plays a crucial role in mitigating potential biases or inconsistencies introduced by automated systems.
Innovation in annotation techniques is also likely to drive progress. This includes further development of methods like semi-supervised learning and continued improvement of automated tools. The push for increased efficiency needs to be balanced with the continued need for high-quality annotations, which are essential for building reliable AI models. Finally, as concerns about ethical data collection and usage gain momentum, future data annotation practices will likely face stricter scrutiny and be guided by more explicit guidelines. The demand for high-quality annotated data will be crucial for ensuring the realization of AI's potential in numerous applications, and this will remain a critical area of development.
1. **Novel Approaches**: We're seeing the emergence of AI-driven labeling techniques like zero-shot learning. This intriguing approach allows AI models to identify and classify things they haven't been explicitly trained on, potentially decreasing the massive amount of labeled data typically needed.
2. **Adaptive Labeling**: The future of video annotation seems to be moving towards dynamic labeling. This means the annotations can change in real-time, adjusting to feedback from the AI model itself. This could lead to more adaptive and continuously learning AI systems, as they refine their understanding with new data.
3. **Detailed Annotations**: We're likely to see a greater emphasis on incredibly detailed annotations. It's not just about recognizing objects anymore; we need to capture behaviors and interactions within the video over time. This level of detail goes beyond the capabilities of basic techniques like bounding boxes, presenting both challenges and exciting opportunities.
4. **Interoperability**: Annotation tools are likely to become more integrated with existing APIs. This means developers could seamlessly combine annotations with other types of data (like location information or environmental data) directly, streamlining model training without needing lots of manual tweaking.
5. **Standardization Push**: With AI's increasing importance in various industries, we can expect a growing need for standard annotation frameworks. This will ensure that labels have the same meaning across different research and development teams, making collaboration easier and reducing ambiguity.
6. **Real-Time Feedback**: We might see the rise of real-time feedback loops between AI and human annotators. This would allow annotators to instantly see how the AI is performing and make adjustments as they go, leading to a more interactive and dynamic training process.
7. **Bridging Data Gaps**: For some scenarios, especially those requiring specific actions in specialized areas, there might not be enough real-world video data to train AI models effectively. In response, the use of synthetic data generation is gaining attention. These technologies could create artificial video data for training, effectively filling the gaps where real-world data is limited.
8. **AR's Potential**: Augmented reality tools could play a more significant role in annotation. This would likely improve the understanding of spatial relationships within the video, leading to potentially more accurate and interactive labeling experiences.
9. **Mitigating Human Bias**: Human bias is a well-known potential problem in annotation. Future methods might involve tools that actively monitor the annotators' behavior to try and detect and reduce bias, leading to better labeling quality overall.
10. **Data's Journey**: There's a growing focus on the "provenance" of annotated data. This involves keeping track of how the data has been annotated and modified throughout its lifecycle, promoting the trustworthiness and reliability of the AI training process.
More Posts from whatsinmy.video: