Analyze any video with AI. Uncover insights, transcripts, and more in seconds. (Get started for free)
Exploring 7 Essential Datasets for Mastering Linear Regression Analysis in Video Analytics
Exploring 7 Essential Datasets for Mastering Linear Regression Analysis in Video Analytics - Viewer Engagement Dataset Tracking Watch Time and Interaction
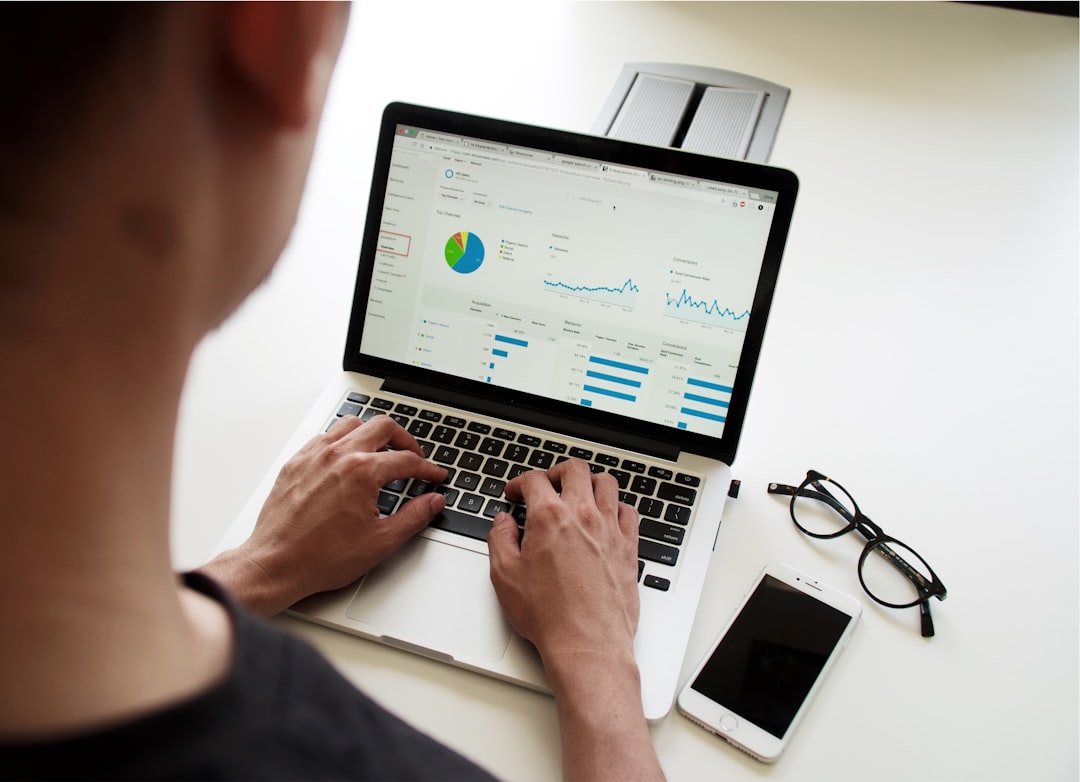
Understanding viewer behavior is paramount in video content creation and distribution. A "Viewer Engagement Dataset Tracking Watch Time and Interaction" focuses on capturing how people engage with videos. It provides a detailed picture of audience interaction, examining key metrics like how long they watch (watch time) and how actively they interact with the video. This kind of data can reveal patterns, helping creators pinpoint moments of high and low audience interest within their videos.
Furthermore, this type of dataset allows for the application of analytical techniques, including AI video analysis, to investigate the underlying reasons for viewer behavior. The impact of social factors and individual viewer motivations on engagement becomes clearer, revealing a level of nuance often missing from simpler engagement metrics. Interestingly, even the basic content structure like whether it's a movie or a series can significantly affect how audiences interact with a video, emphasizing the need to craft content specifically for its intended audience and format. Analyzing viewer engagement in this comprehensive manner provides creators with deeper insights to improve their work and refine their video distribution strategies.
Tracking viewer engagement through datasets can reveal fascinating patterns in how audiences interact with video content. We can identify moments of peak and trough interest by looking at how engagement changes over a video's duration. Metrics like watch time and interaction rates (likes, shares, comments) are vital for understanding how well videos resonate with viewers. While engagement rate itself can be calculated by comparing the total time played to total plays multiplied by the media length, it's important to remember that these figures don't always reveal the full story about viewer loyalty or depth of connection.
Netflix, for example, relies heavily on data analysis to craft a personalized experience for each viewer. The company's intricate dataset lets them optimize content based on engagement signals. However, even outside large platforms, engagement can be tracked on educational videos, too. For instance, with educational videos, engagement might be a binary variable (true/false) representing if a viewer fully watched the video.
Understanding how people engage with videos goes beyond the numbers. It's influenced by social motivations. Viewers might watch for entertainment, a sense of community, or simply to connect with others online. Indeed, a study that tracked 53 million YouTube videos in 2016 underscores how massive the scale of engagement can be across platforms. Also, how people engage differs based on content types. Movies versus short series have different viewing patterns due to their structure and length, suggesting different strategies for engagement may be required depending on the type of content.
It's a fascinating area of exploration because AI-driven video analysis reveals a lot about the things and people that drive engagement, such as brands, events, and personalities. Still, external social factors can also play a role. It becomes clear that simply looking at metrics like watch time might not capture the complexities of how and why people choose to engage with videos. Therefore, deeper research into the audience's motivations is often needed to fully understand engagement in the context of larger social trends and behaviors.
Exploring 7 Essential Datasets for Mastering Linear Regression Analysis in Video Analytics - Content Categorization Data for Genre-based Performance Analysis
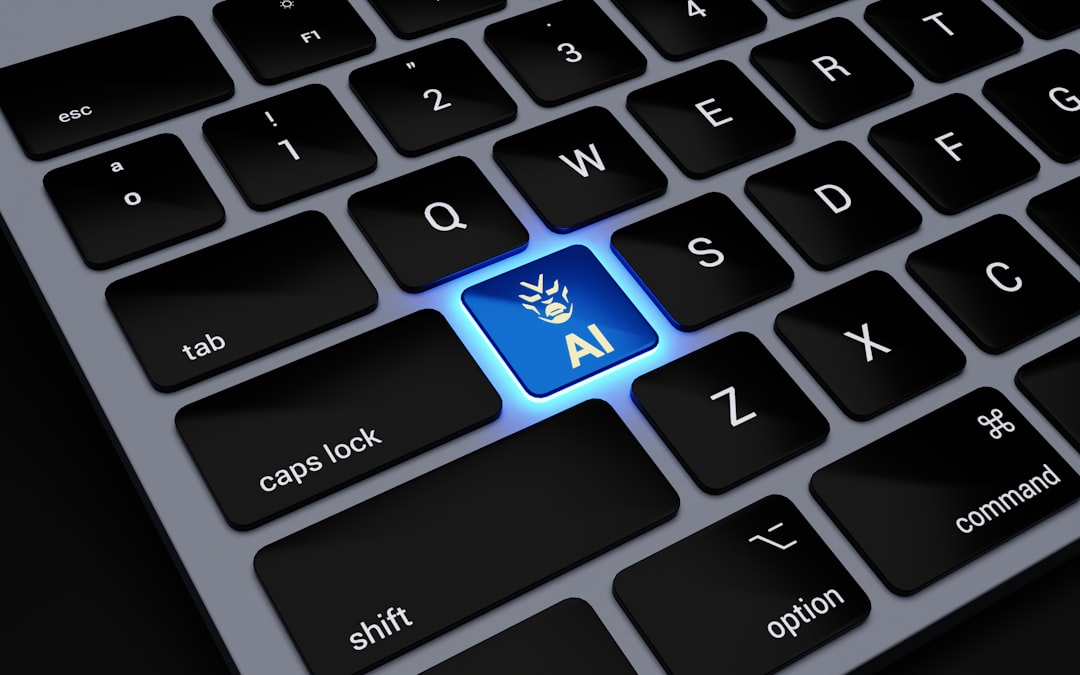
Content categorization data is essential for understanding how different types of video content perform. By categorizing videos into genres, we can analyze how viewers interact with each genre and identify patterns in engagement. This analysis considers not only the structure and function of the content within a particular genre but also its broader social impact on viewers.
This categorization process helps us understand the relationship between content type and viewer engagement, allowing us to apply linear regression to analyze datasets and glean deeper insights. Effectively categorizing content allows for more accurate and nuanced video analytics, leading to improved strategies for content creation. However, creating robust categorization systems requires careful consideration of definitions and frameworks to accurately represent the diverse range of viewer behavior across genres, and the sometimes-conflicting nature of how people engage with videos in different settings. While seemingly straightforward, the complexities of defining genres and how their connection with viewer behaviour can become a challenge, making it crucial to implement a strong methodological approach when employing such data in linear regression models. The insights from analyzing this kind of data can be a strong foundation to improve strategies for creating and sharing content on platforms like whatsinmy.video.
Content categorization data, when applied to genre-based performance analysis, presents a fascinating and complex landscape. It's not as simple as slapping a label on a video; we need to dive deeper. Sophisticated machine learning is often required to capture the nuances of genre, going beyond just themes and into areas like setting and even the way the story unfolds emotionally.
Viewer preferences are a dynamic element. What's popular with younger viewers—think horror and fantasy—might not be as appealing to older demographics, who often favor genres like drama and documentaries. This underlines the need to consider demographic factors when analyzing engagement with different genres. Data analysis has shown that certain genres inherently tend to boost viewer retention. Thrillers and action films, for example, often see higher completion rates due to their suspenseful nature and fast pace.
The emotional impact of genres is a captivating area. Comedy elicits different responses from viewers compared to drama, with each genre triggering specific emotional responses that play a vital role in engagement. This highlights the importance of understanding the emotional landscape of each genre to effectively strategize content creation. The accuracy of genre tags is surprisingly important. Misleading or incorrect labels can significantly affect audience expectations, leading to disappointment and lower retention. Viewers feel misled when the content doesn't match the genre they selected.
Content categorization data directly influences recommendation algorithms on platforms like Netflix and YouTube. The ability to suggest videos based on viewer preferences for specific genres is a critical factor in keeping users engaged. Interestingly, the reception of a genre can vary greatly depending on cultural context. What resonates with one audience might fall flat with another, emphasizing the importance of tailoring content strategies and data analysis based on regional and cultural nuances. It's even possible to break down genres into sub-genres and niches. Defining "psychological thriller" as a specific subcategory allows for targeted promotional strategies to reach a particular audience group more efficiently.
The traditional notion of genres is being challenged by the rise of interactive video content. The lines are blurring as user-driven narratives change the nature of the viewing experience, requiring novel categorization methods to accurately capture this dynamic aspect. We also can't ignore the role of external cultural trends, news events, and societal issues on genre popularity. Data analysis has shown how spikes in viewer engagement for particular genres can align with real-world events, which necessitates adaptability in content strategy for maximizing viewer interest. This constant change reminds us that engagement is not static, and content needs to evolve with the times.
Exploring 7 Essential Datasets for Mastering Linear Regression Analysis in Video Analytics - Video Quality Metrics Dataset Measuring Resolution and Bitrate Impact
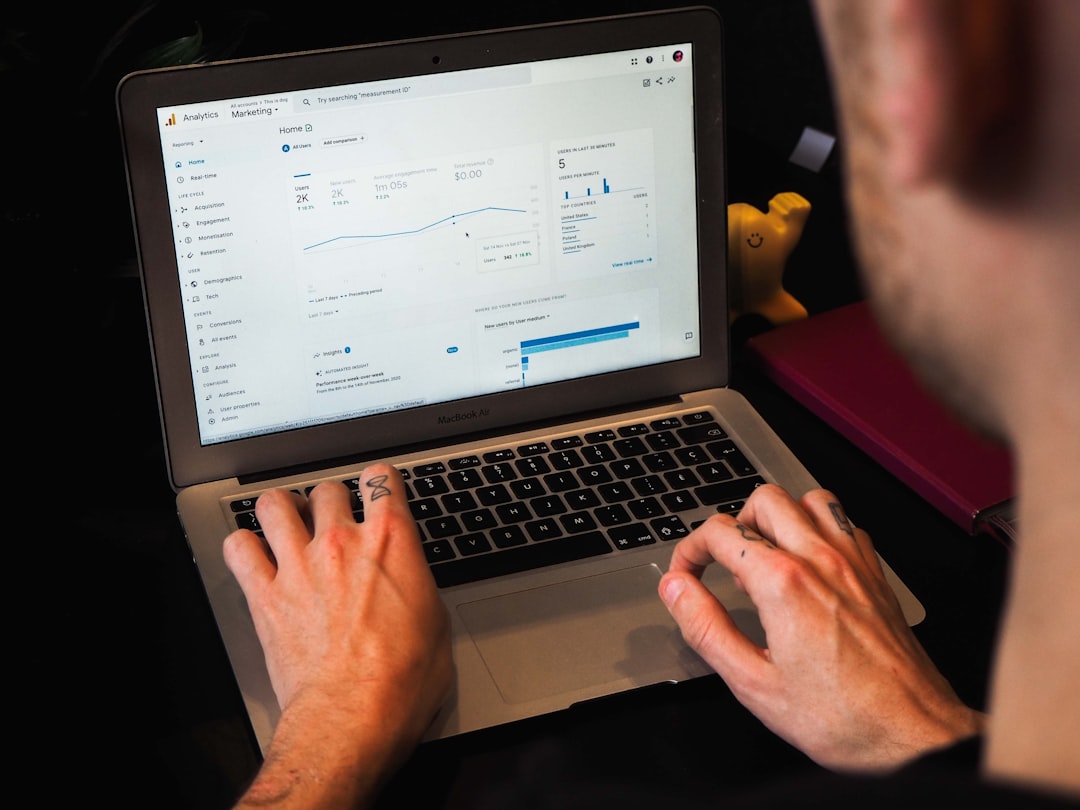
The "Video Quality Metrics Dataset Measuring Resolution and Bitrate Impact" delves into how video quality is affected by resolution and bitrate, considering different video compression standards. This dataset contains a substantial collection of 4K videos, each lasting 10 seconds and evaluated using standard subjective methods. Notably, it moves beyond older codecs like H.264 and H.265 by also incorporating newer standards like AV1 and VVC. The research that generated this dataset suggests that existing studies are somewhat limited in the range of video codecs and distortion types they have considered, leading to a call for more exploration. The dataset, therefore, offers a crucial tool to understand how modern compression techniques impact perceived video quality. This information is particularly useful for those seeking to utilize linear regression models in video analytics to gain a better understanding of the connections between video quality metrics and factors like resolution, bitrate, and codec choices. The combination of human subjective assessment and the inclusion of various codecs makes this dataset valuable for anyone looking to deepen their knowledge of video quality metrics and how they relate to the technical aspects of video encoding and compression.
This dataset, comprising 5,500 4K videos each 10 seconds long, is designed to help us understand how resolution and bitrate impact perceived video quality. It uses subjective ratings based on the ITU-R BT.500-13 standard, offering a valuable way to gauge how humans react to different video settings. It's important because modern video codecs like AV1, VVC, and LCEVC rely heavily on deep learning and perceptual metrics for optimization, but most research so far has focused on older codecs like H.264 and H.265. This dataset, with its focus on higher resolutions, provides a much-needed opportunity to expand our understanding across a wider range of codecs.
We've relied on common objective metrics like PSNR, MS-SSIM, VMAF, and LPIPS in evaluating video quality, particularly in super-resolution techniques. But it's worth noting that there isn't always a strong agreement between these objective measures and what viewers actually perceive as 'good' quality. This new benchmark helps bridge this gap.
A related dataset includes roughly 2,500 video streams encoded using various standards including AVC, HEVC, AV1, VP9, and VVC. Examining these encoded streams using tools like the MSU Video Quality Measurement Tool (VQMT) lets us compare how different compression methods impact quality. VQMT is particularly useful as it allows for both reference and non-reference metrics, leading to more detailed analysis.
Interestingly, we've found that the effectiveness of a video quality assessment method often relates to the codec used to compress lower-resolution videos. This suggests that our understanding of how these metrics work may need adjustments depending on the video's initial compression. Furthermore, not many codecs have been thoroughly evaluated alongside subjective evaluations, making this dataset valuable.
Essentially, what we hope to get out of this dataset and associated benchmarks is a clearer picture of how compression standards affect what people see as good quality video. This knowledge is also important for understanding how reliable video quality metrics truly are in this context.
However, it's important to note some limitations and future considerations. While the dataset helps us understand the relationship between resolution, bitrate, and viewer perception, there can be inherent biases in the subjective evaluations. People might tend to favor higher resolutions and bitrates, potentially leading to skewed results. We also need to consider that factors like lighting and motion within a video can affect quality perception. These findings emphasize the need for a more holistic view of video quality assessment.
Furthermore, access to video content and internet infrastructure differs globally. Someone in a developed country might be much more likely to have access to 4K streaming compared to someone in a developing country. These geographic factors will undoubtedly impact how people experience video and need to be considered during the analysis.
It's also important to consider that there may be a ‘quality fatigue’ phenomenon at play. If people become accustomed to very high quality, they might not be as sensitive to further improvements. This implies that video delivery strategies might need to continually evolve to remain engaging.
Finally, the rapid development of VR and AR technologies makes it crucial to consider how these types of immersive experiences differ from more traditional formats. This dataset, while valuable, provides a strong foundation for further research into the unique challenges of immersive video quality. This research will be important for designing future streaming and viewing technologies that cater to these evolving needs and viewer expectations.
Exploring 7 Essential Datasets for Mastering Linear Regression Analysis in Video Analytics - User Demographics Data for Audience Segmentation Insights
User demographics data provides a crucial foundation for segmenting audiences within video analytics. By examining characteristics like age, gender, location, and income levels, we can gain a deeper understanding of viewer behavior and preferences. This information is instrumental in developing more effective marketing strategies that resonate with specific audience segments. For instance, understanding that younger viewers tend to favor horror and fantasy while older viewers gravitate towards dramas and documentaries allows for tailored content creation and promotion.
Furthermore, the insights derived from user demographics help in fine-tuning customer journey analysis and campaign design. We can optimize marketing efforts by tailoring messaging and content to align with the specific needs and interests of distinct demographics. The ability to create dynamic audience segments, which adapt automatically as new data becomes available, ensures that our understanding of the audience remains current. While seemingly straightforward, it's crucial to remember that successful segmentation relies on robust data collection and careful interpretation. Failing to gather and interpret data correctly can lead to inaccurate insights, hindering efforts to create truly impactful campaigns. The use of demographic insights, however, can be a significant step towards achieving a higher level of understanding in how we reach and influence specific audiences.
User demographics data is a valuable resource for understanding audience behavior and preferences, which is essential for effective audience segmentation. By grouping viewers based on shared traits like age, gender, or occupation, we can tailor content and marketing efforts more precisely. This kind of segmentation can lead to a deeper understanding of the customer journey.
Tools like Usermaven, designed for this type of audience analysis, can create dynamic segments that update automatically as new data arrives, ensuring that the analyses reflect the most current trends in viewer behavior. This continuous update feature is crucial, since viewer preferences can change rapidly, especially for younger audiences.
A great illustration of this approach is Coca-Cola's "Share a Coke" campaign, which targeted teens and young adults. This campaign demonstrated the power of creating content that resonates with specific demographics. However, it's important to note that effective segmentation relies heavily on the quality of the data being collected. A thorough and well-defined approach to gathering and analyzing the data is necessary, particularly when determining which questions to ask and selecting relevant audience samples.
It's easy to get caught up in the sheer volume of data, but the true value comes from how it informs strategic decision-making. Going beyond simply compiling raw numbers, interpreting the data is critical. For instance, creating detailed "customer personas" based on demographics allows marketers to understand the unique needs and preferences of different audience segments. This level of understanding is essential to create more relevant and effective marketing messages.
The language and messaging used in marketing campaigns should align with the target audience. This approach can significantly enhance engagement and conversion rates. It's about speaking to the specific concerns and motivations of different groups.
Ultimately, demographic segmentation is a foundational element of audience segmentation strategies. It helps businesses develop targeted and impactful marketing campaigns. While it's a powerful tool, it's crucial to be aware of its limitations. Factors like cultural differences, socioeconomic status, and evolving technological landscapes can influence how people interact with content. Understanding these factors can lead to a better segmentation strategy.
However, we need to be mindful of how these insights are used. The goal should always be to create a more engaging experience for viewers, not to reinforce stereotypes or exploit vulnerabilities. Furthermore, the rapid changes in the media landscape require a flexible approach to segmentation. It's not a one-time activity, but a continuous process that needs to adapt to evolving audience needs and expectations. We must stay cautious and strive for an approach that respects users' privacy and data security while using this information responsibly.
Exploring 7 Essential Datasets for Mastering Linear Regression Analysis in Video Analytics - Temporal Viewing Patterns Dataset Capturing Time-of-Day Effects
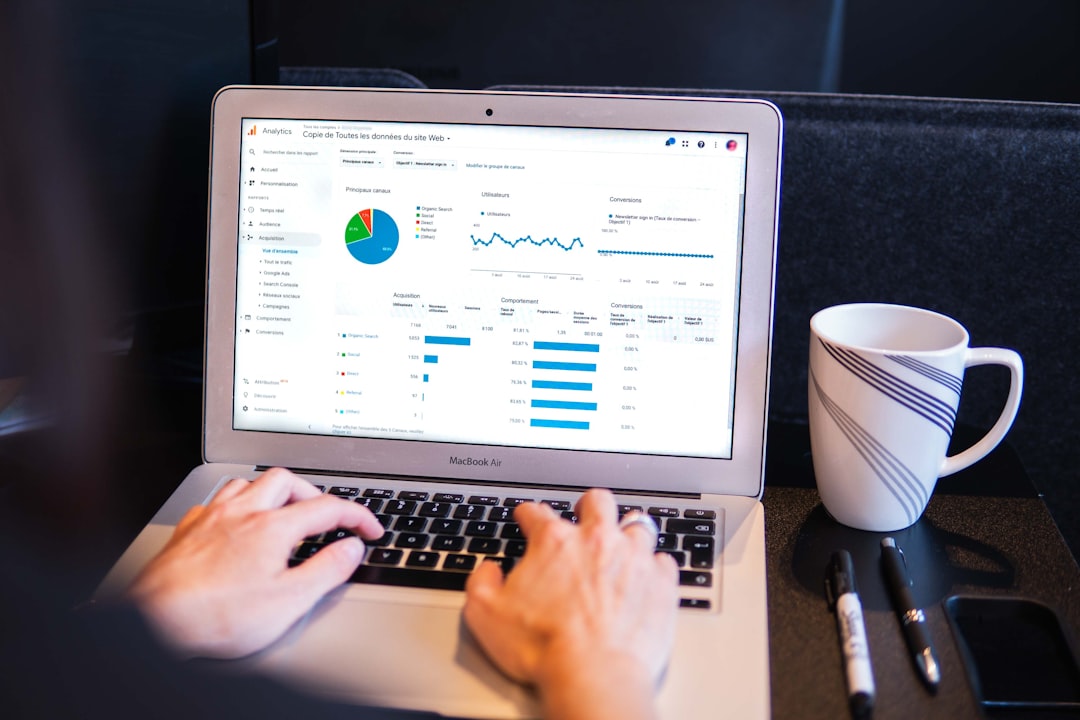
A "Temporal Viewing Patterns Dataset Capturing Time-of-Day Effects" focuses on how the time of day impacts viewer behavior and engagement with video content. It emphasizes that analyzing viewing patterns over time is crucial for understanding audience dynamics within video analytics. This dataset highlights how the time of day can influence the type of content preferred by viewers, providing valuable insights for content creation and distribution decisions.
Understanding the specific times when viewers are most engaged with certain content types offers a more nuanced view of viewer interactions. This dataset reveals that viewer engagement can vary significantly based on time, impacting how we interpret overall engagement trends. By incorporating the time-of-day factor, it becomes possible to optimize content delivery strategies to capitalize on periods of peak engagement. It's a reminder that viewer preferences and engagement levels are not static, but fluctuate throughout the day, making temporal data analysis a vital part of comprehensive video analytics. Ultimately, this dataset allows for a more precise analysis of viewer engagement by factoring in the crucial element of time, enhancing our overall understanding of viewer behavior.
### Temporal Viewing Patterns Dataset Capturing Time-of-Day Effects
This dataset is fascinating because it shows how people's engagement with videos changes throughout the day, largely due to our natural body rhythms (circadian rhythms). It's interesting to consider how this biological aspect influences how we interact with media. We see a clear trend of higher viewership in the evenings, particularly between 7 PM and 10 PM, suggesting that strategically releasing content or running promotions during those hours could be beneficial.
However, it's not just about overall viewing. The types of content that people watch also seem to change depending on the time of day. Perhaps lighthearted content performs better during the day, while darker, more intense genres like horror might have their peak in the evening hours. It makes sense when you think about it – our mood and energy levels shift throughout the day, and the content we choose may reflect that.
This dataset also lets us do some very specific behavioral analysis by segmenting viewer activity based on the time of day. We can identify which demographic groups become more engaged in viewing at different times, which could be very useful for tailoring content recommendations and other personalized experiences.
It's not just routine viewing that's affected by time. Unexpected global events or major news can disrupt these typical patterns. We might see a spike in engagement with live news or crisis-related content during those periods, highlighting how quickly we need to adapt our video analytics methods to capture these changes.
One intriguing observation is the drop in engagement during the early morning hours. There's a research suggestion that people are less likely to engage with longer-form content before their day gets started. It makes us question when the optimal time might be for longer videos or series releases.
We also find that people take longer to respond to content posted in the early morning hours compared to something put out in the evening. It demonstrates how the timing of content release can actually impact the speed of the feedback loop.
Mobile devices also play a role in changing these temporal viewing patterns. Viewership spikes during specific times can be different based on whether someone's using a phone or a computer, reminding us that mobile access and lifestyle patterns are shaping how people engage with video.
This time-of-day effect isn't just about behavior; it's tied to our psychology. Our emotional and mental state seems to influence what we choose to watch. It goes beyond just what's available; people are making content choices based on how they feel at a particular time.
As we rely more on AI and machine learning in video analytics, understanding these temporal viewing patterns can empower us to make predictions about viewer behavior. This could have a significant impact on how we approach marketing, content scheduling, and release strategies in the future. It's a fascinating area of research and a key to understanding how we consume video in the modern world.
Exploring 7 Essential Datasets for Mastering Linear Regression Analysis in Video Analytics - Platform Performance Data Comparing Mobile vs Desktop Usage
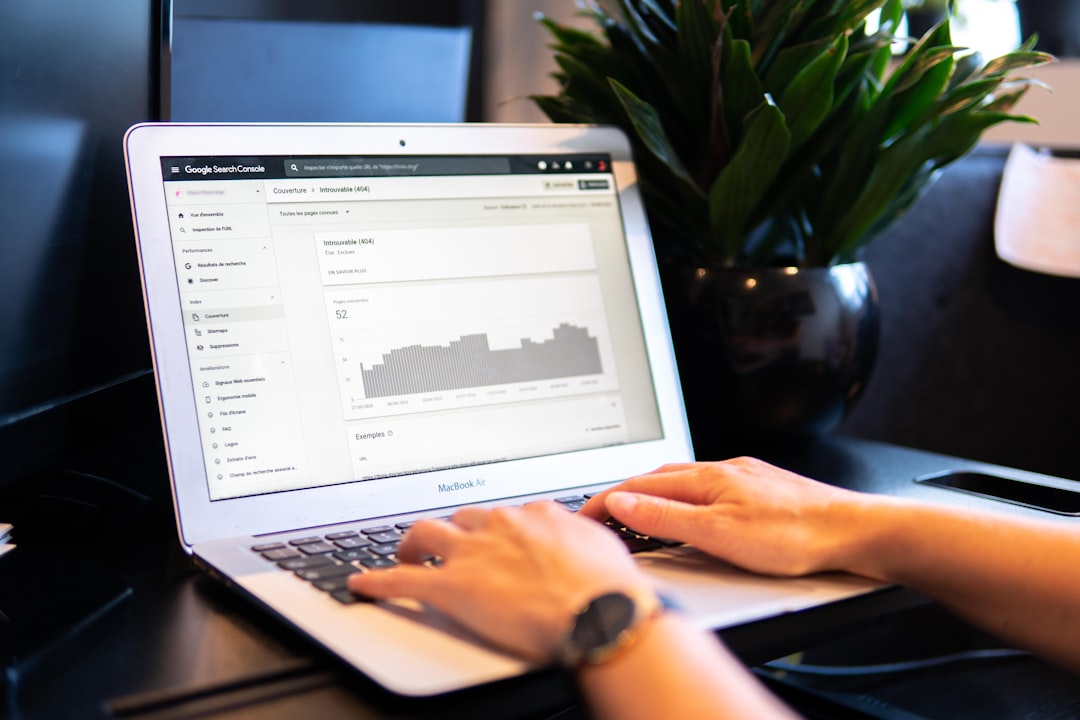
Data on platform usage reveals a substantial shift towards mobile internet access, a trend expected to solidify in 2024 with mobile usage exceeding desktop. This change mirrors a broader societal shift towards on-the-go convenience and accessibility. While mobile adoption is surging, it's important to acknowledge the performance differences between platforms. Mobile users often experience slower webpage loading and higher rates of abandoning a site (bounce rates) compared to desktop users. This is highlighted by longer average load times and a lower conversion rate for mobile visitors versus desktop users. Consequently, businesses need to prioritize optimization for mobile users, yet at the same time address the performance limitations related to mobile browsing, if they want to achieve a balance between user expectations and strong engagement across both desktop and mobile platforms. The goal is to provide a smooth and effective experience across both to fully benefit from the growth in mobile usage while mitigating the potential downsides of that shift.
The shift towards mobile internet access is undeniable, and it's fascinating to observe how this impacts how people consume video content compared to traditional desktop usage.
It's interesting to see that mobile users generally have shorter viewing sessions, sometimes as much as 40% shorter than on desktop. This could be due to the fact that people are more likely to be casually browsing on mobile, possibly in transit or during breaks, leading to more frequent interruptions. Desktop users, on the other hand, often engage in more focused, dedicated viewing sessions.
This context-based usage also impacts the effectiveness of ads. Mobile ads often suffer from higher skip rates, around 25% higher than those seen on desktop, likely due to the smaller screen size and higher potential for distractions. It makes sense that ads tailored for mobile users would need to be crafted with careful consideration for these factors.
Interestingly, mobile users seem to be more accepting of lower video quality, likely due to bandwidth limitations. We often see mobile users favoring 480p or 720p, while desktop users with consistently high-speed connections are more likely to seek out 1080p or higher resolutions. It raises questions about how these technical limitations shape content preferences and accessibility for mobile users.
Also, there are clear differences in interaction patterns. Users tend to interact with content, through things like comments and shares, much more on desktop than on mobile, about double the rate. This hints at a possible shift in how people engage with content based on the platform.
There's also the matter of content type preferences. It's intriguing how some content genres seem more suited to certain platforms. For instance, educational videos seem to do better on desktop, while entertainment-focused content seems more popular on mobile. It makes you wonder if this reflects people's intentions and habits when using different devices.
Generational preferences also play a role. Younger audiences are shifting to mobile as their primary viewing platform, with Gen Z strongly leaning toward mobile devices. Older audiences, however, are more inclined to stick with the more traditional desktop experience. It will be interesting to see how these viewing patterns evolve as younger generations grow older and perhaps shift habits.
Desktop users often have longer viewing sessions, sometimes double the duration of mobile sessions. This may be tied to how they might often watch multiple videos in a row, while mobile sessions are more likely to be shorter, single-video experiences.
Search behavior differences are also telling. Mobile users often engage in impulsive viewing, perhaps catching trending content or viral videos. Desktop users, conversely, are more likely to engage in planned viewing, searching for specific content or revisiting past favorites. It seems to underline that the viewing experience on mobile is different than on desktops.
What's particularly interesting is that a notable percentage of users—roughly 30%—move between platforms during a viewing session. Maybe they begin on their mobile device and finish on their desktop or vice versa. It suggests that there's a strong need for a seamless experience across platforms to cater to this behavior.
In conclusion, while the shift towards mobile internet is a significant trend, there's a lot of nuance in how platform usage impacts video consumption habits. Understanding these differences is crucial for both video creators and platforms hoping to improve user experience. It is a space of great change and a fascinating area for further exploration.
Exploring 7 Essential Datasets for Mastering Linear Regression Analysis in Video Analytics - Social Media Sharing Dataset Correlating Virality with Video Metrics
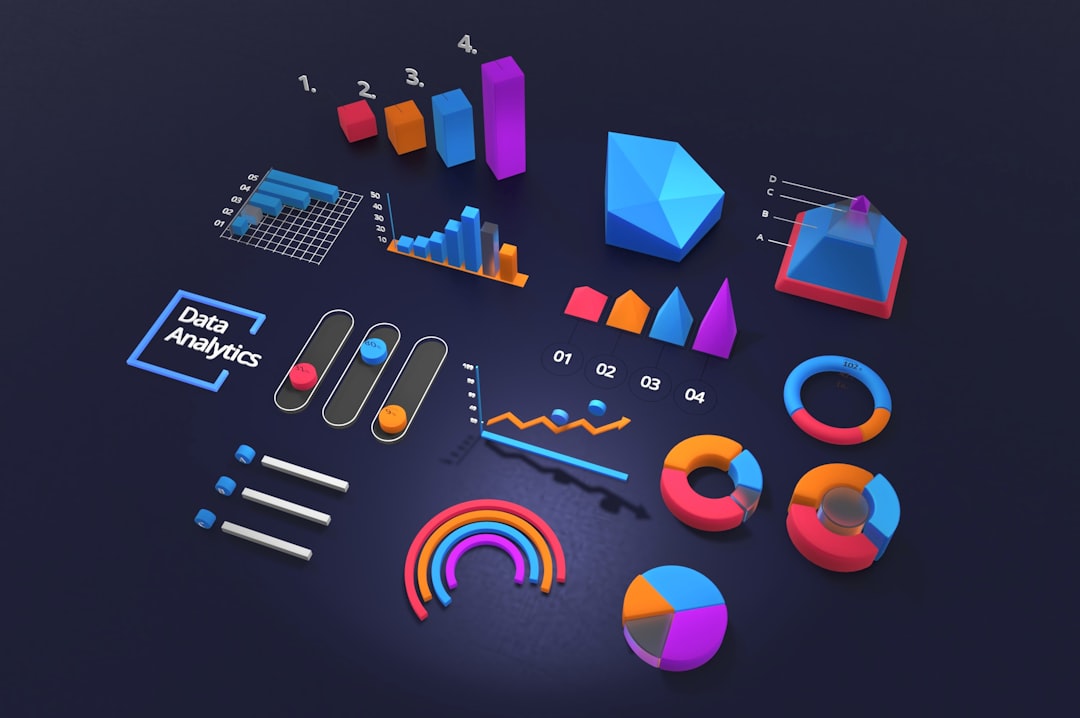
This dataset focuses on understanding what makes video content go viral on social media. By examining over a million tweets, specifically those that aren't retweets, researchers can isolate factors that truly drive viral sharing. The dataset looks at how things like multimedia elements, topical relevance of current events, and even emotional triggers in the content affect how often people share videos on platforms like Twitter and YouTube. It suggests there's a complex interplay between video characteristics and viewers' psychological responses that determines whether something becomes viral. Interestingly, the analysis shows that understanding these dynamics might require looking at data from different platforms, like YouTube and Twitter, to get a clearer picture of how video content spreads. This dataset offers a way to study the connections between various video metrics and the resulting social sharing, helping creators and analysts better understand what leads to viral success.
This particular dataset is quite interesting as it focuses on how videos become popular on social media. It's a bit different from simply looking at how many people watch a video; instead, it dives into the concept of "virality." It seems like virality isn't just about how many viewers a video gets, but also how engaged those viewers are. Things like comments, shares, and likes—those engagement metrics—appear to be far more important than just the raw view count in determining if something will go viral.
One of the more surprising findings is that videos have a very small window of opportunity to become popular. It seems that if a video doesn't get a lot of shares within the first few hours of being posted, it's less likely to go viral later on. It highlights the importance of timing for social media strategy. There's a lot of emphasis on the quick feedback loops associated with social media.
Another curious observation is the impact of influencers. If a video gets a share from a well-known or popular social media user, it's much more likely to go viral. Some studies suggest a single share from an influencer can boost views by a huge amount (over 150%) in a short time, indicating just how much these types of shares impact potential reach.
Interestingly, the kind of content plays a part in virality, too. Things like humorous videos or ones that teach people something tend to get shared more than, say, ads. It makes you wonder if there's a push toward content that viewers find valuable.
Emotional impact seems to be a strong factor. Videos that get a strong emotional response from viewers—whether it's joy, surprise, or even anger—get shared a lot more. It suggests that creating content with strong emotions might be more successful than simply making a highly produced video.
Thumbnail images seem to influence shares, too. If the thumbnail is eye-catching and compelling, it leads to a significant jump in shares, suggesting it’s very important for people to quickly grasp what a video is about.
Different platforms seem to work differently. For example, the dataset shows that videos shared on a platform like TikTok become popular much faster than on older platforms like Facebook, suggesting creators need to tailor their strategies to the specifics of the platform.
The role of user-generated content is notable, as well. Sometimes, videos made by everyday people that resonate with viewers can become hugely popular and get more shares than professionally made videos. It shows how authenticity can be very appealing in today's media landscape.
Hashtags are important, too. Videos that utilize trending hashtags often reach more people, boosting their chances of going viral. It makes sense, as using hashtags can greatly improve a video's searchability and discovery.
Another point from the dataset is that social proof seems to be a significant factor in video sharing. People are much more likely to share a video that their friends or others in their social circle have already engaged with. This reveals how social influence can be used as a multiplier for content reach.
Overall, this dataset is valuable for understanding how videos gain popularity on social media and why some content succeeds where others fail. It's a glimpse into the intricate dynamics of online sharing and demonstrates that simple metrics like view counts are only part of the equation. Factors like engagement, emotional impact, content type, and social proof play a significant role in the journey from video to viral sensation. Understanding these aspects can be invaluable for anyone trying to craft content that is both engaging and widely shared. There's still a lot to be learned about how social media virality works, but this dataset provides a strong basis for creating compelling content that resonates with online communities.
Analyze any video with AI. Uncover insights, transcripts, and more in seconds. (Get started for free)
More Posts from whatsinmy.video: