Exploring the Role of Lowpass Filters in Video Noise Reduction A 2024 Perspective
Exploring the Role of Lowpass Filters in Video Noise Reduction A 2024 Perspective - Evolution of Lowpass Filter Technology in Video Processing
The journey of lowpass filters in video processing has been marked by a steady pursuit of better noise reduction and enhanced image quality. Early filter designs have given way to more sophisticated approaches. For instance, the emergence of compact Butterworth filters, designed specifically for video frequencies, has minimized power usage while retaining a high level of signal fidelity. This drive for efficiency has also spurred innovations like chopper-stabilized filters, which achieve noise reduction without the complexities of traditional amplifier-based designs. Moreover, the ability to combine lowpass filters with other filter types, such as highpass or bandpass filters, enables a greater degree of customization in addressing diverse video content and noise characteristics. As we move forward, the evolving nature of video processing, especially in the context of 2024's anticipated technological advancements, will likely demand further refinements to lowpass filter technology. This includes pushing the boundaries of noise reduction within increasingly intricate and demanding video environments.
The journey of lowpass filters in video processing started with analog circuits, which, while functional, suffered from limitations in their ability to precisely control frequency response and transition bandwidth. The advent of digital processing in the 1980s, alongside the Fast Fourier Transform (FFT), significantly advanced filter design. It allowed for much finer control over frequency components, pushing the boundaries of what was achievable with analog techniques.
Oversampling emerged as a crucial strategy in digital lowpass filters. It's particularly important for video capture and playback as it helps reconstruct signals accurately while minimizing the introduction of unwanted artifacts, like aliasing.
Modern lowpass filter designs often leverage adaptive algorithms. This means they can dynamically adjust their behavior in response to the video signal, enhancing noise reduction without compromising image details. The development of the Discrete Cosine Transform (DCT) created a link between lowpass filtering and video compression standards like JPEG and MPEG. DCT became a pivotal part of how these formats work, showcasing a tight integration of signal processing with the demands of data efficiency.
Beyond DCT, wavelet transforms have shown significant promise. They can offer a more nuanced approach to noise reduction and detail preservation compared to traditional lowpass methods, indicating a fertile ground for future research and development. Researchers have also embraced machine learning to design lowpass filters that can adapt to specific video content. This 'intelligent' approach allows for tailored noise reduction, thereby minimizing artifacts that could arise from applying a universal filter across vastly different video sources.
Furthermore, nonlinear techniques like median filtering have risen in prominence due to their impressive ability to tackle noise while preserving important features like edges. This is especially valuable in scenarios with low light, where noise is more prevalent. The steady increase in processing power has been instrumental in refining real-time lowpass filter design. Complex algorithms can now be implemented 'on the fly' during video streaming, eliminating the need for separate pre-processing steps.
Looking towards the future, there's considerable interest in photonic based lowpass filters. They hold the potential for accelerating video processing and reducing latency, especially crucial for high-resolution video environments. While still in development, they may fundamentally alter how lowpass filtering is integrated into next-generation video systems, offering a new frontier in video quality and performance.
Exploring the Role of Lowpass Filters in Video Noise Reduction A 2024 Perspective - Impact of Butterworth Filters on High-Frequency Noise Reduction
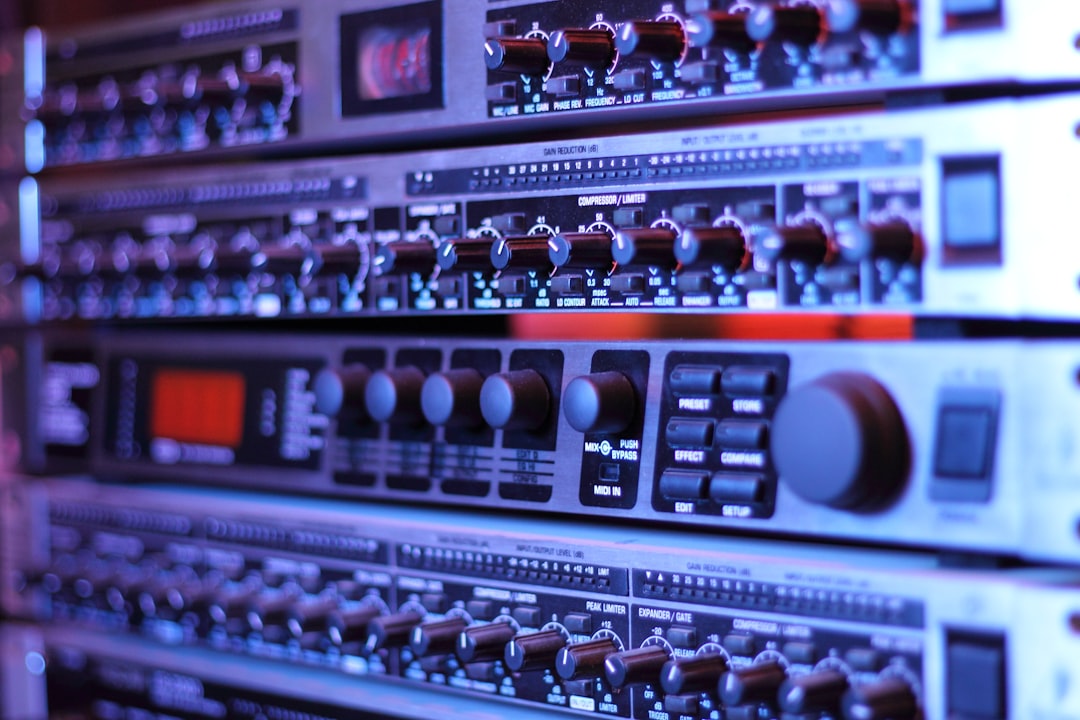
Butterworth filters are valuable for mitigating high-frequency noise, primarily due to their smooth frequency response and adaptable design. Engineers can tailor the filter's cutoff frequency and order, enabling fine control over which frequencies are attenuated. This makes them useful in various applications where maintaining signal clarity is crucial, including video processing. The capability of digital Butterworth filters to handle different signal types, such as those in electrocardiography (ECG), demonstrates their versatility in modern noise reduction systems.
While effective, Butterworth filters have a gradual roll-off characteristic, meaning they don't always achieve the sharpest noise reduction. In situations demanding more aggressive high-frequency attenuation, filters like the Chebyshev may be preferable. As the need for improved video processing persists, understanding the unique strengths and potential shortcomings of different filtering options, including the Butterworth design, is crucial. This careful consideration helps ensure the selection of the most suitable filtering approach for specific video processing tasks.
Butterworth filters are recognized for their ability to effectively diminish high-frequency noise in various signal processing scenarios, including video. Their unique characteristic is a maximally flat frequency response in the passband, which minimizes the introduction of phase distortions. This quality is vital for video, where preserving temporal and spatial coherence is crucial for avoiding noticeable visual glitches.
The precision with which we can establish the cutoff frequency significantly impacts the effectiveness of Butterworth filters in noise reduction. Even minor deviations in this setting can lead to substantial differences in how high-frequency noise is filtered, highlighting the importance of accurate filter design and system calibration.
The order of a Butterworth filter is another key factor, influencing both its sharpness in transitioning from the passband to the stopband and the complexity of its implementation. Higher-order filters, while offering a sharper transition and better suppression of noise, come with a trade-off of increased computational demands and a potential for instability.
In comparison to filters like Chebyshev or elliptic, Butterworth filters generally have a gentler roll-off characteristic, which means they might not be as effective in rapidly attenuating frequencies outside the passband. This characteristic underscores the need to carefully evaluate the nature of the video noise when selecting the optimal filter for a specific application.
Integrating Butterworth filters into video systems can sometimes introduce unforeseen complexities due to their interactions with other processing stages, particularly compression algorithms. These interactions can sometimes lead to unexpected artifacts, reminding us of the importance of a holistic understanding of the entire system when designing filters.
The computational efficiency of Butterworth filters makes them well-suited for real-time video processing tasks. However, it's important to acknowledge that efficiency is context-dependent and varies with the filter's order and implementation method. Therefore, a thorough performance analysis during the design stage is crucial for meeting real-time demands.
The design process for a Butterworth filter involves a balancing act between noise reduction and the desired response time of the video processing system. Employing an excessively aggressive filter can potentially eliminate desirable high-frequency details in the video, ultimately degrading image clarity, particularly in scenes with significant motion.
In many advanced noise reduction systems, Butterworth filters are frequently used as initial filtering stages. This role highlights their foundational importance, preparing signals for further refinement in subsequent processing steps.
The choice of sampling rate plays a crucial role in the efficacy of Butterworth filters in suppressing high-frequency noise. Insufficient sampling rates can introduce artifacts like aliasing, which can undermine the effectiveness of the filtering process. Consequently, meticulous system design, including a careful selection of sampling rate, is necessary for optimal results.
Integrating Butterworth filters with machine learning techniques is a promising research direction, potentially allowing us to design adaptable filters. These adaptive filters could not only adjust to different video content but also dynamically respond to changing noise conditions in real-time. This exciting field suggests a future where video processing systems become increasingly intelligent and robust.
Exploring the Role of Lowpass Filters in Video Noise Reduction A 2024 Perspective - Advancements in Signal Accuracy through Lowpass Filtering
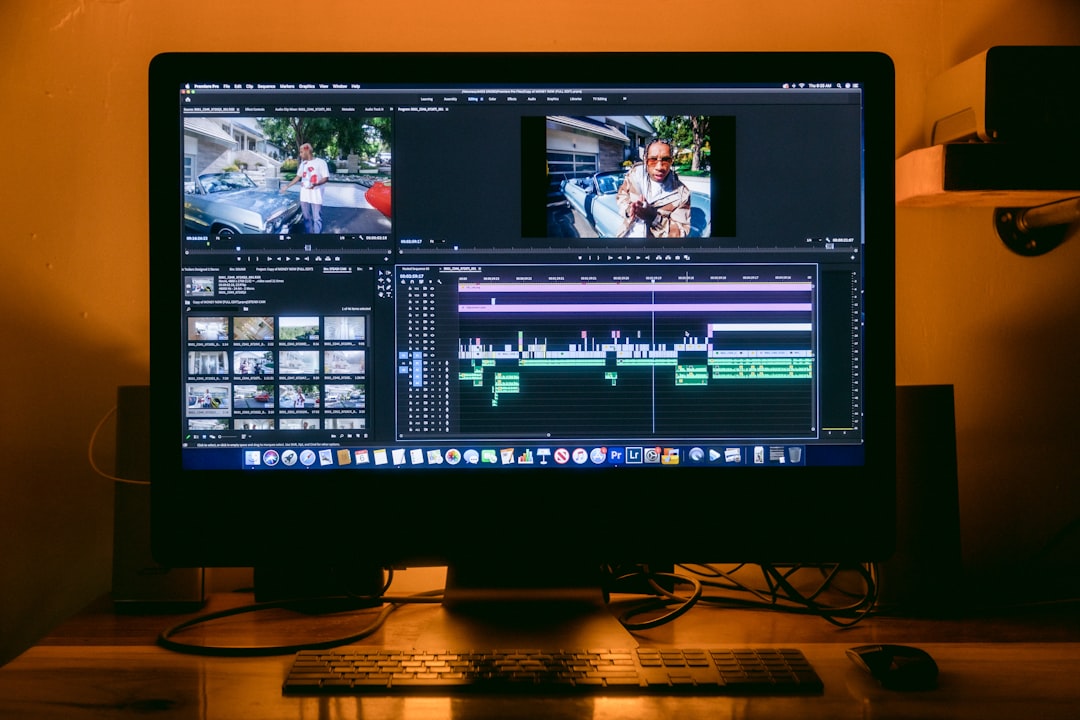
Advancements in signal accuracy through lowpass filtering are a direct result of the increasingly sophisticated demands of video processing. The effectiveness of lowpass filters in reducing high-frequency noise, particularly those with Gaussian designs, is now well-established. These filters are appreciated for their ability to minimize noise while retaining crucial signal information. The capacity for real-time adaptation, particularly within adaptive filter techniques, provides significant improvements. These filters can dynamically change to match the noise levels present, allowing for more optimal performance across a range of scenarios. Furthermore, newer approaches such as machine learning and adaptive algorithms show promise in furthering refining filter design. This suggests a potentially transformative future for signal accuracy in the realm of video applications. As lowpass filtering technology progresses, it's vital to rigorously evaluate filter performance and how they interact with other stages in the processing chain to ensure the best possible results. There are complexities and limitations that need to be recognized in this process.
Lowpass filters have undergone a significant transformation with the advent of digital signal processing. We've moved beyond the limitations of analog designs, enabling sharper frequency responses and reduced phase distortions in our filters. This precision is critical for maintaining signal fidelity, especially in video processing.
However, the success of lowpass filtering hinges on careful attention to the sampling rate. Neglecting the Nyquist theorem can introduce aliasing, a distortion that significantly degrades video quality by introducing unwanted artifacts. This emphasizes the importance of proper system design and calibration.
Modern approaches often leverage adaptive filtering techniques. These filters are designed to dynamically adapt to the unique noise and content characteristics of the video in real-time. This flexibility significantly enhances robustness, allowing them to handle a wider range of noise environments effectively.
But, it's not just about the filters themselves. How they interact with compression algorithms is a crucial aspect. Their integration can sometimes lead to unexpected artifacts that compromise the visual quality of the final video. It's a reminder that a holistic system understanding is needed when designing filters to avoid introducing unintended issues.
Wavelet transforms have emerged as an attractive alternative to traditional methods. Their multi-resolution analysis capabilities offer a more refined approach to separating noise from vital details across various scales within the video signal. This makes them promising for applications requiring precise noise reduction.
The field of machine learning is also beginning to have an impact on filter design. Researchers are using machine learning to create "intelligent" filters that learn from the characteristics of specific video content. These filters can then adapt to optimize noise reduction while minimizing damage to crucial image details. This is a fascinating area, with the potential to create more robust and efficient filtering solutions.
The order of a lowpass filter is a significant factor in balancing noise reduction with computational load. Higher-order filters are more effective at noise suppression but also come with a greater processing burden. This trade-off needs to be considered when designing real-time applications, as computational constraints can become a limiting factor.
Nonlinear filtering techniques like median filtering provide unique advantages over their linear counterparts. They excel at preserving edges while simultaneously reducing noise. This makes them particularly suited to high-contrast scenes where maintaining image details is paramount.
As video resolutions continue to increase, the challenges for effective filtering become even more pronounced. Insufficient filtering can lead to more visible artifacts and noise. This emphasizes the importance of designing filters that can handle these increasingly complex scenarios with precision and efficiency.
Looking to the future, photonic-based filters hold a great deal of promise. These filters could significantly reduce latency and accelerate real-time processing speeds, particularly crucial for high-resolution video. While still in their development stages, they might fundamentally change how video is processed and delivered. The potential for improved video quality and performance is quite exciting.
Exploring the Role of Lowpass Filters in Video Noise Reduction A 2024 Perspective - Integration of Lowpass Filters in Modern Computer Vision Systems
The incorporation of lowpass filters within contemporary computer vision systems underscores their crucial role in enhancing image quality and mitigating noise across a range of applications. These filters are essential for initial image processing, facilitating improved feature extraction and analysis, particularly in scenarios where high-frequency noise is prevalent. The development of adaptive algorithms and machine learning has significantly advanced the capabilities of lowpass filters, allowing them to dynamically adjust to diverse video content and noise characteristics, thus optimizing their performance. However, the intricacy of filter design, encompassing interactions with other processing stages and the potential introduction of artifacts, demands a meticulous approach during system integration. As technological advancements continue, the exploration of more sophisticated filtering techniques, such as those leveraging photonic principles, holds promise for further enhancing the efficacy of lowpass filtering in computer vision tasks.
Lowpass filters within modern computer vision systems frequently employ polyphase structures to enhance processing efficiency. By breaking down the filtering process into smaller, more manageable steps, these structures can considerably reduce computational demands, making them particularly valuable in real-time applications where speed is crucial. However, lowpass filters can sometimes lead to "ringing artifacts", especially when dealing with sharp edges in video frames. These artifacts, characterized by oscillations near edges, can degrade image quality, presenting a challenge for filter designers to balance noise reduction with visual integrity.
Some sophisticated lowpass filtering techniques incorporate phase-shift methods to create non-causal filters. These filters, by looking at future and past data, improve the robustness of image processing during rapid changes in the visual scene without compromising temporal accuracy. This ability to anticipate change can be particularly useful for tracking objects that move quickly.
Dedicated lowpass filter integrated circuits (ICs) have emerged as a promising approach for achieving better power efficiency in video systems. By seamlessly integrating analog and digital filtering components, these hybrid ICs can reduce power consumption compared to traditional methods, leading to potential improvements in battery-powered systems and devices.
Moreover, the advancement of programmable lowpass filters allows engineers to adjust filter parameters in real-time. This adaptability is a major advantage, as it enables them to fine-tune noise reduction strategies based on moment-to-moment analysis of the video content. This can be incredibly useful, for example, in automatically adjusting filtering levels for different video scenes.
Machine learning models are increasingly contributing to the design of lowpass filters that can better understand the context of image and video data. Through learning from vast amounts of data, these machine learning filters can more effectively distinguish between real signal and noise, leading to more adaptive and contextually aware filtering. This approach could potentially refine the filtering process based on the type of content.
The integration of lowpass filters with temporal filtering techniques further improves noise reduction throughout video sequences. This is particularly useful in rapidly changing video scenarios like live events or action footage, as noise can be reduced over a sequence of frames. In these dynamic situations, it's vital that the lowpass filter adapts to maintain clarity.
Wavelet-based lowpass filters have become more popular due to their capacity to analyze data across multiple resolutions. In video processing, where details vary in scale and significance, this multi-resolution analysis can be particularly valuable. Wavelet transforms allow the filter to operate at different levels of detail, helping to maintain image quality across the entire range of the video.
However, using lowpass filters in conjunction with high dynamic range (HDR) video systems presents its own set of challenges. Accurate filtering across the wider range of brightness levels present in HDR video demands more intricate filtering techniques that can dynamically adjust to the signal's intensity. It is still an ongoing area of research to achieve the best results.
Recently, researchers have begun exploring the application of holographic lowpass filters. These filters use light diffraction to suppress noise, suggesting that novel filtering approaches may revolutionize how video systems function. It's a fascinating area that, though in its early stages, hints at future improvements in video processing, potentially enhancing high-performance video systems. These explorations are important for ensuring that video processing keeps pace with technological advancements in fields like display technology.
Exploring the Role of Lowpass Filters in Video Noise Reduction A 2024 Perspective - Optimizing 8K Video Coding with Lowpass Filter Preprocessing
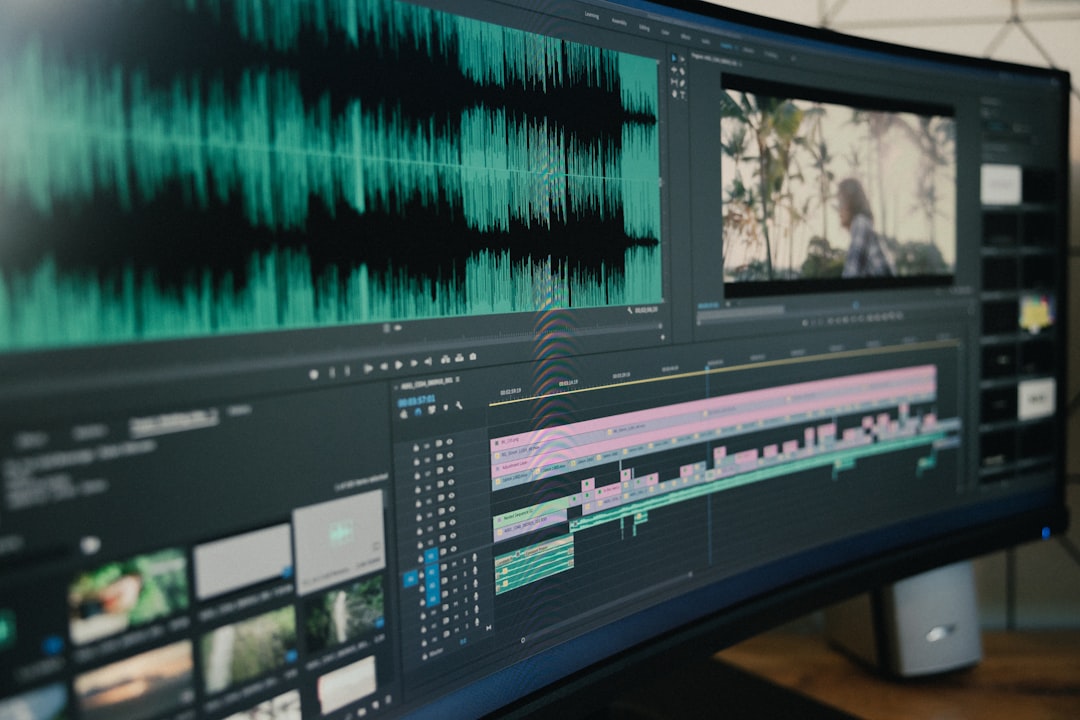
Optimizing 8K video coding has seen significant progress with the use of lowpass filter preprocessing. These techniques, incorporating modern methods like wavelet shrinkage, are designed to significantly reduce noise, improving the overall quality of 8K videos. By minimizing the influence of minor noise and subtle edges that aren't perceptually significant, these filters help video codecs achieve a better balance between data rate and picture quality, which is a challenge with very high-resolution video. Compared to the traditional linear filter approaches, the newer methods show marked improvement. Moreover, the introduction of adaptive Discrete Cosine Transform techniques provides a greater degree of control over the filtering process, adapting to various video content more effectively. The expanding world of 8K content necessitates sophisticated lowpass filter preprocessing to minimize compression artifacts and ensure optimal image quality. While there are still challenges, this is a vital area of development for future video compression technologies.
Recent advancements in 8K video coding have seen the development of preprocessing equipment that leverages lowpass filtering for improved efficiency. This approach hinges on the idea that reducing high-frequency components, often imperceptible to the human eye, can significantly streamline the compression process. This is especially crucial since uncompressed 8K video requires enormous bandwidth, potentially upwards of 48 Gbps.
These preprocessing methods often incorporate novel wavelet shrinkage techniques, utilizing distinct shrinkage functions (S1 and S2) to effectively minimize noise and enhance visual quality. The core idea is to remove weak-edge details and noise components that contribute minimally to the perceived quality of the video, but significantly inflate the data size.
The goal is to achieve rate-distortion optimization. In other words, researchers are trying to find methods that reduce the data rate (bandwidth needed) while keeping the impact on the video quality (distortion) as low as possible. This has led to a specific area of research that previously had not received much attention. Historically, preprocessing in video compression wasn't given as much importance as the compression algorithm itself.
Traditional noise filtering techniques often rely on linear filters. These filters smooth out pixel values in a local neighborhood, reducing noise while simultaneously potentially blurring or attenuating minor details before the encoding process.
Early experimental results suggest that employing lowpass filtering and noise reduction during preprocessing improves the encoded image quality, especially noticeable in reduced compression artifacts like block distortions. This is because perceptually less important elements in the video are removed. The less complex the video, the easier it is to compress efficiently.
One interesting approach is the integration of perceptual preprocessing filters that aim to selectively remove details that are not visually significant. This lessens the burden on subsequent video encoding steps and contributes to overall quality improvements.
Within the scope of rate-perception optimization, new methods employing an adaptive Discrete Cosine Transform (DCT) have been explored. The DCT's ability to represent video data efficiently makes it a useful tool for enhancing compression performance.
The overall message here is that preprocessing is a crucial step for better video compression performance. It allows for better video compression algorithms, suggesting a path towards further advances in video compression technology.
However, it's important to be cautious about over-reliance on any preprocessing step. The goal is to improve compression efficiency, not at the cost of introducing artifacts or unintended visual degradation. Further research and development will likely focus on perfecting these methods, striking a balance between the visual quality we expect from 8K and the need to transfer large amounts of data with lower bandwidth. The challenges of dealing with potential aliasing due to increased resolution will also need continued attention.
The burgeoning field of machine learning offers promise in further improving these processes. AI-driven lowpass filter design could allow for adaptive noise reduction based on content type and complexity, leading to more intelligent and context-aware filtering. Finally, there's considerable interest in the future of photonic-based filters. This relatively nascent field could potentially revolutionize the speed and efficiency of filtering, leading to significant improvements in how we handle and deliver 8K content.
Exploring the Role of Lowpass Filters in Video Noise Reduction A 2024 Perspective - Comparing Linear and Nonlinear Lowpass Filters for Noise Discrimination
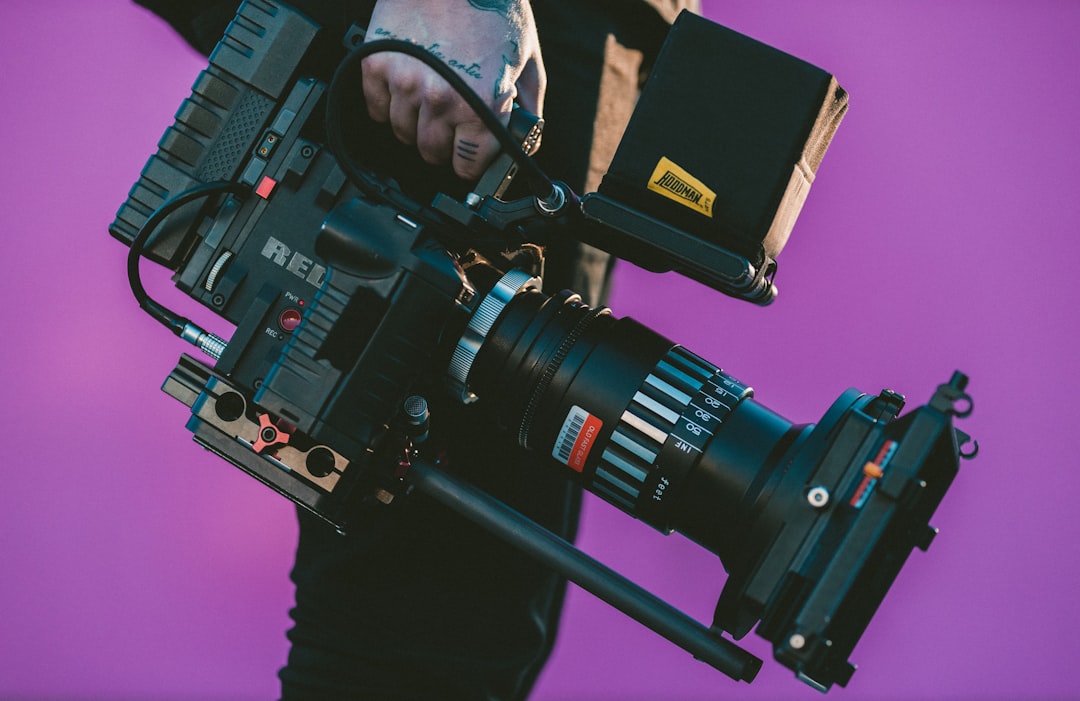
When exploring lowpass filters for noise reduction, a key consideration is the choice between linear and nonlinear filter designs, particularly for their impact on noise discrimination. Nonlinear filters, known for their ability to preserve sharp signal peaks while suppressing noise, are valuable in situations where preserving those peaks is important. This makes them a good choice for many video applications. On the other hand, linear-phase filters are often used in the initial processing stages, efficiently filtering out high-frequency noise that isn't part of the desired signal. Understanding the advantages and limitations of each approach is vital in choosing the best filter for specific noise reduction tasks, as there's no one-size-fits-all solution. As video processing continues to advance, recognizing these differences and the trade-offs they involve will be essential for achieving optimal noise reduction and high-quality video.
1. **Noise Discrimination and Edge Preservation:** Nonlinear lowpass filters, such as median filters, often demonstrate a greater ability to maintain fine details, especially edges, while simultaneously reducing noise. This can be particularly beneficial in video sequences with sharp transitions or high-frequency artifacts that linear filters might struggle with.
2. **Dynamic Frequency Response:** Linear lowpass filters typically have a predictable and consistent frequency response, while nonlinear filters can adapt their behavior based on the local characteristics of the signal or image. This adaptive nature can be advantageous when dealing with a diverse range of noise profiles or signal variations.
3. **Computational Requirements:** Linear filters, like Butterworth filters, usually involve simpler calculations compared to their nonlinear counterparts. This computational simplicity can make linear filters more suitable for real-time video processing, especially when dealing with high-resolution formats that place high demands on processing power.
4. **Adaptability to Noise Characteristics:** Nonlinear filters can be designed to respond dynamically to specific noise patterns, offering a more tailored approach to noise reduction. This contrasts with linear filters, which often rely on fixed parameters, potentially limiting their efficacy in conditions where the noise characteristics are constantly evolving.
5. **Balancing Noise Reduction with Detail Preservation:** While nonlinear filters can be effective at noise reduction, they can sometimes lead to unintended consequences, such as oversmoothing, which can diminish subtle details in the image or video. It's a constant trade-off between efficiently removing noise and preserving important visual information.
6. **Filter Parameter Impact:** In linear filters, increasing the order usually has a predictable effect on the transition between the passband and stopband, allowing for finer control of frequency attenuation. In nonlinear filters, adjustments to parameters may not have the same clear and predictable outcome.
7. **Performance in Challenging Scenarios:** Nonlinear filters tend to be more robust when facing conditions like low lighting or heavy noise. They can maintain clarity and visual fidelity in situations where linear filters might struggle to efficiently eliminate noise without introducing significant blurring or distortion.
8. **Filter Application Areas:** Linear lowpass filters remain fundamental for noise reduction in many video processing applications. However, nonlinear filters, including techniques like bilateral filtering, are seeing increased adoption when tasks demand simultaneous noise reduction and detail preservation. This is particularly true in specialized applications such as artistic image rendering.
9. **Practical Applications:** Nonlinear filtering approaches are increasingly prevalent in real-world contexts like preprocessing for medical imaging or satellite imagery, where careful preservation of crucial structural details while suppressing unwanted noise is of paramount importance.
10. **Understanding Limitations:** While nonlinear filters provide distinct advantages, it's essential to be aware of their potential limitations. They can sometimes introduce specific artifacts or distort certain features in the video or image, emphasizing the importance of careful filter design and parameter tuning to minimize such effects and match the desired outcomes for a given application.
More Posts from whatsinmy.video: