Analyze any video with AI. Uncover insights, transcripts, and more in seconds. (Get started for free)
How Video Speed Reduction Affects Motion Analysis A Technical Deep Dive
How Video Speed Reduction Affects Motion Analysis A Technical Deep Dive - Frame Rate Reduction Impacts Accuracy In Golf Swing Analysis At 60fps vs 240fps
The precision of analyzing a golf swing is significantly impacted by the frame rate used in capturing the video. Utilizing a higher frame rate, such as 240fps, provides a more accurate and detailed representation of the swing, especially during fast-paced movements. The increased number of frames minimizes motion blur, offering a clearer image of the golfer's body in motion. While a 60fps capture rate is often considered a baseline for analysis, it lacks the finesse necessary to thoroughly dissect the complexities of the golf swing, potentially overlooking subtle but crucial details in the movement. Achieving an optimal video recording that facilitates clear analysis also relies heavily on elements like sufficient lighting, which ensures the captured image quality is conducive to the intricate analysis required. Therefore, the transition to higher frame rates directly enhances the ability to delve into the intricate biomechanics of a golf swing, laying a foundation for more targeted improvements in the golfer's technique.
Our visual perception of motion is comfortably within the 24 to 60 frames per second (fps) range, but scrutinizing athletic movements, especially the rapid dynamics of a golf swing, necessitates frame rates well beyond that threshold to truly capture the nuances of movement.
Utilizing a frame rate of 60 fps, we see noticeable motion blur in fast-paced actions. This can mask pivotal details related to body mechanics and the precise timing of a swing, potentially leading to imprecise assessments of swing quality.
Elevating the frame rate to 240 fps provides four times the visual information per second. This dramatically enhances our ability to dissect muscle activation and clubhead path with much greater detail, paramount for conducting a precise golf swing analysis.
Research has suggested a strong correlation between higher frame rates and the accuracy of biomechanical assessments. Some practitioners have noted a substantial decline in analytical error rates – from exceeding 20% at 60 fps to less than 5% at 240 fps.
The improved temporal resolution afforded by higher frame rates enables us to better track kinematic variables, such as angular velocity and acceleration. These factors are instrumental in understanding the intricacies of a proficient golf swing.
Analyzing the consistency of a golfer's swing across multiple attempts can reveal significant insights into their overall technique. Higher frame rates provide the granularity needed to confidently execute this type of analysis, a feat that can be problematic with lower frame rates.
A phenomenon known as "aliasing" can occur with lower frame rates. It causes similar motion patterns to blend together, making it challenging to differentiate subtle differences in swing techniques. These subtle differences can hold the key to performance improvements.
Examining the intricacies of swing variations or specific swing flaws, frame rates of 240 fps offer a unique lens. We can detect very minute changes in grip pressure or subtle shifts in posture midway through a swing, which are difficult, if not impossible, to observe at lower frame rates.
Professional golf analysts commonly use frame rates far exceeding typical video standards. Some systems even reach 1000 fps. This illustrates a growing industry consensus on the importance of higher resolution capabilities for producing accurate performance analyses.
While the technology has advanced to support capturing and processing 240 fps video, the computational demands are higher. This means, although 240 fps capability exists, the resources are not universally available. This technology gap may limit the analytical tools available to some golf coaches and instructors, which can affect their ability to offer insightful feedback to golfers.
How Video Speed Reduction Affects Motion Analysis A Technical Deep Dive - Motion Artifacts During Jump Height Measurements At Different Playback Speeds
When analyzing jump height using video, altering the playback speed can introduce noticeable motion artifacts that impact the accuracy of measurements. For example, high-speed recordings at 240 frames per second (fps) can create a considerable amount of motion blur, potentially leading to an 11 centimeter error when measuring a 40 centimeter jump. This blur can easily extend across 28 pixels vertically on a typical 720p camera, obscuring the precise details needed for accurate analysis.
Studies have found a weaker correlation between jump height measurements made from videos and those from more traditional methods, suggesting that relying solely on video analysis for jump height assessment may not be as reliable as previously thought. This inconsistency highlights the challenges involved in obtaining consistent and trustworthy data from video recordings, particularly at higher speeds.
While advanced technologies like markerless motion analysis are attempting to improve jump height calculations, different measurement techniques still yield a degree of variability in results. This variability emphasizes the need for researchers and practitioners to be mindful of the potential limitations inherent in using video analysis for accurate jump height measurements, especially when manipulating playback speed.
Motion artifacts, particularly motion blur stemming from high takeoff velocities, can significantly interfere with the accurate measurement of jump height. For instance, a 40 cm jump might produce around 11 cm of blur when captured at 240 frames per second (fps). This blurring can extend across a considerable number of pixels in a standard 720p video, making it challenging to pinpoint the exact peak of the jump during analysis.
Interestingly, studies comparing video analysis to more traditional jump height measurement tools like force platforms have revealed lower correlation coefficients than expected. This suggests a potential gap in the accuracy of video-based analysis when motion artifacts are present. Various methods exist for quantifying jump height, ranging from direct measurements using markers or specialized equipment to more indirect methods derived from position-time data obtained through motion capture systems.
Researchers have begun using readily available high-speed cameras, like those found on iPhones, along with more established professional systems, such as Prime 13 cameras. This trend underscores the increasing accessibility of tools for motion analysis, potentially democratizing access to sophisticated performance insights.
However, despite the growing availability of tools, we find inconsistencies in the accuracy and precision of different jump height measurement methods. Whether using force platforms or motion capture, results can vary, which highlights the importance of understanding the inherent limitations of each technique.
While smartphone applications and programs like Kinovea have emerged as potentially valuable tools for measuring jump height, their accuracy can fall short compared to more specialized equipment. This suggests a trade-off between affordability and precision.
Direct methods of jump height measurement, like those that utilize markerless motion analysis systems to track the center of gravity during the jump, provide a way to mitigate the influence of limb movements on the final height calculation.
It's important to acknowledge that how feedback is provided during jump testing can influence performance metrics. This emphasizes the need for careful consideration of the context and method when evaluating jumps for the purpose of assessing athletic capacity.
The field of markerless motion analysis is continuously evolving, leveraging advances in computer vision to refine jump height calculations in sports biomechanics. The accuracy of these automated methods promises to provide a more standardized and efficient means of jump height evaluation.
There’s a clear discrepancy between the tools available to professional researchers and athletes compared to what’s readily available to amateur and recreational individuals. This gap in access to high-frame-rate video analysis and associated software creates a disparity in the quality of jump height assessment, potentially hindering effective training for a portion of the athletic community.
How Video Speed Reduction Affects Motion Analysis A Technical Deep Dive - Data Loss Patterns In Running Gait Analysis With Progressive Speed Reduction
When analyzing running gait using video, reducing the playback speed can lead to a notable loss of data, specifically in the details of running mechanics. This data loss becomes more significant as the speed is progressively decreased, ultimately impacting the accuracy and reliability of gait assessments. Traditional methods of analyzing running often struggle to mimic real-world scenarios, which is why innovations in video technology and analysis play a crucial role in achieving accurate and reliable gait measurements.
The relationships between frame rates and different measurement techniques, whether they involve wearable sensors or advanced video analysis systems, add a layer of complexity that needs careful evaluation to ensure data consistency and quality. These intricacies, particularly those related to progressively reducing playback speed, illuminate the complexity inherent in motion analysis and the importance of striving for high data integrity. Understanding these challenges is important for practitioners and researchers to ensure they are making sound conclusions based on quality data.
Running gait analysis, especially when exploring the effects of speed, reveals intriguing data loss patterns that can significantly impact our understanding of running biomechanics. As running speed increases, particularly beyond 5 meters per second, even high-frame-rate video systems can struggle to capture all the crucial details needed for a complete analysis, leading to potential inaccuracies in how we interpret a runner's mechanics.
It's been noted that the starting speed of a run has a noticeable effect on data integrity. Sometimes, subtle variations in a runner's gait at slower speeds can mask key insights that only become evident at higher speeds, complicating comparisons and overall analysis. This highlights how speed influences what we see and how we might misinterpret the information.
When we manipulate video playback speed, the patterns of data loss become more apparent, introducing timing discrepancies in the measurements of various kinematic factors. This can lead to significant errors, especially when analyzing rapid changes during a runner's stride. It's as if the clock gets slightly off, causing us to misinterpret the sequencing of movements.
One of the challenges of analyzing high-speed running is that video systems might misinterpret joint angles due to incomplete frame capture. This can create problems with key measurements like knee flexion or hip extension, crucial elements in understanding how an athlete moves. It's like a blurry photograph of a complex joint, making it difficult to get a precise angle.
While increasing frame rates helps reduce data loss, it also creates more data to process. This can strain computing resources, and it makes it challenging for researchers to effectively extract useful information without introducing errors, particularly with systems that don't have enough processing power. It's like having a flood of information, but not having the tools to filter and analyze it effectively.
High-speed running also has a tendency to disrupt marker-based tracking systems, causing them to misalign or drift during analysis. This can distort coordination data and make it difficult to fully understand the interplay between different body parts during the running cycle. It's as if the markers are starting to move independently from the body they're supposed to be tracking.
The delays in processing high-speed data can cause a mismatch between what is actually happening and what the system is recording. This can lead to complications when trying to provide feedback to runners and coaches during performance evaluations, potentially hindering their ability to make adjustments in real-time. It's as if the system is a little behind in providing a helpful response.
Changing terrain during high-speed running adds further complexity to data loss. The changes in biomechanics that happen when someone runs on uneven ground might not be as easy to see at slower speeds. This can lead to skewed analysis and a potentially misleading understanding of how running mechanics adapt to different surfaces. The ground itself starts to factor into how easy or difficult it is to observe the movement and could be easily misconstrued if not considered.
The algorithms that power advanced motion analysis are often sensitive to changes in frame rate. This variability can introduce inconsistencies in data integrity, which makes us question whether these automated analyses are as reliable as we'd hope across different playback speeds. It's like the automated systems themselves might be changing their behavior slightly, making it harder to interpret their outputs.
Lastly, if athletes train at slower speeds to refine their movements, that training might not perfectly translate to how they move at higher speeds. This difference can be because of variations in muscle activation or coordination patterns. It emphasizes the importance of matching the video speed used in the analysis to the speed at which the athletes are training. It is as though the body adapts in unique ways to different speed intensities, leading to behaviors in testing environments not indicative of real-world performance.
How Video Speed Reduction Affects Motion Analysis A Technical Deep Dive - Visual Tracking Precision In Ball Sports With 50 Percent Speed Decrease
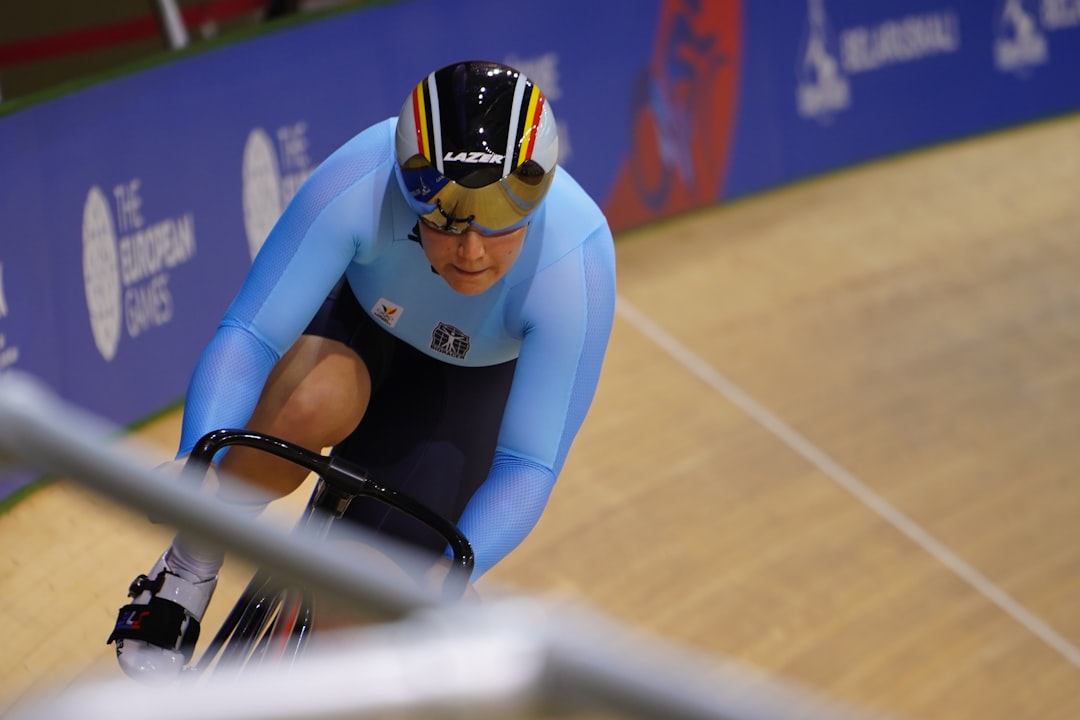
When analyzing ball sports with a 50% reduction in video playback speed, the precision of visual tracking is significantly impacted. While slower speeds can enhance the clarity of motion, making it easier to track fast-moving objects like balls, they can also introduce challenges. The smaller size of balls compared to the frame often makes them difficult to track accurately, and reduced speed may exacerbate these difficulties. Although slower video can improve the visibility of subtle movements, it can also lead to increased motion blur and potential discrepancies in timing, making precise analysis of ball trajectories, player performance, and strategic decision-making more complex. This highlights the ongoing need to find a balance between leveraging speed reduction for better visualization and maintaining the integrity of data collection to enable effective performance analysis and training optimization in sports. The field of sports analysis is constantly evolving, pushing the need for innovation in tracking technologies to address the nuanced challenges posed by speed manipulations.
Reducing the speed of sports videos by 50% can offer advantages for analysis, but it also introduces a number of potential issues for accurate assessment. While slowing down the footage allows for a more detailed examination of player movements and ball trajectories, it can also distort our understanding of the dynamics of the sport.
For instance, reaction times may appear longer than they actually are, potentially leading to incorrect interpretations about decision-making during play. The slowed-down perception might also make it more challenging to accurately assess visual-motor coordination, as the split-second timing needed in many ball sports is not fully represented. Additionally, analysts might miss rapid changes in body position or ball trajectory that are crucial for evaluating performance, potentially resulting in inaccurate assessments of technique.
Fatigue analysis can also be affected, as players' movements may appear less dramatically altered at half-speed, masking the true impact of fatigue. However, slowing down videos does allow for the observation of subtle movements, like wrist flicks or stance adjustments, which might be missed at normal speed. These micro-movements are critical in sports like tennis or badminton, where precision is paramount.
The subjective perception of an athlete's performance can also change with slower playback. Athletes reviewing their own footage might see their skills in a different light than when they are played at full speed. This disparity could lead to a disconnect between perceived performance and actual effectiveness.
Furthermore, the way players recognize cues in ball sports could be altered by changes in video speed. Auditory or visual cues might be interpreted differently at a slower pace, possibly leading to an inaccurate understanding of the context of the game. The reduced speed can also affect biomechanical analysis, potentially masking the true forces and angular velocities involved in player movements, which could be critical for injury prevention efforts.
Maintaining accurate equipment calibration for slower playback is another challenge, as any latency introduced can distort results. This is especially problematic in fast-paced environments where precise tracking is critical.
Finally, athletes viewing their performances in slow motion might focus on subtle details that don't translate into real-game situations. This shift in attention can lead to an incomplete picture of effective tactics when playing at normal speed.
In conclusion, while decreasing video speed can offer unique insights into certain aspects of ball sports, it's crucial to acknowledge that it can also introduce distortions in our understanding of the sport's nuances. As researchers, we must be mindful of these potential issues to avoid misinterpreting the information and to maintain the integrity of our analysis. This knowledge enables us to develop more robust analytical approaches that consider the limitations and benefits of altered video playback speeds.
How Video Speed Reduction Affects Motion Analysis A Technical Deep Dive - Hardware Processing Requirements For Real Time Motion Analysis At Various Speeds
The demand for real-time motion analysis across a range of speeds is driving a shift from conventional PC-based processing towards embedded systems. This change is particularly relevant in fields like home automation and autonomous systems, where swift and precise motion analysis is critical. The need for efficient processing of complex algorithms in these situations has led to a reliance on specialized hardware platforms.
Systems utilizing hardware acceleration, particularly GPUs, FPGAs, and multicore architectures, have demonstrably pushed processing speeds beyond 120 frames per second. This increased computational power provides a significant advantage for tackling the challenging task of motion estimation in real-time. However, this increase in processing speed is not without its limitations. As frame rates rise, so do the demands on CPU resources. This relationship underscores the need for ongoing improvements in hardware technology to keep pace with the increasing computational demands of modern motion analysis.
Ultimately, the ability to perform accurate and reliable motion analysis in high-speed environments relies on the development of effective hardware solutions. These solutions must be capable of handling the increased computational load imposed by faster video speeds without introducing error or compromising data integrity. This remains a crucial area of focus for researchers and developers working in this field.
The demands on hardware for real-time motion analysis are closely tied to the video's frame rate. For example, processing 240 frames per second (fps) requires significantly more powerful processing than 60 fps due to the four-fold increase in data. This highlights the growing importance of graphics processing units (GPUs) for real-time video processing, especially with high-speed footage. GPUs excel at parallel processing, which is crucial for simultaneously handling multiple frames and accurately extracting motion data.
However, hardware speed can be a critical factor in real-time analysis. If the processing of frames lags behind the actual motion, the resulting analysis might be out of sync with the athlete's current actions, decreasing the effectiveness of coaching interventions. This highlights a key design constraint for these systems. Similarly, data compression techniques can introduce unwanted artifacts that might distort the portrayal of motion during fast-paced action. Carefully selecting compression methods that minimize impact on motion tracking is essential.
There's a trade-off between processing power and battery life, particularly in portable motion analysis systems. High-speed cameras often require powerful processors, but these processors tend to consume a significant amount of power and generate heat. Effectively managing the heat generated during the high-resolution video processing is critical to avoid hardware limitations due to overheating and slowdowns.
When using remote processing or cloud-based systems, network latency can introduce a significant obstacle. Any delays in data transmission can blur or distort the video feed, potentially rendering real-time analysis ineffective. Also, balancing frame rate and resolution is often a challenge for capturing hardware. Higher frame rates sometimes reduce resolution, which can negatively impact the visual clarity needed for analysis.
Complex motion analysis systems often employ multiple sensors. The incorporation of additional sensors necessitates increased computational power, posing challenges for hardware designers to prevent bottlenecks and optimize analysis. This is tightly coupled to software compatibility. The usefulness of any motion analysis hardware depends on efficient, compatible software that can properly utilize available processing capabilities. Balancing the requirements for software efficiency and hardware capabilities across multiple motion analysis environments is a continuing engineering challenge.
These aspects are essential to consider when developing hardware designed for real-time motion analysis. As the demand for more accurate motion data in a variety of applications grows, refining the capabilities of motion analysis systems requires continuous exploration of the interplay between hardware capabilities, software algorithms, and frame rate performance.
How Video Speed Reduction Affects Motion Analysis A Technical Deep Dive - Motion Blur Effects On Joint Angle Calculations During Speed Adjustments
When we analyze motion using video, especially when altering playback speed, motion blur can significantly impact the accuracy of joint angle calculations. This is particularly problematic when analyzing rapid movements, like those seen in sports, where blurred images can obscure the precise details needed for accurate biomechanical analysis. The issue is especially relevant in markerless motion capture systems where the absence of physical markers makes relying on image analysis for joint angle calculations even more susceptible to errors. While higher frame rates and improved lighting can help minimize the effects of blur, analysts often encounter challenges when working with conventional video analysis methods. This means that extracting reliable and accurate joint angles from video, especially when speeds are modified, remains a key challenge. The pursuit of more precise motion analysis, particularly in dynamic scenarios, necessitates ongoing development of tools and techniques that can mitigate the impact of motion blur across various playback speeds.
High-speed cameras are a valuable tool for accurately measuring joint angles in sports science, often utilizing either 2D systems with a single camera or more comprehensive 3D systems with multiple cameras. While 2D methods have been shown to provide reliable joint angle data when compared to 3D systems, the presence of motion blur can introduce significant challenges, particularly when analyzing rapid movements. This is especially true for markerless motion capture systems where motion blur can severely impact the accuracy of lower extremity kinematics calculations.
Optimizing video clarity is crucial for reducing the impact of motion blur. This can be achieved by adjusting camera settings such as resolution, lighting, shutter speed, and frame rate. These adjustments, however, can sometimes be constrained by hardware limitations. Developing a robust system that accurately captures kinematic data remains a difficult problem in areas like sports biomechanics and rehabilitation.
The choice of video recording parameters, such as frame rate and shutter speed, can significantly affect the accuracy of joint angle detection, especially when analyzing high-speed movements. Issues like marker occlusion, scatter, or reflection during capture can add to the difficulty of obtaining accurate measurements, complicating joint angle calculations.
Analyzing video for joint angle calculations can be a time-consuming task. Traditional methods either require time-consuming marker placement or less efficient manual calculations, suggesting a need for more streamlined and automated methods. There is a growing demand for more efficient ways to extract accurate kinematic information from video data.
Researchers have explored using video analysis to optimize athletic performance, for example, determining the optimal take-off angles for maximizing jump height or throwing distance. Video analysis provides valuable information without the need for more intrusive measurement techniques, which is a significant benefit. However, there are still drawbacks associated with this methodology.
Specifically, the presence of motion blur during speed adjustments can significantly impact joint angle calculations. Motion blur can skew the perceived angles of joints, particularly during rapid movements like knee flexion and hip extension, potentially leading to inaccuracies in assessing biomechanics. This misinterpretation of the athletes’ true movements can negatively affect the outcome of training.
As the speed of video playback decreases, the asymmetry in data capture related to joint angles becomes more evident, leading to a discrepancy between actual joint angles and those calculated from blurred frames. Consequently, interpreting an athlete's movement strategy and efficiency becomes more challenging. It is as though the information collected does not accurately represent the athlete’s actions.
Higher frame rates are critical for accurately capturing fast movements and resulting changes in joint angles. When frame rates are reduced, the temporal resolution suffers, making it more difficult to link specific joint movements with the outcomes of an athletic performance. This makes it hard to accurately identify the cause and effect of the athlete’s actions.
Moreover, motion blur can amplify errors in joint angle calculations by increasing the visual difference between successive frames. Reduced frame rates worsen this problem and can lead to misinterpretations of movement dynamics, possibly contributing to ineffective training advice. It is easy to misconstrue how the athlete is moving because of motion blur, leading to unhelpful conclusions.
The link between joint angles and kinetic forces can also be masked by motion blur, potentially leading to misrepresentations of the force distribution during speed changes. Understanding these forces is very important in sports biomechanics, as it helps in implementing strategies for injury prevention. However, inaccurate observations of motion can limit this aspect of sports science.
Coaches using blurred video may end up offering feedback that is not aligned with an athlete's true performance. This can lead to a vicious cycle where corrections are based on an inaccurate view of the action and can impede improvement. This underscores the significance of clarity in motion to promote accurate training practices.
Assessing how athletes adjust speed, as in sprinting or deceleration, is prone to inaccuracies because of motion blur. This loss of clarity can obscure critical timing details that are essential for understanding the nuances of an athlete’s athletic ability. The lack of sharpness makes it harder to separate and dissect actions, creating a problem with the ability to correctly understand actions.
When automated tracking systems analyze videos with lower quality because of lower frame rates and motion blur, they struggle to estimate joint angles accurately. This can lead to erroneous conclusions regarding an athlete's technique and movement effectiveness, further emphasizing the importance of data quality in athletic analysis.
Post-processing efforts to correct motion blur can also introduce new sources of errors, making joint angle calculations even more complex. The importance of acquiring high-frame-rate videos from the start, thereby minimizing the need for potentially faulty corrections, cannot be overstated. The most ideal approach is to optimize the video capture from the start and thereby reduce any needed post processing.
Repeated frame reductions, particularly when they fall below the standard 60 frames per second, lead to data loss. This creates major knowledge gaps in understanding the intricate joint dynamics involved in fast-paced movements. It underlines the criticality of adhering to appropriate frame rates for dependable motion analysis. It is important to acknowledge the influence of frame rates on the information extracted from video analysis.
Analyze any video with AI. Uncover insights, transcripts, and more in seconds. (Get started for free)
More Posts from whatsinmy.video: