Implementing a Python High Pass Filter for Video Audio Analysis A Practical Guide
Implementing a Python High Pass Filter for Video Audio Analysis A Practical Guide - Understanding High Pass Filters in Audio Processing
High pass filters (HPFs) are instrumental in audio processing, selectively allowing higher frequencies to pass through while suppressing lower frequencies. Their main purpose is to improve audio quality by mitigating low-frequency noise, which can muddle the sound and make it less clear. This ability to isolate specific frequency ranges is highly beneficial in various audio contexts, especially music production and live sound mixing where controlling the sonic landscape is crucial. Python, with libraries like SciPy, offers a convenient way to implement these filters, processing audio data from either live streams or pre-recorded files. However, a critical aspect to consider is the filter's cutoff frequency. It dictates the boundary between frequencies that are passed and those that are attenuated, directly affecting the overall filtering effect. Getting this parameter right is crucial to effectively remove unwanted noise and preserve the desired sound characteristics.
High pass filters (HPFs) essentially let higher frequencies pass through while diminishing those below a specific cutoff frequency. This characteristic is quite useful in audio work, especially for eliminating unwanted background noise and improving audio clarity.
It's important to understand that a HPF doesn't entirely remove frequencies below the cutoff point. Instead, it reduces their strength, resulting in a cleaner sound without necessarily introducing unwanted distortions.
The rate at which a HPF reduces frequencies below the cutoff, often described as the filter's order, impacts how it shapes the sound. A higher-order filter produces a quicker reduction in these frequencies, leading to a sharper cutoff that can subtly change how we hear the sound.
One thing to be mindful of with HPFs is the possibility of "ringing", a phenomenon where a peak in the frequency response occurs near the cutoff. This can color the audio in an unexpected way, potentially highlighting frequencies you intended to attenuate.
Beyond noise reduction, HPFs find creative applications in audio engineering. For instance, in sound design and synthesis, applying HPFs can facilitate layering sounds more effectively by reducing low-frequency overlap.
Our ears respond differently to frequencies at different levels. Lower frequencies require a greater amplitude to be perceived as the same loudness as higher frequencies. Considering this, HPFs can be tweaked to adjust audio for the human ear, leading to a more balanced listening experience.
These filters don't just exist in isolation. Combining them with low pass filters (LPFs) can result in a bandpass filter, which allows only a selected range of frequencies to pass, allowing for more fine-tuned sound manipulation.
Keep in mind that applying HPFs can affect the phase response of the audio signal. This could lead to timing differences between the frequencies, especially if the filter doesn't preserve phase linearity.
Many audio production tools, often called Digital Audio Workstations (DAWs), have integrated HPFs. These often allow real-time manipulation of audio during playback, letting engineers assess changes and make adjustments immediately.
While HPFs are widely used to tackle low-frequency noise, it's beneficial to closely study the audio being processed. Sometimes, maintaining low frequencies, even at a lower amplitude, can contribute to the overall richness and fullness of the sound, especially in music styles that rely heavily on bass.
Implementing a Python High Pass Filter for Video Audio Analysis A Practical Guide - Setting Up Python Environment for Audio Analysis
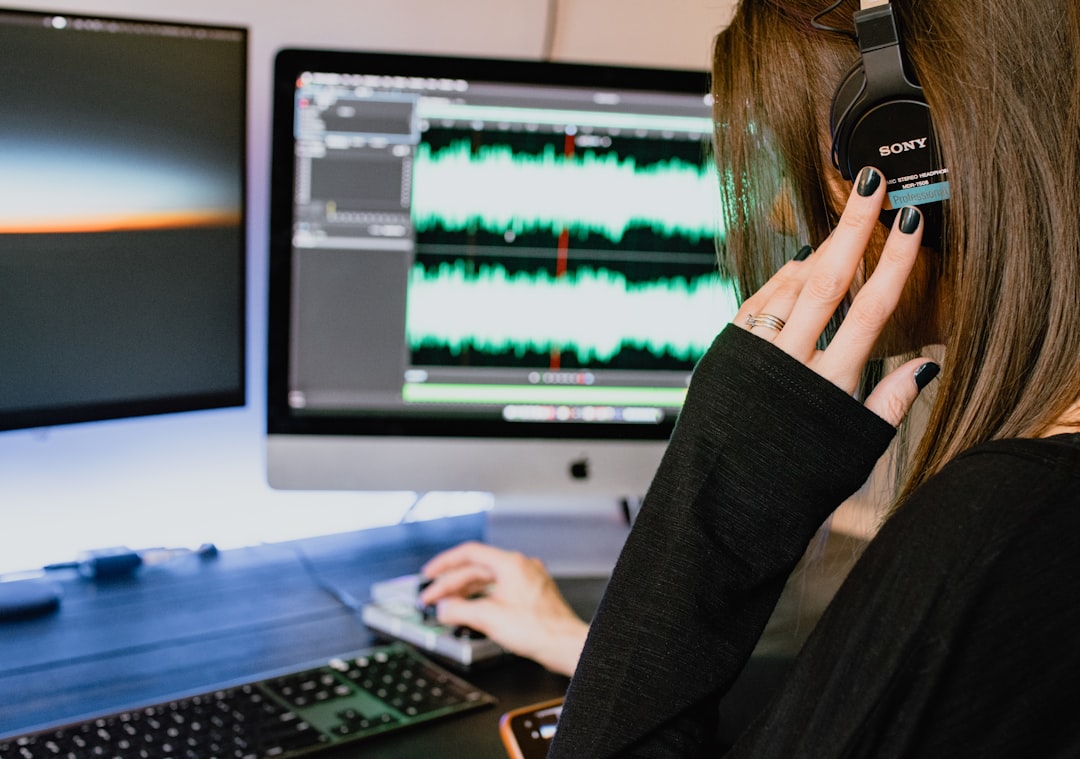
Before you can delve into implementing audio processing techniques like high-pass filtering within Python, you need to establish a suitable environment. This involves setting up your Python installation and installing the necessary libraries for audio manipulation and analysis. Libraries like SciPy, a foundational library for scientific computing, play a vital role in implementing filters and performing mathematical operations on audio data. Libraries like PyDub are particularly useful for handling audio files, allowing for easy loading and manipulation of audio data in different formats. Further, LibROSA, specialized for audio analysis, expands your capabilities with tools for feature extraction and classification.
It's also important to consider the specific needs of your audio analysis project. Audio files can come in various formats and bit depths, and your chosen environment needs to be able to handle those differences smoothly. The ability to work with raw audio data, including extracting and manipulating it in its native format, can be a crucial aspect of some audio processing projects. As Python's audio analysis capabilities are consistently expanding, taking the time to learn and understand the different functionalities offered by these specialized libraries will provide you with a solid foundation for implementing more complex audio processing solutions.
Python's flexibility extends beyond audio filtering, encompassing libraries for waveform visualization, spectral analysis, and even real-time audio processing. This makes it a potent tool for in-depth audio investigations.
Audio data can be treated as multidimensional arrays using NumPy. This allows engineers to apply mathematical operations directly to the audio samples, enabling advanced manipulations like frequency transformations and amplitude adjustments with speed and efficiency.
The choice of sampling rate directly affects the accuracy of audio analysis. A higher sampling rate captures more details, but this comes at the expense of increased storage needs and computational demand. This can lead to bottlenecks in real-time situations.
Digital signal processing (DSP) concepts like convolution and Fourier transforms are core to understanding how filters, including HPFs, work. Python's SciPy library offers functions for implementing these DSP techniques, allowing for streamlined audio processing without requiring a deep dive into DSP theory.
When dealing with real-time audio, latency can become a challenge. Processing delays introduced by filters can affect timing-sensitive applications like live sound mixing, emphasizing the need for optimized Python scripts.
Applying windowing techniques when analyzing audio signals helps to reduce spectral leakage during Fourier transforms. Common windows like Hanning and Hamming are easily implemented in Python, highlighting its versatility in various analysis scenarios.
Designing stable filters is crucial, especially in systems with feedback. Python allows engineers to explore various filter designs using libraries like SciPy, but understanding the stability implications is important to avoid issues.
Python adeptly handles multi-channel audio files, including stereo or surround sound formats. This is particularly beneficial in applications like video audio analysis where maintaining spatial sound properties is paramount.
For computationally intensive audio tasks, Python can interface with C/C++. This allows engineers to write performance-critical parts in a lower-level language, leveraging Python's ease of use while mitigating speed limitations of pure Python implementations.
Python's active audio analysis community has created a wealth of tutorials and open-source projects. Exploring these resources can make setting up an audio analysis environment much easier, providing reliable implementations to build upon.
Implementing a Python High Pass Filter for Video Audio Analysis A Practical Guide - Implementing a Basic High Pass Filter Using NumPy and SciPy
Implementing a basic high-pass filter using Python's NumPy and SciPy libraries is a straightforward process, ideal for introductory audio signal processing. The core of building a high-pass filter involves defining key parameters, primarily the cutoff frequency, using the `butter` function within the SciPy library. This function generates the necessary filter coefficients. A key function in SciPy, `filtfilt`, proves particularly useful because it minimizes phase shifts by filtering the audio signal in both forward and reverse directions. This preserves the length of the signal and avoids certain artifacts related to phase changes. It's often beneficial to visualize the filter's impact on frequencies using libraries like Matplotlib, aiding in understanding how it shapes the audio. While using these libraries offers a fairly simple route to implementing high-pass filters for different audio analysis tasks, careful attention to the choice of cutoff frequency is essential. Also, it is worth being aware of potential artifacts such as ringing effects near the cutoff frequency, which can alter the sound in unexpected ways. Overall, NumPy and SciPy give Python users a strong foundation for exploring high pass filtering.
1. **The Mathematical Underpinnings of HPFs**: High pass filters are rooted in intricate mathematical concepts, specifically relying on the Fast Fourier Transform (FFT) to dissect audio signals into their frequency components. A solid grasp of Fourier analysis is fundamental to effectively implement and utilize these filters within audio-related tasks.
2. **The Delicate Balance of Cutoff Frequency**: The cutoff frequency, while pivotal to HPF functionality, can drastically affect how we perceive sound. Even slight alterations to the cutoff can significantly reshape the tonal characteristics of audio, underscoring the importance of meticulous tuning in practical audio applications.
3. **Real-Time Processing: A Challenge for Python?**: Python can capably manage various audio operations, but it may encounter hurdles in real-time audio processing due to its interpreter-based nature. This constraint emphasizes the need for highly efficient code and potentially incorporating lower-level languages for sections requiring maximal performance, particularly in scenarios demanding immediate audio processing, such as live audio mixing.
4. **Phase Distortion: An Unwanted Side Effect**: Employing HPFs can introduce phase shifts in the frequency response, potentially leading to noticeable phase distortion. This implies that distinct frequencies might reach our ears at different moments, which can affect our perception of audio clarity, particularly within complex audio environments.
5. **Ringing Artifacts: Unexpected Frequency Boosts**: The phenomenon of ringing can manifest as an unintended surge in amplitude around the cutoff frequency, which could be counterproductive if not thoughtfully addressed. This highlights the need for a careful approach to filter design and fine-tuning, focusing on the role of the filter's order and how it shapes ringing behavior.
6. **Beyond Preset Filters: Tailoring Filters to Needs**: Python provides the flexibility to not only apply predefined filter designs but also to build custom ones. Engineers can craft bespoke filter coefficients using techniques like the Bilinear Transform, paving the way for specialized high pass filters that are tailored to the specific demands of individual audio analyses.
7. **Visualization as a Diagnostic Tool**: Leveraging libraries such as Matplotlib in conjunction with NumPy, engineers gain the ability to visually scrutinize the impacts of HPFs on audio signals. This capability proves valuable in gaining a deeper understanding of the filtering process and in troubleshooting issues with the output that might not be immediately apparent from simply listening.
8. **The Sampling Rate Conundrum**: The decision about the sampling rate poses a trade-off. A higher sampling rate enhances the accuracy of frequency response but can also strain processing capabilities and complicate data management, factors to consider when developing real-time audio frameworks.
9. **Multichannel Audio: Complexity Increases**: Implementing HPFs in multichannel audio scenarios, like surround sound, adds another layer of intricacy to managing phase relationships and frequency interactions. Successfully navigating the nuances of spatial audio processing requires a robust understanding of both the technical components and the creative implications of applying filters in these situations.
10. **Expanding Filter Capabilities through Combinations**: HPFs can be seamlessly coupled with low pass filters to create bandpass filters, demonstrating the versatile role of Python in the broader landscape of audio filtering. This capability empowers engineers to pinpoint and selectively modify specific frequency ranges, allowing for finely calibrated control over the sonic landscape.
Implementing a Python High Pass Filter for Video Audio Analysis A Practical Guide - Integrating PyAudioAnalysis for Advanced Audio Feature Extraction
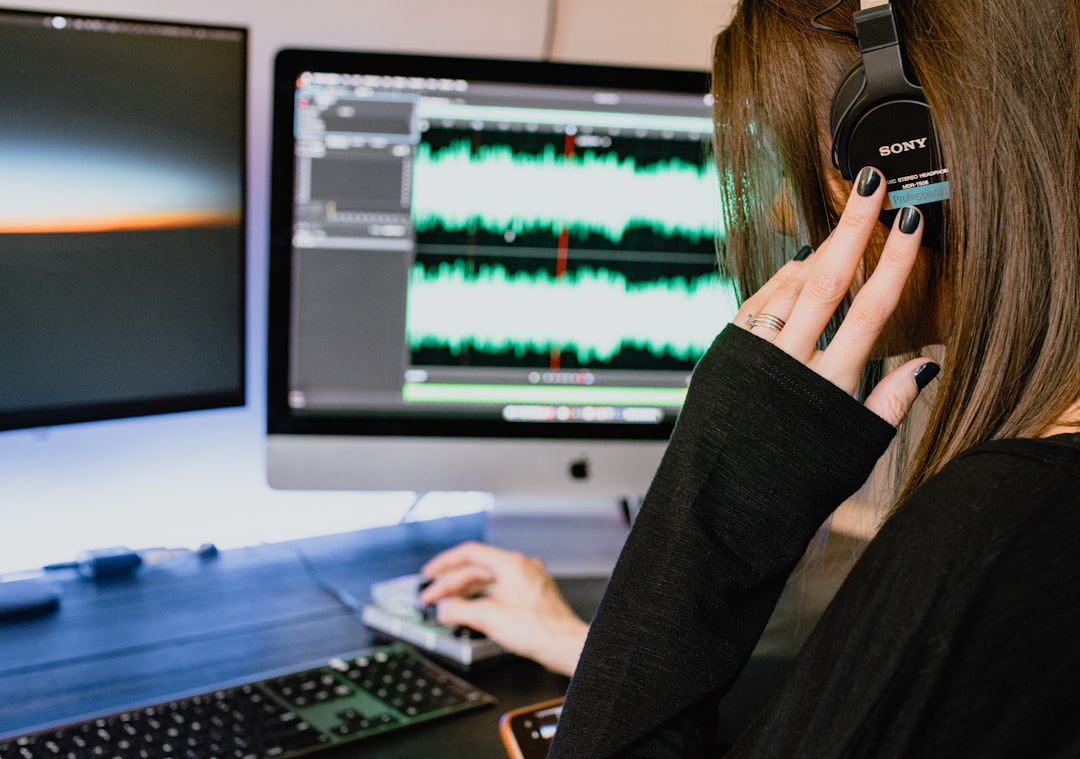
Integrating PyAudioAnalysis into your Python audio analysis workflow can elevate your capabilities beyond basic filtering. This library, designed for a wide array of audio analysis tasks, allows for the extraction of detailed audio features, including Mel-frequency cepstral coefficients (MFCCs) and spectrograms. This can be crucial when you need to delve deeper into the characteristics of audio signals.
One of the noteworthy aspects of PyAudioAnalysis is its support for both supervised classification and regression. This opens doors for more sophisticated applications like identifying emotions in speech. The ability to detect audio events and segment lengthy recordings, removing silent sections, streamlines analysis, particularly in cases where you need to extract information from longer audio samples.
While it does have its strengths, it's also worth considering some of the potential limitations. Working with PyAudioAnalysis involves understanding the different stages of its feature extraction process, which can have a learning curve. But overall, adding PyAudioAnalysis to your arsenal of Python audio libraries provides a strong foundation for advanced audio feature extraction, allowing for more insightful analysis and ultimately better audio processing results.
PyAudioAnalysis is a versatile open-source Python library tailored for a range of audio analysis tasks. It's particularly strong in feature extraction, classification, segmentation, and visual representation of audio data. You can extract a wide array of audio features using it, including MFCCs, spectrograms, and chromagrams, which are all crucial for detailed audio understanding. The library's ability to handle both supervised learning with labeled datasets and regression makes it flexible for different goals, including tasks like recognizing emotional cues in speech. It also offers methods to identify specific sounds in recordings and filter out periods of silence in long audio files.
Feature extraction within PyAudioAnalysis involves two steps. First, the input audio is sliced into shorter frames. Then, a series of features is calculated for each of these frames. This process can be fine-tuned to extract specific features related to a given project, providing flexibility. It also allows for the training, evaluation, and adjustment of classifiers on audio chunks, providing users more control over how audio is categorized. PyAudioAnalysis makes it easy to categorize unknown sounds. You can feed audio into the library and automatically classify it.
PyAudioAnalysis was developed under the leadership of Theodoros Giannakopoulos, who specializes in multimodal machine learning. Documentation on its functionalities and usage guides is readily available on the library's GitHub repository. The versatility and completeness of PyAudioAnalysis make it a go-to choice for audio analysis applications in areas like speech processing and multimedia content analysis.
While PyAudioAnalysis offers powerful tools for advanced audio feature extraction, some things to consider are:
- Real-time feature extraction can be computationally intensive, especially with complex features. Careful resource planning is necessary to prevent performance bottlenecks, particularly in low-latency applications.
- Some pre-trained models are provided, but depending on the specifics of a project, retraining or creating custom models might be needed for best results, and that can demand a significant amount of time and resources.
Overall, PyAudioAnalysis provides a strong foundation for leveraging audio features in diverse applications, providing a useful supplement to tools like high pass filters. It has the potential to greatly improve the quality of analysis performed on audio from videos, although using it successfully will necessitate a thoughtful approach to optimizing code for performance and dealing with the potentially intensive computations involved.
Implementing a Python High Pass Filter for Video Audio Analysis A Practical Guide - Visualizing Filter Results with Matplotlib
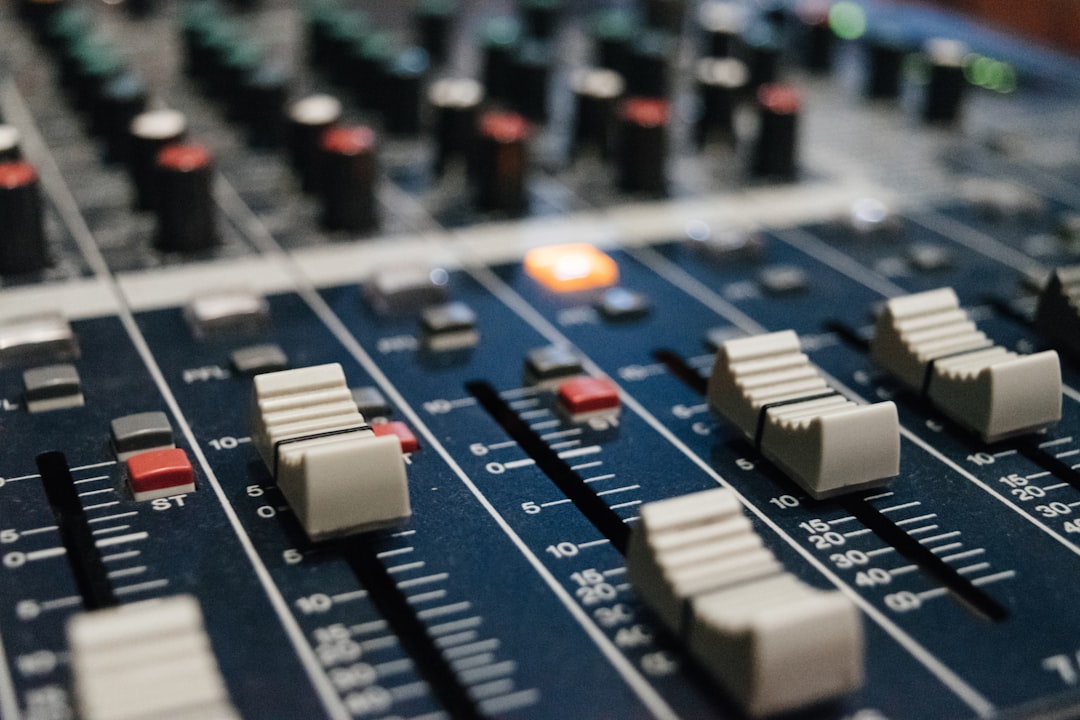
Understanding how a high pass filter (HPF) affects an audio signal is crucial, and visualization plays a key role in this process. Matplotlib's `imshow` function provides a powerful means of visualizing the outcome of applying a high pass filter to audio data. By displaying the frequency response graphically, we can gain a much deeper understanding of the filtering process. This visualization allows for the examination of the filter's effect on different frequencies, including how it attenuates lower frequencies and lets higher frequencies pass.
Furthermore, visual representation can help identify subtle issues like ringing effects, which can manifest as unwanted increases in amplitude around the cutoff frequency. Phase distortions, which can create discrepancies in timing between frequencies, are also more readily apparent through visualization.
By visualizing the filter's impact, practitioners can gain valuable insights that can inform the choice of filter parameters. This leads to a more controlled and ultimately more effective filtering process. As the complexity of audio processing projects increases, using tools like Matplotlib for visualization will continue to be an essential aspect of designing and troubleshooting high pass filters to ensure the optimal audio outcomes.
Visualizing the results of filter operations, particularly high pass filters, can be very insightful when working with audio data. Matplotlib's `imshow` function stands out as a useful tool for this purpose, letting us view 2D image representations of the filtered audio. Understanding how the filter impacts the frequency spectrum can be key to troubleshooting issues and improving our audio processing outcomes. For example, we can see if the chosen cutoff frequency effectively attenuates unwanted noise, or if there are any artifacts like ringing that might be affecting the quality of the processed audio.
However, visualizing these results is only part of the journey. It's important to keep in mind that high pass filters operate by making use of the Fast Fourier Transform (FFT), a core mathematical tool in signal processing. Understanding the FFT process is essential to fully grasp how high pass filters work.
The precise setting of the cutoff frequency directly impacts the perceived tonal balance of the filtered audio. Even slight adjustments can lead to noticeable changes in how the sound is experienced, emphasizing the need for careful tuning. As Python scripts are typically executed by an interpreter, we need to consider that potential delays introduced by filters can pose challenges in real-time situations. This concern is heightened in contexts like live sound mixing, where immediate response times are crucial.
Another aspect to consider is that applying a high pass filter can result in the audio frequencies being shifted in time, a phenomenon known as a phase shift. While this isn't always problematic, in complex audio mixes it can lead to audio that sounds less clear, since various frequencies might reach the listener at slightly different times. Also, the effects of ringing can emerge as a surprise to some. These are amplitude spikes that appear around the cutoff frequency, and if not handled properly, can introduce unwanted changes to the sound.
The good news is that Python libraries give us the flexibility to develop custom filter designs tailored to the precise needs of an audio project. By understanding the filter design parameters and utilizing the visualization features of tools like Matplotlib, we can gain a better grasp of how a filter will impact audio before implementing it. It's also crucial to be mindful of the sampling rate we use, since higher sampling rates offer improved audio detail at the expense of increased computational complexity. This is especially critical when working with audio from multiple channels, like with surround sound. In these multichannel scenarios, managing the phase relationships between different channels is paramount to maintain the intended spatial qualities of the sound.
Further, Python's libraries allow us to merge filters, such as high pass and low pass filters, to create band pass filters. These filters enable us to control a precise frequency band within the audio signal, giving us more sophisticated audio processing options. With a proper understanding of filter concepts and efficient Python implementations, visual feedback through Matplotlib can lead to a better understanding of audio data.
Implementing a Python High Pass Filter for Video Audio Analysis A Practical Guide - Optimizing Performance for Real-Time Video Audio Processing
Optimizing performance when processing video and audio in real-time is crucial for achieving a smooth and responsive experience. Python, with libraries like PyAudio and NumPy, offers tools for capturing and manipulating audio, making it a viable option for various audio processing tasks. The Fast Fourier Transform (FFT) algorithm is a vital component in real-time audio analysis, enabling efficient feature extraction from audio signals. However, achieving real-time processing in Python can be challenging, especially when dealing with computationally intensive operations.
To improve performance, techniques like GPU acceleration can significantly speed up processing, potentially leading to a 5x improvement in some cases. Furthermore, frameworks like Streamlit can streamline the development of real-time video analytics applications. It's important to acknowledge that Python's interpreter nature can introduce latency that can hinder real-time processing, especially when immediate feedback is needed, such as in live audio mixing or sound manipulation. Carefully managing code efficiency and utilizing resources optimally is key to overcoming these hurdles. This includes considering the potential trade-offs that come with higher sampling rates and complex audio feature extraction methods. In essence, a well-optimized workflow can minimize performance bottlenecks, helping create real-time applications that provide clear, responsive, and accurate audio processing results.
1. **Balancing Latency and Real-Time Performance**: Achieving true real-time audio processing in video applications hinges on minimizing latency, the delay between audio capture and output. While we aim for near-instantaneous feedback, usually under 20 milliseconds, the reality is that these systems often encounter delays that can influence user experience, especially in interactive contexts.
2. **Navigating Buffer Size Dilemmas**: The selection of an audio buffer's size is a balancing act. Smaller buffers lead to quicker responses and reduced latency, which is vital for users. However, this comes with the demand of more frequent processing, a burden that can quickly overwhelm system resources. If the processing can't keep up, it leads to interruptions, such as audio dropouts, which obviously degrades the overall quality.
3. **How Our Ears Shape Filter Design**: Understanding how humans hear, or psychoacoustics, adds another layer to high pass filter design. Humans tend to be more sensitive to certain frequency ranges. This means that even small adjustments to the filtering can have a significant impact on how people perceive audio. It's an area that's important to consider in order to create filters that both remove unwanted noise and maintain audio quality in a way that's pleasant to listen to.
4. **Cascade Filters: Power and Complexity**: Sophisticated audio applications sometimes use multiple filters in a sequence, or cascaded. This can give engineers very precise control over the sound, but it also brings complexity. If not handled properly, the interactions between these filters can create issues, like unintended alterations in frequencies or unexpected phase issues.
5. **Adapting to Changes: Dynamic Audio**: Moving beyond standard, fixed filters are adaptive techniques. In these systems, algorithms adjust the filter's parameters in response to the changing properties of the audio. This approach can be particularly useful in dynamic environments, such as live music, where the sonic landscape shifts often.
6. **Filter Order: More Power, More Issues**: Higher-order filters are beneficial in that they sharpen the filter's edges. This means that they're better at eliminating unwanted frequencies. However, they can also introduce more phase distortions, which can result in a less natural-sounding result. It's often a trade-off engineers have to manage: more accuracy in cutting out unwanted frequencies or maintaining the fidelity of the audio as it passes through the filter.
7. **Spectral Leakage: An Analysis Challenge**: A problem that comes up during audio analysis is the possibility of spectral leakage. This is when frequencies that shouldn't be present, "leak" into adjacent frequency ranges. This can skew our understanding of the true characteristics of the audio. Carefully designed filters can minimize this leakage, which can lead to a more accurate analysis.
8. **The Sampling Rate and What We Can Hear**: The chosen sampling rate has a significant influence on the quality of filtering. When the sampling rate is too low, certain frequencies in the audio signal may not be adequately captured, impacting the effectiveness of a high pass filter. A generally recommended minimum sampling rate for audio processing that adequately captures the human range of hearing is 44.1 kHz.
9. **Real-Time Visualization: A Resource Hog**: The ability to visualize the processing of audio data in real-time can be a useful troubleshooting tool, but it often comes at a cost. High-resolution visualizations can consume a lot of computational resources, potentially impacting the overall performance of the real-time processing, specifically increasing latency. This highlights the need to carefully manage system resources to maintain a balance between visualization and real-time audio processing.
10. **Machine Learning's Growing Role**: In the past few years, machine learning has begun to play a more central role in the improvement of audio processing. Machine learning algorithms can be trained on huge amounts of audio data to create optimized filtering parameters. These algorithms can adapt to different audio environments and refine the filtering process over time, resulting in better-quality output in a wide range of scenarios.
More Posts from whatsinmy.video: