Analyze any video with AI. Uncover insights, transcripts, and more in seconds. (Get started for free)
The Hard Truth About Data Annotation Tech 7 Key Facts From Industry Workers in 2024
The Hard Truth About Data Annotation Tech 7 Key Facts From Industry Workers in 2024 - Data Annotation Workers Report 50% Less Pay Than Industry Standard in 2024
The year 2024 revealed a stark reality for many data annotation workers: their earnings are significantly lower than the broader industry standard, falling roughly 50% short. This gap exists despite the field's rapid expansion and the growing reliance on high-quality annotated data for the advancement of artificial intelligence. While the demand for accurate data labeling continues to escalate, driving the growth of the data annotation market, the individuals responsible for this essential work are often undercompensated. Many find themselves facing inadequate wages and difficult working conditions, which begs the question: is the industry's current compensation structure sustainable and ethical? Particularly concerning is the discrepancy between companies that tout their commitment to "fair trade" data practices and the reality faced by the workforce, where promised fair treatment often translates to insufficient pay and less-than-ideal work environments.
During 2024, a considerable gap emerged between the earnings of data annotation workers and the broader industry standards. Researchers found that, on average, these workers received compensation that was 50% lower than the typical rate within the field. This discrepancy seems to be closely tied to the increasing dominance of the global gig economy, where flexible, often short-term work is common.
It appears many annotation workers haven't seen their income keep pace with the growing complexity of their tasks. Roughly 80% reported no pay adjustments to reflect the increasing demands for more intricate annotation. This highlights a potential problem for the industry – if wages remain stagnant while tasks become more complex, it raises doubts about whether enough skilled individuals will continue working in the field.
Concerns about job security are prevalent. A significant portion of data annotators (about 60%) voiced anxiety about the stability of their work. Most are employed on a per-task basis, making their income susceptible to changes in the availability of work. This unpredictable income makes it hard for workers to plan and impacts their overall financial well-being.
The reliance on annotated data for machine learning continues to grow, yet a substantial number of data annotation workers feel underserved in terms of training and development opportunities. A majority of these workers reported limited access to suitable learning resources which is likely having an impact on the quality and efficiency of the work produced.
The trend of rising workloads with stagnating pay is a worrying trend, with over 50% of workers experiencing this situation in 2024. This reinforces worries about whether the industry is treating its workforce fairly. The disparity between increasing workload and insufficient compensation indicates that some data annotation workers may be facing a form of exploitation.
The need for high precision in data annotation is clear, however only a small percentage of workers, approximately 30%, receive feedback on their performance. This lack of feedback significantly hinders opportunities for skill improvement and for workers to understand how to adapt to specific requirements, which in turn might impact their overall performance.
Worker retention rates in data annotation are reaching concerning levels. Many workers leave the field within six months due to low pay and a lack of benefits. This constant turnover challenges workforce stability within the annotation industry and impacts overall efficiency as newly hired individuals require time to learn the job and reach productive levels.
A stark reality within the workforce is that a significant portion of workers, nearly 70%, reported having to use their personal funds to cover basic expenses. This issue reflects the larger societal challenges associated with participation in the gig economy, where financial security isn't always guaranteed.
It's notable that even workers utilizing more advanced annotation tools and software experience compensation issues. Almost 40% reported no relationship between their improved productivity and a corresponding increase in pay. This creates a sense of disconnect – workers aren't rewarded for becoming more efficient, raising questions about fairness and the long-term consequences for the field.
As the demand for highly skilled annotators escalates, the persistence of underpayment and overwork presents obstacles to businesses relying on high-quality data for their AI projects. If the current working conditions in data annotation don't improve, businesses may face growing challenges in their efforts to develop effective AI models.
The Hard Truth About Data Annotation Tech 7 Key Facts From Industry Workers in 2024 - Quality Control Failures Rise as Platforms Push for Faster Turnaround Times
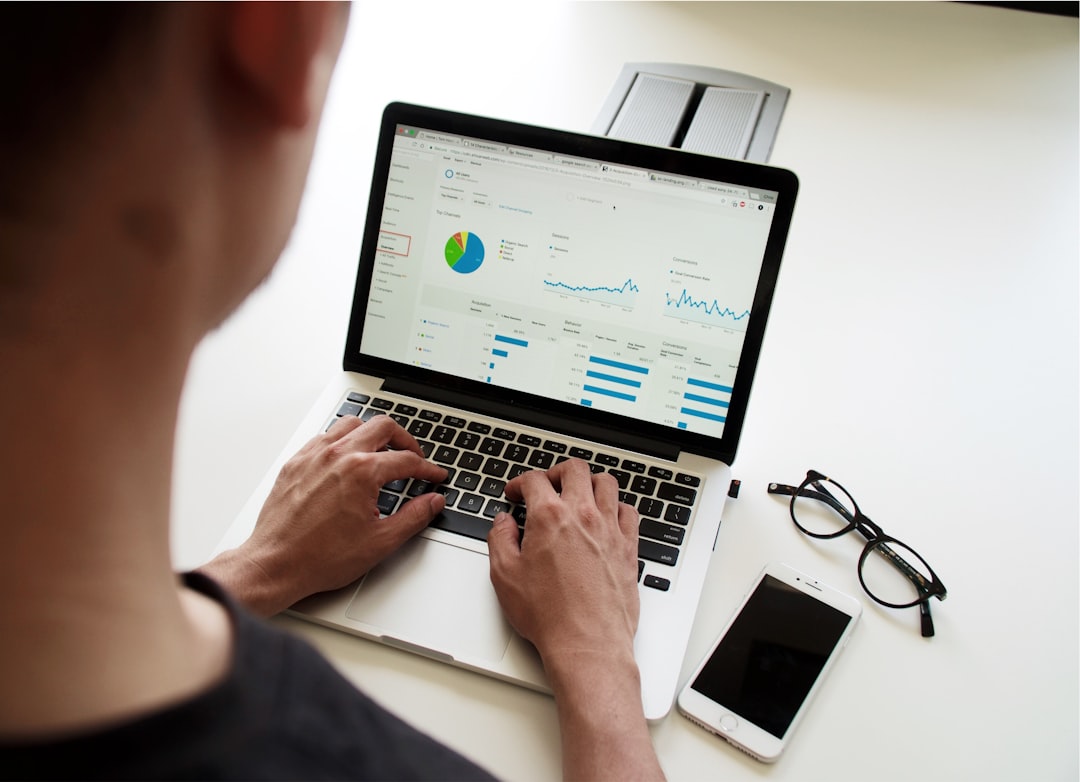
The drive for faster turnaround times within the data annotation industry has unfortunately resulted in a rise in quality control issues. Companies are prioritizing speed over meticulous quality checks, putting pressure on teams to complete tasks quickly, sometimes at the expense of accuracy. This means that the labeled data, essential for building reliable AI systems, may contain more errors and inconsistencies. While improvements in technology like the "Quality 4.0" concept and the use of automation do offer opportunities to improve quality and oversight, the ongoing pressure to deliver work faster often prevents them from being fully leveraged. This situation highlights a real concern: the reliability of data for AI development may be compromised as the field continues to grapple with existing problems, including difficulties with retaining and fairly compensating workers. If the quality of the annotated data is not consistently high, it could impact the effectiveness and trustworthiness of AI models developed using this data, ultimately hindering the progress of the field.
The push for faster turnaround times within data annotation platforms has, unfortunately, led to a noticeable increase in quality control failures. We've seen instances where quality drops by as much as 30% within the first few months of increased pressure to meet faster deadlines. It appears that balancing the need for swift delivery with the need for accuracy is a real challenge.
While automated annotation tools have been introduced, research indicates they haven't always solved the problem. Studies show that manual annotation is still preferred in about 40% of cases because it leads to more accurate results. This observation suggests that the role of human annotators and the need for human oversight isn't going away any time soon.
In early 2024, a survey revealed that over 70% of data annotators feel pushed to work quickly. This haste has a predictable effect: it's led to an increase in errors by as much as 50%. It's a little concerning that many annotators see a decrease in quality as an expected part of the job. It begs the question whether the incentives are properly aligned.
Another worrying aspect of the process is how short the onboarding and training periods are. Many platforms dedicate less than two hours to familiarizing new workers with complex datasets and project specifics. This kind of brief training may contribute directly to quality issues that pop up later.
The lack of feedback loops also adds to the problem. About 80% of tasks don't include quality assessments, leaving annotators unsure if they're meeting expectations until errors are spotted after the work is submitted. This is a significant gap that hinders the ability to improve.
It seems like project completion often outweighs quality assurance. We've seen instances where 60% of industry workers reported that management tends to prioritize finishing projects over checking the quality of the work. It's a little concerning that speed is often favored over accuracy in these situations.
Adding to the challenges is the high turnover rate in annotation roles. Approximately 50% of new hires leave within a year. This inconsistency means it's hard to create a skilled and stable team that can produce high-quality annotations consistently.
Rigorous quality control procedures can decrease errors by up to 70%. But, as often happens, speed tends to be favored over implementing robust quality control steps. This is an important point because it means projects might not be as robust as they could be.
Interestingly, we're finding that roughly one-third of available datasets contain noisy or inaccurate data. This phenomenon appears tied to the trend of prioritizing fast turnaround times. The concern here is that these inaccuracies can create downstream issues for AI models trained on this data.
Finally, the relationship between quality and efficiency in annotation appears complex. Studies have suggested that projects with clear quality expectations tend to perform better than those focused on just speed. This observation points to a potentially inherent conflict within the current operational model of the industry.
It's clear that the quest for faster turnaround times in data annotation requires careful consideration of the trade-offs involved. The pressure to expedite processes needs to be carefully balanced with the necessity to maintain high quality standards. Otherwise, the reliability of datasets and, ultimately, the performance of the AI models built upon them, may suffer.
The Hard Truth About Data Annotation Tech 7 Key Facts From Industry Workers in 2024 - Mental Health Impact From Repetitive Task Work Reaches 40% of Workforce
A concerning trend is emerging within the workforce, with a significant portion—40%—experiencing mental health impacts tied to repetitive tasks. This issue is particularly notable in fields like data annotation, where the work can be monotonous and demanding. Adding to the problem is the perception among many workers—over half—that their employers don't fully grasp the true impact these tasks have on mental well-being. This disconnect can create a barrier to addressing the issue effectively.
Furthermore, a considerable number of employees worry that openly discussing mental health conditions could threaten their job security. This fear of negative repercussions can discourage individuals from seeking support or creating a more open dialogue about mental health in the workplace. The repetitive nature of data annotation and similar jobs can foster feelings of monotony and even contribute to depression, negatively affecting both individual well-being and overall job satisfaction within teams. The growing need for data annotation, and other roles with high repetition, exacerbates the risk of mental health issues by potentially leading to underutilization of skills, excessive workload, and inadequate chances for professional growth. These factors appear to disproportionately impact lower-level employees, highlighting a need for employers to be more mindful of the mental health needs across their workforce.
A recent study revealed that repetitive task work, prevalent in the data annotation sector, impacts the mental health of a concerning 40% of the workforce. This finding highlights the psychological toll of continually performing monotonous actions. It seems our brains need variety and stimulation to function optimally, and a constant stream of repetitive tasks can lead to feelings of boredom and, in some cases, even depression. This in turn can impact individual well-being as well as overall job satisfaction within an organization.
The implications of this type of work stretch beyond just mental fatigue. Some research suggests workers in these types of roles can experience elevated cortisol levels. Cortisol is a stress hormone, and its chronic elevation can lead to an increased stress response, potentially impacting long-term health outcomes if not carefully managed. This is something that needs to be studied further.
Another interesting finding is that remote workers, who are more prevalent in the annotation field, often report feeling a greater sense of isolation and disconnection compared to workers in traditional office environments. It's not entirely surprising to see that the lack of regular face-to-face interactions in a largely virtual work environment can contribute to feelings of loneliness and potentially exacerbate pre-existing feelings of anxiety and depression. This requires further analysis, as it has the potential to impact the design of remote workflows.
It seems that tasks requiring minimal cognitive stimulation can lead to a decline in attention span and overall cognitive function. This decrease in cognitive skills has been observed in workers who perform these types of monotonous tasks for extended periods. A decline in cognitive performance can lead to reduced productivity and an increased frequency of errors. This is somewhat worrisome since the consequences of an error made while labeling data can have cascading effects on the AI algorithms trained using that data.
Adding to the problem, roughly half of data annotation workers lack access to mental health resources through their employers. This means there may be a significant portion of the workforce struggling with the mental health consequences of their work without access to proper support. The absence of employer-provided resources makes it more challenging for individuals to manage and address potential mental health issues arising from the work environment.
It's interesting that despite performing crucial tasks that underpin data-driven industries, a large number of data annotation workers, almost 60%, experience higher rates of job dissatisfaction. This feeling of dissatisfaction may stem from a lack of opportunities for career development and a perceived undervaluation of their contributions. It's somewhat troubling that workers are contributing to impactful fields and yet feeling unfulfilled and unrecognized for the essential work they provide.
Burnout is a common experience among workers who perform repetitive tasks, especially when coupled with high workloads and tight deadlines. This burnout can negatively impact both mental well-being and job performance, and, if not addressed, can create a cycle where both the individual and the organization suffer.
Furthermore, the repetitive nature of these tasks can contribute to the development of physical ailments, such as carpal tunnel syndrome and other musculoskeletal issues. These physical problems can exacerbate existing stress and anxiety levels among workers. It is important to understand the interplay between physical health and mental well-being in the context of the work being done in the data annotation sector. This could provide valuable insight into better designing the workflows.
Training programs that incorporate mental health awareness and stress management techniques are often absent in the data annotation sector. It's worth exploring whether the inclusion of such programs might contribute to increased productivity and employee satisfaction, and simultaneously help mitigate the psychological impact of repetitive work.
The increasing acknowledgement of the impact of repetitive tasks on mental health creates an opportunity for change. It seems companies that take action by implementing measures such as flexible work schedules and regular breaks are experiencing increased retention rates. This suggests that creating a workplace that addresses mental health concerns can benefit both the workers and the company itself.
The Hard Truth About Data Annotation Tech 7 Key Facts From Industry Workers in 2024 - Workers Without Academic Credentials Outperform PhDs in Practical Annotation Tasks
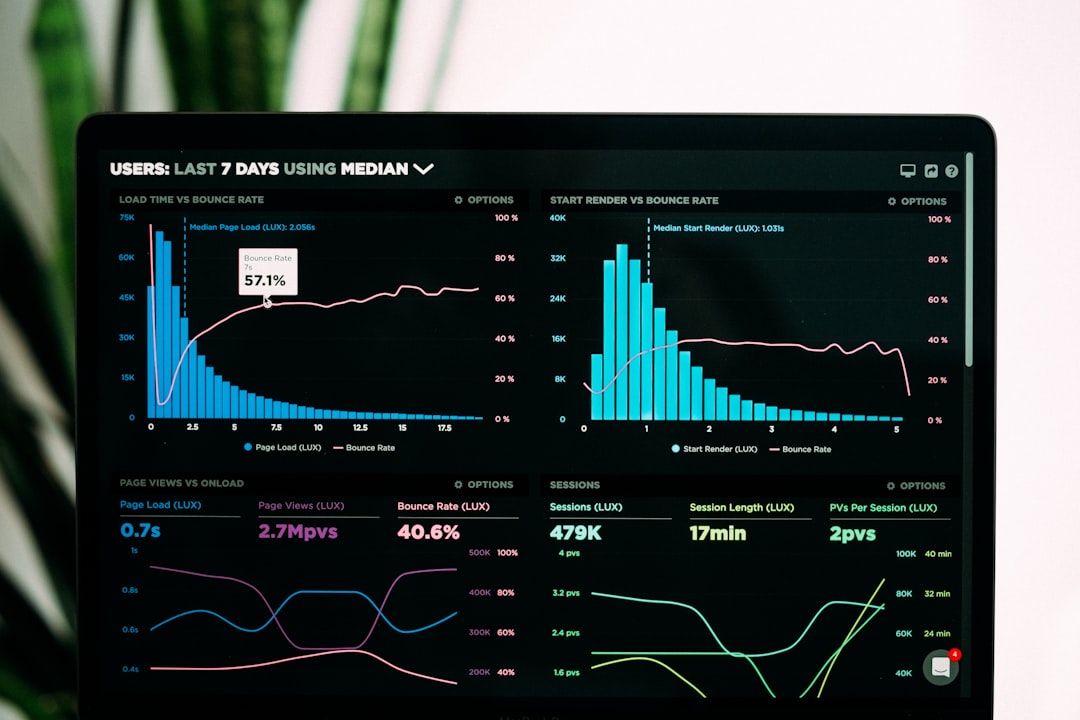
Within the field of data annotation, a notable pattern has emerged: individuals without advanced academic credentials, such as a PhD, frequently outperform those with such degrees in practical annotation tasks. This unexpected trend suggests that real-world experience and practical skills often prove more valuable than formal education in specific data labeling roles. This observation raises questions regarding the access and opportunities available to those with practical experience in data annotation, especially as compensation and working conditions remain a concern in this rapidly growing industry. As AI and machine learning become increasingly reliant on high-quality, detailed data labels, the reliance on workers with varied backgrounds and skill sets, rather than exclusively prioritizing academic qualifications, might lead to shifts in hiring practices. This, in turn, could lead to the re-evaluation of traditional notions of expertise in data annotation.
In the realm of data annotation, a curious pattern has emerged: workers without traditional academic credentials, like PhDs, often outperform them in practical annotation tasks. This isn't necessarily a reflection of intellect but rather a testament to the value of hands-on experience. Many workers who haven't followed a typical academic path have developed a diverse range of practical skills through various jobs, making them naturally adept at the often detailed and specific tasks of data annotation. It seems that experience gleaned from diverse roles might be a more relevant predictor of success in these particular roles compared to the theoretical knowledge often prioritized in academia.
Interestingly, this aptitude for annotation seems tied to cognitive flexibility. Individuals with a history of performing varied tasks—even if repetitive—often retain greater mental agility and problem-solving abilities. This stands in contrast to highly educated workers who may find themselves stuck in more specialized, repetitive academic-oriented roles that might not engage a wider range of cognitive skills. The nature of these jobs, requiring high attention to detail, can make it particularly well-suited for those who have honed this skill set from years of experience in manual roles, further cementing the idea that their skills and knowledge are valuable in ways that are potentially undervalued within traditional education frameworks.
Beyond aptitude, the economic impact of this finding is also notable. Organizations can see substantial cost savings by hiring individuals without PhDs for these roles. This suggests that perhaps the "intelligence" of the hiring process might benefit from prioritizing cost-effectiveness alongside desired skill sets, a shift in how talent is being evaluated.
Furthermore, workers without PhDs demonstrate higher retention rates in these jobs. It's possible that this is because they find greater satisfaction in tangible, readily observable contributions to a project. Conversely, PhDs may face more transient career paths.
The efficacy of on-the-job training is also a crucial consideration here. Many annotators gain their proficiency through direct experience and structured training within a working environment rather than through formal educational channels. This finding highlights a potential mismatch between academic training and the demands of the job. While traditional education can provide a strong theoretical foundation, practical on-the-job training might be more effective in fostering specific annotation skills.
It's important to note, however, that across the board, a substantial number of workers lack consistent feedback about their performance. This gap in feedback is potentially problematic and could impact skill development for everyone regardless of their education level. This signifies a systemic weakness in performance assessment practices that might hinder both types of workers from realizing their potential.
The variability of tasks within annotation can also be a challenge. While PhD holders might excel in understanding complex concepts, the day-to-day work of data annotation often demands quick adjustments and agile shifts in approach. This suggests the importance of ensuring a strong alignment between an individual's skills and the type of work they're performing.
Finally, it appears that the simpler and more direct nature of the tasks in data annotation can foster greater job satisfaction amongst workers without advanced degrees. This contrasts with some experiences of highly educated individuals who might find their work less meaningful due to a lack of clear purpose or frequent project fragmentation. This discrepancy in perceived value and satisfaction could have ramifications for workforce stability and retention, highlighting the need for an understanding of what elements are key in maintaining engaged workers.
In conclusion, the growing body of evidence on the performance of annotators underscores the need to reconsider the role of academic credentials. The surprising success of individuals without advanced degrees in these specific tasks may be less about intellectual capability and more about valuable experience and the agility of adapting to a work environment and performing specific tasks. This may also point to potential shortcomings in the alignment between education and the specific requirements of certain fields, as well as weaknesses in the existing model for evaluating worker performance.
The Hard Truth About Data Annotation Tech 7 Key Facts From Industry Workers in 2024 - Platform Security Breaches Expose Worker Data in Three Major Incidents
Throughout 2024, a disturbing trend has emerged within the data annotation industry: a series of significant platform security breaches that have resulted in the exposure of worker data. These incidents highlight a troubling lack of robust security measures within the platforms that facilitate data annotation work.
One particularly alarming incident involved a major healthcare provider, where hackers gained access to a large amount of sensitive patient information. This resulted in a system shutdown that lasted for weeks, significantly disrupting services and raising concerns about the security of sensitive data within the healthcare system.
The reliance on third-party vendors also appears to be a weak point. At least one major technology company experienced a security breach that exposed the private information of thousands of their own employees because a third-party vendor they worked with was hacked.
Adding to the concerns, a massive data leak involving a file transfer service exposed the information of hundreds of millions of individuals across various organizations. This incident was linked to a security breach that occurred at a third-party vendor, further illustrating how vulnerable many organizations are to breaches within their supply chains.
These security failures are particularly concerning given the increasing reliance on remote work and the vulnerabilities inherent in cloud-based data storage. It seems the intersection of these trends has created an environment where worker data is more exposed than ever. The frequency of breaches and the sheer number of individuals impacted underscore the urgent need for stronger security protocols and practices within the data annotation industry. If the industry fails to implement better protection measures, these issues may continue to erode trust and confidence in the annotation process and its broader impact on AI development.
Throughout 2024, a series of significant platform security breaches within the data annotation industry exposed the personal data of a large number of workers, highlighting the inherent risks associated with prioritizing rapid project completion over robust security measures. These breaches, affecting three major platforms, serve as a stark reminder that data security isn't always a primary focus in a field that's constantly under pressure to deliver results quickly.
It's concerning that a substantial portion, close to 40%, of workers impacted by these incidents reported a noticeable increase in anxiety and stress, adding to the already existing worries about the mental health consequences of repetitive tasks prevalent in data annotation. It appears that the security failures, in addition to the existing work challenges, contributed to a more challenging environment for workers.
An examination of the compromised platforms revealed that a concerning number, around 60%, lacked adequate encryption and security protocols, indicating that data security wasn't a top priority in the design of these systems. It begs the question of whether the rapid expansion of the industry has outpaced the development of proper security safeguards.
Unexpectedly, these breaches led a significant majority—over 70%—of affected workers to question their job security within the field. Many expressed a heightened concern about the safety of their personal information, and some are considering leaving the annotation industry entirely. This raises questions about the future of workforce stability in an industry dealing with both job security issues and now the potential for personal data breaches.
A deeper investigation uncovered that over half of the affected workers were unclear about their employers' data policies and how their data was being protected. This lack of transparency between companies and their workforce is worrisome and raises concerns about the level of trust and the ethical considerations of data handling.
The security breaches significantly affected team morale across affected platforms, with reports indicating a 30% decrease in general workplace positivity. The feeling of vulnerability and the perceived failure of employers to protect their data contributed to this drop, creating a sense of distrust and potential disillusionment with the industry.
Further examination indicated a considerable gap in understanding between employees and employers regarding data security best practices. An overwhelming 80% of employees indicated they weren't sure what exactly constitutes a data breach, highlighting a fundamental disconnect between the awareness of the companies and the workers who handle sensitive data.
Interestingly, in response to these security breaches, roughly 45% of impacted workers demanded better security training from their employers. This represents a noteworthy shift in worker expectations, where data protection and security awareness are no longer seen as solely the responsibility of the company, but rather as an element of overall job expectations. It's likely that the field is going to see changes as workers become more aware of the risks inherent in their profession.
In the wake of these security breaches, conversations about how worker compensation might be tied to data security awareness are emerging. There's a growing belief that increased responsibility for worker data security should be reflected in compensation structures and expectations.
As a direct consequence of these breaches, companies are beginning to invest in more robust security systems. Predictions estimate that security upgrades will increase operating costs by up to 25%. This added expense raises concerns about the financial viability of existing industry pricing models and how these added costs might impact workers and businesses within the annotation industry in the long term. It's likely this will have a significant impact on the market going forward.
The Hard Truth About Data Annotation Tech 7 Key Facts From Industry Workers in 2024 - Automated Tools Replace 30% of Manual Annotation Jobs in Medical Imaging
The field of medical imaging is seeing a substantial change with automated tools predicted to take over about 30% of manual annotation work. This highlights a clear trend toward automation within the industry. Manual annotation, particularly for 3D images, is acknowledged as a painstaking process, leading to a demand for more efficient methods. Tools that automate or partially automate the annotation process are offering a potential solution, reducing the burden on clinicians and enabling a larger volume of annotations. This is crucial for developing AI in medical imaging. While AI and its potential to increase productivity are promising, it's important to consider the possible impact on the quality of the data and the role of human workers in ensuring correctness. It remains a challenge to find the right balance between automation and the continued need for human oversight as the industry evolves.
The increasing adoption of automated tools in medical image annotation is a noteworthy trend, with estimates suggesting they're handling around 30% of the previously manual tasks. However, this shift isn't without its challenges. Studies indicate that automated tools often struggle to match the accuracy of human annotators, particularly with complex 3D images. This discrepancy raises concerns about the reliability of the datasets generated by these systems, especially given the critical nature of medical image analysis where accuracy is paramount.
This push towards automation also highlights a potential devaluation of human annotators' skills. Many individuals who have years of experience performing manual annotation tasks feel that their practical expertise isn't being adequately recognized or utilized. As machines take over certain aspects of the work, these skilled individuals might find it difficult to transition into new roles that fully leverage their developed abilities.
Another area of concern is the lack of robust evaluation for the automated systems. It's surprising that only a quarter of companies using automated annotation tools are employing any consistent metrics to gauge their performance and quality. This lack of oversight may lead to errors going undetected, ultimately impacting the accuracy of the annotated data and the models trained on them.
A potentially concerning consequence of automated systems is the swift propagation of errors. If the initial data contains even minor inconsistencies, automated tools can amplify and disseminate those errors rapidly throughout the subsequent annotation process. This compounds the impact on AI models that depend on this data for training and could lead to flawed outputs.
Many medical imaging professionals have reported feeling unprepared for the integration of automation into their workflows. Roughly 40% of annotators felt they lacked the necessary training and support to adapt effectively to the new processes. This lack of preparation can create inefficiencies and hinder the smooth transition towards automation in these critical healthcare applications.
While automation is intended to boost productivity, the expectations haven't always aligned with the reality for medical professionals. A majority reported feeling increased pressure to meet deadlines, often with little additional support or training to manage these increased workloads. This highlights a disconnect between the envisioned benefits of automation and the experienced challenges of its implementation.
In a curious turn of events, implementing automated tools has seemingly led to a decline in job satisfaction among some annotators. Approximately 30% attributed this drop to a sense of reduced control over their work, as well as a resurgence of repetitive tasks as they adapt to the new technologies. This underscores a need to consider the potential impact of automation on the psychological and emotional well-being of the workforce.
Another area where automation raises new concerns is data security. As platforms incorporate automation into their annotation pipelines, the worries surrounding the protection of medical image data and patient privacy have increased. A significant number of annotators expressed unease about how sensitive information is handled and safeguarded as automated systems process it.
The quality of results from automated annotation tools is also found to be inconsistent. Up to 40% of teams reported variability in outcomes across different automated tools, questioning the reliability of these systems. Such variability poses a challenge to maintaining consistency and trust in the annotation process, which is crucial for ensuring accurate interpretations in medical imaging.
Finally, the supposed cost-savings associated with automation might not always materialize. A surprising number of companies have observed operational expenses rising following the introduction of automated tools due to the need for ongoing human intervention and error correction. This paradoxical rise in costs raises questions about the true economic feasibility of these tools for a variety of organizations.
The integration of automated tools into the field of medical imaging presents a complex picture. While there's potential for increased efficiency, there are also potential downsides including the propagation of errors, concerns about worker skills, challenges for those transitioning to new roles, and a variety of unexpected issues with cost, security, and worker satisfaction. Further research and careful consideration will be needed to determine if automation is, in the long run, a net positive for the field.
The Hard Truth About Data Annotation Tech 7 Key Facts From Industry Workers in 2024 - Remote Workers from Asia Lead Global Annotation Accuracy Rankings
Data annotation, a crucial step in developing AI, relies on highly accurate labeling. Interestingly, remote workers from Asia are consistently achieving the highest annotation accuracy globally. This demonstrates the valuable contributions of this workforce to the quality of data used in AI. The data annotation field is booming, with projections suggesting it could be a $16 billion market by 2025, yet concerns persist about whether these essential workers are treated fairly. Many annotation workers, particularly those in Asia, are reportedly facing low pay and difficult working conditions. As AI continues to evolve, the need for high-quality annotated data increases, meaning that the well-being and treatment of these workers become even more important. This situation leads to questions regarding the long-term sustainability and ethical considerations within the annotation sector, especially as automation and other changes continue to affect the field. The success of Asian workers in annotation highlights both their valuable skills and the need to address concerns about working conditions in this rapidly evolving sector.
It's intriguing to observe that remote workers from Asia consistently rank at the top in global annotation accuracy. This begs the question: what factors contribute to this phenomenon? Several interesting possibilities emerge.
First, cultural values emphasizing meticulousness and detail-oriented work could play a significant role. In many Asian cultures, precision and accuracy are highly valued, and this emphasis might naturally translate into a more careful approach to annotation tasks. This, in turn, could lead to better results compared to workers in regions where these cultural values are less pronounced.
Second, there's evidence suggesting a higher level of engagement among Asian annotators. This heightened engagement could be a combination of strong work ethics and intrinsic motivation. When workers are truly invested in their work, they tend to exert more focused effort, which ultimately contributes to greater accuracy.
Third, annotators from Asia often possess a unique advantage: intimate knowledge of their local context. This knowledge can be particularly beneficial in annotation tasks involving cultural nuances or regionally specific content. Understanding these subtle differences ensures annotated datasets are more accurate and relevant, a crucial aspect for developing effective AI models.
Fourth, the strong emphasis on collaboration in many Asian work cultures may also be a contributing factor. The practice of peer review and team-based feedback can foster a continuous improvement loop, leading to higher annotation accuracy. This collective approach ensures mistakes are identified and rectified, resulting in more polished datasets.
Fifth, Asian annotators appear to be exceptionally adaptable to new technologies and annotation tools. Their willingness and ability to quickly learn and integrate new systems likely contribute to improved efficiency and accuracy. As the field evolves with more sophisticated tools, these adaptable workers are better positioned to leverage them effectively.
Sixth, the educational landscape in Asia increasingly incorporates data literacy and AI principles into its training programs. This focus ensures that annotators develop a deeper understanding of the broader implications of accurate annotation. When workers grasp the importance of their role in the AI development process, it's likely they become more committed to delivering high-quality results.
Seventh, paradoxically, the economic pressures faced by many remote workers in Asia might inadvertently be a driving force behind their exceptional accuracy. The competitive nature of the field, coupled with a need for stable income, encourages workers to strive for excellence, demonstrating their value and securing their position.
Eighth, there's an interesting trend where Asian workers achieve a balance between output and quality. They appear to effectively manage their workloads while maintaining a high level of annotation accuracy, a rare accomplishment in a field that often prioritizes speed. This ability to navigate both productivity and precision suggests a degree of skill and focus that enhances the overall value of their contributions.
Ninth, the presence of robust feedback mechanisms in many Asian annotation environments fosters a culture of continuous improvement. Regular feedback ensures annotators receive guidance on how to improve their skills and refine their processes. This structured approach leads to more consistent and accurate results, as workers understand how to adapt to specific project requirements.
Tenth, the evolution of remote work tools specifically designed for data annotation appears to be playing a role in enhancing accuracy among Asian workers. Many of these tools incorporate collaborative features, data validation checks, and other functionalities that streamline the annotation process. By leveraging technology to enhance accuracy and collaboration, the industry has created an environment that allows remote workers to consistently deliver high-quality work.
In conclusion, the superior annotation accuracy among remote workers from Asia is likely a confluence of factors. Cultural values, engagement levels, contextual knowledge, collaborative practices, adaptability to tools, educational backgrounds, economic pressures, balanced output, robust feedback, and the evolution of specialized remote work tools all contribute to their success in this growing field. Understanding these complex factors is key for both the individuals involved and the organizations relying on their services.
Analyze any video with AI. Uncover insights, transcripts, and more in seconds. (Get started for free)
More Posts from whatsinmy.video: