The Science Behind Slow-Motion Video Converters How Frame Interpolation Enhances Footage
The Science Behind Slow-Motion Video Converters How Frame Interpolation Enhances Footage - Understanding Frame Interpolation Algorithms
To grasp how slow-motion video converters work their magic, understanding the inner workings of frame interpolation algorithms is key. These algorithms effectively boost the frame rate of a video by generating new frames between existing ones. This process results in smoother and more fluid motion.
Modern approaches leverage sophisticated techniques, like multiscale motion reconstruction networks and convolutional neural networks, to tackle the complexities of scenes with substantial motion or objects obscuring the view. These advancements enhance video quality and offer a more immersive viewing experience.
However, current methods often face difficulties when confronted with intricate motion sequences. This highlights that continuous refinement and further research are necessary. Frame interpolation is a dynamic area, constantly evolving. It's a crucial technology with far-reaching applications, impacting everything from animation and video restoration to a range of other video-related tasks.
Frame interpolation algorithms work by predicting the movement of pixels between existing frames, essentially generating new frames to create a smoother, higher frame rate video. Some more sophisticated algorithms utilize deep learning, enabling them to predict intricate motions with impressive accuracy, even in scenes with rapid or unpredictable movement. Beyond simple frame insertion, these algorithms can adapt to different frame rates, which is quite useful when converting between display standards like converting 24 frames per second footage to a higher frame rate like 120fps.
However, there's a potential downside known as temporal aliasing. When original footage features fast-moving objects, the interpolated frames can appear jittery or have a ghosting effect if the algorithm misjudges the motion. Furthermore, interpolation can introduce its own visual artifacts, such as blurring or unnaturally smooth motion trails. This is particularly noticeable in scenes with sudden camera movements or where objects obstruct each other.
Many frame interpolation methods rely on optical flow calculations to track pixel movement between frames, but they struggle with transparency and reflective surfaces, producing subpar results in these situations. To mitigate some of these shortcomings, some approaches blend traditional interpolation techniques with machine learning enhancements, which allows for a more adaptive and responsive output tailored to the individual characteristics of each scene.
The level of sharpness or softness applied to the generated frames has a considerable impact on the viewer's experience. Too much sharpening can lead to an artificial look, while excessive softness can cause loss of crucial details within the image. Generating high-quality interpolated frames is computationally demanding. Real-time processing usually requires advanced hardware like specialized GPUs for efficient handling of complex calculations.
While primarily used for enhancing slow-motion, frame interpolation shows promise in other areas like virtual reality and augmented reality. By creating a smoother, more fluid motion experience, it can contribute to more immersive and engaging interactions within these digital environments.
The Science Behind Slow-Motion Video Converters How Frame Interpolation Enhances Footage - The Role of Artificial Intelligence in Video Enhancement

Artificial intelligence has become a pivotal force in improving video quality, particularly within the realm of frame interpolation for slow-motion effects. AI-powered algorithms are capable of generating new frames between existing ones, leading to a seamless flow of motion and a richer visual experience. This process not only enhances slow-motion video but also refines the overall quality of various video formats.
Advanced AI algorithms, such as those employing convolutional neural networks, excel at predicting pixel movement and creating smooth transitions in video footage. These systems can account for complex scene details, including depth, resulting in more realistic slow-motion and other enhanced effects. However, AI methods are not without their limitations. Challenges like temporal aliasing, which can cause a jittery or ghosting effect, and the potential for artificial-looking blur or smoothing continue to be areas of active development and research.
The integration of AI into video processing offers exciting prospects for the future of video enhancement. It's a dynamic field with the potential to refine not only slow-motion video but also to elevate the standards for all forms of video playback. While challenges remain, the continual development and application of AI promise to create even more realistic, fluid, and visually stunning videos in the years to come.
Artificial intelligence is playing a growing role in enhancing video quality, particularly in the area of frame interpolation. AI-powered algorithms can take videos captured at relatively low frame rates, perhaps 24 frames per second (fps), and boost them to much higher rates, like 120 fps or even more. This can lead to significantly smoother motion, without requiring the original footage to be shot at a higher frame rate.
The ability to learn complex motion patterns is a key advantage of using convolutional neural networks (CNNs) for frame interpolation. These algorithms can refine their predictions over time as they encounter more diverse visual scenes. However, challenges remain, especially in scenes with significant occlusions, where objects partially obscure others. In these scenarios, artifacts like ghosting or blurring can arise, compromising the overall realism of the interpolated frames.
Some of the more advanced uses of frame interpolation are found in professional environments. For instance, sports broadcasters rely on AI-based frame interpolation for smooth slow-motion replays. These improved replays offer viewers more comprehensive analyses of the action, enhancing the viewing experience.
Beyond simply recognizing motion, modern interpolation techniques are becoming increasingly sophisticated. They can differentiate between different kinds of movement, like translational and rotational shifts, which helps them generate more precise frames in various contexts. Interestingly, the benefits extend beyond traditional video. Frame interpolation can also be used in animation to smooth out transitions between frames, resulting in more polished character movements and overall animation quality.
One of the persistent issues with frame interpolation is temporal aliasing. It can create a range of artifacts, from ghosting to the duplication or smearing of fast-moving objects. When objects move very quickly, the interpolated frames might misinterpret their positions, leading to a distorted or unrealistic appearance of the scene, which shows some inherent limitations of current techniques.
Achieving high-quality interpolated frames requires significant computing power. Even with advanced GPUs, processing video in real-time for interpolation can be a demanding task. This places strain on available resources, especially in consumer-grade electronics.
It's not just about slow motion though. Frame interpolation finds uses in film restoration where it can help breathe new life into older footage, improving both details and smoothing out any jumpy motions originally captured during filming.
We're also seeing the incorporation of frame interpolation into augmented reality (AR) applications. Here, the smoother, higher frame rate footage allows for seamless blending of virtual elements onto live video, creating a more immersive experience where the virtual and real appear seamlessly integrated in a user’s environment.
The field of frame interpolation, especially when powered by AI, is constantly evolving, continually exploring ways to improve the accuracy and efficiency of the frame generation process. It will be fascinating to see how these developments continue to refine video viewing experiences in the future.
The Science Behind Slow-Motion Video Converters How Frame Interpolation Enhances Footage - Frame Rate Conversion From Standard to High FPS
Frame rate conversion is a technique used to change the speed at which video frames are displayed, often converting standard frame rates like 24 or 30 frames per second (fps) to higher rates like 60 fps or beyond. This is commonly done to achieve smoother playback and create the effect of slow motion. The process generally relies on frame interpolation, which essentially involves creating new frames in between existing ones, leading to a more fluid visual experience. While this technology has progressed significantly, traditional methods of frame rate conversion have sometimes struggled with producing high-quality results. The process could often lead to unwanted artifacts or inconsistencies in the output. However, newer methods using AI are showing promise in overcoming some of these limitations. These AI-powered approaches can more effectively analyze motion within the video and adapt the frame generation process, producing superior slow-motion results. Despite these advancements, challenges remain. One ongoing issue is the risk of creating temporal aliasing, which can lead to unwanted jitters or ghosting effects in fast-moving scenes. Additionally, achieving a visually pleasing balance between sharpness and smoothness while minimizing artifacts continues to require refinements. Given these persistent challenges, frame rate conversion technology remains a dynamic area of research and development, with improvements constantly emerging to achieve more natural and effective slow-motion effects.
Frame rate conversion, the process of transforming a video's frame rate from standard rates like 24 or 30 frames per second (fps) to higher rates like 60 fps or beyond, is more than just increasing the frame count. It fundamentally changes how we perceive motion. For example, while 24 fps is typical in film, converting it to 120 fps can result in a far more fluid, smoother representation of movement, altering the viewer's sense of time.
One of the main challenges in frame rate conversion is managing motion blur, especially when dealing with quickly moving objects. Algorithms have to accurately predict not only the object's path but also the blurring that naturally occurs in lower frame rate footage, making the frame creation process much more complex.
Frame interpolation seems to be quite sensitive to the direction of motion within a scene. Diagonal movements tend to lead to a higher number of artifacts compared to vertical or horizontal ones. This is often due to optical flow techniques being more effective at calculating linear motions versus movements involving complex shifts in trajectory.
Interestingly, the efficacy of frame interpolation might actually decrease as we push frame rates higher. While upscaling from 24 fps to 60 fps can be visually impressive, attempting to go to 120 fps or higher can bring certain algorithm shortcomings to the forefront, like jitter or unnatural-looking motion trails in rapid movement scenes.
The accuracy of these interpolation algorithms can depend on the type of video being processed. Videos featuring high levels of detail and intricate textures pose a significant challenge for these algorithms, requiring them to navigate and predict frame transitions without introducing unwanted distortions to the intricate visual elements.
Some of the more advanced interpolation techniques use Generative Adversarial Networks (GANs) where two neural networks essentially compete to generate more lifelike and higher quality interpolated frames. This competitive approach has led to improvements in the visual quality of the final output compared to more traditional techniques.
Temporal aliasing, a common outcome of frame interpolation, can take different forms, including ghosting and strobing effects. These artifacts are often seen in instances of rapid motion and highlight limitations inherent to the process, reminding us that there's still room for refinement in algorithm design.
Beyond improving video, frame interpolation has applications in the gaming industry. Generating frames in real time can reduce latency and enhance the smoothness of gameplay, creating a more immersive and enjoyable experience for gamers.
It's important to note that not all video content benefits equally from frame interpolation. For example, scenes with minimal or slow motion might not see major improvements with frame interpolation, and the insertion of additional frames in these cases could sometimes result in unnatural smoothness that deviates from the original aesthetic.
Finally, the computing power required for real-time frame interpolation is substantial, requiring high-performance hardware for optimal results. This limits broader adoption in consumer electronics where cost and processing power remain key factors.
The Science Behind Slow-Motion Video Converters How Frame Interpolation Enhances Footage - Applying Deep Learning to Generate New Frames
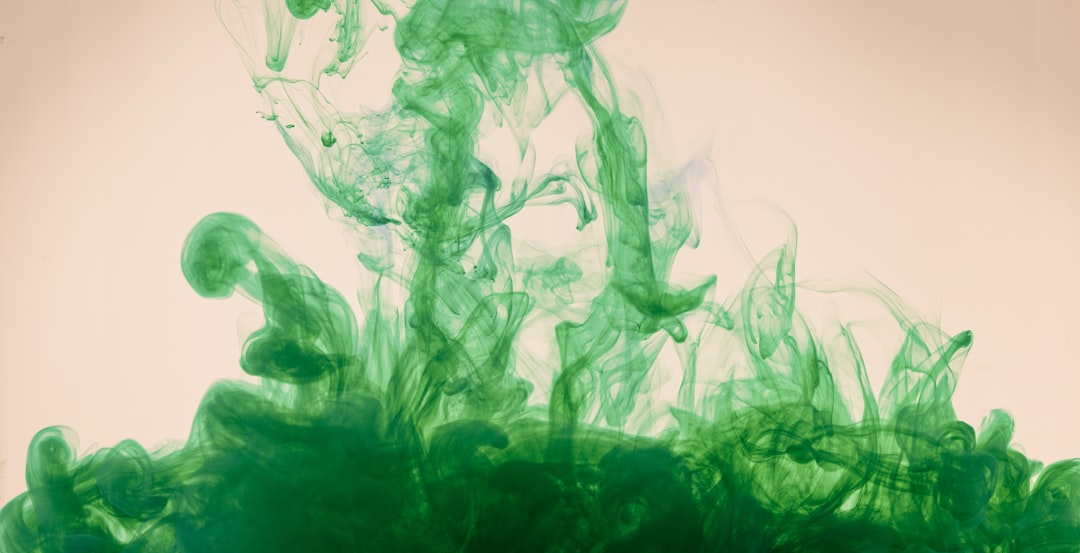
Deep learning has significantly impacted how we create slow-motion videos by enabling the generation of entirely new frames within existing video sequences. This capability allows for a smoother, more fluid playback experience, offering a richer visual representation of motion. Methods utilizing neural networks, like the Super SloMo project, demonstrate the potential for AI to precisely generate intermediate frames, which is particularly beneficial in scenes with rapid movement or objects that obscure the view, situations that have traditionally been difficult for simpler techniques.
Despite these advancements, some challenges remain. For instance, the potential for temporal aliasing persists, leading to occasional visual glitches like ghosting or jittery movement, especially when rapid motion is involved. This highlights that while deep learning algorithms are very effective at creating intermediate frames, they are not perfect. Nonetheless, ongoing research into new methods, such as motion-aware latent diffusion models, shows that developers are working to address these issues.
The marriage of deep learning and frame interpolation offers a compelling future for video enhancement, with the possibility of generating even more realistic, visually stunning, and fluid videos. However, it's essential to acknowledge that these powerful techniques are not without their limitations and can still produce certain visual artifacts that require attention.
Deep learning techniques, particularly those utilizing convolutional neural networks, have revolutionized how new frames are generated in video interpolation. They excel at predicting intricate pixel movement, which is crucial for creating smooth slow-motion effects. However, they can face difficulties when confronted with complex scenes containing fast-moving objects, sometimes resulting in artifacts like inaccuracies or ghosting.
Some advanced approaches now use generative adversarial networks (GANs) to improve frame quality. GANs work by pitting two neural networks against each other—one generating frames, and the other evaluating their realism. This competitive structure has helped to push the realism of interpolated frames beyond what was achievable with older methods.
While boosting frame rates significantly improves smoothness and delivers an improved viewing experience, going too far—like pushing from 60fps to 120fps or higher—can sometimes exacerbate existing weaknesses in the algorithms. This often manifests as unnatural motion trails or jitters, negatively impacting the overall aesthetic.
Traditional methods often rely on optical flow calculations to track motion. These methods, however, can stumble when dealing with complex visual elements like reflective or transparent surfaces, leading to issues such as ghosting or inaccurate frame generation.
Generating high-quality interpolated frames in real-time requires powerful computing resources, typically involving specialized GPUs. This demand for advanced hardware can make it challenging to integrate into consumer electronics, where cost and power efficiency are major concerns.
Temporal aliasing, a known limitation in frame interpolation, can appear in various forms, such as strobing or ghosting, particularly in scenes with rapid motion. This underscores the need for ongoing research into improved methods to reduce these types of artifacts.
The effectiveness of interpolation algorithms can depend on the kind of content being processed. Footage with complex textures and detailed visual elements can be challenging, demanding careful management of the frame creation process to avoid introducing distortion or visual degradation.
The core principles of frame interpolation also find applications beyond enhancing slow-motion video, including the gaming world. The real-time creation of additional frames in games can reduce latency and create a more smooth and immersive experience. However, this benefit comes at the expense of high hardware demands.
The level of improvement through frame interpolation varies between different types of video content. Clips with very little or slow motion may not see significant benefits, and the addition of frames can sometimes result in an artificial or unnatural smoothness that deviates from the original video's character.
Newer deep learning approaches can analyze a video's depth and the spatial layering of elements within a scene. This enables them to predict more accurate frames by accounting for the positions of objects relative to one another. However, this enhanced functionality comes with increased computational demands.
Frame interpolation is a continually evolving field, particularly due to the advances in AI-driven algorithms. Future research will likely focus on refining algorithms to produce even more seamless and realistic video experiences.
The Science Behind Slow-Motion Video Converters How Frame Interpolation Enhances Footage - Overcoming Limitations of Traditional Slow-Motion Capture
Traditional methods for capturing slow-motion often struggle with accurately representing high-speed motion, frequently resulting in noticeable blur and other artifacts. This limitation stems from the inherent constraints of capturing a limited number of frames within a brief timeframe. However, the field of video frame interpolation has emerged as a powerful tool to overcome these shortcomings. Modern approaches, especially those incorporating deep learning, can generate new frames between existing ones. This allows for the creation of slow-motion effects that are significantly smoother and more detailed than what was previously possible.
Sophisticated techniques like motion-aware and content-aware frame interpolation are designed to address particularly challenging situations like those with significant motion blur or changes in lighting. These techniques leverage advanced algorithms and machine learning to predict the movement of pixels with greater precision, ultimately enhancing the quality and realism of the generated slow-motion. Although these advancements are promising, there are still limitations that researchers continue to explore.
The ongoing development of video frame interpolation signifies a critical step forward in how we capture and perceive motion in video. The technology holds the potential to redefine slow-motion video, allowing for a more immersive and visually engaging experience. As research progresses, we can expect continued improvement in the ability to generate high-quality slow-motion, effectively addressing past limitations and opening new possibilities for creative storytelling.
Traditional slow-motion capture usually relies on cameras capable of capturing very high frame rates, like 120 frames per second or even higher. However, the advancements in frame interpolation allow us to enhance videos shot at standard frame rates, such as 24 or 30 frames per second, effectively mimicking the look of high-speed capture without requiring specialized hardware. This opens up possibilities for creating slow-motion effects in a wider range of scenarios.
One of the challenges that older techniques face is their dependence on optical flow calculations. These calculations can sometimes misinterpret quick movements of pixels, which can lead to problems like ghosting in the resulting video. More recent methods that leverage artificial intelligence often mitigate this by learning patterns from the video data instead of just calculating paths, resulting in a better ability to predict motion.
Certain types of video scenes prove difficult for frame interpolation techniques to handle properly, specifically those containing transparent or reflective surfaces. Conventional algorithms often struggle to accurately track the movement of these elements, leading to a noticeable dip in quality compared to other kinds of video footage.
Interestingly, the performance of frame interpolation techniques can sometimes worsen when excessively high frame rates are targeted. For instance, converting video from 30 frames per second to 60 frames per second usually produces a smooth outcome, but pushing it to 120 frames per second or higher can result in the appearance of jitters due to limitations in the current algorithms.
Temporal aliasing, which produces unwanted motion artifacts like flickering or duplicated images in fast-moving parts of a video, continues to be a common challenge for both conventional and AI-driven methods. Despite the progress in the field, this challenge shows us that there is still room for further development in the algorithms.
Recent innovations employing Generative Adversarial Networks (GANs) have demonstrated a promising path toward improving the quality of the generated frames. By having two neural networks compete with each other, GANs help generate frames with a greater sense of realism, thereby addressing some limitations inherent in older interpolation methods.
The level of detail found in a video has a major impact on the final quality of the interpolated output. Video clips that have intricate textures and complex motion patterns need algorithms that can both preserve details and generate smooth transitions. This process is also quite computationally intensive.
Frame interpolation isn't solely valuable for slow motion, but also widely used in the gaming world. The ability to generate extra frames can enhance the smoothness of gameplay. This rising demand in gaming has led to a greater need for more powerful hardware to handle real-time frame processing.
The computational requirements for producing high-quality interpolated frames shouldn't be overlooked. Even with advanced graphics processing units (GPUs), real-time processing can be a challenge. This makes it difficult to bring this kind of sophisticated capability to more affordable consumer electronics without impacting other performance aspects.
As frame interpolation algorithms continue to advance, future research is likely to focus on methods that are less computationally demanding while maintaining the visual integrity of the interpolated frames. This will contribute to wider adoption and an even better user experience.
The Science Behind Slow-Motion Video Converters How Frame Interpolation Enhances Footage - Future Developments in AI-Powered Video Processing
The future of AI-powered video processing, specifically within frame interpolation for slow-motion effects, holds exciting potential for reshaping our visual experiences. Emerging techniques like Depth-Aware Video Frame Interpolation (DAIN) and Content-Aware Frame Interpolation (CAFI) are pushing the boundaries of frame rate conversion, enabling the transformation of standard video footage, say 30 frames per second, into dramatically higher rates like 120 or even 480fps. This opens the door to remarkably smooth and fluid motion in slow-motion scenarios.
However, the pursuit of visually perfect slow motion is not without hurdles. Challenges associated with temporal aliasing, often manifesting as jitter or ghosting, and the introduction of unwanted artifacts continue to hinder the quest for entirely natural slow-motion results, especially in scenes with complex movement. The ongoing development and application of generative AI models, for instance Google's Frame Interpolation for Large Motion (FILM), suggest a continued push to refine frame interpolation algorithms. These AI approaches are being explored to produce more realistic, higher quality frames while addressing the limitations of traditional methods.
Moving forward, the integration of AI into video processing will undoubtedly continue. It's crucial, though, to carefully consider the balance between achieving exceptional performance and the associated increases in computational demands that AI methods often impose. The goal is to deliver a video experience where motion transitions are seamless and look realistic, all while being efficient enough for use in a wide range of applications.
The future of AI-powered video processing, particularly within frame interpolation, holds intriguing possibilities. We're likely to see advancements in generating frames in real-time, which could significantly impact live broadcasts and video games by dynamically increasing frame rates with minimal delay. This could drastically change how we experience immediate visual feedback.
Current methods primarily focus on spatial motion, but future algorithms could also incorporate temporal dynamics, meaning they could better understand how objects move and interact over time. This enhanced understanding should result in a more refined generation of frames, particularly in complex or fast-paced scenes.
Perhaps future interpolation algorithms will incorporate non-linear approaches to frame creation, enabling them to adapt to different speeds of motion rather than relying on a uniform prediction of movement. This could lead to even more realistic slow-motion effects, as the generated frames would more accurately reflect the nuances of motion within a scene.
There's growing interest in the intersection of frame interpolation and video compression techniques. Researchers are exploring ways to integrate these technologies to optimize video quality without a large increase in file size. This could have a substantial impact on how we store and stream video in the future, allowing for high-quality video without the need for massive storage or high bandwidth.
We can also imagine AI models that are more adaptive to user preferences. This could involve systems that learn from user feedback, essentially allowing them to identify and correct errors in real-time. Users could directly influence the frame generation process, leading to a more tailored and personalized viewing experience.
Algorithms might also become more adept at recognizing and adjusting to environmental conditions. Future systems could adapt their interpolation techniques based on factors like lighting and motion context, producing slow-motion footage that appears more natural and seamlessly integrated within varied settings.
One of the exciting potential applications is the ability to enhance the quality of older or lower-resolution video footage. Frame interpolation could be leveraged to improve older film or even poorly captured videos, making it possible to enhance their quality without needing access to high-quality original source material.
Perhaps in the future, viewers will be able to interactively control the frame rate adjustments during playback. Users might be able to experiment with different slow-motion rates, giving them a greater degree of freedom to experience and analyze scenes at different speeds. This could open up a more customized and interactive video consumption experience.
Optimizing the algorithms for parallel processing seems to be a crucial direction for future research. This could involve utilizing multi-core processors and distributed computing resources more efficiently, leading to faster and better-quality frame interpolation.
Finally, it would be beneficial to see wider cross-platform compatibility with frame interpolation technology. Currently, some implementations require expensive hardware or are limited to certain devices. Moving forward, it would be valuable to make these advanced capabilities more widely accessible on consumer-grade hardware, providing a more inclusive and readily available experience for everyone interested in exploring enhanced slow-motion video.
More Posts from whatsinmy.video: