Analyze any video with AI. Uncover insights, transcripts, and more in seconds. (Get started for free)
Top 8 Open Source Video Datasets for Computer Vision Research in 2024
Top 8 Open Source Video Datasets for Computer Vision Research in 2024 - KITTI Dataset Enhances Autonomous Driving Research
The KITTI dataset plays a central role in advancing autonomous driving research by providing a rich collection of data from real-world driving situations in Karlsruhe, Germany. It captures diverse traffic scenarios using a variety of sensors, including high-resolution cameras and 3D laser scanners. This multi-sensor approach allows researchers to tackle challenging tasks like stereo vision, optical flow estimation, and 3D object recognition. Furthermore, the dataset's detailed annotations, including object bounding boxes, make it ideal for training and evaluating algorithms for tasks such as object detection and tracking.
KITTI's inclusion of high-accuracy GPS and IMU data makes it invaluable for evaluating the performance of navigation and localization systems in autonomous vehicles. The dataset's influence has extended to promoting innovation in areas like real-time processing and depth estimation. While new datasets for autonomous driving have emerged, KITTI continues to be a benchmark for evaluating algorithms and remains a crucial resource in pushing the boundaries of research in mobile robotics and computer vision.
The KITTI dataset, a product of the Karlsruhe Institute of Technology and Toyota Technological Institute, has become a cornerstone for autonomous driving research due to its rich collection of traffic scenarios captured in and around Karlsruhe, Germany. It employs a multi-sensor approach, utilizing high-resolution cameras and a 3D laser scanner to capture detailed data. This allows researchers to tackle a wide range of challenges within the field, including stereo vision, motion estimation (optical flow and visual odometry), object detection and tracking in 3D space.
One of the key features is its detailed annotation system. Bounding boxes meticulously outline objects in each frame, enabling researchers to train and evaluate algorithms for tasks like object detection with a substantial training set of 7481 images. Additionally, precise GPS and inertial measurement unit (IMU) data are incorporated, providing crucial information for algorithms that tackle navigation and vehicle positioning.
The KITTI dataset has gained broad acceptance within the computer vision community, establishing itself as a benchmark against which other datasets and algorithms are assessed. This has fostered healthy competition, as exemplified by the emergence of other prominent datasets like Audi's A2D2. It's notable that KITTI has sparked significant advancements in areas such as 3D perception and real-time processing for autonomous driving. This has been fueled by its influence on the development of new algorithms, which have pushed forward the capabilities of autonomous driving technologies.
The KITTI Vision Benchmark Suite remains a significant force in propelling innovation in areas like mobile robotics and computer vision. It acts as a catalyst for future research endeavors in these fields, highlighting the enduring relevance of this valuable resource. However, it's important to acknowledge that, despite its impact, the field is continuously evolving with newer datasets addressing specialized areas and the growing complexity of autonomous driving research.
Top 8 Open Source Video Datasets for Computer Vision Research in 2024 - CIFAR Datasets Provide 60,000 Labeled Images for Classification Tasks
The CIFAR datasets, primarily CIFAR-10 and CIFAR-100, provide a valuable starting point for computer vision research, offering a collection of 60,000 labeled images. These images are categorized into 10 classes in CIFAR-10 and expanded to 100 classes in CIFAR-100. Each class has 6,000 images, making them a suitable size for training machine learning models, especially CNNs. The datasets are comprised of 32x32 pixel images depicting common objects, making them versatile for various classification tasks.
While the CIFAR datasets have played a significant role in establishing foundational knowledge in computer vision, researchers should be aware of their limitations. The image resolution and diversity within the datasets may not be sufficient for more contemporary challenges. The images are quite small and the variety of objects is not extremely complex. Despite these limitations, the datasets remain readily available and serve as a beneficial starting point, particularly for those beginning their research journey into image classification. The accessibility of CIFAR datasets through platforms like TensorFlow Datasets contributes to their continued relevance in the research community.
The CIFAR datasets, specifically CIFAR-10 and CIFAR-100, offer a valuable resource for researchers exploring image classification tasks. CIFAR-10, in particular, provides a manageable set of 60,000 labeled images, neatly divided into 10 categories with 6,000 images per class. Each image is a relatively small 32x32 pixels, making them ideal for initial experiments in the field. These datasets are derived from the 80 million tiny images dataset, a project spearheaded by Alex Krizhevsky, Vinod Nair, and Geoffrey Hinton.
Their popularity stems from their accessibility and wide use within the machine learning community. Researchers leverage them to compare and contrast different algorithms, particularly convolutional neural networks (CNNs), in basic visual recognition challenges. This ease of access and use has established them as a standard benchmark in the field. CIFAR-100 presents a more complex challenge with its 100 classes, each containing 600 images. It also introduces a hierarchical class structure (superclasses), presenting a useful testbed for exploring fine-grained classification.
One of the benefits of these datasets is their ability to facilitate quick experimentation and model development. Their small size allows researchers to quickly iterate and test new approaches. However, it's important to remember that they are rather simplistic compared to the growing complexity of real-world visual data. Researchers often find that achieving peak performance can be challenging due to issues like overfitting, a consequence of model complexity exceeding the limited data available. Despite these limitations, the CIFAR datasets have been essential for demonstrating the potential of CNNs for image classification.
Beyond basic classification, CIFAR datasets have become integral to research on transfer learning, where a pre-trained model is adapted to new tasks. This highlights their value in understanding model generalization across various image classification problems. Moreover, the small image size makes these datasets useful for studying adversarial attacks. Because smaller images can be easier to manipulate for malicious purposes, it's a good test environment for developing more resilient models against adversarial perturbations. These datasets foster a vibrant research community. Researchers regularly publish results on benchmark leaderboards, driving innovation and progress in image classification techniques.
Ultimately, the CIFAR datasets are a cornerstone of computer vision research, particularly for newcomers to the field. They provide an excellent starting point for experimenting with various approaches to image classification and understanding the strengths and limitations of current algorithms. Although the field is constantly developing, these foundational datasets continue to serve as a benchmark for developing and evaluating new methods and advancing research in the field. They are a useful stepping stone for researchers to refine their skills before moving on to more complex, and sometimes less accessible, datasets.
Top 8 Open Source Video Datasets for Computer Vision Research in 2024 - VideoPrism Tackles Multiple Video Understanding Challenges
VideoPrism is designed as a general-purpose video understanding model, capable of tackling a wide array of tasks, including video classification, object localization, information retrieval, automatic captioning, and answering questions related to the video's content. The model benefits from a comprehensive training process using a massive collection of 36 million high-quality video and text pairs, coupled with 582 million video clips containing diverse, sometimes automatically generated, text. VideoPrism employs a specialized pretraining method that refines semantic video information through innovative techniques. Notably, it excels at applying learned knowledge to unseen video types, even surpassing models specifically tailored to specialized fields like science. This versatility is demonstrated by its superior performance across 31 out of 33 widely used benchmarks compared to other video foundation models. While it shows promise, it's important to remember that it's a general-purpose solution and its effectiveness may depend on the specific context of the video data and the particular task. It's a single, pre-trained model design, making it simpler to use than multiple different video models for different tasks. Its success across different datasets in various application domains like neuroscience and ecology suggests that it could be useful for various kinds of video understanding problems.
VideoPrism is a versatile video encoder built for a wide array of video understanding tasks, like classifying what's happening, finding specific objects, searching for videos, generating descriptions, and answering questions about the video content. It's been trained on a massive and diverse set of data: 36 million high-quality video-caption pairs and another 582 million video clips that often have noisy or machine-generated text related to them. This broad training approach is interesting, since it includes a mix of curated and less-structured information, which could potentially lead to more robust models.
VideoPrism uses a training method that expands on a standard technique called masked autoencoding. They've introduced some tweaks like distilling semantic video information in a global and local way, alongside a token shuffling scheme. This novel approach appears to have boosted performance in general.
One thing that stands out is that VideoPrism demonstrates good generalization capabilities across various areas, and it seems to outperform specialized models built for specific fields, especially within AI4Science applications. Researchers are hopeful this indicates a potential for broader applicability of video processing. It has performed very well on a bunch of video understanding benchmarks, achieving state-of-the-art results on over 30 out of 33 common benchmark tests. It's clear from these results that it's competitive with current foundation models in the space.
Its usefulness has been confirmed across several standard computer vision datasets and even in more specialized domains like neuroscience and ecology. This shows promise for VideoPrism to be applicable in various research areas. The way it works is that it primarily focuses on the visual aspect of a video, but it also leverages related text information to help improve the learning process. This multi-modal approach is common but it's interesting that they primarily focus on video understanding while still integrating this supplemental textual information.
The wide variety of training data is an advantage, as it includes a good mix of high-quality video-caption data, plus a significant amount of more varied video clips. It's plausible that this helps make the training more robust, but this might also be a challenge as the noise level and quality variation in the training data could hinder accuracy. VideoPrism is dubbed a "Video Foundation Model," suggesting that it's intended as a basic building block for general-purpose video understanding. It simplifies a lot of video tasks because it can act as a single, frozen model architecture—meaning you don't need multiple, specialized models. This has benefits in streamlining research and potentially makes the process easier to start with, but we need to consider that this 'one size fits all' approach might not be optimal for very specialized domains.
Top 8 Open Source Video Datasets for Computer Vision Research in 2024 - Google's CVPR 2024 Paper Generates Looping Videos from Single Images
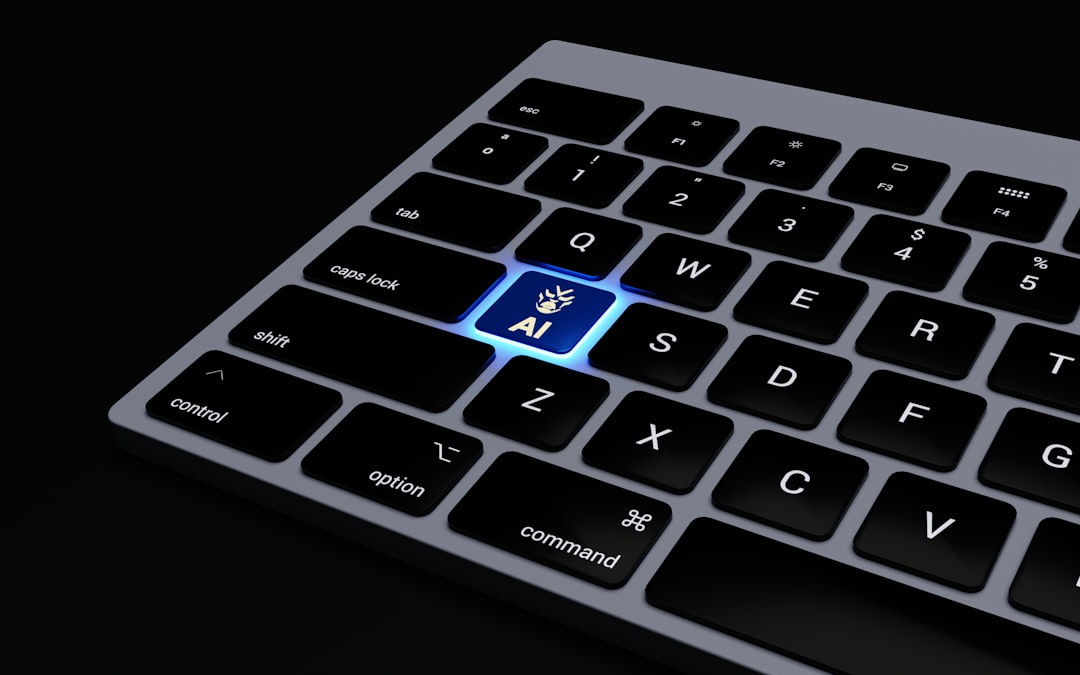
At the recent CVPR 2024 conference, Google researchers presented a paper that details a method for creating continuous looping videos from just a single image. This work, highlighted by the conference's best paper award, was led by Zhengqi Li and Richard Tucker. The researchers trained a model on how things move in actual video clips to capture natural movements like swaying trees or fluttering flowers. The model uses a special technique to predict the motion of all the pixels in the image, essentially generating movement from a still image.
This is a significant step forward in video generation. Not only is it interesting for artistic reasons, but it also hints at a range of potential uses in computer vision and related fields. The CVPR 2024 conference featured a lot of discussion around generative AI and how to better understand the content of videos, and this Google research is a strong example of these growing trends. It will be interesting to see how this work impacts future research on generating and interpreting video.
At CVPR 2024, Google researchers presented a paper that tackles a fascinating problem: generating seamless looping videos from a single image. It's a challenge because standard methods for creating videos rely on sequences of frames, not just one static snapshot. They managed to train a model on a large collection of real-world video sequences, focusing on how objects move within those clips, and apply this learned knowledge to create the illusion of motion in a still picture.
This is a notable achievement because it essentially tries to figure out the natural, repetitive movements that objects like trees or flowers might exhibit. It's like having the model learn from a wide variety of video examples of such motions, then applying what it's learned to infer and recreate those movements in a new still image. It's likely they used some combination of techniques like GANs or RNNs to generate the video sequences. The paper itself is noteworthy, being among the best presented at CVPR 2024, a highly respected conference in computer vision.
While the research is promising for areas like gaming or augmented reality, where you might need to add a little motion to a static image, it's likely that there are limits to its capabilities. I imagine they might struggle with extremely complex images that have a lot of intricate details and non-repeating movements. Still, it's very clever that they can leverage learned motion dynamics from a huge library of video sequences to effectively generate these videos. This sort of generative approach can influence how we think about creating and editing video content, possibly making it easier to enrich still photographs with motion in a way that hasn't been easily achievable in the past.
The computational efficiency of the model is also interesting. Initial indications are that the system is fast enough to create these looping videos in real-time, which has implications for applications like interactive filters on social media platforms. It's exciting to see the potential for creators to have tools to instantly convert static images into dynamic visual content. While this approach is currently in its early stages, it suggests a shift in the way we might create video content and also raises intriguing questions for future research on understanding motion itself. It pushes the boundaries of what we can infer about motion simply by looking at a single, motionless picture. However, as is often the case with these very advanced AI approaches, it's likely that the model will have limitations when faced with extremely complex and diverse visual scenes. Nevertheless, this model, at the very least, hints at a potential disruption in the world of video creation and digital art.
Top 8 Open Source Video Datasets for Computer Vision Research in 2024 - DINOv2 Advances Self-Supervised Training in Computer Vision
DINOv2, a self-supervised learning method created by Meta AI, significantly advances computer vision by enabling the extraction of strong visual features without needing to fine-tune the model. This makes it a powerful and flexible foundation for a variety of computer vision tasks, including image recognition and video analysis. A key advantage of DINOv2 is its capacity to learn from diverse sets of images, making it adaptable to a wide range of applications across different fields. Furthermore, DINOv2 has outperformed other self-supervised learning methods in a number of tests, successfully addressing the limitations of previous approaches that were often trained using smaller and less diverse datasets. Because the model and its associated code are available as open source, DINOv2 can be readily used and further developed by the computer vision research community. This accessibility potentially leads to wider adoption and innovation in both research and real-world uses of computer vision.
DINOv2, developed by Meta AI, is a self-supervised learning method designed for training high-performance computer vision models. This approach avoids the need for large labeled datasets, which can be quite a limitation in many areas of computer vision. Instead, it leverages unlabeled images to extract robust visual features. This shift in approach makes it potentially more accessible to a wider range of researchers since they don't need to spend large amounts of time gathering large labeled datasets.
One of the key advantages of DINOv2 is its ability to achieve strong performance without requiring further fine-tuning on new datasets. It's like it learns a general understanding of visuals that then readily applies to new tasks or domains. This is really powerful because it means the trained model can serve as a foundational component for a variety of computer vision problems, potentially simplifying the workload of building systems. Its design allows it to learn effectively from virtually any collection of images. It seems like its framework has a natural ability to adapt to different kinds of image datasets and domains.
The resulting visual features produced by DINOv2 are quite powerful. It's demonstrated through its use in a wide range of applications, from image recognition and retrieval to complex video tasks like motion and scene understanding. The results from DINOv2 appear to be very competitive in several areas, as it's been shown to achieve top results when compared with other self-supervised and weakly-supervised techniques. Notably, it tackles a key limitation of prior methods, namely their tendency to be heavily reliant on smaller and less diverse datasets like ImageNet.
Instead, DINOv2 excels in developing a generalized understanding of images and visual patterns. This means that researchers can largely use the same core model architecture for a variety of downstream applications, customizing only the decoders to fit specific tasks. It's an efficient and arguably easier-to-use approach. This training approach provides consistent and high-quality visual features across different tasks. While the research appears very promising, it will be interesting to see how well it performs as we progress with more specialized video datasets and potentially more complex use cases.
The model's source code and pre-trained models are available on GitHub using PyTorch. This open access approach supports the community's efforts to explore and expand upon the capabilities of DINOv2. Recent work indicates that DINOv2's performance can rival or even surpass that of conventionally trained models. This observation is certainly intriguing and perhaps indicative of a broader shift towards self-supervised learning within computer vision. It's still early days for DINOv2, and the broader community's efforts to adopt and improve upon the model will ultimately shape its impact on the field.
Top 8 Open Source Video Datasets for Computer Vision Research in 2024 - GitHub Hosts Over 420 Million Computer Vision Projects
GitHub has become a central hub for computer vision, currently hosting a massive collection of over 420 million projects. This makes it a prime location for researchers and developers to share, improve, and collaborate on various aspects of computer vision. These projects encompass a broad range of applications, from basic image processing to more complex tasks like recognizing objects in real-time. While this wealth of projects fosters collaboration, it also presents challenges, particularly in terms of discerning high-quality contributions amid a large number of projects. The dominance of GitHub in the field also underscores the increasing interest in computer vision techniques and highlights the ongoing development and refinement of both existing datasets and novel research efforts. The importance of exploring open source video datasets in 2024 continues to be crucial in advancing the applications of computer vision and pushing the boundaries of related research endeavors.
GitHub has become a central repository for over 420 million computer vision projects, reflecting the rapid growth of the field and the widespread collaboration among developers and researchers. This sheer volume hints at how computer vision is increasingly being integrated across various industries, from self-driving cars to medical imaging.
The diversity of projects on GitHub reveals a wide range of innovative applications, including everything from facial recognition to automated surveillance systems. This breadth demonstrates the potential of computer vision to address real-world problems, but it also raises critical questions about privacy and potential misuse.
Many of these projects utilize deep learning techniques, specifically convolutional neural networks (CNNs), which have shown impressive results in tasks like image classification and object detection. This success has led to a noticeable trend: developers often prioritize fine-tuning existing models rather than designing entirely new ones.
The expanding open-source software landscape within computer vision allows researchers to build upon existing code, potentially accelerating development and experimentation. While helpful, this can also lead to stagnation, with many projects repeating similar algorithms without proposing genuinely novel methods.
GitHub's built-in version control facilitates collaboration by allowing multiple contributors to work on complex computer vision projects concurrently. However, managing these contributions effectively can be challenging, as ensuring coherence and preventing fragmentation becomes increasingly difficult as projects grow.
The quality of documentation across GitHub repositories is uneven. Some are exceptionally well-structured, containing detailed explanations, while others fall short, creating hurdles for those new to the field seeking to grasp modern computer vision techniques.
A notable trend is the incorporation of pre-trained models in many GitHub projects. This allows developers to achieve high accuracy without needing enormous training datasets. However, this reliance on transfer learning can obscure the need for robust, domain-specific training data, leading to potential biases in results when applied to new or unique data.
The sheer number of computer vision projects raises concerns regarding reproducibility. Many projects lack clear instructions for replication, highlighting a potential disconnect between cutting-edge research and reliable results due to inconsistent experimental methods.
Developers often encounter challenges related to hardware limitations when working with complex computer vision algorithms, as these tasks can be computationally demanding. This has spurred the creation of lightweight models designed to bring advanced computer vision capabilities to mobile devices and edge computing environments.
Finally, with a growing number of projects addressing ethical AI and fairness in computer vision, GitHub is evolving into a space for not only technical knowledge but also a forum for discussion about the wider societal implications of image recognition technologies. This signifies a welcome, more thoughtful approach to the field's ongoing advancement.
Top 8 Open Source Video Datasets for Computer Vision Research in 2024 - AI-Assisted Tools Speed Up Video Annotation Process
AI-powered tools are significantly accelerating the process of annotating videos, potentially boosting efficiency by up to ten times. This is a welcome development, as manual annotation can be a slow and labor-intensive task. These tools aim to streamline the process, enabling researchers to spend less time labeling and more time on other critical research aspects. There's a growing variety of annotation tools available, some of which, like Label Studio and CVAT, are particularly well-regarded for their intuitive interfaces and advanced features. However, the user experience with these tools can vary. While many users praise the ease of use, others highlight challenges, especially with more complex systems. This trend towards automated or AI-assisted annotation is not just about productivity. It also opens doors to more thoroughly annotated and larger video datasets, which is crucial for developing and evaluating increasingly sophisticated computer vision models across a wider array of applications. Given the rapid expansion of publicly available video datasets, these tools are becoming indispensable for driving forward research in this ever-evolving field.
AI-powered tools are revolutionizing the video annotation process, potentially boosting efficiency by a factor of ten. This significant speed-up could be a game-changer for researchers, allowing them to spend more time on the insightful part of their work—analyzing the data—rather than the painstaking task of manually labeling each frame.
Many of these tools utilize advanced algorithms, including convolutional neural networks (CNNs), to automatically pinpoint and label objects within video frames. This automated labeling is particularly beneficial for massive datasets where the risk of human error during manual annotation can be substantial.
A noteworthy aspect of these AI-assisted annotation systems is their capacity for continuous learning. They refine their accuracy and speed through iterative processes, incorporating feedback from human annotators to continuously enhance their algorithms.
Research suggests that AI-assisted annotation can achieve accuracy levels comparable to expert human annotators, underlining the value of these tools in projects that rely on precise object detection and tracking. For example, some AI annotation systems are capable of handling intricate scenarios, such as tracking objects across multiple frames in dynamic environments, making them ideal for domains like autonomous driving where precise object tracking is critical.
While these tools streamline the annotation process and boost accuracy, they also come with certain limitations. Ambiguous situations where object boundaries are unclear still pose challenges. Researchers need to exercise caution and avoid relying solely on AI-generated annotations in such cases.
Many of these AI-powered tools provide intuitive user interfaces, enabling annotators to easily review and modify automated labels. This interactive approach is essential for maintaining high dataset quality, combining the efficiency of automated tools with the vital layer of human oversight.
Furthermore, the integration of AI into video annotation promises a substantial decrease in labeling expenses. For projects involving extensive manual labeling, this cost reduction can significantly improve feasibility, especially for large-scale research initiatives.
However, it's crucial to be mindful of the potential for biases introduced by the training datasets used to develop these tools. Using datasets that aren't fully representative can perpetuate inaccuracies, emphasizing the importance of careful curation in building robust and unbiased datasets.
The ongoing advancement of AI-assisted video annotation is reshaping the landscape of video data processing. It not only accelerates the annotation process but also has the potential to significantly broaden the scope of computer vision research by enabling the creation of far larger and more diverse datasets than was previously feasible. This suggests that the possibilities for computer vision research may increase substantially as a result of this technological development.
Top 8 Open Source Video Datasets for Computer Vision Research in 2024 - Diverse Datasets Fuel Computer Vision Innovation in 2024
The availability of diverse datasets is a major catalyst for innovation within computer vision in 2024. Researchers are increasingly leveraging these resources to train more powerful and adaptable models across a wider array of applications. While foundational datasets like KITTI and CIFAR have established a strong baseline for understanding, newer datasets like VideoPrism are pushing the frontiers of video analysis and comprehension. The emergence of self-supervised methods like DINOv2 also emphasizes the value of broad datasets, as it demonstrates that strong visual features can be extracted from unlabeled data. Essentially, the greater variety and specific nature of these datasets become crucial for addressing the increasing complexity of computer vision challenges and ensuring the models are ready for real-world problems. This reliance on dataset diversity will likely become even more important as the field continues to evolve and demand a more nuanced understanding of the visual world.
The increasing availability of diverse datasets is a key driver of innovation in computer vision throughout 2024. The ability to train models on a wider variety of visual data has shown a significant impact on model robustness and accuracy. For example, we're seeing improved resilience to attacks where models are tricked into making wrong predictions when trained on more diverse data. This increased diversity also seems to help with a new generation of few-shot learning approaches, allowing models to perform well even with limited data. This is useful for many real-world situations where collecting large amounts of training data is difficult.
Another area where diverse datasets are proving essential is in self-supervised and unsupervised learning methods. With the ability to learn from massive amounts of unlabeled data, we're potentially seeing a reduction in the need for laborious manual annotation of data. A related trend is the generation of synthetic data, which helps address a limitation with real-world datasets. We can use generative models to create datasets of specific types of objects or scenes, and this can be helpful for training specialized models in areas like robotics where we might need models that learn about unique environments or circumstances.
The temporal aspect of video data, especially in tasks like action recognition, has seen progress thanks to specialized architectures trained on datasets containing different kinds of actions and events. This requires datasets that capture the details of motion over time, and that is becoming more achievable thanks to increased availability of video data from a variety of sources. In addition, we're seeing increasing use of hierarchical labels within datasets. This is a great step because it lets us capture more detailed information about a scene, which could potentially boost the performance of models that need to handle more complex image classification tasks.
Multi-modal learning, which combines visual, textual, and audio data, is another area showing progress. This approach makes sense intuitively because humans often learn about the world by associating what we see with the sounds we hear and the things that we read or write about. We're seeing the adoption of this kind of model architecture, and this is leading to better understanding of video content.
Ultimately, diverse datasets are allowing computer vision to move beyond academic exercises. They're facilitating real-world applications in areas like healthcare, transportation, and robotics. As computer vision models are trained on datasets that capture the unique characteristics of different domains, it allows them to better generalize and solve problems in a wider range of environments. The open access trend among many of the key datasets encourages collaboration and the sharing of valuable insights and best practices. This accelerates innovation and helps to improve the quality of models in the community.
Despite all the potential benefits of diverse datasets, it's essential to acknowledge the potential issues surrounding bias in these datasets. Models can easily inherit biases present in the data they're trained on. This is a concern that needs to be carefully considered in computer vision research. Researchers need to be cognizant of this potential problem and actively work to mitigate bias in the models and datasets that are being used. It's a critical issue that will have a significant impact on how these techniques are used in practice.
Analyze any video with AI. Uncover insights, transcripts, and more in seconds. (Get started for free)
More Posts from whatsinmy.video: