7 Essential Datasets for Linear Regression Analysis in Video Content Optimization
7 Essential Datasets for Linear Regression Analysis in Video Content Optimization - View Duration Analysis Using the YouTube Dataset
Analyzing YouTube view duration offers a window into how viewers interact with online video content. This analysis typically relies on datasets like those available through the YouTube API, including video and channel statistics, alongside publicly available sources like Kaggle's Top US Trending Videos. A key metric is average view duration, which essentially represents the total time spent watching a video divided by the number of views. Understanding the factors that influence view duration is crucial. Studies suggest that metrics like the ratio of likes to views can be indicative of how long viewers engage with a video.
However, pinpointing the exact causes of longer view durations isn't straightforward. The wide variety of viewer interests and the absence of universally accepted video categorization systems create hurdles for comprehensive analysis. Despite these challenges, employing techniques like linear regression and even more complex models can help optimize video content. These tools can enhance understanding of what makes videos more successful and how to improve their visibility to viewers. As video consumption remains a dominant online activity, understanding the factors that contribute to viewer engagement is increasingly important for creators and those involved in video optimization.
Examining view duration within the YouTube dataset offers insights into user behavior and how it relates to video performance. Researchers can leverage datasets like those found on Kaggle and through the YouTube API, focusing on statistics like view counts, watch time, and channel metrics. Calculating average view duration, a common approach, involves taking the total watch time for a video and dividing it by the total views. This allows for a more nuanced view of engagement than just the number of views.
Interestingly, research shows that metrics like likes and comments can be associated with view durations. Videos with a higher ratio of likes to views often exhibit better retention, suggesting that audience sentiment and initial reactions might play a role in how long someone watches. However, the sheer variety of viewer tastes and video topics presents difficulties in neatly categorizing and understanding video preferences.
To unravel this complexity, researchers employ methods like linear regression and neural networks, aiming to understand how factors like video content and format influence watch times. The goal is to develop models that predict video performance and optimize content for greater engagement. It's important to note that video watching is a dynamic and prevalent online activity, making continuous analysis and adaptation necessary for creators and marketers.
Beyond general engagement, researchers delve into specific elements like video summarization. Deep learning techniques show potential for distilling key information from longer videos, perhaps making them more accessible and improving retention. Overall, the link between video popularity and how much of it people actually watch highlights that understanding viewer behavior is key to optimize video content. If creators can understand why people choose to watch a video and how long they stay, then perhaps they can create videos that are more effective and engaging, thereby capturing a larger audience.
7 Essential Datasets for Linear Regression Analysis in Video Content Optimization - Engagement Metrics from TikTok's Short Video Database
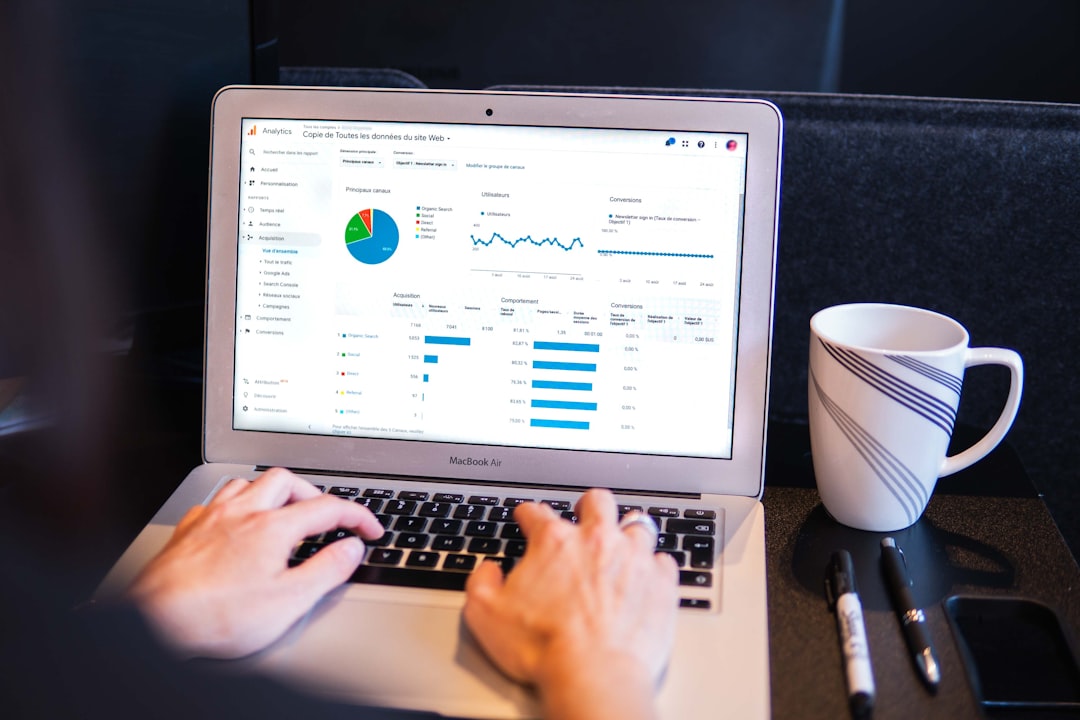
TikTok's short-form video database offers a unique lens into user engagement, especially given the platform's emphasis on videos under a minute. The platform's algorithm significantly influences how users encounter content, creating highly personalized viewing experiences. This algorithmic influence has reshaped how we understand video consumption and engagement.
Understanding audience interactions with these short videos requires examining specific engagement metrics like likes, comments, and shares. These metrics, alongside the overall sentiment expressed toward a video, give us a better grasp of audience preferences. Differences in engagement patterns can be observed based on the video's content type. For example, videos created by news outlets might see engagement patterns that differ from those produced by individual creators.
However, the current state of research on user engagement within the TikTok environment is still limited. There is much that we do not understand. Understanding the relationship between these engagement metrics and the platform's recommendation systems remains an ongoing challenge. Nevertheless, leveraging the available data on video engagement can shed light on how to optimize content to achieve greater reach and user interaction within the dynamic TikTok landscape.
### Engagement Metrics from TikTok's Short Video Database
1. **Short but Sweet, Yet Highly Engaging**: TikTok's focus on short-form videos, typically under a minute, doesn't seem to deter users. In fact, people spend a considerable amount of time on the platform daily, highlighting the power of this format to keep viewers hooked. This begs the question of what exactly makes these short videos so captivating.
2. **The Algorithm's Guiding Hand**: TikTok's recommendation engine plays a major role in what users see, which is not surprising given that a large portion of user interaction stems from the "For You" page. Understanding how this algorithm works and which factors it prioritizes is essential for creators seeking to optimize their content for maximum exposure.
3. **Emotions Drive Engagement**: It appears that videos evoking strong emotional reactions tend to be more successful in capturing user attention. Whether it's joy, surprise, or even laughter, it seems that emotionally resonant storytelling can significantly boost viewer retention. It would be interesting to further study which specific emotions are most effective on this platform.
4. **Hashtags Matter**: The use of relevant hashtags appears to be a powerful tool for increasing the visibility and engagement of videos. There's some evidence suggesting that including popular hashtags can significantly boost interactions, making it a valuable strategy for content creators to stay informed on trending topics and effectively categorize their content.
5. **Soundtracks and Engagement**: It's intriguing to see that leveraging popular audio tracks can lead to higher engagement. A large percentage of users interact with videos using trending sounds, making it evident that aligning content with popular audio can be a strategic approach. It's important to understand, though, whether this is due to the sound itself or the type of content typically associated with that sound.
6. **Visual Impact on Retention**: Certain visual elements, like bright colors and dynamic movements, are connected to increased completion rates. Researchers have observed that videos with engaging visuals have lower drop-off rates as viewers progress. Understanding the specific combination of visual elements that resonate most with users will be vital for optimizing video design.
7. **The Power of User-Generated Content**: Studies show that user-generated content seems to outperform branded content in terms of engagement. This suggests that authenticity and genuine interactions might be more effective in connecting with audiences than traditional advertising approaches. Further research is needed to delve into the specific characteristics of this content that make it more appealing.
8. **Engagement Varies Across Demographics**: The types of videos that resonate with users appear to be influenced by their age and potentially other demographic factors. This highlights the need for content creators to tailor their content to specific audiences for maximum impact. It would be beneficial to conduct detailed research into the preferences of different demographics within the TikTok ecosystem.
9. **Optimizing Video Length for Retention**: Interestingly, researchers have found that keeping video lengths concise tends to yield better retention rates. This implies that a clear and focused message delivered within a shorter timeframe can lead to more engaged viewership. More research into the optimal lengths for different content formats will further refine this understanding.
10. **Influencer Impact**: Videos featuring influencers are shown to drive significantly higher engagement rates than other types of videos. This underlines the role of social proof and credibility in driving viewership and provides a strong incentive for brands to collaborate with influencers to expand their reach. It is crucial to explore what aspects of influencer collaborations lead to this strong engagement.
7 Essential Datasets for Linear Regression Analysis in Video Content Optimization - Content Categorization with Netflix's Genre Classification Set
Netflix's genre classification set offers a valuable approach to content categorization, particularly within video streaming platforms. This dataset utilizes techniques like multilabel classification, where a movie can be categorized into multiple genres simultaneously, and hybrid convolutional networks, which are seen as vital for effectively recognizing film types, particularly in cloud environments. To improve model accuracy, training often involves incorporating a wide range of content, a practice aided by self-supervision methods that blend on-platform and external sources. The rise of streaming services and viewers' desire for automatically categorized content have driven the need for robust genre classification tools. While these techniques offer significant advantages, there are still areas for improvement, notably in applying multilabel classification to image and video content, suggesting an opportunity for ongoing research and development. This, in turn, can be used to refine recommendation systems and strengthen engagement by providing users with a more nuanced and personalized experience when browsing for video content.
### Surprising Facts About Content Categorization with Netflix's Genre Classification Set
1. Netflix's genre system goes beyond basic categories like "Action" or "Comedy." They've developed a very detailed approach with subgenres like "British Sci-Fi & Fantasy" or "High-Concept Action," making it possible to pinpoint viewer preferences more precisely. It's interesting to think about how effective this kind of specificity actually is, especially in how it shapes viewer habits.
2. Instead of just making up categories, Netflix bases their genre choices on viewer data. They analyze how people interact with the content and use that to predict what they'll enjoy next. This seems to be the key to how they keep people coming back, but I wonder if the biases inherent in the data get perpetuated in the classification system.
3. Netflix's genre classifications aren't set in stone. They constantly tweak the system, taking into account how people are currently interacting with content. This adaptability is probably vital to keeping up with the ever-changing landscape of viewer tastes, but it makes me wonder how stable these classifications truly are over the long term.
4. The way Netflix classifies content isn't just for finding things – it also affects what shows get surfaced in their UI. This raises a thought-provoking point: is it possible that some genres get more exposure simply because of how they're classified? Could this introduce biases in what people discover?
5. Parents use Netflix's genre system to control what their kids watch. This emphasizes that precise genre classifications are important for helping families manage what content is appropriate for different age groups. It's crucial to ensure that these classifications are accurate and consistently applied, especially considering the potentially broad range of content that fits within a specific genre.
6. What's considered a particular genre in one country may not be the same in another. This is quite insightful in terms of cultural nuances in content consumption. It highlights the challenge of developing a universally applicable genre classification system, especially in a global media landscape.
7. It seems that genre classification strongly influences how people watch shows. People spend more time watching content in genres they already like, which reinforces Netflix's targeted recommendations. It's an interesting cycle, but one that reinforces the power of these classifications to shape viewing habits, and potentially limit exploration outside of familiar genres.
8. Some genres dominate viewership thanks to how they're classified and promoted. For example, the "Documentary" genre has become more popular as more documentary content is categorized and promoted. This suggests that the classification system can significantly influence the popularity of certain genres.
9. Netflix often uses A/B testing to find the best ways to classify and recommend genres. They experiment with different options to see what works best. This suggests that their algorithms are constantly evolving and trying to get more granular in their understanding of user preferences. But this begs the question, are viewers fully aware of the experimentation that is happening?
10. The way content is classified doesn't just impact viewers; it also affects the creative process. Producers and directors look at these genre classifications to make sure they're creating content that fits the expected style and format, leading to a more predictable content pipeline. While this may streamline content production, it also raises a question about the potential for creativity to be stifled by pre-existing genre expectations.
7 Essential Datasets for Linear Regression Analysis in Video Content Optimization - Thumbnail Effectiveness Study via Twitch Stream Data
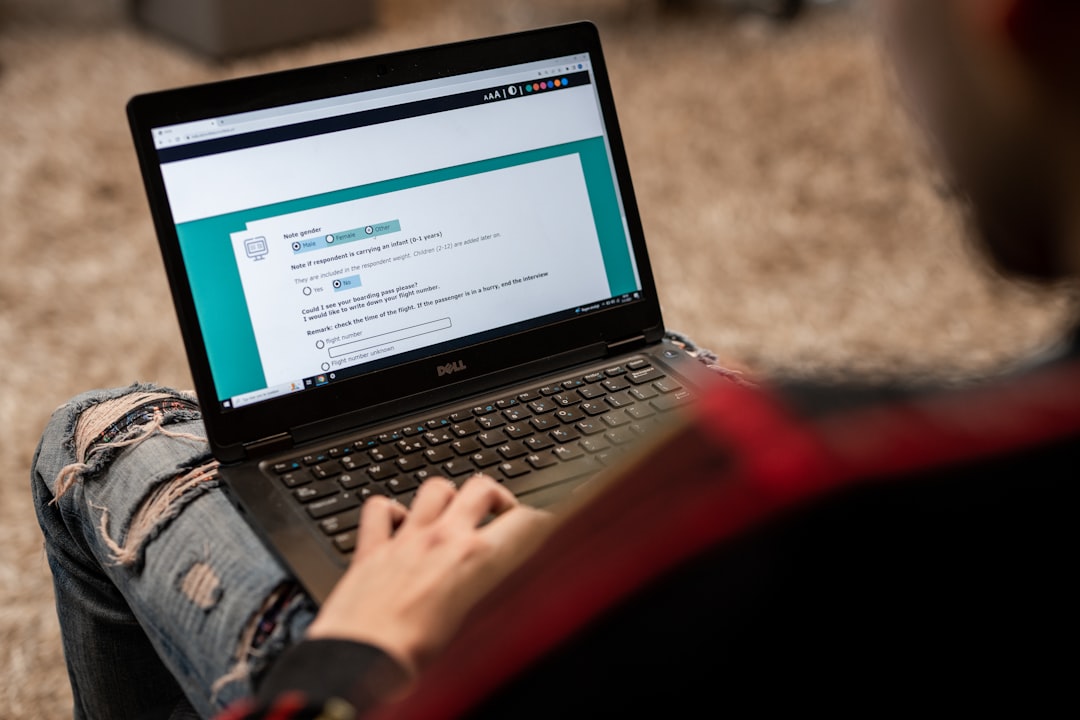
The study of thumbnail effectiveness using Twitch stream data reveals a critical, yet often overlooked, element of video optimization: the impact of visual design on viewer behavior. Most existing research on thumbnails has concentrated on automated selection techniques, rather than meticulously examining how specific visual attributes drive engagement. Considering that Twitch boasts a massive user base and billions of hours of content watched each month, this analysis gains significant relevance. The study suggests that employing linear regression to examine Twitch data can unveil the subtle relationship between thumbnail design and viewer interaction, like watch time and engagement. This highlights a possible need for creators to adopt a more strategic and thoughtful approach to designing thumbnails that resonate with their viewers. As video consumption continues to increase and dominate online interaction, this area of research offers valuable insights for creators seeking to enhance their audience reach and engagement.
### Surprising Facts About Thumbnail Effectiveness Study via Twitch Stream Data
1. Most studies on thumbnails have focused on automated selection methods rather than on the specific visual elements that make them effective. This is a missed opportunity to understand the underlying principles of visual attention in a streaming environment.
2. Twitch is a huge platform, with millions of unique users and billions of hours of content consumed each month. This suggests a large potential audience, but also a very competitive environment where standing out visually is crucial.
3. Twitch's user base mainly watches video game content, indicating that thumbnails within this sphere might have unique features that drive clicks and viewership. Understanding what those features are could benefit creators in this domain.
4. Although YouTube is another massive platform with a broader range of content, Twitch's data offers a more focused view on the impact of thumbnails in the context of video games and live streaming. Comparing results from these platforms would be fascinating.
5. Video traffic continues to grow and is projected to dominate online consumption. This highlights the need for a thorough understanding of factors that influence viewer engagement, including, and perhaps especially, the visual cues that attract attention.
6. While tools like graphic design software are crucial for creating professional thumbnails, using a simple still image is often a bad choice. This suggests that thoughtful planning and the application of visual design principles are needed for effective thumbnails, regardless of platform.
7. The study found that users watched over 12 billion hours of content on Twitch in just one month. This highlights the platform's power and the importance of understanding the content consumed on Twitch specifically, to determine if video game streaming differs in how viewers engage with thumbnails.
8. Some of Twitch's top streamers have become significant personalities in popular culture. This indicates that streamers and their content can reach very broad audiences, potentially suggesting that thumbnail quality is linked to a streamer's growth in popularity.
9. Linear regression analysis is a valuable tool for studying how thumbnails impact viewer engagement. Using this technique on datasets of Twitch viewing data could reveal which visual attributes have the biggest influence on click-through rates and other engagement metrics.
10. Finding and using appropriate datasets is crucial for effective analysis and optimization. Twitch's data, in combination with other data on streaming behavior and demographics, could be invaluable for researchers looking to develop guidelines for more effective thumbnail creation.
7 Essential Datasets for Linear Regression Analysis in Video Content Optimization - Audience Retention Patterns in Facebook Video Analytics
Analyzing how viewers interact with Facebook videos, specifically how long they stay engaged, is crucial for improving content. Facebook offers detailed analytics that show, minute by minute, how many viewers are still watching. This allows creators to spot when viewers tend to stop watching, pinpointing parts of the video that might need improvement. It's become increasingly important to understand retention patterns, especially as more people share longer videos on Facebook. The data helps understand what parts of the video hold viewers' attention and what causes them to drop off. This insight can be used to improve content for future videos. It's not just about how long people watch, though. Other important metrics, like likes, comments, and even negative feedback, also reveal important details about viewer preferences and overall video effectiveness. By examining both the length of time viewers stay and their other responses, creators can refine their video content to maximize engagement and guide viewers towards intended actions within the video.
### Surprising Facts About Audience Retention Patterns in Facebook Video Analytics
1. When viewers stop watching, it reveals a lot about what they're looking for. Facebook's video analytics, particularly the minute-by-minute breakdown, shows us exactly where people tend to drop off. These "drop-off points" become really interesting clues about how well the content is doing and what viewers expect. Keeping them engaged throughout the entire video is clearly important.
2. It seems that longer videos often struggle to hold onto viewers on Facebook. Shorter videos, on the other hand, have a better chance of keeping people watching. This hints that grabbing attention quickly is really crucial in a fast-paced environment where people are constantly scrolling through content.
3. Interactive stuff like polls or prompts to do something (calls to action) seems to make people stick around longer. It's like adding little games or prompts can get people to pay closer attention and stay engaged with the video.
4. The time of day a video is posted really matters. Audience retention changes quite a bit depending on when the video is shared. This means creators need to consider when their target viewers are most likely to be online if they want to maximize how long people watch.
5. Videos that get more comments and shares tend to hold onto viewers better. This suggests that viewers are more likely to stay engaged if they feel like they're part of a community around the content. It's almost like a sense of connection leads to a more invested audience.
6. The thumbnail—the picture that previews the video—seems like a crucial first impression. Research shows that videos with more attention-grabbing thumbnails (ones that are brighter or more active visually) often get more clicks. This shows us that the initial visual appeal has a big role in whether people decide to watch.
7. The type of content matters a lot. Videos that teach something or explain how to do something seem to do better at keeping people watching than just entertainment videos. This suggests that viewers are often looking for specific types of value from their videos – information versus just being entertained.
8. Videos with influencers in them often see better audience retention compared to brand-only videos. This might be because viewers are drawn to people they know or like. It's like having someone familiar helps people feel a stronger connection and get more invested in the content.
9. How long people stay engaged with a video differs across age groups. It seems that younger viewers tend to drop off more quickly than older ones. Understanding this kind of difference can help creators tailor their content in more meaningful ways for specific age groups.
10. It's really interesting how important it is to have consistent branding throughout a video. Videos with a strong visual style, a specific tone of voice, and overall theme often see better retention. This consistency seems to help viewers recognize the content quickly and builds a stronger sense of familiarity and association with the brand, leading to improved retention.
7 Essential Datasets for Linear Regression Analysis in Video Content Optimization - Audio Quality Impact Assessment through Spotify's Music Features
Examining how audio characteristics influence a song's popularity on Spotify offers a detailed view into listener preferences. Researchers leverage Spotify's audio features, including energy, danceability, and emotional tone (valence), to analyze their impact on a track's popularity using linear regression models. This approach helps uncover which features are most closely associated with success in different music genres, such as pop, rock, rap, and dance-pop. The Million Playlist Dataset, a valuable resource containing information about millions of playlists, provides a foundation for understanding larger music trends and feature associations.
Interestingly, some audio features like danceability were found to be statistically significant predictors of success, while others had little impact, suggesting that listener preferences are diverse and not easily predicted solely by audio features. These findings can provide valuable insights for musicians and producers who are trying to improve their music's appeal. Although the research suggests that there is a connection between certain audio features and song popularity, it also highlights the limitations of predicting listener response with certainty. Understanding how these complex relationships function is vital for navigating the constantly evolving musical landscape where listener engagement is crucial.
### Surprising Facts About Audio Quality Impact Assessment through Spotify's Music Features
1. While most people can't hear a difference between audio sampled at 44.1 kHz and higher, many use services like Spotify, which offer 320 kbps Ogg Vorbis for premium subscribers. This raises interesting questions about how people perceive the quality of sound when listening to music through platforms like Spotify.
2. Spotify's loudness normalization feature aims to keep the volume of music consistent across tracks. It's surprising, however, that this can lead to a less desirable listening experience, as individuals may be accustomed to the natural volume fluctuations in a song rather than a constant, flattened volume.
3. We might expect that higher bitrates always mean higher quality sound, but it turns out that people often care more about how music makes them feel or if they're familiar with the song. This seems to challenge the idea that technical specifications alone define audio quality.
4. Spotify's algorithms often create playlists based on musical features like tempo and key. Interestingly, these recommendations can have a big impact on what people listen to. It seems like algorithms may be encouraging us to listen to music that aligns with what we've previously indicated we enjoy, potentially overriding the importance of the audio quality itself.
5. When we decide if a song has good sound quality, it's not just about the technical aspects. There are psychological factors at play. For example, we often like songs that are associated with significant memories or emotions, which can strongly influence our perception of the audio quality regardless of the objective details.
6. Features like Spotify's "Canvas," which presents short, looping videos with the music, can be distracting when trying to judge how good the audio actually is. This highlights how visuals can impact our assessment of music quality and enjoyment.
7. The same song can sound very different depending on the device it's played on. Comparing a track played on high-end headphones versus the speaker of a standard phone reveals just how much the context of playback influences our experience of audio quality.
8. It's possible that music specifically designed to trigger certain emotions could be rated as higher quality. This suggests that a strong emotional response can really affect how we perceive a track, potentially skewing our idea of "good" audio.
9. Our preferences regarding sound quality aren't just determined by technical aspects. Our upbringing and the types of music we're exposed to throughout life play a large role in shaping our aesthetic tastes. People from different cultures or musical backgrounds may gravitate towards different sonic attributes, challenging the idea of universal "quality" in sound.
10. Streaming services rely on audio compression techniques to reduce file sizes, which makes the music more efficient to stream. The issue here is that compression can sometimes sacrifice subtle nuances within the audio, leading to a tradeoff between the need for efficient streaming and the loss of fidelity in the music. This begs the question of whether audio engineers can optimize a track to address the artistic intent as well as ensure quality while being optimized for streaming consumption.
7 Essential Datasets for Linear Regression Analysis in Video Content Optimization - Seasonal Viewing Trends Exploration with Hulu's Time-Series Data
Hulu's time-series data, when analyzed for seasonal viewing trends, reveals how audience behavior shifts throughout the year. This data provides insights into how viewer engagement with content rises and falls in response to various factors, including holidays, weather changes, and cultural events. By breaking down the viewership data into components like trends and cyclical patterns, analysts can gain a more comprehensive picture of how these seasonal fluctuations impact video consumption. Using methods like linear regression, they can investigate the relationship between these patterns and various factors, such as genre, day of the week, or the time of year. The ability to understand these relationships is increasingly important for video content strategists.
Content creators and programming managers can benefit from understanding the factors driving seasonal viewership trends. This knowledge allows them to anticipate changes in audience behavior and refine their content strategies accordingly. In today's landscape, where viewers are presented with a vast amount of options, the ability to tailor content and marketing efforts to seasonal trends is crucial for achieving optimal audience reach and engagement. It's a key factor in adapting to the ever-changing preferences and patterns within the viewing audience. Understanding and adapting to these seasonal trends is an important element of competitive advantage in the video content ecosystem.
Examining Hulu's time-series data reveals fascinating seasonal shifts in viewing behavior. We see clear spikes in viewership during certain times of year, particularly the colder months, suggesting that seasonal factors significantly impact content consumption. It's intriguing to consider which content types perform best during these periods.
For example, when full seasons of shows are released, we see a jump in binge-watching in the fall and winter. It's tempting to think that people are more likely to settle in for extended viewing sessions when the weather isn't as appealing to be outdoors.
Beyond overall viewership, we also observe genre-specific shifts. Horror and thriller content, for instance, see a boost around Halloween, hinting at how cultural events influence viewing preferences. It's an interesting area of research to understand how specific events shape what viewers seek out.
The time-series data also illustrates a lifecycle of user engagement with content. Newly released shows naturally attract a lot of attention, but we also see older programs experiencing surges in viewership potentially tied to social media trends or word-of-mouth. It's a complex interplay of factors affecting how people choose to watch over time.
As more platforms release more content, we can start to see some saturation effects. During peak seasons, Hulu might see a decline in average viewing durations, highlighting the importance of content that stands out. It's not just enough to have a show, creators need to find ways to make their content distinctive to capture viewers' attention.
Interestingly, different age groups show differing preferences through the seasons. For example, younger viewers seem more drawn to current releases and specific genres, whereas older viewers may opt for classic content during certain times of the year.
We can also observe a direct relationship between major holidays and viewership spikes. Data points towards increased engagement with content related to Thanksgiving or Christmas movies during the relevant months, prompting questions on how culturally important dates factor into content planning.
The data also hints at the role of weather in influencing viewing behavior. For example, periods of heavy rain or snow are often correlated with increases in Hulu usage, reminding us of the importance of external factors that could affect audience engagement.
These seasonal spikes are often preceded by buzz on social media, showing that external marketing can greatly affect viewing trends. It's not just about the content itself, but how it is presented to viewers outside the Hulu platform.
Finally, we observe a correlation between Hulu's introduction of interactive features, like watch-alongs, and an uptick in social viewing experiences during certain festive seasons. This signifies an evolving engagement pattern with content that goes beyond traditional viewing methods.
In conclusion, Hulu's time-series data suggests that seasonal viewing patterns are influenced by a complex combination of cultural, environmental, and engagement factors. It's a fascinating area for further research to better understand viewer behaviors and optimize video content accordingly.
More Posts from whatsinmy.video: