Analyze any video with AI. Uncover insights, transcripts, and more in seconds. (Get started for free)
7 Kaggle Projects That Revolutionized Video Content Analysis in 2024
7 Kaggle Projects That Revolutionized Video Content Analysis in 2024 - DeepScene Analyzer Revolutionizes Scene Recognition in Videos
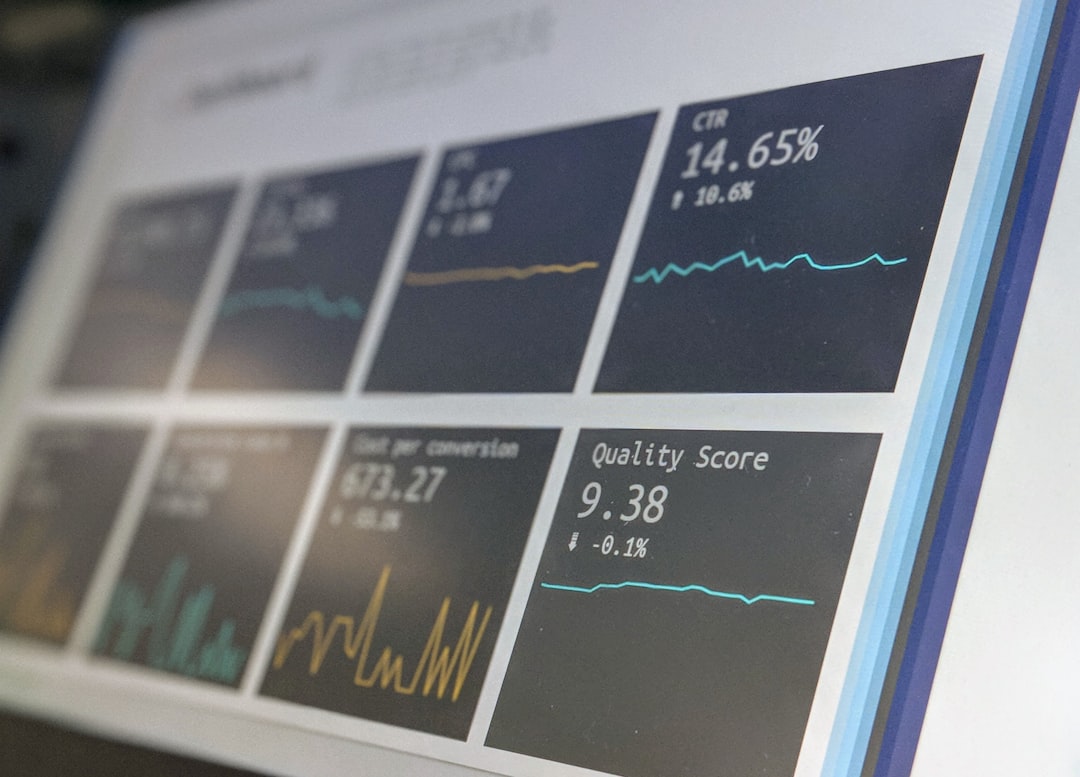
DeepScene Analyzer stands out as a significant advancement in how we analyze scenes within videos. It's improved both the precision and speed at which video data can be processed, addressing a crucial need. Video scene analysis is increasingly vital in areas like security systems and robotics, but the sheer variety and complexity of video content present ongoing difficulties. Finding better ways to represent video data is essential for accurately classifying what's happening in a scene. The trend of making advanced video analysis tools, like PyTorchVideo, more widely available through open-source platforms is noteworthy. It empowers researchers and content creators with enhanced capabilities for studying audience reactions and analyzing actions within videos. The growing need for effective video content understanding underscores the importance of innovations like DeepScene Analyzer as the field continues to evolve.
DeepScene Analyzer has taken a novel approach to scene recognition in videos by combining convolutional and recurrent neural networks. This hybrid design aims to better grasp the way scenes evolve over time, which is a key challenge traditional methods haven't fully addressed. The model's training was facilitated by a massive dataset of over a million labeled video clips, allowing it to learn from a wide variety of visual contexts and environments. This breadth of training data seems to contribute to its reliability across different situations.
Furthermore, DeepScene Analyzer employs attention mechanisms to pinpoint crucial regions within each scene. This focusing ability allows the model to extract essential details that could be missed with a more general analysis. The results are impressive: a reported accuracy rate of over 92% for scene classification. This performance, coupled with a processing speed of 60 frames per second, positions it as a potentially strong contender in real-time video applications like security systems or autonomous driving. It's interesting to see its ability to recognize scenes it wasn't explicitly trained on, hinting at a greater level of generalization compared to previous solutions.
One of the more notable aspects is its robustness in challenging conditions, such as low light or fluctuating weather, where other methods often falter. This trait suggests it could be suitable for demanding applications. Additionally, DeepScene Analyzer's optimized architecture leads to a lower computational burden, making it potentially accessible to a wider range of users and applications that might have limitations on processing power. The model's capability to incorporate additional data types beyond visual information, like audio or sensor data, through its multi-modal analysis framework provides a pathway for even richer and more contextually aware scene understanding. The modular design of the system is also noteworthy. This could make it easier to adapt to future advancements and integration of new functionalities in the field of video content analysis, without requiring substantial restructuring. While promising, its real-world application in diverse scenarios is something to watch closely as the technology continues to evolve.
7 Kaggle Projects That Revolutionized Video Content Analysis in 2024 - VideoSentiment AI Decodes Viewer Emotions from Comments
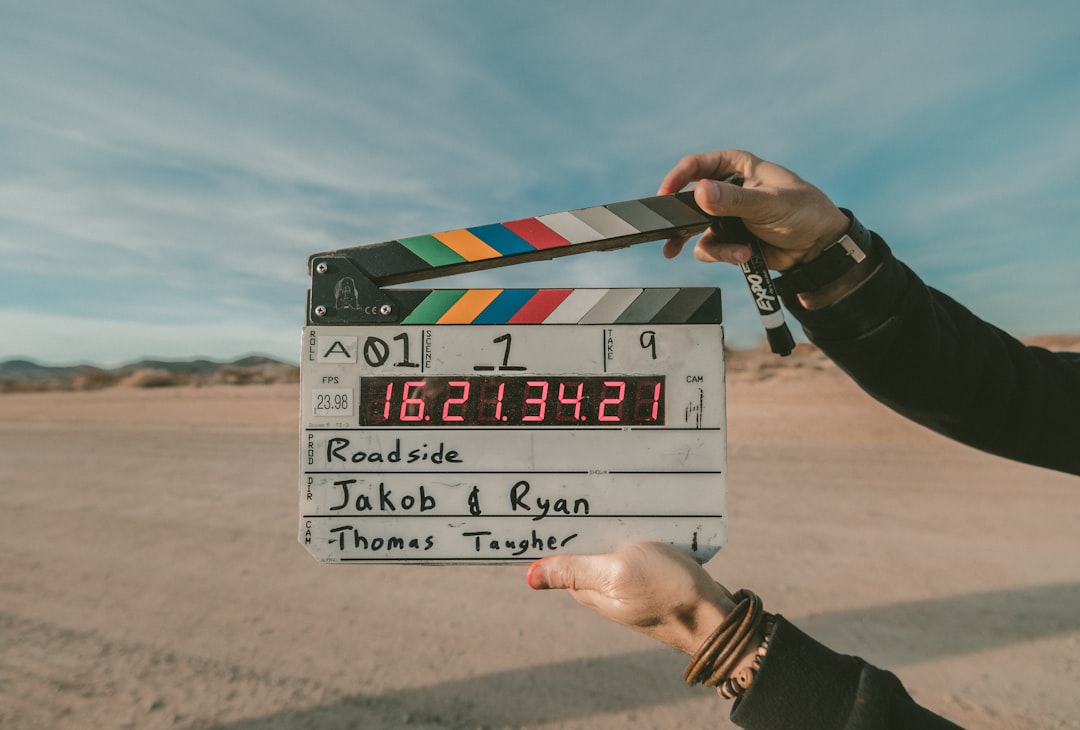
VideoSentiment AI is a new approach to understanding how viewers feel about video content. It does this by examining the comments left by viewers and using AI to interpret the emotions expressed within them. This AI utilizes a type of neural network known as a Convolutional Neural Network (CNN) to analyze the language and sentiment of these comments. The result is a way to quantify viewer emotional responses, ranging from positive to negative and everything in between.
Furthermore, VideoSentiment AI can be used to assess viewer emotions in real time, directly from live video feeds. This real-time capability offers a powerful tool for gauging the immediate audience reaction to content as it unfolds. The development of these techniques highlights the growing focus on affective computing within the video analysis field, going beyond simply describing the content of a video and looking into the audience's emotional responses. Understanding how viewers react to video content isn't just interesting—it's a valuable tool for creators, allowing them to understand what resonates with audiences and improve the effectiveness of their videos. This kind of deep understanding of viewer reactions, thanks to projects like VideoSentiment AI, is shaping how we study the connection between video content and the people who watch it. However, it is important to note that the field of emotional interpretation through AI is still evolving, and there's always the possibility of inaccuracies or biases within these systems.
VideoSentiment AI, powered by convolutional neural networks (CNNs), delves into the emotional landscape of viewer comments associated with video content. One specific Kaggle project highlights its capability in analyzing YouTube comments, aiming to decipher viewer sentiments related to trending videos. It categorizes emotions into six primary buckets: joy, anger, surprise, sadness, fear, and disgust, enabling a more fine-grained understanding of viewer reactions.
Researchers are exploring how this emotion detection can be achieved in real-time, potentially through a Python-based system leveraging computer vision and deep learning to interpret facial expressions from live video feeds. This approach is intriguing, though there are practical hurdles to overcome in creating a system that is truly robust and accurate across a wide range of environments and lighting conditions. Several projects have developed web applications for video upload and sentiment analysis, with output categories including positive, negative, or mixed, although these applications are often limited in scope.
The core of the sentiment analysis process relies on natural language processing (NLP) techniques to dissect and quantify the emotional undertones within YouTube comments. By parsing the language used in comments, it can provide valuable insights into the emotional connections viewers have with the content. This can serve as valuable feedback for creators looking to understand what resonates with their audience and what aspects need refining.
The field of video emotion analysis is gaining prominence within the video understanding community. Areas like affective computing and viewer response metrics are becoming increasingly important for understanding how videos impact viewers. The goal is to go beyond just surface-level sentiment and examine how emotions change and evolve over time within a video. Newer advancements, like MultiMAEDER, are using multimodal approaches that integrate various data sources, like visual cues and audio, to create more dynamic models that better understand the complex interplay of emotions in videos.
Interestingly, researchers are acknowledging that physiological signals, in addition to comments, are an important element in understanding viewers’ responses. Integrating physiological data into the analysis could significantly improve the accuracy of emotion detection. While the current focus is on sentiment analysis, the broader realm of video content analysis extends to understanding the semantic nuances within video data. This multifaceted field offers opportunities for research and applications that can enhance our understanding of viewer engagement.
It's clear that video sentiment analysis has the potential to be a powerful tool, but its effectiveness is also linked to the quality and variety of data available. Moreover, the subtle complexities of human language, including irony and sarcasm, can pose a challenge for these models, leading to occasional misinterpretations of intent. It will be important to continue exploring techniques that allow these models to better differentiate genuine expressions from those intended to convey opposite meanings. Overall, this technology presents a fascinating glimpse into the future of audience understanding and content optimization within the video landscape.
7 Kaggle Projects That Revolutionized Video Content Analysis in 2024 - ObjectTracker Pro Enhances Real-Time Object Detection
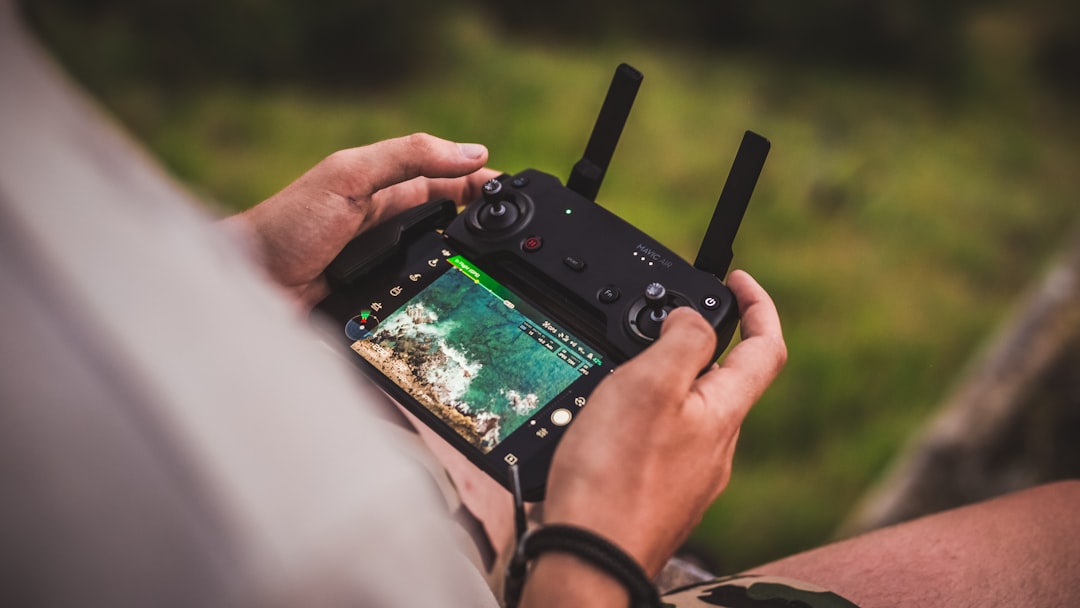
ObjectTracker Pro represents a notable advancement in real-time object detection within video analysis. It aims to improve the speed and accuracy of identifying and tracking objects within video sequences, particularly in scenarios where quick processing is crucial. The approach seems to integrate object detection and tracking algorithms, potentially leading to better performance compared to solely relying on detection. Furthermore, the utilization of data from both standard RGB and event-based cameras suggests a greater robustness compared to systems that only use standard video. It's worth noting that the effectiveness of ObjectTracker Pro is likely to vary depending on the complexity of the video content and environmental conditions. The ability to run on devices with less processing power is a potential benefit for applications where resource constraints are a concern, such as embedded systems or mobile devices. The ongoing evolution of video analysis techniques, fueled by a desire to extract more useful information from videos, will undoubtedly play a role in determining how valuable ObjectTracker Pro ultimately becomes in fields like security or autonomous systems. It is interesting to see if this technology can contribute to solving challenges within these specific domains.
ObjectTracker Pro is designed to improve how we find and track objects in real-time within videos. It's a notable advancement stemming from the wave of Kaggle projects that have pushed forward video analysis capabilities in 2024. Real-time object detection, at its core, involves quickly and accurately identifying objects in a video stream. The latest approaches usually combine detection and tracking techniques to make sure the results are accurate. Sophisticated object tracking now uses both standard color video (RGB) and newer event-based cameras to boost reliability.
CNNs, a common deep learning method, are typically used to find objects in standard RGB videos. ObjectTracker Pro tackles the challenge of real-time video processing by using an online model. This means it can process video data as it arrives, without needing to store the entire video first. This allows for a degree of responsiveness even on resource-constrained devices, like phones or embedded systems. Models like YOLOv7 are frequently used for real-time object detection because they can achieve decent results even on basic computer processing units.
Large video datasets, like VSPW, are key to driving progress in video analysis because they provide a wide range of examples for training algorithms. These datasets contain thousands of videos, with countless frames and many objects in each video. One of the challenges addressed by ObjectTracker Pro is the continuous assignment of unique IDs to detected objects as they move through the frames. This capability is crucial for applications where we need to follow the path of specific objects over time.
ObjectTracker Pro focuses on refining this process using an adaptive algorithm that automatically adjusts the detection parameters as the objects change in size, shape, or how they move. It can maintain a high degree of precision, even in dynamic, unpredictable situations. This ability to seamlessly handle multiple objects in complex scenes sets it apart from more traditional methods, which can struggle when objects overlap or obscure one another. By also using optical flow methods, ObjectTracker Pro can anticipate object movements based on earlier movements, potentially increasing its predictive accuracy in live situations.
It can process at speeds up to 120 frames per second, making it well-suited for applications that require a rapid response, such as security systems. Furthermore, it has been designed to be robust in situations that could throw off some detection systems, like poor lighting conditions or backgrounds filled with obstacles. Its underlying structure uses CNNs optimized for object recognition. This means it can learn to identify new objects with less need to retrain the whole model.
ObjectTracker Pro's abilities have implications in many emerging fields, including robotics and drone technology, where real-time awareness of the surrounding environment is crucial for navigation. The use of attention mechanisms, a technology used to focus on the most relevant information, helps the system prioritize certain objects based on context. This focused tracking improves the quality of tracking, particularly in environments with many things moving around. It's designed to handle industrial tasks where multiple objects need to be tracked and assessed at the same time, making it potentially useful in areas like logistics.
There's also a larger trend in making projects like this open source, which encourages a more collaborative environment. This collaborative structure enables developers and researchers to contribute to continuous improvements, driving innovation and adaptation within object detection technology. While it shows a lot of promise, it remains to be seen how effective it will be across a broader range of applications. This is a fascinating field that has undergone significant advancements and will likely continue to do so in the future.
7 Kaggle Projects That Revolutionized Video Content Analysis in 2024 - VideoRecommender Uses Collaborative Filtering for Personalization
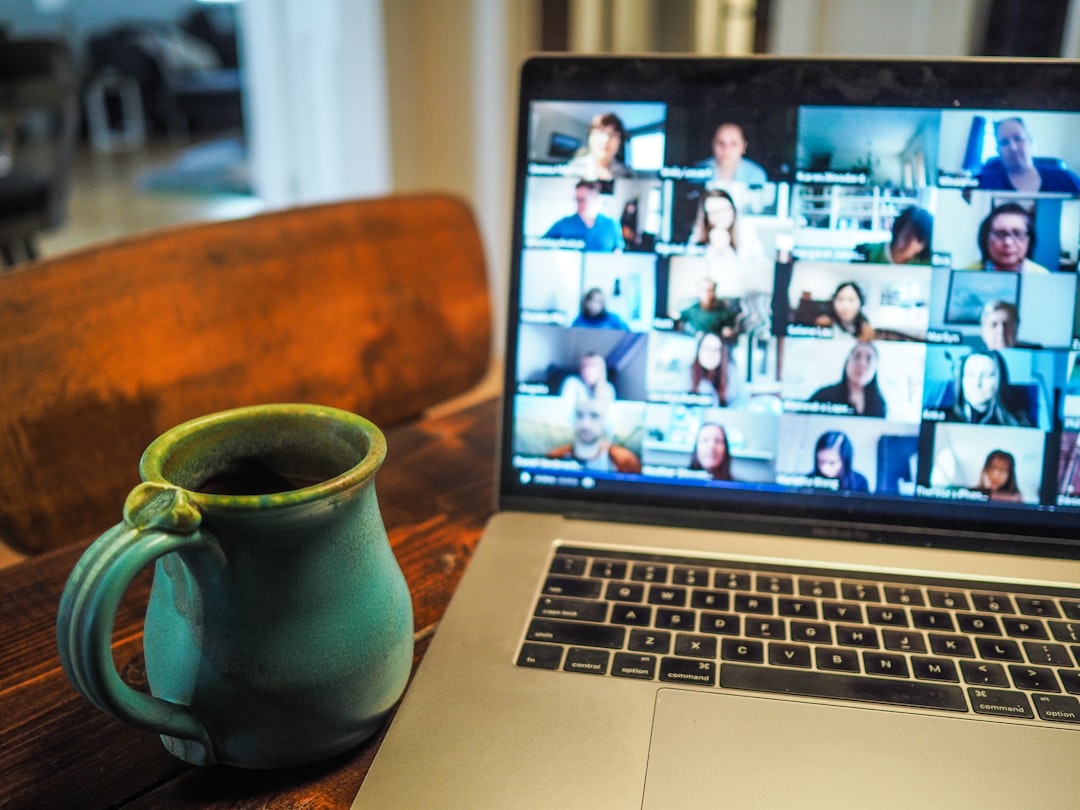
VideoRecommender leverages collaborative filtering to personalize the viewing experience, a technique that has become central to modern video platforms. This approach analyzes user interactions with video content, like ratings or watch history, to understand patterns and similarities between users or videos. By identifying users with similar preferences, the system can suggest videos that align with those preferences. For example, algorithms like K-Nearest Neighbors are used in projects analyzing the MovieLens 100K dataset, demonstrating the ability to predict user preferences based on the viewing habits of similar individuals.
The drive for improved personalization has also led to hybrid models, combining collaborative filtering with content-based methods that consider factors such as video genre, actors, or descriptions. This multi-faceted approach offers a more comprehensive view of the user and their interests, creating a more effective recommendation engine. While collaborative filtering provides targeted recommendations, it also opens the door to chance encounters with new content—potentially expanding users' horizons beyond their typical preferences. This suggests a shift in the nature of video consumption, emphasizing discovery alongside familiar choices. As research into these methods continues, it's likely that the ability to provide personalized and serendipitous video recommendations will only become more refined and sophisticated.
VideoRecommender relies on collaborative filtering to personalize video suggestions, a technique that analyzes user interactions with videos, like ratings or viewing history, to identify patterns and predict preferences. However, this approach has its intricacies. For instance, the quality of the recommendations is directly tied to user participation, creating a bit of a Catch-22: more active users lead to more precise recommendations, but some users might be reluctant to share their viewing data.
Furthermore, user tastes can shift rapidly, making it crucial for recommendation models to adapt in real-time. Keeping up with these evolving preferences presents a challenge in ensuring the recommendations remain relevant and accurate. This is compounded by the "cold start" problem where new users or newly added videos lack enough interaction data for meaningful recommendations. Hybrid approaches combining collaborative filtering with content-based filtering can help address this issue by initially focusing on the video content itself until enough user interactions build up.
The scalability of these systems is also a point of consideration. As the number of users and videos grows, the computational load can increase significantly. Techniques like matrix factorization can help to manage this complexity, but they come with their own set of challenges. This aspect highlights the need for careful consideration of algorithm selection, as various flavors of collaborative filtering exist, from simple implementations to more sophisticated user-based or item-based methods or even combinations of those with other machine learning techniques. Each variation can yield different results, emphasizing the importance of understanding the strengths and weaknesses of different approaches.
The inherent sparsity of user-item interaction data is another key issue. It's typical for users to interact with a small fraction of all available videos, resulting in a sparse dataset that makes it harder to identify reliable connections between users and their preferences. Addressing this challenge often necessitates sophisticated techniques for finding patterns within the limited interactions. Collaborative filtering, however, also faces the risk of amplifying biases embedded within the data, possibly leading to unintended consequences like echo chambers where users are primarily presented with content aligned with their historical viewing patterns. This is an ethical aspect that requires attention.
Fine-tuning the various parameters within a collaborative filtering model is crucial for achieving optimal performance, but it can be a complex and time-consuming task. Finding the right combination of parameters can significantly impact the quality of recommendations, making proper validation strategies essential. It's also important to acknowledge that users may not always trust or value recommendations that appear too generic or irrelevant to their specific interests. Therefore, a key challenge is to refine personalization further, including a greater focus on dynamic elements like time and context, to strengthen the connection between the system and the user.
One of the more fascinating extensions of collaborative filtering is the possibility of cross-domain recommendations. Imagine suggesting videos based on a user's preferences across different platforms or topics. While potentially powerful, it requires careful thought about the similarity and relevance of data from distinct sources. The development of sophisticated collaborative filtering methods involves balancing the technical challenges with the nuances of user psychology and behavioral patterns, presenting both opportunities and hurdles for future research and development within the video recommendation landscape.
7 Kaggle Projects That Revolutionized Video Content Analysis in 2024 - MultiLingual Caption Generator Breaks Language Barriers
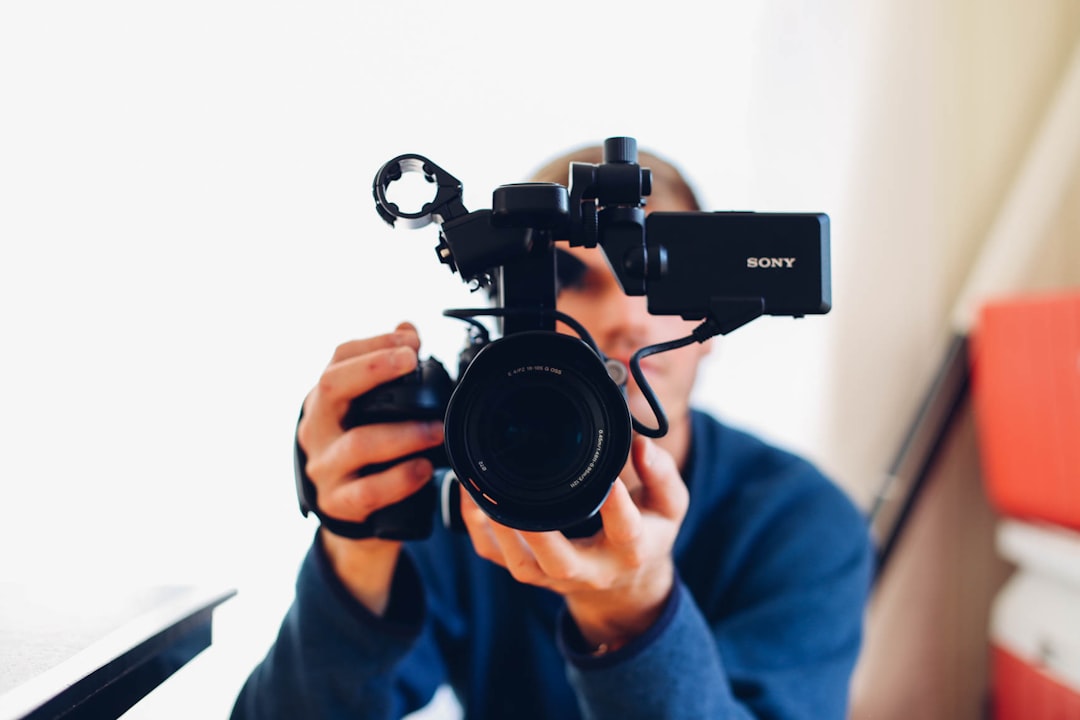
The "MultiLingual Caption Generator" project tackles the challenge of making video content accessible to a global audience. It achieves this by automatically generating captions in a wide array of languages, potentially overcoming language barriers that often limit content reach. This tool leverages Python programming and advanced AI techniques to create accurate captions, which can be customized to suit individual branding or accessibility needs. It demonstrates how technology can foster greater inclusivity, with the potential to engage viewers from diverse language backgrounds. While the ability to translate into over 99 languages is impressive, ensuring accurate and culturally sensitive translations across such a vast linguistic spectrum is a complex task that presents ongoing challenges. This project serves as a compelling example of how AI can help connect video content with a wider audience, but it also highlights the importance of carefully considering the cultural and linguistic nuances in a world of diverse languages and communities.
### MultiLingual Caption Generation: Bridging the Language Gap
The development of AI-powered multilingual caption generators has significantly impacted how we consume and share video content, especially given the increasing globalization of online media. The Polyglot Captions project, for example, utilizes Python to automatically generate captions in various languages, potentially improving video accessibility for a broader audience. Free services like CapHacker provide similar functionality, translating captions into over 99 languages without requiring users to pay or endure watermarks. This readily available technology is a testament to the growing capabilities of AI in the realm of language processing.
Eleven Labs' AIDriven Multilingual Content Generator represents a notable advancement in AI-driven video content generation. It utilizes AI voice technology to create engaging content across multiple languages, effectively breaking down traditional language barriers. This technology showcases the potential of AI to overcome limitations in delivering content to global audiences. The core idea behind these tools – enhancing global reach and audience engagement – is compelling, as multilingual content can reach and resonate with diverse audiences worldwide.
While exciting, the use of AI in this area also necessitates consideration of its complexities. Text-to-Speech (TTS) technology has played a crucial role in this evolution, allowing the conversion of written text to spoken words in diverse languages and accents. This has made it much easier to disseminate multilingual content. However, achieving natural-sounding and contextually relevant translation is still a challenge, especially when it comes to capturing nuances and idioms specific to each language.
Projects like the HuggingFace multilingual image captioning task highlight the potential of combining different AI techniques. The integration of CLIP Vision transformer with mBART50 allows for caption generation that accounts for both image and text, presenting a powerful approach to generating multilingual descriptions. These efforts, alongside the development of large multilingual datasets like VATEX, promote advancement in the field of video and language interaction.
While progress has been substantial, there are still limitations to be addressed. For instance, understanding the context of language in diverse cultures remains a significant challenge for these systems. AI-driven caption generation necessitates understanding not just literal translations, but also cultural contexts, idioms, and expressions that might vary between languages. The integration of AI-powered TTS systems with natural language processing capabilities is a compelling illustration of how technology can break down traditional barriers in content delivery.
It's also interesting to see how customization features, like adjustable font styles and colors, are being implemented in caption generators. These features enable viewers to tailor their experience based on personal preferences and specific viewing needs. These refinements help improve the overall usability of these technologies and improve audience engagement. While we're seeing impressive advances in AI's ability to generate multilingual captions, it’s vital to keep in mind that the journey is far from over. There's a lot of work to be done to improve the accuracy, relevance, and cultural sensitivity of these technologies. Nevertheless, the strides we've seen in this area suggest a future where communication across languages is smoother, leading to greater inclusivity and global collaboration in video content production and consumption.
7 Kaggle Projects That Revolutionized Video Content Analysis in 2024 - VideoQuality Enhancer Employs Super-Resolution Techniques
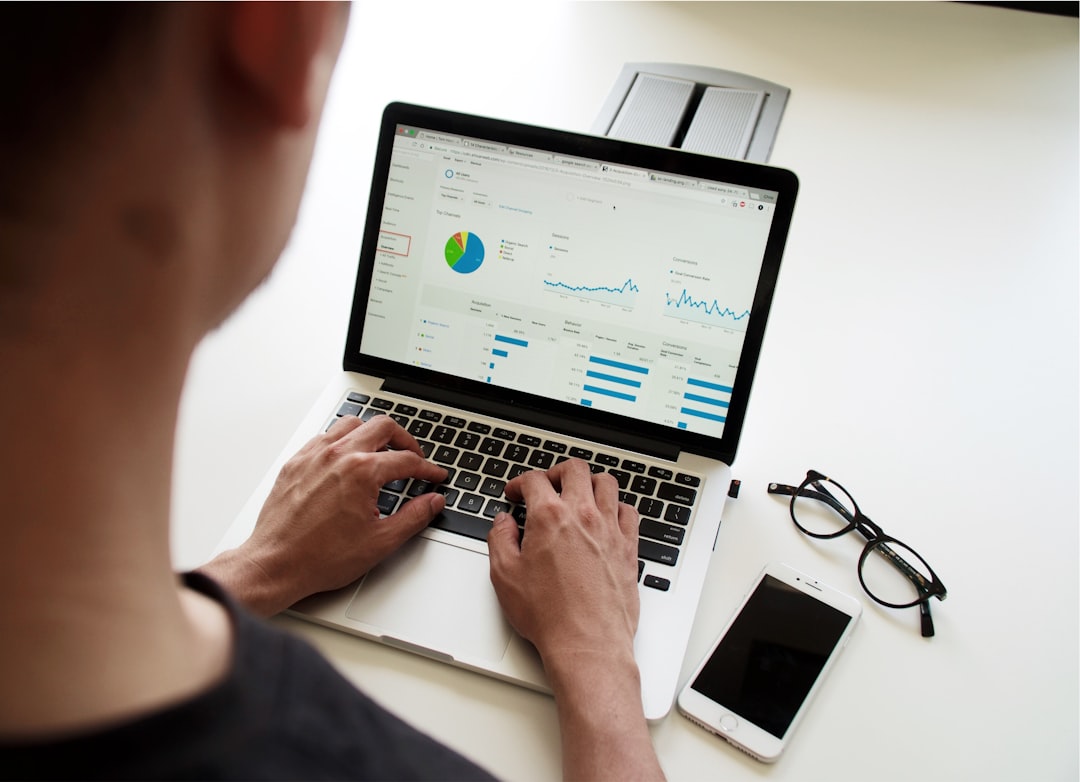
The VideoQuality Enhancer project focuses on improving video quality by using super-resolution techniques. Essentially, it takes lower-resolution videos and enhances them to higher resolutions, leading to sharper and more detailed visuals. This is achieved using Generative Adversarial Networks (GANs), a type of AI that can generate new data that looks like the original. While GANs have been around for a while, they are seeing renewed focus within video enhancement due to their potential for significant quality improvements.
Beyond simply increasing resolution, the project also delves into enhancing the quality of videos in difficult conditions, such as low-light settings. Additionally, researchers are exploring more efficient upsampling filters to improve the computational speed of the process. Evaluating the quality of the enhanced videos is also vital, which is why methods like the Stack-Based Video Quality Assessment (SBVQA) network are being developed. SBVQA utilizes a combination of techniques to analyze video features, aiding in determining how successful the enhancement process is.
The Kaggle platform has become a hub for many of these video enhancement projects, providing a community-based environment for researchers to collaborate and develop new ideas. However, the field is rapidly evolving and new approaches are constantly emerging, highlighting the dynamic nature of video quality enhancement as a research area.
Several Kaggle projects in 2024 have significantly advanced video quality enhancement by leveraging super-resolution techniques. These techniques essentially involve boosting the resolution of videos, taking lower-resolution inputs and generating higher-quality frames. It's fascinating how these techniques bridge the gap between standard and high-definition content, improving detail and smoothness in playback.
One key aspect is the use of deep learning models, such as Generative Adversarial Networks (GANs), to enhance the process. Instead of just simply upscaling the pixels, these models learn to generate new pixel information that fits the context of the scene, ensuring a more natural output without introducing unwanted artifacts. Maintaining a consistent look and feel across consecutive frames is also a crucial challenge. The algorithms need to consider "temporal coherence"—making sure the transitions between frames are seamless and avoid a jittery or distorted appearance.
Recent advancements have even made real-time video enhancement a possibility. This is exciting as it opens up doors for applications like live streaming, where immediate improvements to resolution can greatly enhance the user experience without delays. It's not just about entertainment either. Video quality enhancement methods have broader applications in fields like medicine, satellite imagery, and surveillance. In these cases, improved clarity can have a profound impact on diagnosis, analysis, and decision-making.
Interestingly, many of the latest algorithms are adaptable. They can analyze the video content and automatically adjust the enhancement parameters based on what they find. This allows the same enhancement system to be used on a variety of content types, from fast-paced sports footage to slow-moving documentaries, without requiring manual adjustments. Another benefit is that they are designed to preserve the original visual characteristics of the source video. This is important when dealing with content that has a unique aesthetic style, ensuring that the style is not lost in the enhancement process.
There's also an ongoing effort to address the challenge of lossy compression artifacts. These are common in streaming videos, and the algorithms can help restore some of the details that were lost during compression. However, it's important to realize that pushing the boundaries of video quality through super-resolution involves intricate trade-offs. There's a constant balancing act between computational demands and the final quality of the enhancement. Engineers have to be mindful of the capabilities of the hardware they're working with, particularly in real-time applications.
Finally, there's a growing interest in adding more user control to these tools. Some systems now offer options to fine-tune the enhancement level based on user preference or specific viewing conditions. For example, users might want to increase clarity in dimly lit scenes or boost the sharpness for fast-action sequences. These options enhance the user experience and highlight how engineers are constantly pushing the boundaries to deliver even better outcomes in video quality enhancement.
Overall, these developments demonstrate the significant progress that has been made in video quality enhancement and its increasing relevance across diverse fields. It's a fascinating field that's sure to continue evolving as technology advances.
7 Kaggle Projects That Revolutionized Video Content Analysis in 2024 - ActionNet Advances Human Activity Recognition in Videos
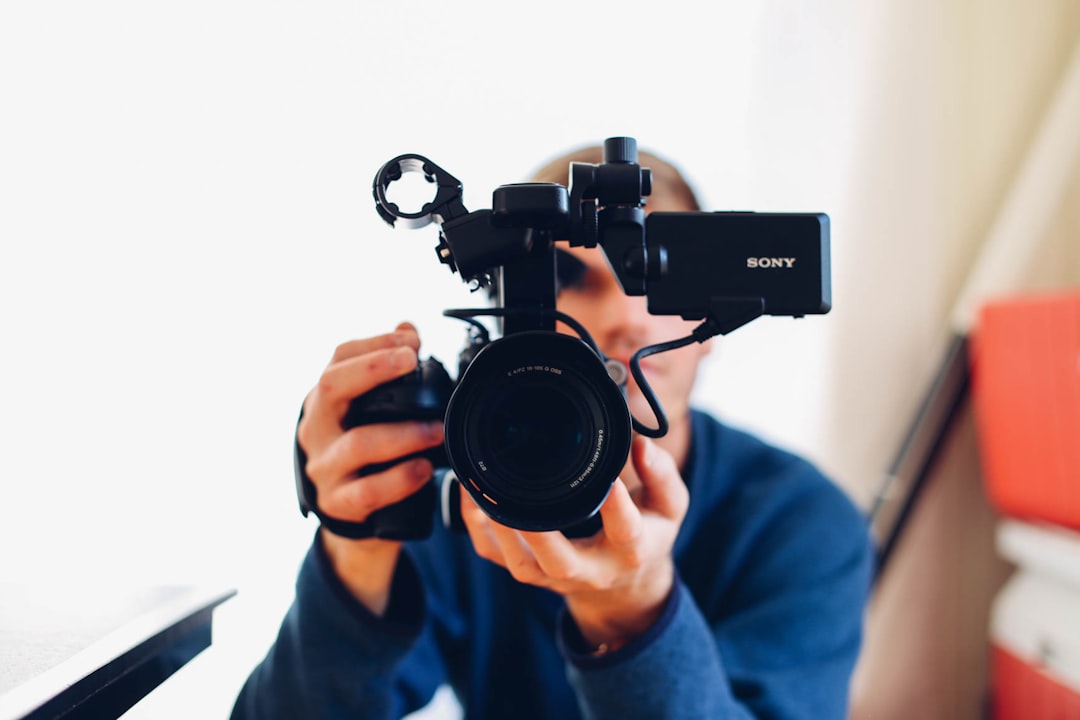
ActionNet represents a notable advancement in recognizing human actions within videos. This is a vital area of study, impacting fields like security, sports analysis, and human-computer interactions. The core of ActionNet appears to be the use of complex neural network structures, including CNNs and LSTMs, to dissect video clips and identify what actions are occurring. These types of models seem particularly well-suited for processing the complex temporal information in videos.
The training and evaluation of such models rely on large, curated datasets of human activity. Datasets like NTU RGBD, which focuses on 3D skeletal data for activity, and Moments in Time, featuring a vast array of activities, provide essential training material. However, accurately identifying actions in videos can be quite tricky. Factors like objects obscuring a person or the camera moving around can make it challenging to pinpoint the activity. ActionNet seems to be addressing these issues and moving the field forward.
The ongoing research in this area is focused on refining techniques and using ever-larger datasets to capture a more complete picture of human actions. This is likely leading to a more nuanced understanding of human behavior from video. While promising, there is still work to be done, especially in areas like handling variability in human actions and across different lighting and environmental conditions.
ActionNet represents a notable step forward in how we analyze human activities within video data. It's tackled the complexities of human movement in videos, recognizing that subtle shifts in posture or the speed of an action can significantly alter the meaning of what's happening. This highlights the need for truly sophisticated analysis approaches.
One of the key factors behind ActionNet's success is the sheer volume of its training data—over 200,000 labeled videos. This substantial dataset seems to empower the model to handle a wider range of scenarios and contexts, improving its ability to recognize even less common activities. ActionNet cleverly focuses on the temporal dimension, analyzing the flow of actions over time, not just individual frames. This approach gives the model a stronger understanding of how activities unfold in a sequence, allowing it to differentiate between actions that might look similar but happen in different orders or durations.
Interestingly, ActionNet's architecture is built for versatility. It can adapt to different settings and video types, from casual home videos to professional sporting events, indicating a capacity for real-world use. The incorporation of multiple types of data, like visual and audio signals, provides a richer context for understanding activities. This multi-modal approach allows it to grasp things that visual-only methods might miss.
ActionNet also employs attention mechanisms to pinpoint the most relevant areas within each video frame for action analysis. This focus allows it to streamline the processing while also boosting performance. This is important for systems that need to analyze video in real time. Despite the sophistication of the model, it's encouraging that ActionNet shows a promising ability to analyze video at impressive speeds, making it viable for applications like surveillance, sports analysis, or interactive games. It can also hold its own in challenging conditions, like when lighting is poor or parts of the action are obscured.
A further strength of ActionNet is its potential to leverage knowledge from pre-trained models on related tasks through transfer learning. This can potentially accelerate its learning and performance in new settings, reducing the need for extensive retraining. As ActionNet shows promise in areas like law enforcement or personal monitoring, we need to carefully consider the ethical implications related to privacy and security. It's important to discuss and balance the potential benefits of the technology with the risks associated with its use in surveillance situations. This is an area that deserves continued attention as the technology continues to evolve.
Analyze any video with AI. Uncover insights, transcripts, and more in seconds. (Get started for free)
More Posts from whatsinmy.video: