Analyze any video with AI. Uncover insights, transcripts, and more in seconds. (Get started for free)
7 Essential Resources for Tracking Machine Learning Advances in 2024
7 Essential Resources for Tracking Machine Learning Advances in 2024 - ArXiv Sanity Preserver Revamp Enhances ML Paper Tracking
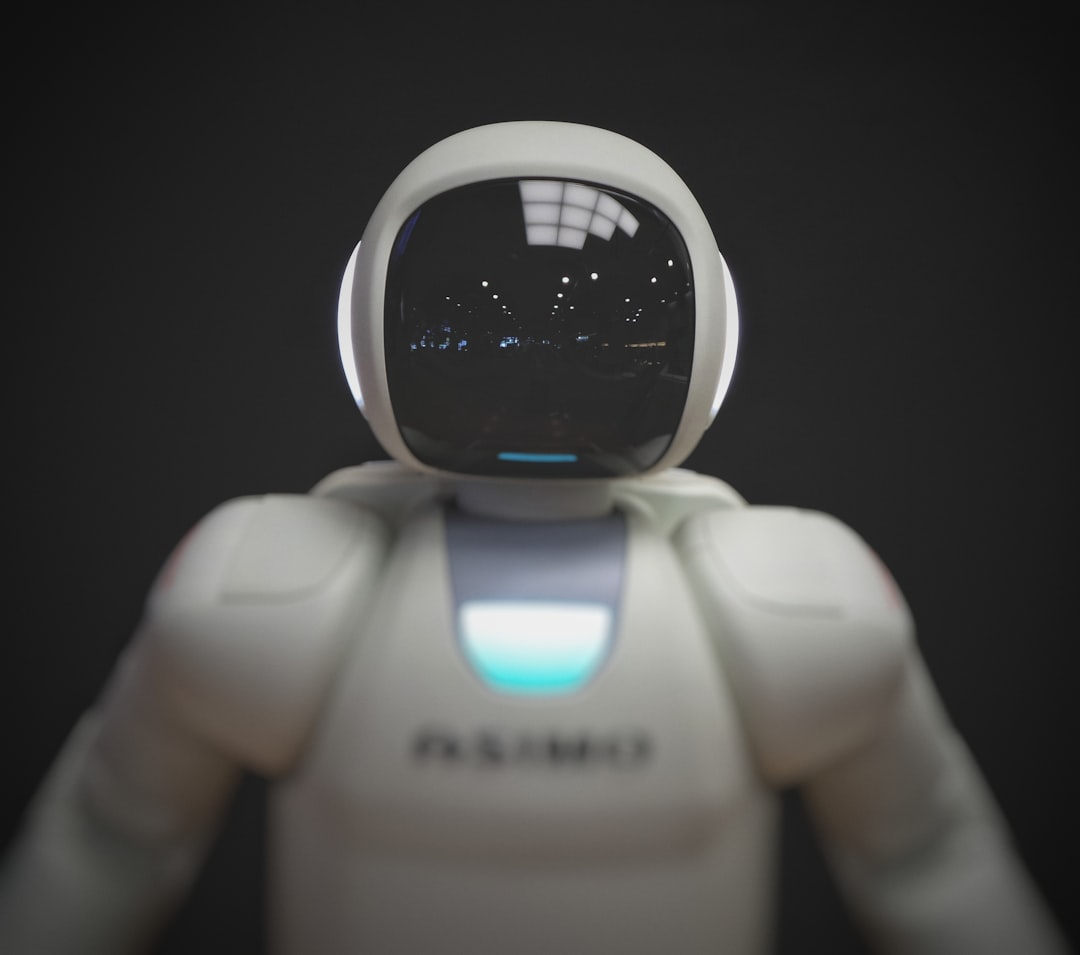
The ArXiv Sanity Preserver has recently undergone a makeover, aiming to make it easier for researchers to track machine learning papers. This revamp brings features such as multiple tags per account and scheduled email updates, which are designed to keep users informed about new developments in their fields. However, some critics point out that the email updates may be overwhelming for those tracking a large number of topics. There are also concerns that this tool might not be ideal for those seeking comprehensive search functionalities, as it primarily focuses on paper alerts rather than broader discovery.
The ArXiv Sanity Preserver has gotten a makeover, aiming to help researchers navigate the ever-growing ocean of machine learning papers. I've been using it for a while, and the new features are definitely useful. For example, I can now tag papers with multiple keywords, which helps me categorize them and quickly find what I need. The personalized recommendations are interesting, but I'm not sure how accurate they are yet. I appreciate the email alerts, though - they let me know when new papers on topics I'm interested in get published. It's definitely a time saver, but I still like to go through the abstracts and conclusions to get a feel for a paper before diving into the details.
I'm curious to see how the platform develops in the future, especially with the recent surge in AI-related research. There's just so much information out there, and I think tools like ArXiv Sanity Preserver are crucial for keeping up.
7 Essential Resources for Tracking Machine Learning Advances in 2024 - MLOps Weekly Newsletter Reaches 100,000 Subscribers
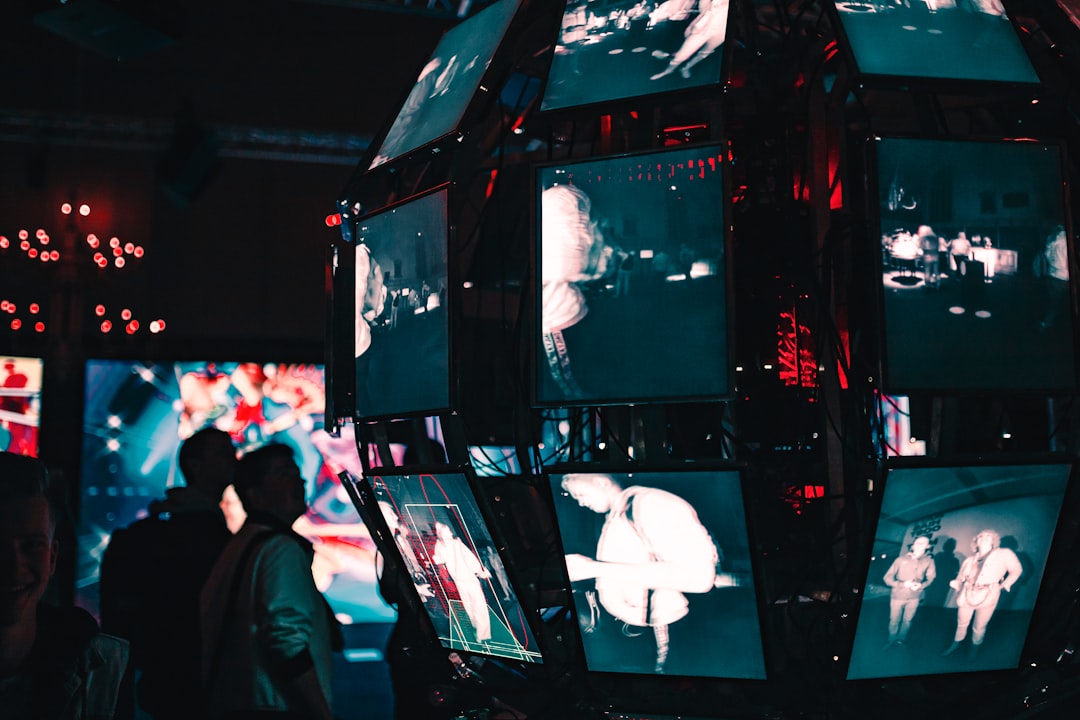
The MLOps Weekly Newsletter has hit a major milestone – 100,000 subscribers. This is a big deal, showing how much interest there is in MLOps, the field that combines machine learning with operational practices for better model development and deployment. As more businesses use machine learning, the need for MLOps expertise grows. This newsletter's popularity reflects a broader trend – professionals in this area are seeking knowledge and solutions to the challenges they face. It's a sign that the field is maturing and becoming a crucial part of the machine learning landscape.
The MLOps Weekly Newsletter hitting 100,000 subscribers is a clear sign of the growing interest in MLOps. It shows that organizations are realizing it's not enough to just build machine learning models. They need to be able to deploy and manage them effectively at scale.
This newsletter has become a valuable resource for practitioners, providing a "how-to" on using best practices, tools, and methodologies. It's definitely become essential as machine learning applications get more complex. It's fascinating to see how the focus has shifted towards collaboration. It seems that data scientists, engineers, and business people all need to work together to make machine learning work. This makes sense, given the complexities of machine learning workflows and the wide range of tools and frameworks out there. It's almost like the industry is struggling to keep up with the latest developments.
The newsletter isn't just about the technical aspects of MLOps. They're also addressing the "human side" of implementing these new technologies, which includes things like organizational culture and change management. They're recognizing that getting people and processes working together is just as important as the technology itself.
I particularly like how the newsletter has started to include case studies and real-world examples. These stories are really useful, as they show how other companies have overcome the challenges of putting MLOps into practice.
The success of this newsletter seems to be a reflection of how important MLOps engineers are becoming. Companies need experts who can not only build models but also make sure they're working correctly in real-world settings. I can see why so many people are eager to learn about this field.
The newsletter's growing subscriber base shows that there's a lot of interest in operationalizing machine learning. It seems people are really hungry to learn more about MLOps and improve their skills in this area. The newsletter is clearly filling a knowledge gap in the industry.
I've been hearing a lot about standardization in MLOps lately. It's almost like people are realizing that having a consistent set of practices can make all the difference in the world. This newsletter is definitely playing a role in these conversations and helping people learn about best practices and the latest standards.
Overall, this news about the MLOps Weekly Newsletter points to a larger shift in the AI industry. Companies are focusing more on operational maturity. They want to make sure that their AI projects are successful and that they're getting a good return on their investment. This is a great development for the industry, as it means that companies are taking a more holistic approach to AI.
7 Essential Resources for Tracking Machine Learning Advances in 2024 - Papers with Code Introduces Interactive ML Model Comparisons
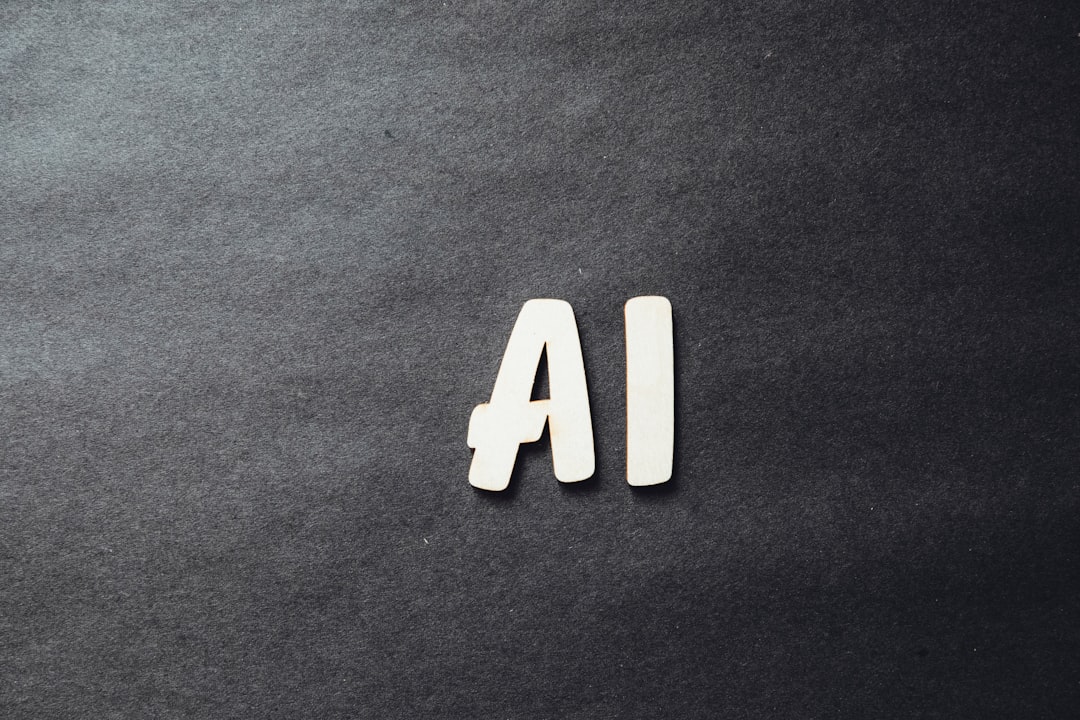
Papers with Code has introduced a new interactive feature for comparing machine learning models. This lets users easily analyze how different models perform. It's meant to help researchers and practitioners understand the strengths and weaknesses of various models. The ability to directly compare models across different datasets and under various conditions should be valuable for making informed decisions about which model to use.
However, this tool's effectiveness will depend on how well it is implemented and the quality of the data it presents. It's still early days, so it's important to see how this feature evolves and how it's used by the community. It's just one more resource that can help keep up with the rapid changes in machine learning in 2024.
Papers with Code has finally gotten around to adding interactive comparisons between models, and I have to admit it's a welcome change. Now, instead of just passively looking at a list of papers with their performance scores, I can actually see how different models stack up against each other on the same benchmarks. It's a bit like having a visual dashboard where you can compare the performance of different cars.
This interactive feature also helps me see how each model performs on different datasets and across different metrics, which is super useful for deciding which model to use for my project. I'm particularly impressed with the direct links to code implementations, which lets me not only see how the models work, but actually run them myself to see if they live up to the hype.
I'm not sure if it's just me, but I've noticed a lack of comprehensive comparisons between models in most academic repositories, so Papers with Code is really filling a gap here. The fact that it's actively updating its information is a huge plus, as it means I can always trust that I'm seeing the latest data.
One thing I'm particularly interested in is how this new feature is being used to compare models in less popular machine learning domains. I think it has the potential to help us better understand the performance of models across a wider range of applications, which is especially important as machine learning is becoming increasingly ubiquitous.
Overall, I'm excited to see how this feature evolves and impacts the way we use Papers with Code. I think it has the potential to become an even more valuable resource for researchers and practitioners. This community-driven approach to knowledge sharing is a breath of fresh air in the world of academic research.
7 Essential Resources for Tracking Machine Learning Advances in 2024 - Twitter's ML Research Feed Curated by Top AI Researchers
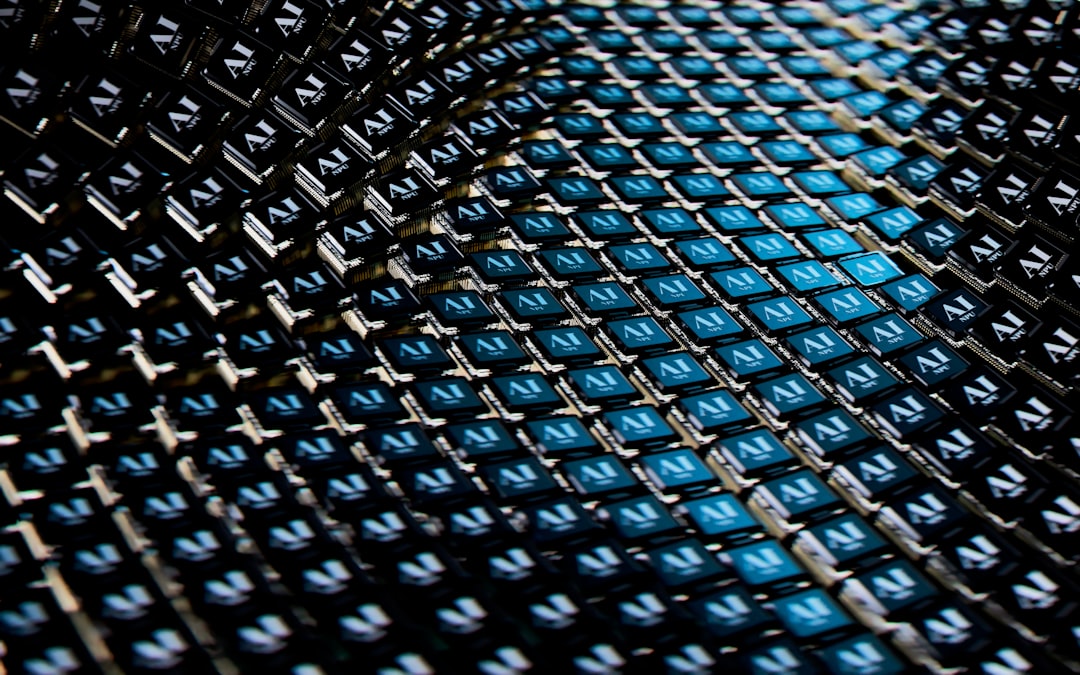
Twitter has become a hotbed for machine learning (ML) research, with top AI researchers using it to share their latest findings and insights. This platform is a goldmine for anyone wanting to stay updated on the latest trends and developments. By following a curated list of influential accounts, you can tap into a constant flow of information from leaders like KaiFu Lee and Andrew Ng. This curated feed also highlights the intersection of art and technology, showcasing the work of pioneers like Refik Anadol. In today's rapidly evolving AI landscape, a curated Twitter feed can be invaluable for anyone wanting to stay ahead of the curve, whether you're a seasoned expert or a newcomer to the field.
Twitter's ML Research Feed, curated by top AI researchers, is a valuable resource for staying on top of machine learning breakthroughs. It provides a real-time glimpse into the field, unlike the more traditional academic publications which often take time to be released. What's interesting is that this feed features not just big names, but also emerging voices in the field, which makes for a more diverse set of ideas and insights. I've also noticed that many of the posts highlight the engagement metrics like retweets and likes. These stats give a good indication of the community's interests, which helps me identify trends that might otherwise go unnoticed.
Another neat thing is that a lot of researchers are now incorporating visual content like graphs and charts alongside their insights. This visual approach really helps to explain complicated data more easily compared to text-only updates. I also appreciate the open nature of Twitter. It allows researchers and practitioners to directly connect and chat. This sort of interaction can lead to spontaneous collaborations and discussions which might not happen in more formal academic environments. What I like most is that the focus is on applied research. The posts often highlight real-world applications of theoretical models, which encourages a mindset focused on practical impacts. It's a great way to bridge the gap between research and development.
It's worth noting that the international nature of Twitter means that the feed features researchers from all over the world. This exposure to different cultural and methodological approaches sparks fresh ideas and unique solutions. Another thing I find helpful is that the feed frequently links to other research resources, including full papers or datasets. This streamlined process allows for quick access to comprehensive information.
Some researchers also use Twitter to discuss the limitations and ethical implications of their work. This type of transparency can foster a deeper understanding of the challenges facing the field. It’s crucial to have critical conversations about the responsibility of machine learning professionals.
The algorithms that curate this feed learn from user interactions, meaning that the most engaging and relevant research topics always stay at the forefront. It's a constantly evolving tool that keeps me informed in this fast-paced world of machine learning.
7 Essential Resources for Tracking Machine Learning Advances in 2024 - Google AI Blog Launches Monthly ML Breakthroughs Roundup
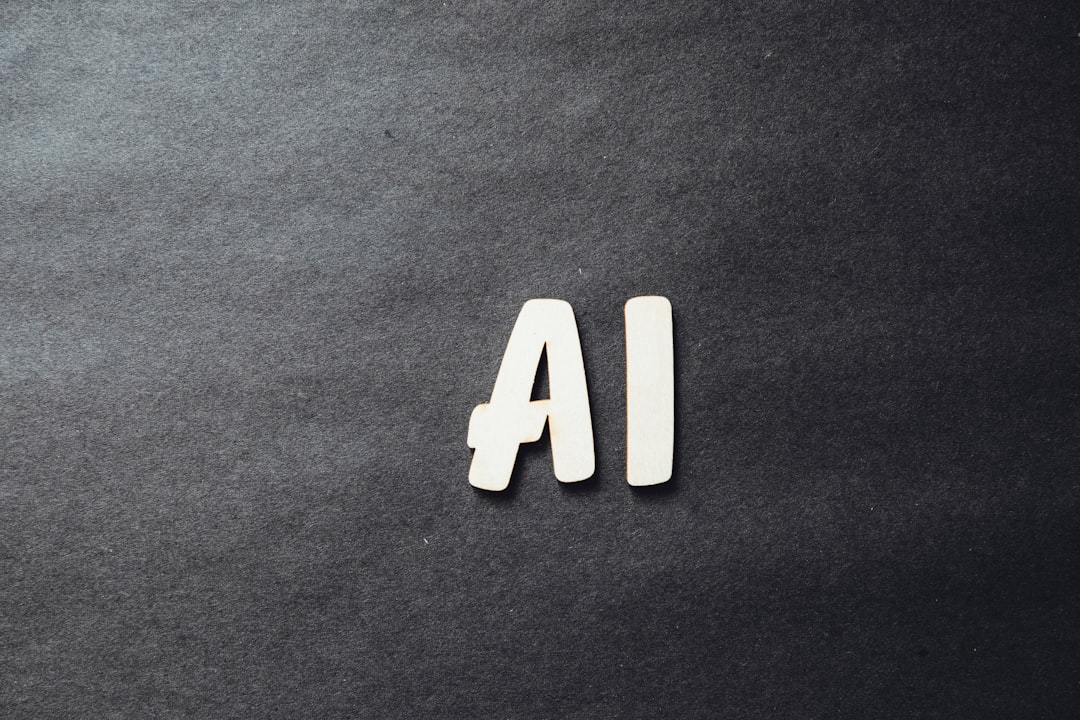
Google's AI blog has started putting out a monthly collection of machine learning breakthroughs. This is an attempt to break down the fast-paced changes in machine learning and make it easier for people to understand. Google has been involved in machine learning for a long time, for over 20 years, so they're not new to this. With the latest developments in things like how computers understand language and other kinds of artificial intelligence, this roundup could be useful for people who are experts in this area and for people who are just interested in learning more about it. It's still important to look at these new developments carefully and think about how they fit into the bigger picture.
The Google AI Blog has started a monthly roundup of machine learning breakthroughs. I'm curious to see what this will offer.
What stands out to me is that they're using a combination of human and machine intelligence to curate this. They've got dedicated teams who are carefully selecting the most important papers, but they're also using algorithms to spot trends. I'm curious to see how this automated approach will perform.
It seems they're also taking a global approach, highlighting contributions from researchers from all over the world. This is good, because a lot of cutting-edge work happens outside of the usual Western centers. I'm hopeful this will be more than just lip service.
Another interesting aspect is the inclusion of ethical discussions alongside the technical stuff. I think it's crucial to think about the societal impact of these technologies, not just how they work. It'll be interesting to see how they balance these two aspects.
I also like the fact that the roundup integrates with Google services. It's not enough just to read about the latest advances. We need to be able to use that knowledge, and this makes it easier to apply those insights.
It'll be interesting to see what kind of case studies they feature. I want to know how these breakthroughs are actually being used in the real world, not just in research labs.
And finally, I appreciate that they're encouraging reader interaction. It's good to have a conversation about this stuff, not just a one-way flow of information.
Overall, this roundup seems to be a promising addition to the growing list of resources for tracking machine learning progress. I'm looking forward to seeing how it evolves in the future.
7 Essential Resources for Tracking Machine Learning Advances in 2024 - DeepMind's Quarterly ML Progress Report Gains Traction
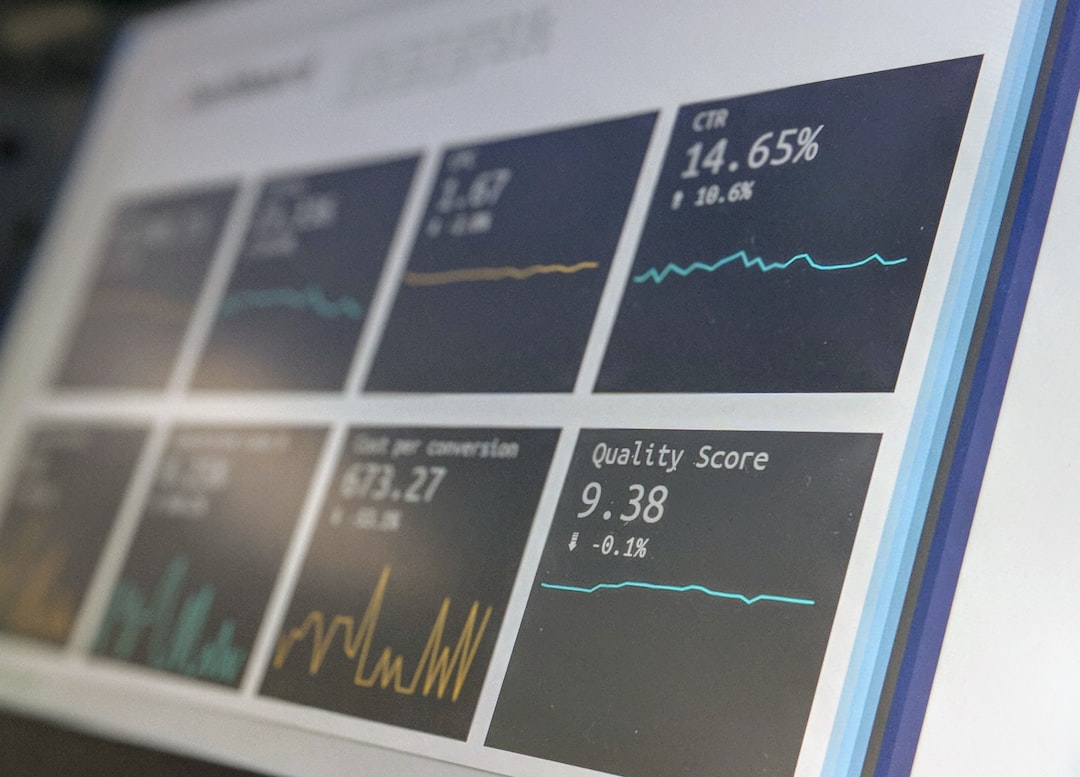
DeepMind's quarterly machine learning progress report has become a popular tool for tracking advancements in the field, especially in 2024. This increased interest comes as the demand for AI professionals is expected to grow by 40% by 2027. With so many new developments happening and an ever-growing amount of research – doubling every two to three months – it's important to have resources that can help you stay up to date. This report is one of those resources, providing insights for anyone interested in machine learning, from experienced professionals to curious learners. But even with a resource like this, you need to be critical about what you're learning and evaluate new developments carefully. The speed at which things are changing in this field is overwhelming, so it’s vital to maintain a discerning perspective.
DeepMind's Quarterly ML Progress Report has become a go-to resource for keeping track of developments in machine learning. I've been following it for a while, and the latest issue has some interesting points. They're really pushing the idea of reproducible research, emphasizing that we need to be able to validate models with publicly available data and code. It's great to see a focus on transparency and accountability, especially with the rapid growth in ML applications.
The report also dives deep into the performance of various neural architectures. Apparently, some newer designs are outperforming established models by a significant margin, particularly when trained on large, diverse datasets. This could be a major shift in how we think about selecting models for future projects.
They're also adding some new performance indicators that go beyond traditional metrics like accuracy and precision. These new indicators are designed to assess the ethical implications of ML applications, including things like bias mitigation and interpretability. This is definitely a hot topic right now, and it's good to see that it's being incorporated into how we evaluate models.
Another interesting point is that the report highlights the increasing trend of interdisciplinary collaborations. They're showing projects that blend insights from fields like neuroscience, cognitive science, and ethics to drive innovation in ML algorithms. It seems that we're moving away from the traditional silos in ML research and embracing a more holistic approach to problem-solving.
One of the more surprising findings is the focus on federated learning. This approach allows models to be trained on decentralized devices without compromising user privacy. This is critical given the increasing concerns about data privacy, and I think it could really take off in the future.
There's also a lot of talk about synthetic data generation, which is being used to address the problem of data scarcity. It seems that in cases where getting real-world data is challenging due to privacy or other issues, synthetic data can be a powerful alternative for training robust models.
The report also looks into the issue of model performance disparities across different demographic groups. It's a tough topic, and it's good that they're not shying away from it. It's clear that we need to do more to address biases in training datasets to ensure fairness in AI systems.
I was also intrigued by the focus on model explainability and user trust. They're suggesting that these are critical aspects of system design, especially in domains like healthcare where ML decisions have significant impacts. It's a good reminder that AI shouldn't be treated as a black box; we need to be able to understand how it works and why it makes certain decisions.
I think it's great that they're trying to make the findings in the report more readily accessible. They're developing API implementations that allow developers to integrate the insights into their workflows. This could lead to faster adoption of research findings in real-world applications.
And finally, the report also addresses the issue of adversarial training. This is a technique designed to enhance model robustness against attacks that are specifically designed to deceive neural networks. It's a crucial area of research, especially as we see more and more cases of AI systems being exploited.
Overall, I found the latest DeepMind Quarterly ML Progress Report to be a really valuable resource. It provides a snapshot of the key trends and challenges in machine learning, and I think it's something that every ML professional should be aware of.
7 Essential Resources for Tracking Machine Learning Advances in 2024 - GitHub's ML Project Tracker Hits 1 Million Repositories
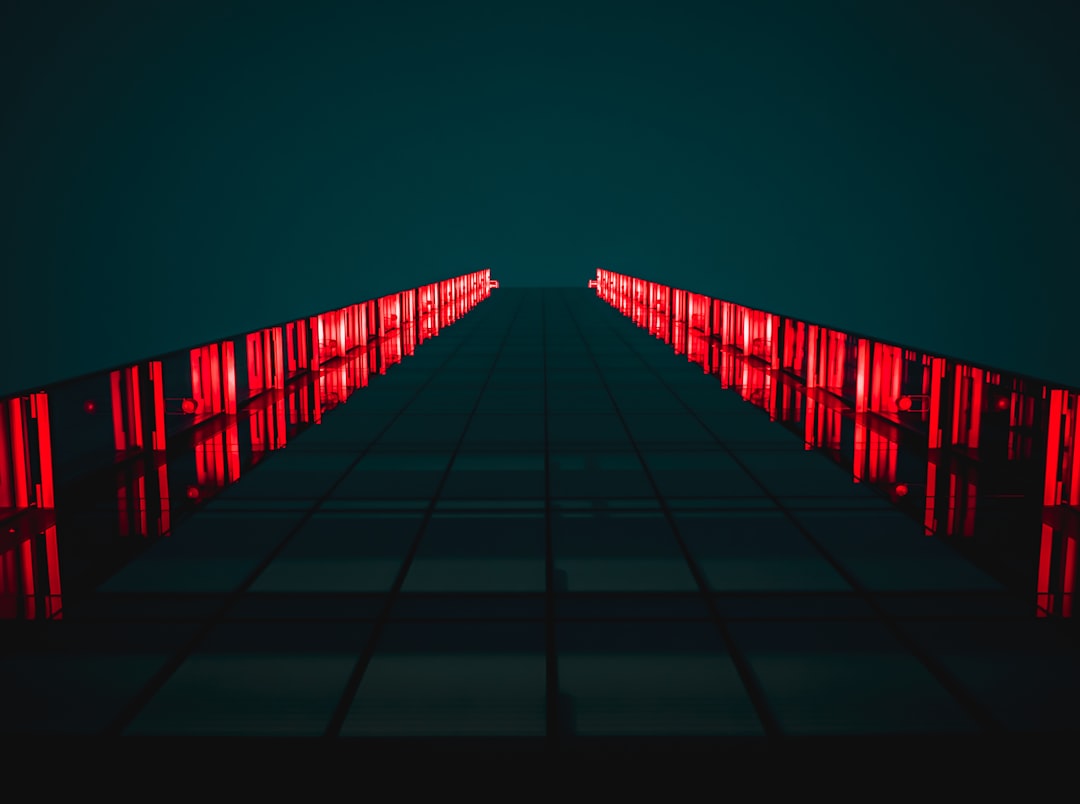
GitHub's ML project tracker has hit a significant milestone, surpassing one million repositories. This reflects a big shift in the way people are working on machine learning projects. It shows how much interest there is in sharing and collaborating on these projects. With so many active users, GitHub has become a kind of central hub where developers and researchers can find and connect with each other. They're using GitHub to track trends in ML, share their code, and even build entire frameworks. It's not just about the quantity of projects, though. It's the quality and complexity of the projects that are really catching attention. The fact that we're seeing this much activity on GitHub shows that machine learning is becoming more complex and more collaborative. As this field keeps growing, GitHub is going to play a big role in shaping its future.
GitHub's ML Project Tracker has hit a significant milestone – one million repositories. This is a big deal, showing just how much interest there is in machine learning projects. It seems like everyone is getting involved, from seasoned researchers to aspiring engineers.
It's interesting to see how the type of projects being shared has changed. There's a lot more focus on ethical AI, bias detection, and interpretability. People are realizing that machine learning isn't just about building models; we need to consider how they impact society as well.
I'm also seeing more projects on data visualization and exploratory data analysis. It's all about making sense of these complex models, making them easier to understand for everyone, not just the experts.
With so many projects out there, it's almost overwhelming. GitHub is using automated tagging systems to help people find what they need, but it's tricky to keep up with all the latest developments. It's like the whole field is evolving so quickly.
And of course, with so much activity, we need to make sure the quality is there. Not every project is going to be top-notch. It's great to have all these projects out there, but we need to have some sort of standard to ensure they're reliable and useful.
Analyze any video with AI. Uncover insights, transcripts, and more in seconds. (Get started for free)
More Posts from whatsinmy.video: