The Enduring Relevance of GANs in AI Research A 2024 Perspective
The Enduring Relevance of GANs in AI Research A 2024 Perspective - GANs Origins and Core Framework
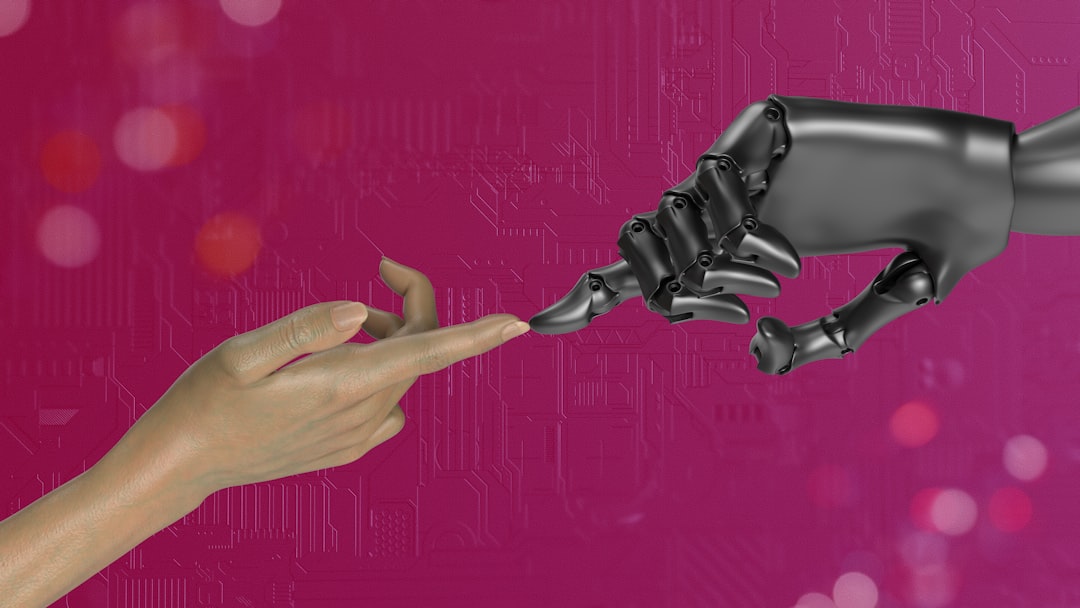
Generative Adversarial Networks (GANs), introduced in 2014 by Ian Goodfellow and his team, represent a landmark development in the realm of generative modeling. This approach, which hinges on the interplay of two fundamental components – a data-generating generator and an authenticity-evaluating discriminator – has unlocked significant advancements in areas such as computer vision. The adversarial training mechanism, which functions akin to a Minimax game, has yielded remarkably realistic outputs. However, while impressive, GANs grapple with inherent challenges, including the phenomenon of mode collapse, where the model prioritizes a limited range of outputs, and training instability. These issues can undermine their effectiveness. The focus of current GAN research is on incorporating human input and feedback to foster a more collaborative process between humans and machines, ultimately enhancing the alignment of generated outputs with human understanding and creative intent.
Generative Adversarial Networks (GANs), introduced in 2014 by Ian Goodfellow and his team, shook things up in the world of AI by offering a novel approach to generating data. The core of GANs is the interplay between two neural networks, a generator and a discriminator. The generator aims to create data that mimics the real deal, while the discriminator is tasked with telling the difference between genuine and synthetic data. They're trained simultaneously, pushing each other to get better - it's a sort of AI arms race!
This adversarial setup has yielded remarkable results, particularly in generating high-quality images, which was demonstrated as early as 2016. However, the "mode collapse" issue remains a concern. This happens when the generator starts churning out the same few types of output, despite being trained on a diverse dataset. This highlights the challenge of ensuring diversity in generated data, something that researchers are continuously working on.
Beyond image generation, GANs have shown promise in fields like data augmentation. In domains with limited datasets, GANs can generate additional samples to improve the training of other models. We're seeing this in areas like facial recognition and medical imaging.
GANs are also becoming more flexible. Conditional GANs take things a step further by allowing us to generate data based on specific inputs. This opens doors for applications like image-to-image translation, where a network can transform one image into another.
Though powerful, GANs aren't without their training challenges. Training instability is a recurring issue, and researchers are exploring different techniques like Wasserstein GANs to address this. Even finding the right balance between the generator and discriminator can be crucial. If one network becomes too dominant, it can stifle the entire training process.
The beauty of GANs is their accessibility, which has spurred a wave of interest both in academia and industry. This has resulted in numerous extensions and variations of the initial framework, with the ultimate aim of making GANs even more powerful and adaptable. The future of GANs seems bright, with researchers continuously finding new ways to refine them and unlock their potential in diverse domains.
The Enduring Relevance of GANs in AI Research A 2024 Perspective - Evolution of GAN Applications Since 2014
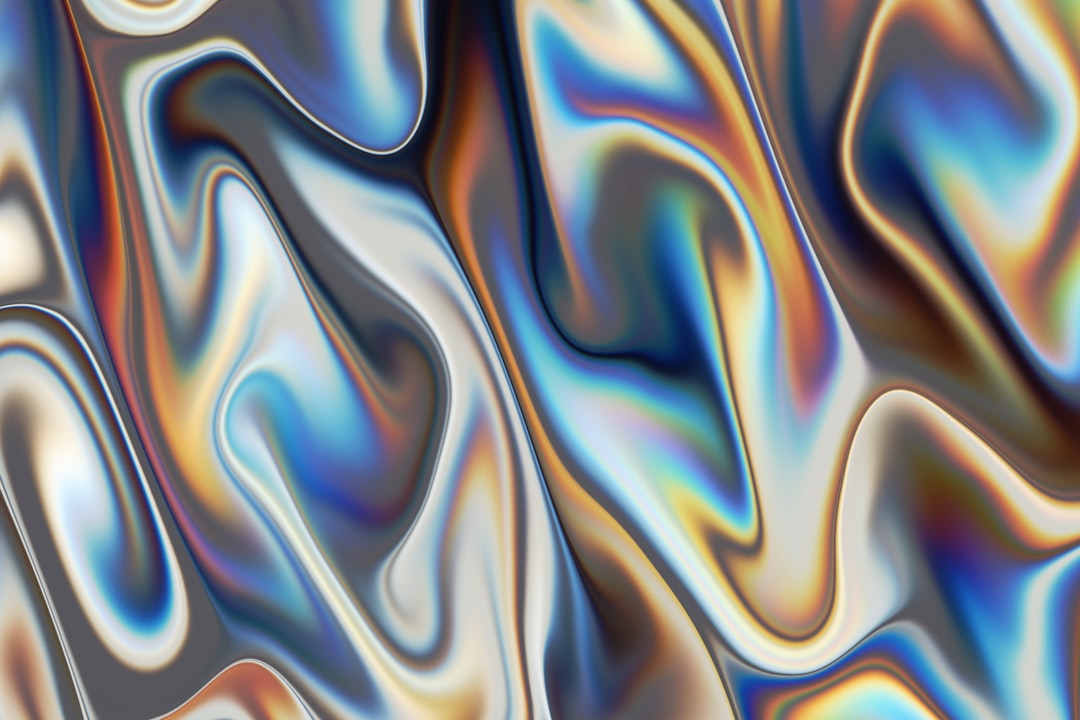
Since their introduction in 2014, Generative Adversarial Networks (GANs) have revolutionized the way we approach data generation in artificial intelligence. The initial GAN architecture, with its two competing networks – the generator and the discriminator – sparked a wave of innovation. Researchers quickly recognized GANs' potential to create realistic data, particularly images, which led to their widespread use in fields like computer vision.
The journey, however, hasn't been without its challenges. While GANs have produced impressive results, the persistent issue of "mode collapse" - where the generator becomes fixated on generating only a limited range of outputs - remains a concern. Researchers have been working diligently to address this, exploring various techniques to ensure that generated data maintains its diversity.
Despite these hurdles, GANs have found their way into diverse applications, from medical imaging, where they can create synthetic data for training models, to data augmentation, where they can increase the size of limited datasets. The adaptability of GANs has also led to new developments like Conditional GANs, which allow for the generation of data based on specific inputs, making them suitable for tasks such as image-to-image translation.
As we move further into 2024, the evolution of GAN applications continues, demonstrating their enduring relevance in AI research. While the potential for misuse remains a concern, particularly in areas like synthetic media creation, the responsible development and use of GANs hold the key to unlocking their full potential for innovation and progress.
Since their debut in 2014, GANs have steadily evolved, revealing new possibilities and tackling challenging tasks. In 2017, Progressive Growing GANs made their mark by generating high-resolution images, gradually building up detail from lower resolution starting points. This led to more stable training and better quality outputs. The same year, CycleGAN showcased the power of transforming images across different domains without requiring paired examples. Imagine turning a photo of a cat into a photo of a dog, all without needing a dataset of matching cat-dog images! This has huge potential in art and style transfer, areas where finding matching data is tough.
Beyond images, GANs are now venturing into video generation. While still in its early stages, we're seeing models capable of generating coherent sequences of frames, opening up a world of dynamic content creation.
The reach of GANs extends even further. Researchers have started using them in drug discovery, generating novel molecular structures that could lead to new pharmaceuticals, promising to accelerate and make the drug discovery process more cost-effective.
Another exciting development came in 2020 with the arrival of StyleGAN2. This improvement allows users to manipulate specific attributes of generated images, like changing the hair color or eye shape of a portrait. This fine-grained control opens up creative doors in fields like fashion and digital art.
But it’s not just about visuals. GANs have shown their versatility in audio synthesis, particularly in music generation. They’re capable of creating complex soundscapes and musical compositions, proving that their talents extend beyond the visual realm.
However, this impressive progress has also raised ethical concerns. The emergence of Deepfake technology, which utilizes GANs for realistic video manipulation, has sparked important discussions about the potential misuse of synthetic media in society. It underscores the critical need for responsible use and a clear understanding of the implications of this powerful tool.
Beyond generating synthetic data, GANs are proving useful in unsupervised learning. They can learn valuable representations from data without the need for labels, opening up new possibilities in various machine learning tasks.
Even in astronomy, GANs are making their mark. They’re being used to enhance images of celestial bodies and improve the quality of data from telescopes, demonstrating their cross-disciplinary potential.
As we move into 2024, GANs are not confined to just image or video generation. They are being increasingly used to generate tabular data, which can be helpful in addressing data imbalances and augmenting datasets in areas like finance and healthcare where gathering real data is often challenging.
Despite their impressive capabilities, challenges remain. While the adversarial training approach is powerful, it can lead to instability. Researchers are continuously working on improvements to address these challenges and enhance the robustness and versatility of GANs.
The Enduring Relevance of GANs in AI Research A 2024 Perspective - Recent Breakthroughs in GAN Technology
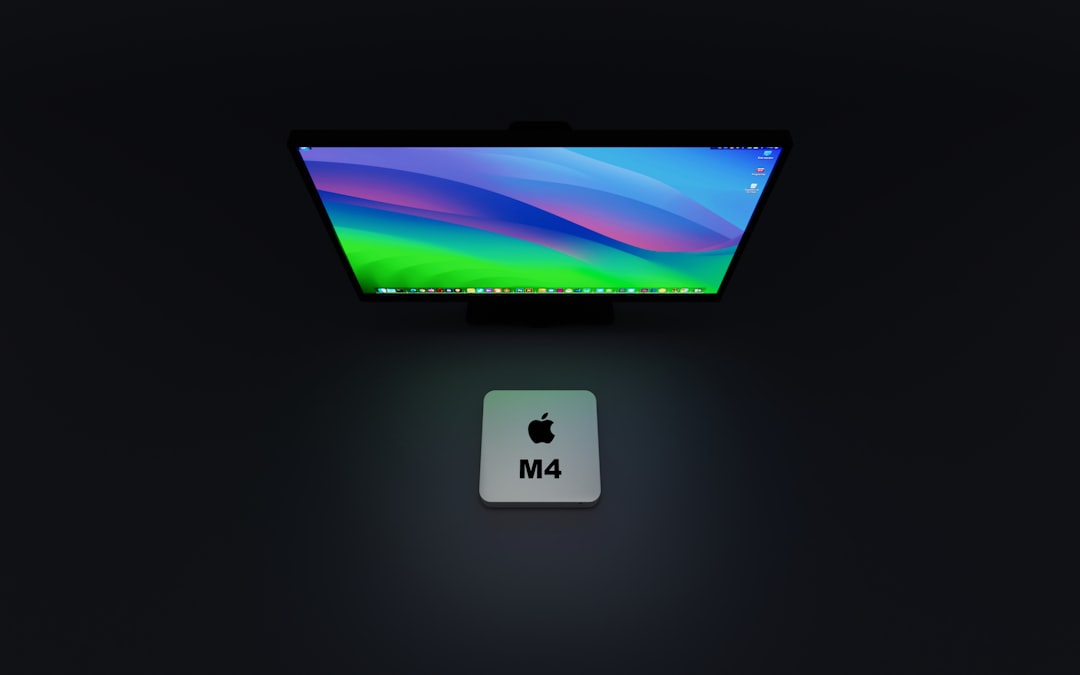
Recent breakthroughs in GAN technology demonstrate their ongoing evolution and potential. NVIDIA's StyleGAN3, for instance, has surpassed its predecessor, generating images with even higher realism and diversity, while tackling long-standing problems like artifacts. GANs are no longer limited to visual domains, showing promise in video creation, sound synthesis, and even drug discovery. Their adaptability across diverse areas is remarkable. However, challenges persist, particularly training instability, and the ethical concerns surrounding the potential for misuse in areas like synthetic media. While pushing the limits of what GANs can achieve, researchers are also grappling with the complex and critical aspects of their development and application.
The field of Generative Adversarial Networks (GANs) is constantly evolving, pushing the boundaries of what's possible with AI-powered data generation. While we've seen impressive progress in image generation and beyond, recent developments are particularly intriguing.
One exciting area is the emergence of multi-modal GANs. These networks can generate data across different formats, like images and text descriptions, simultaneously. This opens up new applications for tasks like scene understanding and content creation.
Researchers have also cracked some of the challenges that plagued early GANs, like the issue of "mode collapse." Innovative techniques, including regularization and architectural modifications, have improved the diversity and quality of generated outputs.
Another exciting development is the ability to integrate self-supervised learning into GANs. This allows these models to leverage unlabeled data, making them more efficient and adaptable to a wider range of tasks without relying heavily on labeled datasets.
The world of video generation is also seeing significant advancements, with GANs now producing short video clips that are temporally coherent, meaning they maintain logical flow across frames. This paves the way for applications like animation and video synthesis, which require realistic and engaging outputs.
While GANs have primarily been associated with visual content, they are now being adapted for text generation as well. Recent research has shown that GANs can create coherent and contextually relevant narratives, opening new doors for applications like story generation and dialogue systems.
As these developments continue to unfold, the potential for GANs in various fields, including augmented reality, drug discovery, and 3D object generation, is only getting more exciting. Despite their impressive progress, challenges remain. Researchers are working to improve the robustness and stability of GAN training, ensuring that these powerful tools are reliable and effective. The future of GANs is bright, promising to unlock even more creative and innovative possibilities in the world of AI.
The Enduring Relevance of GANs in AI Research A 2024 Perspective - Challenges Facing GAN Research in 2024
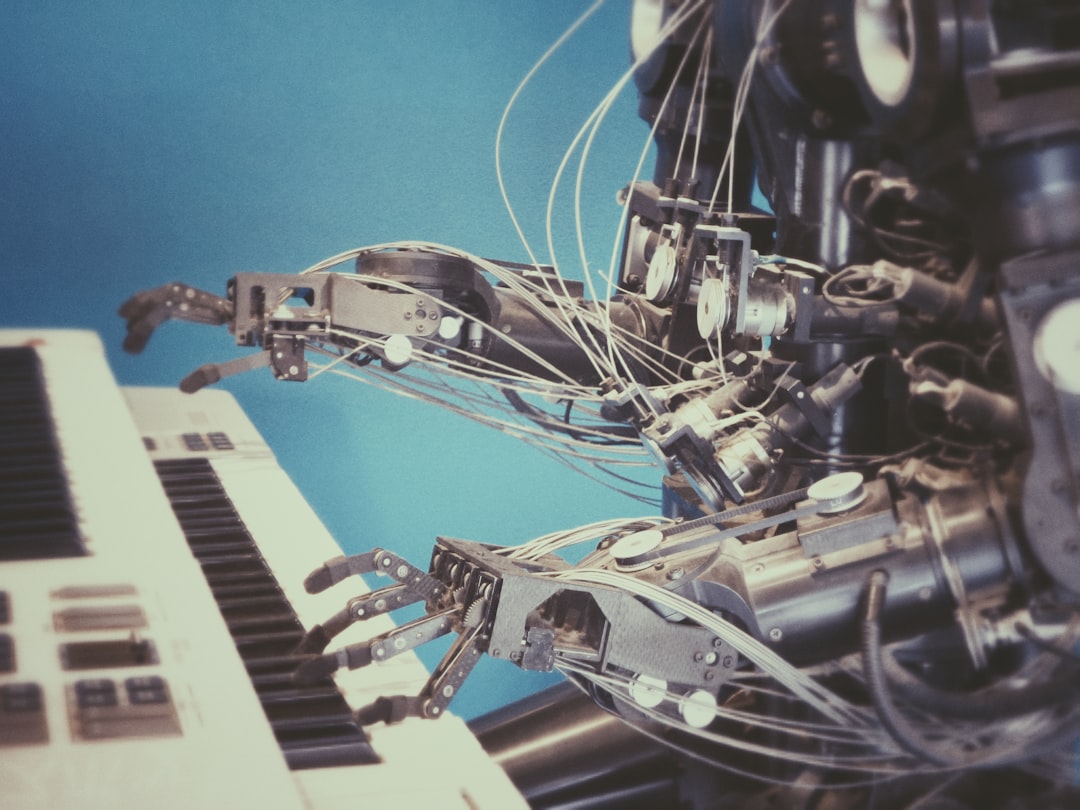
As we enter 2024, the world of Generative Adversarial Networks (GANs) continues to evolve at a rapid pace, but not without its fair share of obstacles. While GANs have shown remarkable progress in areas like image generation and beyond, researchers still face hurdles like training instability, which can significantly impact a model's effectiveness. The issue of mode collapse also persists, often leading to a lack of diversity in the generated data.
The search for solutions is ongoing. Researchers are experimenting with new GAN architectures and strategies, striving to address these shortcomings. There's a growing interest in incorporating human feedback into the training process, aiming to refine the generated outputs and ensure they better align with human understanding and expectations.
Despite these challenges, the pursuit of stability and diversity in GAN applications underscores the immense potential and the ongoing challenges within this rapidly developing field of AI research.
GANs are proving to be a powerful tool in AI research, but even as they evolve and become more sophisticated, there are some fundamental challenges that remain. One of the most persistent issues is the so-called "gradient penetration" problem. In essence, the gradients for the generator can fade away during training if the discriminator gets too good, making it hard for the generator to learn. This emphasizes the delicate balance between these two networks during training.
Adding to this complexity is the issue of hyperparameter sensitivity. GANs can be quite finicky, requiring meticulous adjustments to training parameters like learning rates and batch sizes. Even small changes can significantly impact performance, making it a challenge to achieve stable training and predict outcomes.
Another issue is the lack of a standardized metric for evaluating GAN performance. While metrics like Inception Score and Fréchet Inception Distance exist, they are not universally accepted, making it difficult to consistently assess model quality and improvement across research.
Another challenge is that GANs can overfit to training data, especially when working with small datasets. This can lead to models generating very similar outputs instead of diverse, creative examples. While researchers are exploring ways to mitigate overfitting, it remains a significant hurdle.
Training times are another obstacle. GANs often require lengthy training periods, sometimes even weeks, which can limit their practical applications, especially when rapid deployment is needed for real-time applications.
Furthermore, the loss functions used in GANs can be complex and difficult to balance. This can lead to scenarios where one component dominates the training process, hindering the cooperative learning between generator and discriminator.
Additionally, GANs often struggle to generalize to unseen domains. They may excel in a specific dataset, but applying them to different scenarios can be challenging. This is why researchers are working on developing more adaptable models.
It is also essential to recognize that the quality and diversity of training data significantly influence GAN performance. However, finding minimally biased and varied datasets can be challenging, especially in specialized fields.
Another crucial consideration is the ethical implications of output control. GANs can generate highly realistic synthetic data, which opens up concerns about potential misuse, like creating misinformation or deepfakes. This highlights the need for guidelines and robust monitoring systems to prevent harm.
Finally, it's important to recognize that the rapid innovation within GAN research depends on a strong collaboration between academia and industry. This interplay is vital for addressing existing challenges and exploring new applications, yet it also creates variability in how advancements are prioritized and developed.
Despite these challenges, the potential of GANs is undeniable. As researchers continue to work on solutions, GANs will undoubtedly become more robust and reliable, expanding their reach and impact in AI and beyond.
The Enduring Relevance of GANs in AI Research A 2024 Perspective - GANs vs Other Generative AI Models
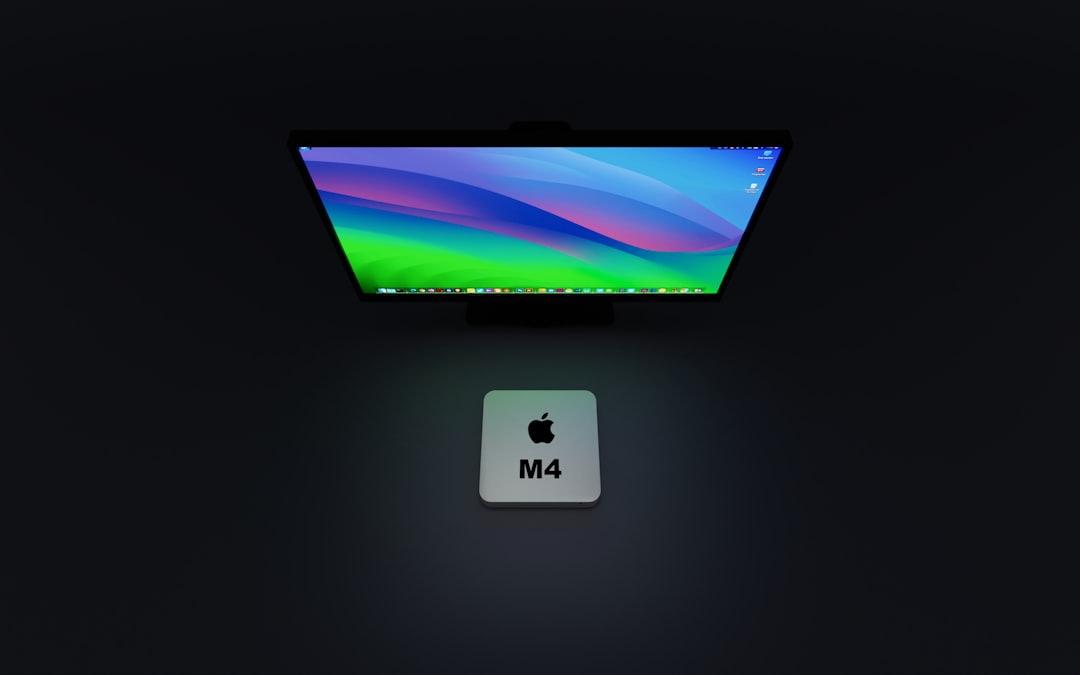
Generative Adversarial Networks (GANs) stand apart from other generative AI models like Variational Autoencoders (VAEs) and diffusion models. GANs are known for creating highly realistic images and multimedia content, but face challenges like unstable training and a tendency to produce limited types of output, hindering diversity. In contrast, VAEs excel in signal analysis and can work well with limited data, making them suitable for specific tasks. Diffusion models, on the other hand, consistently generate high-quality outputs, but require considerable computational power and time. As AI research progresses, it's crucial to weigh the pros and cons of each model and ensure that each plays its part in advancing different applications.
GANs, despite their impressive achievements in generative modeling, face numerous challenges that keep researchers on their toes in 2024. One persistent issue is the dynamic tension between the generator and discriminator. When the discriminator gets too good, the generator struggles to learn, resulting in a phenomenon called 'gradient vanishing'. Other generative models like VAEs bypass this by taking a different approach to learning data representations, avoiding the adversarial tug-of-war.
Another hurdle is 'mode collapse,' a situation where the generator becomes stuck generating only a limited range of outputs. Interestingly, generative models like normalizing flows manage to maintain greater diversity in their outputs by employing invertible transformations that prevent output distributions from becoming too concentrated.
Assessing the performance of GANs is also a bit of a head-scratcher. While the common FID metric is a helpful tool, it's mainly focused on image quality, leaving other generative tasks underserved. Models like VAEs, on the other hand, often have more straightforward loss functions that directly measure data likelihood, allowing researchers to more easily track improvements.
The adversarial approach inherent in GANs often leads to volatile training outcomes, requiring meticulous fine-tuning of hyperparameters and architectures. In contrast, models like diffusion models display more stable training behavior, producing high-quality outputs with less sensitivity to initial settings.
Training a GAN can also be computationally expensive and time-consuming, sometimes taking weeks to converge. Models like autoregressive transformers, in contrast, often require less computing power for similar tasks, allowing for quicker iterations and faster development cycles for new applications.
Beyond 'mode collapse', generated data can also exhibit undesirable biases inherited from the training data. Flow-based models, with their robust ability to condition generation on multiple aspects of the data distribution, have shown promise in mitigating these issues, delivering higher output diversity and quality.
While GANs have made great strides in image generation, other models, like diffusion models, have surpassed them in generating high-fidelity images with fine-grained control over attributes, making them a more popular choice for certain applications.
GANs have also shown great promise in areas like medical imaging and art generation, but their misuse in media landscapes raises serious ethical and regulatory concerns. Other generative models can be developed with ethical considerations baked into their architecture and training protocols, paving the way for more responsible deployment.
Although GANs have sparked numerous variations and extensions, they often require extensive retraining for specific tasks. Models like VAEs and autoregressive models, on the other hand, can adapt to new tasks more readily through transfer learning, making them more appealing for developers aiming to quickly apply existing models to new applications.
The challenges facing GAN research are a testament to their powerful potential and the rapid pace of progress in AI research. As researchers continue to refine GANs, we can expect to see even more groundbreaking developments in the world of generative AI.
The Enduring Relevance of GANs in AI Research A 2024 Perspective - Future Directions for GAN Development
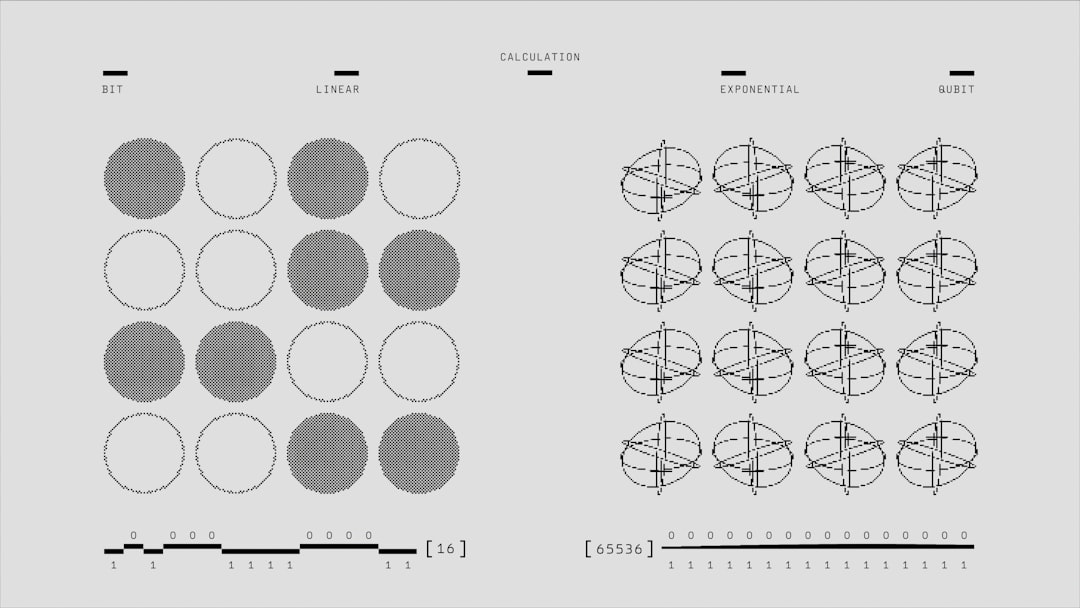
Looking ahead in 2024, Generative Adversarial Networks (GANs) hold significant promise, yet face significant obstacles. The focus of current research is on refining GAN architectures to improve stability, diversity, and control over the output. Researchers are also exploring ways to incorporate self-supervised learning and human feedback into the training process. Meanwhile, the emergence of newer deep learning technologies like diffusion models and Transformers is prompting GANs to adapt and integrate with new methodologies. This evolution is pushing researchers to explore novel applications across a wide range of domains, aiming to uncover both the potential and limitations of GANs in practical scenarios. However, as GAN technologies continue to mature, ethical considerations and the potential for misuse remain critical concerns that must be carefully addressed.
GANs have come a long way since their 2014 debut. They're not just about images anymore - now they're tackling videos, text, even sound! The way they're trained is getting smarter too. We're seeing more stable training through regularization, and they can even learn from unlabeled data, making them more versatile. There's a big push to make them faster, which would be a game-changer for real-time applications like games or interactive media.
But, the more powerful GANs become, the more ethical questions arise. How do we prevent them from being used for misinformation, especially as they get better at making things look and sound so real? And, while they're getting good at generating different kinds of data, they're also very sensitive to the training data. We still need to figure out how to make them less susceptible to bias, which is a big concern when using them in fields like medicine or finance.
Looking beyond image generation, GANs are opening up new avenues in areas like drug discovery, where they're helping researchers to design new molecular structures. It's exciting to see their potential reaching beyond art and entertainment and into the world of scientific advancement.
GANs are definitely changing the game, but there's still a lot of work to be done to ensure they're used ethically and responsibly, and to make them truly reliable in all their applications.
More Posts from whatsinmy.video: