Analyze any video with AI. Uncover insights, transcripts, and more in seconds. (Get started for free)
7 Essential Skills for Aspiring Data Annotators in 2024
7 Essential Skills for Aspiring Data Annotators in 2024 - Mastering Annotation Tools and Software
In the dynamic landscape of data annotation, proficiency in using a variety of tools and software is paramount. It's not simply helpful, but crucial for achieving high-quality results. This is especially true when working with visual data like images and videos, where accurate labeling and markup are critical. The rise of AI has further emphasized the importance of using annotation tools effectively, as the quality of training data directly impacts the performance of AI models.
The field of data annotation is constantly evolving, meaning that continuous learning and keeping abreast of the latest software and techniques is a necessity for aspiring annotators seeking to thrive. Proper training programs that focus on the specific tools and techniques used in the industry are invaluable for developing annotators who can meet the challenges and demands of this fast-paced sector. Understanding the proper usage of these tools enables annotators to deliver superior data, playing a critical role in the growing reliance on AI and machine learning.
Gaining mastery over the specific tools and software used in data annotation is essential for any aspiring annotator. The effectiveness of these tools can significantly impact the quality of the final dataset, ultimately affecting the performance of machine learning models trained on that data. For instance, researchers have shown that meticulously labeled data can lead to a considerable increase in accuracy in the models, demonstrating that the tools themselves are critical components in the overall process.
Collaboration within annotation projects is often facilitated through various software features. Many platforms include features allowing for simultaneous editing and feedback, potentially streamlining the process of preparing larger datasets. This type of functionality becomes increasingly valuable when the annotation workload is high, such as for training cutting-edge AI models.
Moreover, a key development in annotation software is the emergence of built-in quality control mechanisms. Many platforms now incorporate automated checks and redundancy systems, aiming to reduce human error by identifying inconsistencies and errors in labeling before the dataset is finalized. These are crucial components in ensuring data integrity, something that's particularly important as the scope and complexity of AI models have grown.
The design of the user interface is another critical aspect for any annotation tool. A well-designed interface can reduce the time needed for annotators to complete their tasks, allowing for greater productivity and potentially speeding up the entire labeling process. Yet, user interface design is only one piece of the broader efficiency puzzle. The increasing use of hybrid systems that combine human annotators with automated annotation tools highlights the limitations of purely automated approaches while suggesting a way forward. While these automated tools can certainly assist annotators, they can't always replace a human's judgment and adaptability when confronted with complex or ambiguous data.
Another noteworthy trend is the increased adoption of tools that handle multiple data types. Instead of separate tools for image, text, or audio annotation, we are seeing increased popularity of platforms that provide these features within a single interface. This can lead to significant time and resource savings in complex annotation tasks, particularly for projects dealing with multimodal data or requiring the integration of diverse data sources.
Furthermore, annotation platforms are exploring creative ways to maintain user engagement and motivation. The implementation of gamification elements, such as points or badges, is becoming more common, which can serve to make the annotation process more engaging and productive for individuals. The impact of these seemingly simple design choices suggests that there's more to annotation tool design than meets the eye, with software developers increasingly focusing on the psychology of user engagement in their design decisions.
It's also important to consider the increasing awareness of the legal and ethical aspects of working with data. The handling of sensitive data and compliance with regulations like the GDPR is a growing consideration in annotation tool development. Tools that support robust data security and user privacy protections are increasingly in demand as society becomes more conscious of the impact of data on individuals.
A further trend involves the development and use of open-source annotation tools. These tools allow for increased customization and flexibility, enabling specific features to be tailored to the exact requirements of a project. However, this approach also presents certain challenges and risks that will need to be carefully assessed and mitigated.
Finally, collaborations between researchers and annotation tool developers are becoming increasingly important. These partnerships foster innovation, introducing new features and functions based on empirical evidence and insights gathered from a variety of sources. This synergy between practical application and research is likely to continue, potentially contributing to new and more efficient annotation tools in the years to come.
7 Essential Skills for Aspiring Data Annotators in 2024 - Developing Sharp Attention to Detail
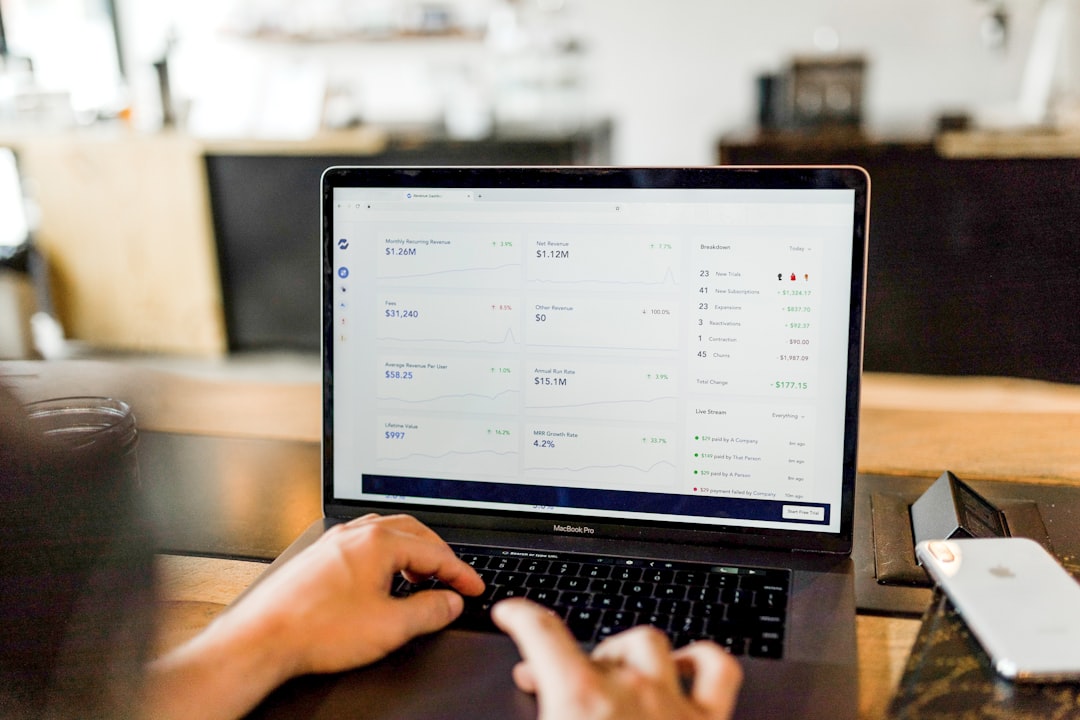
Developing sharp attention to detail is fundamental for aspiring data annotators. The accuracy of their work directly impacts the quality of datasets used to train AI and machine learning systems. In this field, even seemingly minor errors can have a substantial effect, emphasizing the need for a thorough and careful approach to data review and analysis. To cultivate this skill, it's beneficial to employ strategies like dedicated training that focuses on developing concentration, and incorporating regular breaks into routines. These breaks not only combat mental fatigue but also improve the ability to notice details. As datasets become more complex, the ability to discern subtle distinctions will become crucial, allowing annotators to align their efforts with project specifications and ultimately improve the quality of the AI training data they produce. It's a skill that requires consistent effort, and annotators who master it can significantly enhance their value within the field.
Developing sharp attention to detail is fundamentally important for data annotators, as it directly impacts the accuracy and quality of the training data used in AI and machine learning. While we've already explored the importance of tools and software in achieving this, the human element remains central. Research suggests a link between attention to detail and improved cognitive functions, including problem-solving and overall mental agility. This makes sense when you consider that the brain regions associated with detailed thinking, like the prefrontal cortex, are also involved in complex decision-making.
In the context of data annotation, this translates into better performance. Studies have shown that individuals with strong attention to detail tend to deliver higher-quality work, which ultimately benefits the AI models being trained on the data. This quality isn't simply inherent though, it can be nurtured. Techniques like "chunking," where information is broken into smaller, more manageable pieces, have been shown to enhance the ability to focus on the fine details, a critical element of successful annotation.
However, the human mind isn't naturally wired for perfect attention to detail. We often experience "inattentional blindness," a phenomenon where we miss obvious things due to our limited focus. Training environments that actively combat this tendency, through specific exercises and feedback, can help mitigate these natural limitations. Furthermore, our emotional state plays a role. Positive moods seem to sharpen our focus, while stress can negatively impact our ability to attend to detail, particularly under pressure.
Beyond cognitive factors, personality traits can also influence an individual's aptitude for detailed work. Conscientious individuals, who tend to be organized and thorough, are often better suited for roles demanding meticulousness like data annotation. Techniques like mindfulness might also help improve concentration and resilience to distractions, thereby enhancing attention to detail. Given the nature of multistep annotation tasks, developing strong pattern recognition abilities is another aspect of enhancing overall attention. The ability to quickly discern subtle patterns and inconsistencies in data can significantly improve speed and accuracy.
Finally, the importance of avoiding fatigue can't be overlooked. Prolonged periods of annotation work can diminish attention span and impair accuracy. Therefore, incorporating regular breaks into annotation workflows is essential to ensure consistency and high quality. By recognizing the various factors that impact our ability to pay attention to detail, data annotators can cultivate these skills to deliver exceptional work that's crucial for building reliable and effective AI systems.
7 Essential Skills for Aspiring Data Annotators in 2024 - Cultivating Strong Time Management Skills
In the fast-paced environment of data annotation in 2024, mastering time management is crucial for aspiring professionals. Being able to effectively prioritize tasks, deciding what's most urgent and important, is key to keeping up with the demands of the job. Additionally, staying organized is vital. It allows annotators to handle a heavy workload without feeling overwhelmed and ultimately contributes to producing consistently high-quality results. The field is ever-changing, so staying current with the latest tools and methods is a must, while also making sure you're laser-focused on accurate annotation. By developing strong time management habits, data annotators can adapt to the evolving demands and complex projects within the industry. It's a skill that's not just helpful, but becoming increasingly necessary to succeed.
In the realm of data annotation, effectively managing time is not just beneficial, it's essential. Data annotators often operate under tight deadlines and need to ensure the timely delivery of high-quality work. This involves making discerning decisions about which tasks to tackle first, a skill known as prioritization. Prioritization, in essence, helps annotators navigate the deluge of annotation tasks, deciding what's most pressing and critical at any given moment. It's like a conductor leading an orchestra of tasks, ensuring that the most important elements receive the appropriate attention.
While prioritizing tasks is critical, it's equally important to maintain a high degree of precision and accuracy. Attention to detail is fundamental for delivering accurate annotations. Annotators need a keen eye to spot even subtle discrepancies in the data, making sure that every element is labelled appropriately. These precise labels are not just decorative, they feed directly into the core of AI and machine learning systems, acting as the raw material for model development. The tiniest oversight can lead to unforeseen consequences down the line, impacting the reliability and accuracy of AI systems.
Beyond meticulousness, data annotators often face situations where they need to analyze information carefully and make well-informed decisions. Critical thinking skills become essential in the annotation process. This involves considering diverse perspectives, assessing the validity of various pieces of information, and understanding the potential consequences of different labeling choices. This critical perspective ensures annotators are not simply following pre-determined guidelines, but actively engaging with the data and contributing their informed judgments.
The landscape of data annotation is in a constant state of flux. New tools, techniques, and applications are constantly emerging. To maintain a competitive edge, continuous learning is essential. This involves keeping up with the latest trends in the industry, understanding emerging software, and proactively developing new annotation abilities. For data annotators, learning is not just about professional development, it's about staying relevant and making sure their skills are aligned with industry expectations.
Effective communication skills are also increasingly important. Data annotators frequently need to explain technical concepts to colleagues, stakeholders, or even researchers working with the data. The ability to clearly articulate complex ideas and collaborate with others ensures smoother project workflows and allows for greater transparency in the annotation process. This shared understanding reduces the chances of misinterpretations and supports efficient problem-solving within a team.
Finally, it's imperative for data annotators to be adaptable and resourceful in addressing challenges. This includes having solid problem-solving and numerical skills. Annotators may encounter unexpected issues, need to analyze patterns in the data, or troubleshoot inconsistencies. Strong problem-solving abilities enable them to think creatively and come up with solutions that maintain project quality and adhere to timelines. This adaptability and resourcefulness will become even more crucial as AI systems grow in complexity, demanding increasingly nuanced and refined annotation datasets.
Beyond these foundational skills, a practical understanding of the various techniques involved in image and video labelling is essential. This could involve identifying specific objects within a frame, annotating their attributes, or even tracking the movement of objects over time. Proficiency in these specific labelling methods lays the groundwork for annotators to work efficiently and accurately in the field.
Organizing the vast amounts of data and tasks involved in annotation projects can be challenging. That's where strong organizational skills come into play. By developing the ability to efficiently manage their workload, annotators can reduce stress, prioritize effectively, and ensure that projects stay on track. It's like having a well-organized toolbox – each tool readily accessible for the task at hand.
Furthermore, an understanding of data visualization tools can be incredibly valuable. These tools help to present and analyze data in a clear and meaningful way. Data visualization not only enhances comprehension but also allows for quicker detection of anomalies or errors during the annotation process.
The ability to manage time, prioritize tasks, and stay attuned to the constantly evolving landscape of AI and machine learning are all crucial elements for aspiring data annotators. It’s a dynamic field that rewards initiative, attention to detail, and continuous learning. These 10 essential skills provide a roadmap for those eager to contribute to the ongoing development of AI and machine learning systems.
7 Essential Skills for Aspiring Data Annotators in 2024 - Building Expertise in Specific Data Domains
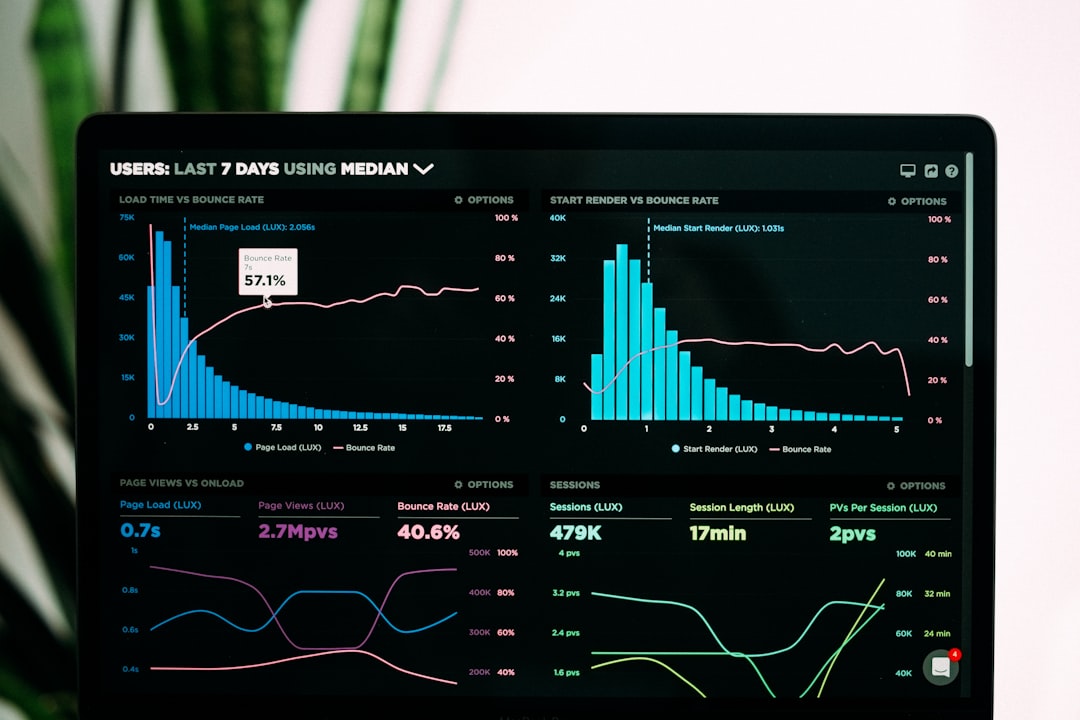
Developing expertise within specific data domains is vital for aspiring data annotators. As the data landscape becomes increasingly complex, possessing a thorough understanding of the intricacies of particular fields – be it healthcare, finance, or other sectors – allows annotators to create more precise annotations that align with the specific requirements of each domain. This specialized knowledge not only enhances their analytical capacities but also improves their ability to tackle complex problems, making them more valuable collaborators within multi-disciplinary teams. Moreover, staying abreast of emerging trends and technologies in their chosen area allows annotators to adapt to evolving demands and contribute more effectively to annotation projects. In essence, this specialized knowledge elevates the quality of annotation output, resulting in more dependable data for the development and implementation of AI and machine learning systems.
When it comes to data annotation, simply knowing how to use the tools isn't enough. Developing a deep understanding of specific data domains is becoming increasingly important for aspiring annotators. It's not just about boosting efficiency—though it can significantly increase productivity by as much as 50% by allowing annotators to make faster and more accurate judgments. It's also crucial for creating datasets that produce better AI and machine learning models, with studies showing a potential 30% improvement in accuracy when domain experts are involved.
The applications of this specialization are wide-ranging. Healthcare, finance, and the growing field of autonomous vehicles all have their own unique requirements and data types, each demanding a specialized approach to annotation. Medical images are vastly different from financial transaction records, and annotating them requires unique knowledge and skills.
One of the key benefits of domain expertise is in cognitive load management. Annotators familiar with the intricacies of a field can sift through data more effectively, focusing on the truly important elements without getting bogged down in irrelevant noise. This heightened focus is a direct result of possessing a mental model for how the data is structured and the context in which it's generated.
Surprisingly, this expertise can often translate across domains. Many of the core principles of annotation—paying close attention to detail, maintaining precision, and understanding the importance of context—are transferable. This creates valuable career opportunities for individuals who develop strong domain expertise in one area.
Annotators can further enhance their abilities by actively participating in domain-specific communities. These communities are a rich source of insights, best practices, and awareness of common pitfalls that can help to elevate an annotator's quality of work. In addition, it's critical to recognize the legal and ethical dimensions associated with different data domains. For instance, understanding HIPAA regulations in healthcare or GDPR in other areas can prevent serious mistakes and ensure compliance, positioning an annotator as a vital and responsible team member.
Some domains may demand expertise in specialized software or platforms. Geospatial data might require familiarity with geographic information systems (GIS), while health data could involve working with electronic health record (EHR) systems. These contextual knowledge and technological skills are vital for leveraging domain-specific software effectively, leading to faster and more accurate annotation.
Domain expertise often involves collaborative work with researchers, data scientists, and other specialists. This interdisciplinary collaboration can result in innovative annotation solutions and approaches, enriching the process for everyone involved.
Finally, as with any field, data domains are continually evolving. This means that annotators need to embrace lifelong learning. Staying current with advancements and trends will make them more valuable contributors to their teams and projects. The landscape of data annotation is dynamic and complex, so developing expertise in specific areas is crucial for those wanting to excel in this exciting field.
7 Essential Skills for Aspiring Data Annotators in 2024 - Enhancing Communication and Collaboration Abilities
In the evolving field of data annotation in 2024, effectively communicating and collaborating has become crucial for success. Aspiring data annotators need strong communication skills to clearly explain complex concepts to a wide range of people, including fellow annotators, clients, and others involved in the project. This clarity ensures everyone is on the same page and working towards shared goals.
Additionally, the ability to work well with others is key. Data annotation often involves teamwork, and annotators need to be able to efficiently collaborate with their colleagues. This includes sharing information, providing constructive feedback, and working together to resolve issues that arise. While mastering annotation software and tools remains important, a modern annotator must also develop strong "soft" skills like communication and teamwork. This means not just being technically proficient, but also being able to understand and respond to the needs and perspectives of others. Developing a keen sense of emotional intelligence and the ability to truly listen are aspects of this, and are crucial for building healthy, productive relationships within teams.
Cultivating these abilities is not just beneficial, it's essential for maximizing the accuracy and effectiveness of the annotation process. Strong communication and collaboration ultimately lead to higher-quality data and better outcomes for projects reliant on accurate annotations.
In the intricate world of data annotation, effective communication and collaboration are not mere perks, but fundamental cornerstones for success. The ability to convey complex ideas clearly and succinctly to diverse audiences is a must-have skill. This becomes particularly critical when dealing with stakeholders who may have varied technical backgrounds. Furthermore, collaboration is no longer a luxury, but a core element of most annotation projects, requiring annotators to work harmoniously with team members, clients, and project managers.
It's worth noting that while technical expertise in handling annotation tools and software is essential, equally important are what we often call "soft skills." The capacity to collaborate effectively and communicate complex ideas—both within a team and to those outside the annotation process—is undeniably important.
Problem-solving is another critical ability. Data annotators are often the first line of defense in identifying potential data quality issues. This means asking the right questions, engaging in critical thinking, and working through ambiguous situations. This often requires more than simply following rigid rules, but also creatively applying knowledge to resolve unforeseen obstacles.
Data visualization is a valuable complement to strong communication skills. The ability to intuitively convey data insights through visuals aids—be it graphs, charts, or other means—enhances the overall impact of an annotation project. It's not enough to meticulously label a dataset, the results need to be communicated in a way that informs and helps others make decisions.
A hallmark of the current technological landscape is rapid change. This means adaptability is not simply a desired trait, but a necessity for data annotators to remain relevant. The requirements of annotation projects can shift frequently, meaning that annotators who are flexible and can handle new situations effectively are more valuable in the long run.
Emotional intelligence plays a vital role in these scenarios. Individuals with high emotional intelligence are often better at navigating team dynamics, understanding diverse perspectives, and resolving interpersonal conflicts that can arise during complex projects. This is crucial in collaborative environments, particularly those involving virtual teams, where the subtle nuances of communication can be challenging to interpret.
Tailoring communication to a specific audience is key to its effectiveness. It's not just about speaking plainly, but also understanding the background and expertise of the listener. Annotators who can communicate effectively are not only valuable within the annotation team, but they can also act as a bridge between the technical complexities of their work and the needs of other stakeholders.
The field of data annotation is constantly evolving. As a result, annotators must consistently learn new things. This includes both technical advancements in annotation tools and emerging best practices within the field. This constant need to adapt means that aspiring annotators must view learning not as a chore but as an ongoing pursuit.
Finally, the ability to build connections and networks can help with career advancement. Networking within the annotation field allows annotators to discover new opportunities and learn from others who are facing similar challenges. It's a crucial element of long-term success in any career, and data annotation is no exception.
In conclusion, while the technical skills required to use annotation tools are undeniably important, the ability to communicate effectively, collaborate with others, adapt to change, and continue learning are essential aspects for aspiring data annotators in 2024. The combination of these abilities leads to the production of high-quality annotated data that underpins the further development of AI systems.
7 Essential Skills for Aspiring Data Annotators in 2024 - Understanding AI Ethics and Data Privacy
In today's landscape, understanding AI ethics and data privacy is a vital skill for anyone aspiring to be a data annotator. As artificial intelligence becomes more integrated into our world, the ethical considerations of its development and use are increasingly important. This includes recognizing and mitigating biases present in data that can lead to unfair outcomes. Furthermore, it's crucial to learn how to protect sensitive data through strong security measures. Annotators must also be well-versed in legal requirements related to data privacy, like various laws designed to safeguard people's information. These ethical and legal considerations are essential for ensuring AI technologies are developed and applied responsibly. By developing a strong foundation in AI ethics and data privacy, annotators not only strengthen their individual skillsets but also contribute to the development of AI systems that are both powerful and trustworthy.
The field of AI ethics is about developing and using artificial intelligence in ways that are fair, transparent, and align with our values. This field has been heavily influenced by over a decade of research and partnerships with organizations that handle massive amounts of data, putting a strong emphasis on real-world experience. One of the core principles of AI ethics is avoiding biases in the data that can lead to unfair or discriminatory results.
Data security and privacy are crucial parts of AI ethics, demanding strong safeguards to protect sensitive information used by AI systems. If these systems are compromised, it can lead to the exposure of private details, like patient records in medical applications, making it important to understand the risks.
Fortunately, lots of learning resources are now available on AI ethics and data privacy, including interactive materials that are easy to access on mobile devices. Currently, ethical AI development involves following data privacy laws and regulations and knowing how compliance frameworks work.
However, there are significant challenges around the application of AI ethics in data annotation. A lot of organizations haven't fully grasped the importance of data privacy, even though studies show that a single data breach can cost over $3 million. This includes legal battles, fines, and a loss of public trust. For someone becoming a data annotator, it's essential to have a firm grasp on these security practices.
It's remarkable that around 90% of data can be linked back to a person through unique identifiers when working with personal information in machine learning datasets. This makes strong anonymization techniques all the more critical. Surprisingly, more than 80% of businesses don't have a formal data governance framework in place, which can cause inconsistencies when handling and annotating sensitive data. Without a solid structure, the risks to privacy and compliance rise quickly.
It's often thought that AI operates independently of humans. But data annotators are fundamental to ensuring that datasets are ethically sourced, addressing issues like fairness and bias. Changes in privacy laws around the world have been triggered by the rapid development of AI. For example, Brazil has introduced regulations similar to the GDPR, demonstrating a global shift towards stricter data protection standards. It's vital for annotators working in various locations to stay on top of these changing legal environments.
Psychological research reveals that people are more likely to share their personal information when they believe it's being handled by someone they trust. This is important because ethical practices and transparency are key to the work of data annotators. About 30% of businesses have mentioned problems implementing data privacy rules due to their workers not understanding the technical aspects. This suggests a potential opportunity for aspiring data annotators to apply their expertise and help fill this knowledge gap.
Interestingly, roughly 60% of data breaches happen because of human mistakes rather than external hacking attempts, even with advanced automated data processing. This emphasizes how essential it is for annotators to be careful and knowledgeable about data handling procedures. The concept of "data ownership" is becoming increasingly complicated. A recent survey indicated that 70% of consumers believe they should fully control their data. But many don't understand their legal rights surrounding this. Data annotators can advocate for practices that put more power in the hands of people regarding their information.
Research into ethics in data handling reveals that it significantly increases user engagement, as people are more inclined to connect with brands they perceive as ethical. For those hoping to become data annotators, this indicates that prioritizing ethical practices can lead to better results. Essentially, AI ethics and data privacy are tightly woven into the field of data annotation, and understanding these concepts and their practical implications is critical for aspiring annotators hoping to make a meaningful contribution in this field.
7 Essential Skills for Aspiring Data Annotators in 2024 - Adapting to Emerging Annotation Techniques
The field of data annotation is in constant motion, and aspiring annotators in 2024 must be prepared to adapt to new methods and approaches. This means being comfortable with both established and newer annotation techniques, including those that leverage automation and combine human and machine efforts. As AI plays a greater role, annotators will need to master tools capable of handling a broader range of data types, improving both efficiency and the accuracy of labeling. Furthermore, staying informed about trends and new developments is essential, as specialized fields like healthcare and manufacturing place unique demands on the annotation process. By embracing continuous learning and maintaining a flexible mindset, data annotators can ensure they're prepared to meet the evolving needs of this fast-paced domain, ultimately leading to the creation of better training data and stronger AI systems. Staying adaptable is a skill that will become increasingly vital for success in data annotation.
The field of data annotation is rapidly evolving, driven by the increasing adoption of AI and the growing complexity of datasets. This evolution introduces new challenges and opportunities for aspiring data annotators. It's becoming clear that simply being proficient with current annotation tools isn't enough; adaptability and a constant drive to learn are becoming crucial.
Researchers are finding that automated annotation tools are being integrated at a rapid pace, potentially automating up to 60% of annotation tasks within a few years. While this surge in automation presents some efficiency gains, it's also leading to a redefinition of the annotator's role. It appears that human judgment and oversight remain essential, particularly in situations involving complex or nuanced data. Studies suggest that datasets with human oversight can achieve accuracy rates as high as 95%, exceeding the capabilities of fully automated systems. This implies a need for human annotators to develop complementary skills that leverage their strengths alongside automation.
Interestingly, individuals with knowledge in related disciplines like linguistics (for text-based annotation) or computer vision (for image/video data) seem to be able to improve the quality and accuracy of their work. Some annotators have reported significant reductions in error rates simply by applying cross-disciplinary perspectives, highlighting the potential for multi-faceted expertise within the field.
Gamification, the use of game-like elements within annotation platforms, has emerged as a surprising factor in boosting productivity. Researchers have observed an increase in annotator output by as much as 20% in projects that implemented gamification, indicating that understanding user psychology can play a significant role in enhancing efficiency and possibly engagement.
Furthermore, fostering collaborative environments where annotators actively engage in peer review and receive feedback has been found to contribute to improved annotation quality. These feedback loops appear to promote a sharper understanding of industry standards, resulting in accuracy improvements of up to 30%.
The increasing complexity of the data itself is also changing the nature of the annotation work. Individuals with training in cognitive load management—understanding their own cognitive strengths and weaknesses—appear to be better equipped to manage the challenges of handling increasingly complex data. This suggests a potential path for annotators to refine their own processes and optimize their efficiency.
Ethics has also become a central consideration. Research has shown that annotators trained in ethical principles and bias mitigation strategies produce datasets that can lead to significantly fairer AI outcomes. This emphasizes the responsibility of annotators to ensure AI systems are developed in a way that reflects broader societal values.
Legal considerations are intertwined with data annotation. Organizations and teams that adhere to data privacy laws and regulations like GDPR have seen reductions in compliance errors by as much as 50%. This signifies the growing importance for annotators to understand the legal framework within which their work exists.
Open-source software and collaborative communities also seem to play a crucial role in annotator growth and innovation. Many annotators report a heightened sense of engagement and a stronger ability to contribute when they actively participate in open-source tool development or engage with other experts in the field.
Finally, it's worth noting that data annotation trends are influenced by advancements in adjacent fields like machine learning and natural language processing. It's estimated that over 70% of new annotation techniques stem from interdisciplinary developments, showing that staying informed on broader technological trends can provide annotators with leading-edge methodologies.
The overall picture suggests that the skills needed to be a successful data annotator in 2024 go far beyond technical proficiency. The ability to adapt to emerging tools and techniques, coupled with an understanding of the ethical and legal context of the field, is becoming increasingly vital. Furthermore, developing a diverse skill set that encompasses cognitive management, collaboration, and critical thinking can contribute to a more rewarding and productive career path for those interested in this essential aspect of the AI revolution.
Analyze any video with AI. Uncover insights, transcripts, and more in seconds. (Get started for free)
More Posts from whatsinmy.video: