Analyze any video with AI. Uncover insights, transcripts, and more in seconds. (Get started for free)
7 Lesser-Known Data Visualization Techniques for Enhancing Video Content Analysis
7 Lesser-Known Data Visualization Techniques for Enhancing Video Content Analysis - 3D Bubble Charts Revealing Viewer Engagement Patterns
3D bubble charts offer a unique way to understand how viewers interact with video content. They utilize three dimensions to represent different aspects of engagement, like watch time, likes, and shares, with bubble size indicating another metric, possibly the number of viewers. This allows for a nuanced view of the relationships between these elements, revealing trends and patterns that might otherwise remain hidden. Similar to other less common visualization methods such as heatmaps or treemaps, they provide an alternative to traditional approaches. However, interpreting complex multidimensional visuals can sometimes be difficult. Consequently, 3D bubble charts may require some adaptation and familiarity on the part of the viewer for optimal comprehension. Even with potential challenges, they present a powerful way to gain a deeper understanding of viewer behavior, ultimately leading to better content optimization strategies.
3D bubble charts offer a way to visualize multi-faceted video engagement data. They can map out three different aspects of viewer interactions along the x, y, and z axes, while the size of each bubble represents a fourth variable like total watch time or number of likes. This approach gives a more holistic view compared to standard 2D charts, allowing us to see various aspects of engagement all at once.
We can use the bubble size to convey different levels of engagement tied to a particular video segment, making it easier to spot which sections resonate with the audience. This approach allows us to compare the engagement of distinct video elements side-by-side.
This type of visualization can reveal how viewer engagement differs across various audience subgroups. For example, we might notice differing engagement levels between younger and older viewers or across different geographic regions. Recognizing these patterns can aid in tailoring content to specific audience groups.
The interactive nature of 3D bubble charts offers an advantage over static charts. You can dynamically manipulate the view to zoom in or rotate, enabling a deeper look at interesting patterns within the data. This exploration is not possible with traditional charts.
Interestingly, these visualizations can reveal groups or clusters within the data. For instance, you might find that educational videos tend to have a certain pattern of engagement distinct from entertainment videos. Recognizing such trends can be helpful in improving the content strategy going forward.
However, these 3D charts also come with the risk of potentially becoming confusing if too many variables are crammed in. This could lead to cognitive overload and hinder the ability to grasp the insights. Therefore, it is crucial to thoughtfully select the data points represented in the chart to prioritize clarity.
Furthermore, analyzing engagement over time, like how it changes over the course of a video or across multiple videos in a series, is possible with bubble charts. This insight into the temporal aspects of engagement could influence the release or planning of future videos.
Introducing color gradients as another data layer can enhance the visualization. For example, different shades of a color might reflect sentiment based on viewer comments. By layering these details in, we can create a richer narrative around the engagement data.
Although they are potentially powerful, it would be useful to see 3D bubble charts more commonly adopted in real-time video analytics. This would allow for instant feedback on engagement during a live stream. Creators could use this feedback to adapt their content based on the live audience response.
Despite their clear benefits, 3D bubble charts are not commonly used in video analysis. This could be because they are inherently complex. It seems like finding the right balance between detailed representation of the data and ease of interpretation remains a challenge to their widespread use.
7 Lesser-Known Data Visualization Techniques for Enhancing Video Content Analysis - Parallel Coordinates for Comparing Multiple Video Metrics
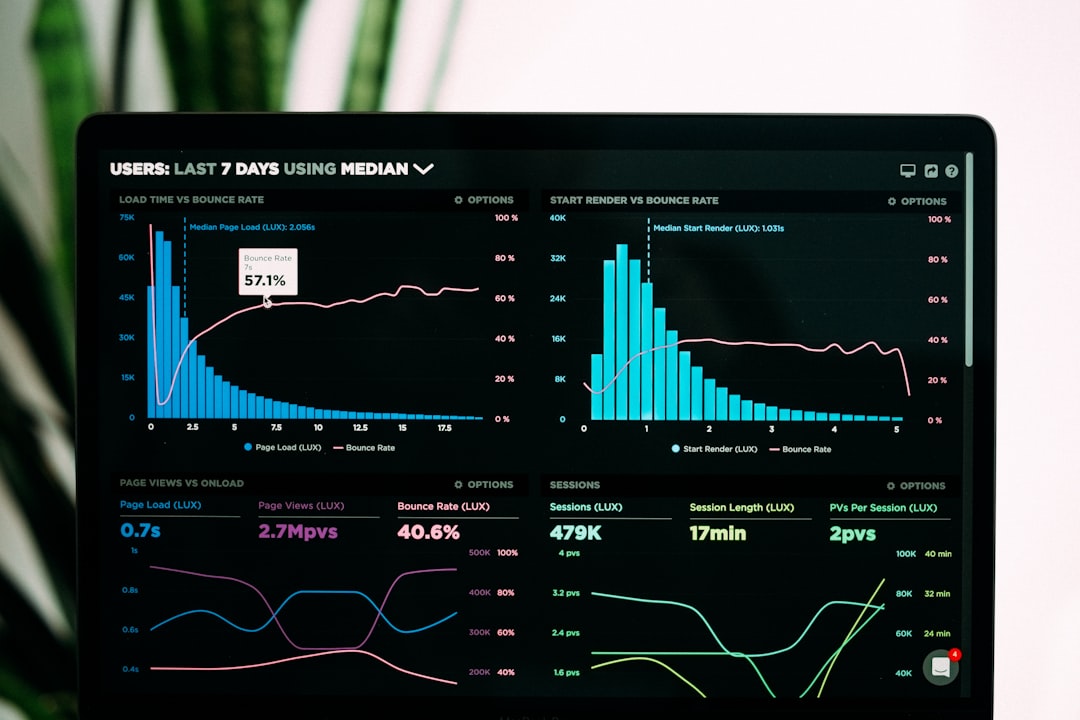
Parallel coordinates present a unique way to analyze video performance by simultaneously comparing multiple metrics within a single visualization. This technique utilizes vertical lines representing different video metrics, such as view count, audience engagement, or watch time. Data points for each video are then connected across these lines, making it possible to spot patterns and relationships that might otherwise be difficult to discern. While the method is potentially powerful, the visual density of overlapping lines can sometimes lead to confusion. Clear labeling and thoughtful design are essential to avoid overwhelming the viewer. However, when used appropriately, parallel coordinates can significantly improve video analysis, helping to spot trends and connections across various metrics. This method offers a compelling alternative to traditional charts, further strengthening the array of tools used for video content analysis.
Parallel coordinates offer a way to visualize multiple dimensions of video data simultaneously, allowing us to compare various video metrics like view counts, engagement rates, and watch times. Think of it as drawing vertical lines for each metric and then connecting the data points related to each video. This helps to more easily see patterns and correlations that might be obscured in simpler visualizations. This technique helps in situations where we need to understand relationships between several variables for each video, which goes beyond what a simple scatter plot can do. It’s particularly effective in helping to spot outliers – those videos that fall far outside the usual performance range – which is a great way to find unusual trends.
However, as with any visualization, it's important to be mindful of the complexity of the technique. One key advantage is the ability to tease out relationships between different performance factors, maybe seeing how changes in watch time influence share rates. This helps in understanding which aspects contribute to overall performance. To deal with very large data sets, parallel coordinates can incorporate sampling methods to make sure we’re not drowning in too much information. Interactivity is a valuable aspect. We can use brushing and linking to focus on specific parts of the data, changing the ranges displayed along each axis to refine our understanding. For instance, we could focus on a particular time period and observe how engagement rates change.
Parallel coordinates can handle categorical data, too, using techniques like colour coding. So, we could integrate information like the video category or when it was published to see if there are relationships between these details and performance. This allows us to delve into the topic in more depth, looking at it over different periods to uncover how things might change. For example, we could see how engagement with educational content fluctuates across the seasons or in relation to trending topics. By visually organizing videos in this way, we might identify clusters of videos that share certain engagement metrics. These clusters could help us better understand content themes that appeal to specific audience groups.
We can even bring in more sophisticated analysis tools. Machine learning techniques can potentially be used to identify the key factors driving audience engagement, which is quite intriguing. But, it's important to remember that, like many other advanced visualizations, this method has its limitations. With too many variables, it can get difficult to interpret. It’s a fine line between representing the data in detail and making it accessible. A good understanding of which metrics to select and how to use colour effectively can make a significant difference to how useful it is. If not managed well, it could end up being a cluttered and hard-to-understand mess.
Despite these challenges, parallel coordinates present a valuable tool for exploring the high-dimensional nature of video data. It offers a powerful way to discover subtle relationships and improve our understanding of how videos perform across a range of factors.
7 Lesser-Known Data Visualization Techniques for Enhancing Video Content Analysis - Chord Diagrams Mapping Content Sharing Networks
Chord diagrams offer a distinct approach to visualizing how content is shared across different platforms and networks. They use arcs and bands, where the width of the band shows the strength of the connection or how often content is shared. This makes them ideal for understanding how various video segments are shared or interacted with across different platforms. Color can be used to separate different types of content or relationships within the network, aiding interpretation. Chord diagrams are especially useful for showing how content is shared within video content, providing a way to visually map how specific elements within videos are interacted with across various channels. But, like any visualization technique, chord diagrams can be prone to information overload if not carefully designed. The use of these diagrams requires thoughtfulness to ensure clarity and prevent visual clutter.
Chord diagrams offer a unique way to visualize the intricate relationships within content-sharing networks. They use arcs and ribbons, where the thickness of the ribbon represents the strength of the connection or the frequency of interaction between different elements. This makes them really useful for understanding how video content flows across multiple platforms.
For instance, we can map how various video segments are shared on platforms like YouTube, TikTok, or Twitter. The thickness of the ribbon shows how often a particular segment is shared between any two platforms, giving us a sense of its popularity across different environments. Adding color coding helps further differentiate various types of content or relationships within the network, making complex information easier to understand.
Chord diagrams help us see how content spreads through a network in a decentralized way. We might find that a piece of video isn't just shared directly from the original creator, but rather bounces around through a number of users before reaching its final audience. This can help reveal the hidden influence of secondary sharers – those who spread a video without being the primary source.
Another interesting aspect is how chord diagrams can illustrate influence. They show not just the quantity but also the direction of content flow, highlighting which parts of a content network are most influential. It becomes easier to spot key figures who might drive a large chunk of the sharing activity for a specific piece of content, helping us understand how influence propagates.
While incredibly helpful, these diagrams can get overwhelming if we try to cram too much information into them. It's crucial to focus on the key relationships and use colors strategically to make the information as clear as possible. Despite this potential complexity, they offer a really valuable tool for uncovering hidden patterns within complex content sharing environments, especially in situations where simple charts don't provide a holistic view.
Chord diagrams could also potentially be used to track how content sharing evolves over time. If we update the visualizations in real-time, we can gain a dynamic view of how these networks change as content is shared and consumed. This type of information would be incredibly useful for anyone trying to optimize content distribution and understand audience behavior.
It's a bit of a challenge to connect things like likes, shares, and comments back to the visualizations on a chord diagram, but if we could effectively link them, we would gain even deeper insights into the dynamics of engagement within the content networks. We might even be able to highlight potential bottlenecks in content sharing. For example, if a certain video or segment has many connections but few pathways out, it could be a sign that the spread of that content is limited in some way. This could lead to valuable insights for improving content distribution strategies.
While chord diagrams present a powerful tool for video content analysis, they're still relatively underutilized. Perhaps part of the reason is the visual complexity that comes with representing multiple interacting elements in a circular format. Balancing detailed representations of interactions with clarity can be a significant challenge, but the insights they offer into the dynamic flow of content within large networks make them a compelling visualization tool to explore further.
7 Lesser-Known Data Visualization Techniques for Enhancing Video Content Analysis - Force-Directed Graphs Visualizing Video Topic Relationships
Force-directed graphs provide a way to visually understand how different video topics relate to each other. They use physics-like rules where related topics are drawn closer together, and unrelated ones are pushed apart. This helps reveal hidden connections within a video's content, making it easier to see the big picture. These graphs also make it easy to explore the data in an interactive way. For example, you can click on a specific topic to see details like view counts or how popular it is. However, like many complex visualization methods, force-directed graphs can become confusing if not designed properly, as it's possible to overload the user with information. If created thoughtfully, they can significantly enhance video analysis, offering a clearer understanding of how viewers interact with the content and guide future content strategy decisions. This method is part of a growing toolbox that analysts are using to better understand how people engage with videos.
Force-directed graphs provide a unique way to visualize relationships between video topics. Each video topic is represented as a node, and the connections, or edges, between these nodes show how topics are related or co-occur within videos. This approach, inspired by network theory, allows us to clearly see how closely related topics tend to group together.
Instead of a fixed layout, force-directed graphs dynamically reposition nodes based on the data. The algorithm simulates forces, causing nodes connected by edges to attract each other while unconnected nodes push apart. This process is akin to magnets interacting and helps to organize complex data structures in a visually appealing way that minimizes overlap.
One of the strengths of these graphs is their ability to handle large amounts of data. This scalability means that we can analyze extensive video libraries with many topics without the visualizations becoming overly complicated or overwhelming. This is a notable contrast to more traditional visualizations that often struggle with large datasets.
By using force-directed graphs, we can identify natural clusters of related topics. This ability to reveal groupings of themes within a video library can be a real asset for content creators trying to understand what their audiences are interested in. It can also help in developing a content strategy, pointing out popular topics and even newly emerging trends.
Many implementations of these graphs include interactive features. Users can click or hover over nodes to see related content or expand clusters of topics. This capability enhances the overall experience and makes exploring the relationships between topics more intuitive and engaging.
These visualizations aren't limited to just simple, direct links between topics. By changing the algorithm's parameters, we can display not only the most direct connections but also the more indirect relationships. This allows us to see hidden correlations that might not be obvious otherwise.
In some cases, these graphs can track changes over time. This feature allows us to see how the relevance of topics shifts as audience interests change. This type of insight is very useful for promptly adapting content strategies to keep pace with viewer preferences.
Further, we can tailor these graphs to highlight specific metrics, like viewer engagement or sentiment analysis related to a topic. This customization can help guide content development efforts, focusing on aspects that generate the most interaction and positive responses.
Excitingly, recent developments have connected force-directed graphs with machine learning. This combination allows for the automatic identification of key topics and relationships that drive viewer engagement. This automated approach could significantly streamline the process of understanding and reacting to audience behavior.
However, it's important to be mindful of a potential downside. With too many nodes and edges, these visualizations can become confusing, potentially causing cognitive overload for the viewer. It's vital to carefully design the graph, emphasizing clarity and simplicity, to ensure that the valuable insights aren't lost in complexity. If the design is not well-thought-out, the viewer may be left with a jumbled mess instead of useful information.
7 Lesser-Known Data Visualization Techniques for Enhancing Video Content Analysis - Alluvial Diagrams Showing Viewer Journey Across Multiple Videos
Alluvial diagrams offer a distinct way to visualize how viewers move through a set of related videos. They utilize colorful, flowing paths to represent viewer transitions, making it simple to see how people navigate from one video to another. This helps identify patterns in viewer behavior, such as where viewers tend to leave a series of videos or switch between different videos. The insights provided can be valuable for optimizing content to improve viewer retention and engagement. However, the intricate nature of alluvial diagrams demands careful design to avoid visual clutter and ensure easy understanding. Despite potential challenges in their application, they represent a valuable technique in the growing set of tools being used to analyze video content.
Alluvial diagrams offer a unique way to visualize how viewers navigate through multiple videos. They effectively showcase viewer journeys by using colored streams to represent different video content, revealing how users move from one video to another. This approach makes it easier to spot patterns in viewer behavior, such as how they might transition from a tutorial video to a related product demonstration.
We can see how viewer segments behave differently by comparing engagement patterns across various demographics or content categories. For instance, one segment might be more likely to watch a series of videos in order, while another might jump between related content in a non-linear fashion. Observing these differences can be valuable for creating more targeted content strategies.
These diagrams can also help visualize how viewer behavior changes over time. For example, we can see if certain videos become more or less popular at specific points, maybe influenced by seasonal trends or events. This temporal perspective adds a layer of depth that is hard to achieve with simpler visualization methods.
By tracing these paths, we can identify points where viewers drop off or switch to different content. This ability to pinpoint content bottlenecks can inform decisions on how to better structure video content and potentially improve retention. Furthermore, the diagrams can be integrated with other engagement metrics. This way, we can observe not just the paths viewers take, but also how successful those paths are in terms of watch time or engagement levels.
Looking at the thickness of the colored streams can help reveal the most popular content flows. A thicker stream indicates a greater number of viewers moving between two specific pieces of content. Understanding these popular pathways can help creators focus their efforts on the elements that are most effective at retaining viewer interest.
While insightful, it's important to acknowledge that alluvial diagrams can easily become cluttered if there are too many videos or viewer segments to analyze. If not designed carefully, the intricate network of connections can become confusing. It's crucial to make choices about which data to emphasize and avoid visual overload.
Interactivity is a desirable feature that can enhance the clarity of these visualizations. If viewers can filter the diagram based on factors like time period or viewer demographic, we can glean insights into specific subsets of users and their patterns.
Beyond a single video library or platform, these diagrams can illustrate how viewers move between different platforms. For instance, if a creator is looking at engagement across YouTube, TikTok, and their own website, alluvial diagrams can show how a viewer might start on one platform and then move to another for related content.
Interestingly, the flow paths in the diagram can reveal signs of viral content. We might see a specific video or segment drive an unusually large amount of traffic to other related content, indicating a potential viral trend. This insight can be useful for predicting which types of content might be more likely to trigger widespread audience sharing.
Overall, alluvial diagrams provide a valuable method for analyzing and visualizing complex viewer journeys. They hold potential for uncovering insights related to viewer behavior, engagement patterns, and content virality. However, as with any complex visualization technique, their effectiveness depends on thoughtful design and effective data selection to prevent unnecessary clutter.
7 Lesser-Known Data Visualization Techniques for Enhancing Video Content Analysis - Radial Trees Displaying Hierarchical Content Categories
Radial trees offer a visual way to understand hierarchical content structures, particularly useful in the context of video content analysis. They arrange data in a circular format, with a central core representing the main category and branching out to depict subcategories and their further subdivisions. This layout simplifies navigating and understanding relationships between different categories, making it easier to visualize complex hierarchies like video classifications or organizational charts. While effective in revealing the structure of complex data, radial trees can become visually cluttered if not carefully designed. Furthermore, individuals not accustomed to interpreting radial layouts might find them initially confusing. The design of radial trees therefore needs thoughtful consideration to ensure labels and relationships are easy to decipher, preventing misinterpretation and maximizing user understanding. Despite potential challenges, radial trees provide a valuable method for representing complex hierarchical information, but effective implementation hinges on user-friendliness and careful attention to clarity.
### Radial Trees Displaying Hierarchical Content Categories
1. **Hierarchical Organization**: Radial trees present hierarchical data in a circular manner, with a central node representing the main category and subsequent subcategories branching outwards in concentric layers. This structure makes it simple to grasp the relationship between the root and its various descendant categories.
2. **Compact Representation**: Unlike traditional tree structures that often sprawl horizontally or vertically, radial trees compactly represent hierarchies within a circular space. This efficiency is particularly beneficial when displaying intricate structures within limited screen areas.
3. **Reduced Overlap and Enhanced Comparison**: The angular arrangement of nodes in a radial tree minimizes overlaps, promoting visual clarity. Compared to linear tree representations, it becomes easier to compare categories at the same level (sibling nodes) and better visualize their hierarchical context.
4. **Attracting Viewer Attention**: The central focus of radial trees tends to capture viewers' attention more effectively than linear layouts. This inherent engagement can lead to better comprehension of the hierarchical structure, especially for audiences not familiar with the topic.
5. **Interactive Exploration of Hierarchies**: Radial trees can be designed to support interactivity, allowing users to explore deeper levels of the hierarchy by clicking or hovering over nodes. This dynamic exploration ensures viewers retain context as they navigate the data.
6. **Multi-Level Categorization**: Radial trees are well-suited for representing complex, multi-level categories. This makes them ideal for video libraries where content is organized into multiple nested layers. It becomes easy to visually comprehend how each piece fits within the overall content structure.
7. **Depth Perception Challenges**: While radial trees efficiently illustrate relationships, depth perception can be tricky when the hierarchy goes too deep. Viewers may struggle to differentiate and trace categories in the outer layers, requiring thoughtful design to maintain clarity for deeper nodes.
8. **Visualizing Node Properties**: Radial trees can incorporate visual cues about each node, such as view counts or engagement metrics. By adjusting node size or color based on these properties, users can quickly identify which categories are performing well, guiding decisions about content strategy.
9. **Scaling Issues**: When dealing with an extensive number of branches, radial trees can become cluttered, hindering easy interpretation. Maintaining a balance between representing the full dataset and ensuring visual comprehensibility is critical for effective presentation.
10. **Identifying Patterns and Trends**: Similar to other visualization techniques, radial trees facilitate pattern recognition and trend identification. By analyzing the structure and distribution of nodes, users can spot emergent categories, unusual patterns in engagement, or understand how different content areas connect to each other.
Analyze any video with AI. Uncover insights, transcripts, and more in seconds. (Get started for free)
More Posts from whatsinmy.video: