Top 7 Underutilized Video Analytics Datasets for Data-Driven Content Creators
Top 7 Underutilized Video Analytics Datasets for Data-Driven Content Creators - YouTube Natural Language Query Dataset
The YouTube Natural Language Query Dataset offers a unique perspective on how viewers interact with videos. It's a collection of human-written queries designed to find specific moments within YouTube videos. Each query is linked to the relevant sections of a video, and these sections are further rated for saliency, or importance. This dataset is invaluable for understanding how viewers phrase their search intents and what aspects of a video they find most relevant. This kind of insight can potentially be used to improve video summarization techniques or to help developers create more accurate video search engines. Despite its usefulness for enhancing video content, this dataset seems to be largely overlooked. There's an opportunity here for content creators and researchers to delve deeper into the data, perhaps uncovering novel approaches to content creation and user engagement.
The YouTube Natural Language Query Dataset is a valuable resource for investigating how people interact with videos using natural language. It's a massive collection of queries, representing a wide range of video topics and how users actually ask their questions. This provides a foundation for building more accurate and intuitive video search algorithms.
It's interesting that the dataset reveals differences in query phrasing depending on user characteristics. This suggests that refining NLP models to account for those nuances can make search engines more responsive to user needs. Besides the queries themselves, it also includes contextual information, such as video IDs and timestamps. This allows researchers to explore how people watch videos and what they're looking for.
Beyond the core queries, the dataset can be used to examine how video features influence a viewer's choice. For example, how video length, title, or description might affect whether it's picked for a specific query. While mainly in English, it also contains a sampling of queries in other languages, which could inform the development of more globally accessible search functionalities.
The dataset has been used in academic competitions designed to evaluate search engine performance. The results have shown that many systems still struggle with informal or nuanced language. Furthermore, studies using this dataset indicate a relationship between query length and successful search outcomes. Short, direct queries often lead to better results compared to lengthy, detailed ones.
This openly accessible dataset has the potential to spark collaboration and development within the video analytics field. This allows engineers to craft enhanced video experiences based on real-world data. Intriguingly, the dataset even shows that user queries can exhibit seasonal trends influenced by holidays or important events. This insight could be helpful when considering content creation timing to capture audience interest during specific periods.
Top 7 Underutilized Video Analytics Datasets for Data-Driven Content Creators - Amazon Prime Video Analysis Project
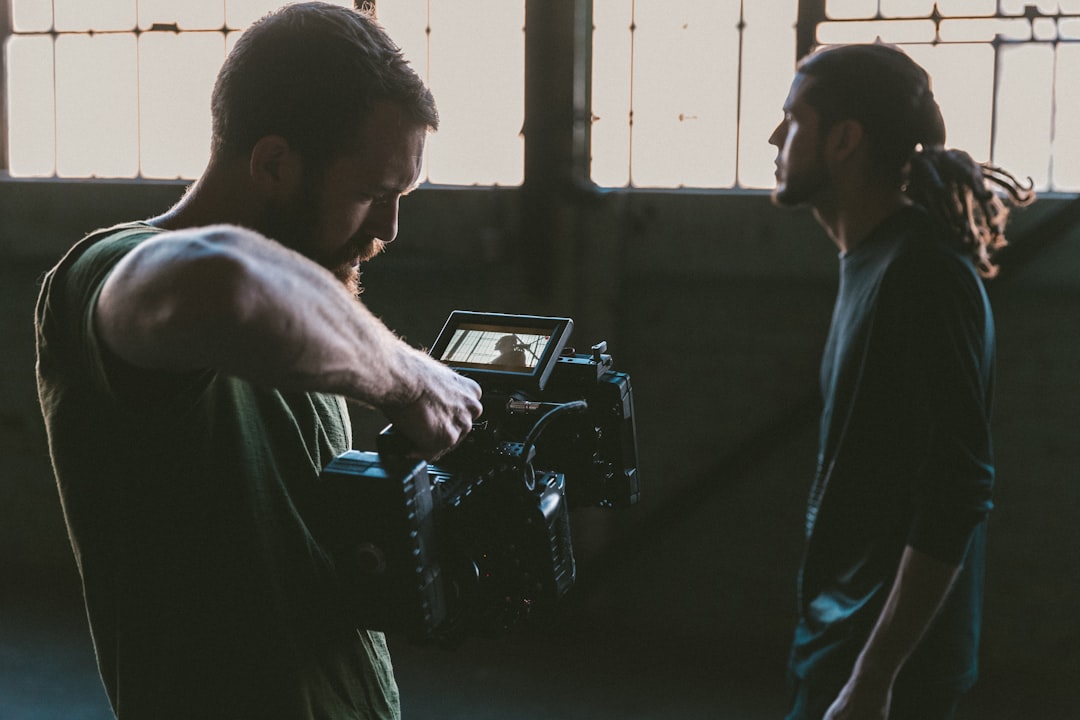
The "Amazon Prime Video Analysis Project" leverages a dataset from Kaggle to delve into the platform's content library. This project employs Power BI to craft an interactive dashboard that offers insights into how content is distributed across genres and how popular those genres are, alongside user ratings. Interestingly, the analysis reveals uneven distribution in user ratings, with some categories being significantly more prevalent than others. A key aspect of this project is showcasing the significance of both data cleaning and visualization, using Excel and Power BI, to accurately interpret the data. Furthermore, it underscores the need for content creators to understand how viewers interact with the platform's content. By utilizing this project's findings, creators can use data-driven approaches to potentially improve content strategies and increase audience engagement within the Amazon Prime Video ecosystem. While Amazon Prime Video undoubtedly uses its own extensive internal data for its purposes, this project provides a glimpse into how publicly available data can contribute to a deeper understanding of the platform and the preferences of its users.
The Amazon Prime Video Analysis Project examines a Kaggle dataset to explore and visualize the content library offered by Amazon Prime Video. They use Power BI to create an interactive dashboard that gives insights into how content is organized, the popularity of different genres, and user ratings. The dataset provides detailed information about movies and TV shows, including genres, release dates, and user ratings, allowing for comprehensive analysis.
The analysis reveals that the '13' rating has the most movies and shows, while 'TVNR' has the fewest. The project's main goal is to uncover interesting trends and patterns within the movie and TV show catalog on Prime Video. They're particularly interested in understanding user behavior and how it impacts content performance through data analytics.
The project emphasizes the value of data cleaning and initial analysis with Excel, and how that information is used to drive visualizations in Power BI. Prime Video relies on machine learning to ensure quality, specifically to detect issues like video corruption or audio problems. Amazon's extensive, proprietary user data provides a huge advantage for understanding customer tastes, giving them a leg up in the market when it comes to marketing.
The techniques and tools employed in this project aim to provide creators with data-driven insights. The hope is that this helps them make better choices for keeping viewers engaged and designing a more effective content strategy. However, while providing insights into viewer behavior, it's important to critically consider the ethical implications of how such granular data is being used and if it is impacting creative freedom in the long run. While providing useful patterns, using data-driven insights alone can limit creativity and diversity if creators become too fixated on the metrics alone. Overall, the Prime Video analysis project provides a good example of how readily available datasets can be used to examine how viewers consume content on streaming services, with a mix of obvious insights and some more specific, subtle, trends.
Top 7 Underutilized Video Analytics Datasets for Data-Driven Content Creators - Highway Traffic Videos Dataset
The Highway Traffic Videos Dataset offers a unique opportunity to study traffic flow and behavior using footage captured by highway CCTV systems. This dataset consists of numerous video clips, enabling researchers and content creators to analyze various aspects of traffic, such as accident detection and traffic density levels. While many cities use extensive camera networks to monitor traffic conditions, manually analyzing the collected footage is often slow and inefficient. This dataset emphasizes the need for innovative solutions to automatically extract valuable information from video data to improve traffic safety and management. With rising traffic congestion in many urban environments, insights derived from datasets like this can empower traffic authorities to make data-driven decisions in areas like urban planning and real-time traffic flow optimization. However, it's crucial to ensure the use of these datasets aligns with ethical standards and respects the privacy of individuals captured in the footage.
### Highway Traffic Videos Dataset
1. **Varied Traffic Conditions**: The Highway Traffic Videos Dataset offers a range of traffic situations, from peak hour congestion to quieter nighttime periods. This diversity allows for in-depth studies of how vehicles behave under different circumstances.
2. **Towards Real-Time Solutions**: It's possible to use the dataset to mimic real-time traffic scenarios for intelligent transportation systems. This can help engineers devise better solutions for managing traffic and create predictive models.
3. **Training Object Detection**: The dataset includes many kinds of vehicles and driving behaviors, making it useful for training machine learning models that can detect objects. By examining such a wide variety of visuals, algorithms can become more precise in real-world settings.
4. **Driver Behavior Analysis**: The dataset reveals not just how vehicles respond to traffic signals and signs but also how drivers behave. For instance, sudden lane changes or abrupt stops can be identified, which is essential for improving safety features.
5. **Identifying Lane Violations**: The data captures various instances of drivers straying outside of their assigned lanes. This can aid in the development of systems that accurately detect such violations, helping enhance law enforcement approaches.
6. **Multiple Camera Views**: Parts of the dataset use multiple cameras to record the same road area. This allows for exploring how different viewpoints can give a better understanding of traffic events and improve the stability of algorithms.
7. **Accident Prevention Insights**: By analyzing the recordings, engineers can pinpoint factors that commonly contribute to accidents. This can lead to better road design and driver education initiatives for increased safety.
8. **Daily Traffic Fluctuations**: The dataset covers traffic at various times of the day, illustrating substantial differences in traffic patterns. This enables researchers to create models that predict traffic based on time.
9. **Weather-Related Challenges**: The recordings include vehicles driving in varying weather conditions, including rain and fog. This highlights the difficulties in vehicle performance and can guide the development of more dependable driving algorithms.
10. **A Stepping Stone for Autonomous Vehicles**: The detailed nature of the traffic interactions in this dataset is valuable for researchers working on self-driving vehicles. It provides essential data for training models that make better decisions in complex driving situations.
Top 7 Underutilized Video Analytics Datasets for Data-Driven Content Creators - YouTube8M Large-Scale Labeled Video Dataset
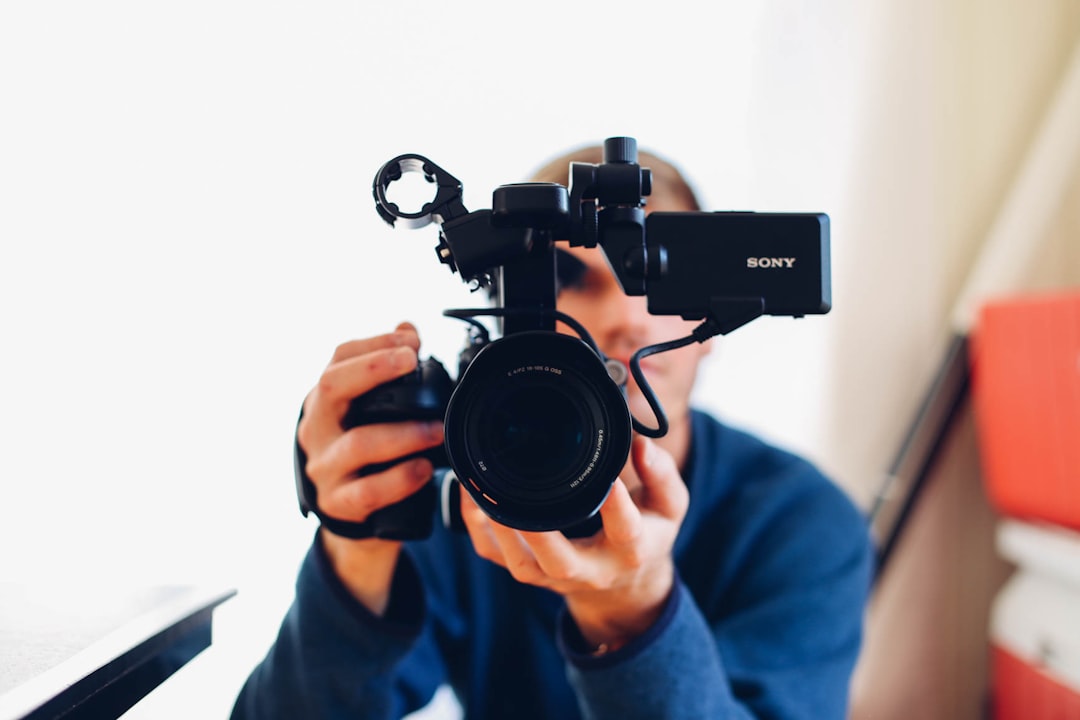
The YouTube8M dataset is a substantial collection of video data, consisting of 8 million videos with over 500,000 hours of labeled content. It's notable for its scale and the use of automated annotations covering a wide range of 4,800+ visual categories. This allows for advanced video classification tasks, particularly multilabel classification, which is a more sophisticated way to analyze video content compared to earlier datasets like Sports1M. YouTube8M also includes pre-calculated features from video and audio segments, simplifying its use for different applications. Notably, YouTube8M Segments introduced human-verified annotations for over 237,000 video sections across 1,000 categories, offering a higher level of accuracy and detail. Despite the usefulness of this dataset, it's surprisingly underutilized in the content creation community. It could be a valuable resource for developers and researchers to experiment with, potentially leading to new methods for video understanding and classification. There's a clear need for a wider awareness and adoption of this large-scale video resource.
YouTube8M is a substantial labeled video dataset containing over 8 million YouTube videos, each tagged with descriptive labels. This massive scale makes it a prime resource for training machine learning models focused on video understanding. Each video is assigned multiple labels, enabling detailed categorization and allowing for more complex classification tasks compared to simpler, single-label datasets. The dataset's content is remarkably diverse, covering 25,000 unique categories, encompassing everything from popular culture to scientific and technological themes. This variety is helpful for developing machine learning models capable of handling a wide range of video types.
Furthermore, YouTube8M offers a temporal breakdown of each video, providing valuable information about the content's structure and allowing for the examination of specific segments. Interestingly, the dataset was specifically crafted for machine learning benchmark tasks, making it highly relevant to video understanding challenges such as identifying events and summarizing video content. Research projects have used YouTube8M to enhance video recommendation systems, leading to a better user experience through more personalized content suggestions based on viewer patterns.
The dataset has also been a popular choice in machine learning competitions, consistently pushing researchers to develop more sophisticated approaches to video analysis. Despite its widespread use, the dataset has revealed a challenge for current state-of-the-art models. Even the most advanced models still encounter difficulty in achieving high accuracy scores, highlighting the need for ongoing improvement in video classification techniques. The dataset also exhibits a wide range of video lengths, ranging from very short to quite long, providing an opportunity to investigate how the length of a video influences a viewer's interaction with it.
However, it's important to acknowledge that models trained exclusively on YouTube8M might encounter difficulties when confronted with video data that doesn't closely match the typical patterns found in the dataset. Their performance might decline, highlighting a need for adaptable training techniques. Yet, the comprehensive nature of YouTube8M makes it useful for transfer learning. Models trained on this dataset can be adjusted to function effectively with other, smaller datasets, or adapted for other, different video domains. This adaptability can be especially helpful when dealing with data limitations in less commonly studied video areas.
Top 7 Underutilized Video Analytics Datasets for Data-Driven Content Creators - Twine Audio-Visual AI Dataset
The Twine Audio-Visual AI Dataset aims to tackle the issue of bias in AI models that deal with human subjects in audio and video applications. They've created a substantial collection of over 150 open datasets, organized by factors such as language, file format, and the demographic makeup of the people in the recordings. This addresses a crucial problem – the lack of easily available, high-quality datasets in these areas. Twine's collection includes specialized datasets like EmoSynth, which consists of short audio clips labeled for emotional content, as well as larger datasets like VSPW, focused on analyzing video scenes at a detailed level. The sheer variety of datasets demonstrates the importance of having rich and accurately annotated data for training AI models effectively. This dataset is a valuable resource for content creators who want to explore better methods for analyzing audio and video content. It highlights the potential for more advanced techniques and tools that leverage diverse datasets, encouraging the field to tap into this often overlooked resource.
The Twine Audio-Visual AI Dataset is a collection of video clips paired with over a million labeled transcripts. This unique pairing makes it useful for studying how audio and visual elements work together. Researchers can use this to explore the relationship between what's being said and what's shown on screen, which is important for understanding how information is presented and received.
Because it combines audio and visual data, the Twine dataset could be useful across different fields like linguistics, psychology, and media studies. This multi-faceted nature opens doors for a wider range of insights into things like how viewers engage with content and how they understand what they see and hear.
A key aspect of this dataset is the detailed semantic annotations. This means that the visual scenes and spoken words are labeled with information about the subject, emotions, and the intended meaning. This added detail can greatly improve the performance of models built for tasks like emotion detection and sentiment analysis.
Researchers can also use Twine to study patterns in how people communicate, such as gestures, eye contact, and the use of multimedia tools in presentations. Understanding these patterns could be valuable for improving educational content or refining training programs.
It's worth mentioning that Twine is a useful tool for evaluating different kinds of algorithms. These algorithms are often designed to analyze audiovisual interactions, like automatic speech recognition or scene understanding. Having a dataset like this helps researchers compare and improve the accuracy and efficiency of their methods.
While the dataset's primary use seems to be in academic research, content creators could also benefit from this resource. They could explore the data to find out how viewers respond to different types of audiovisual narratives, helping them create content that better aligns with audience understanding.
The dataset's temporal annotations can be used to study how communication evolves over time. Researchers can examine changes in both speech and visuals within the content, leading to a better understanding of the influence of pacing and sequence on the overall storytelling experience.
Twine is a good example of a dataset that supports multi-modal machine learning. This field aims to combine information from various sources (audio, video, and text) to improve a system's understanding of human communication.
By training algorithms on this dataset, it may become possible to analyze audience reactions in real-time. This could lead to improvements in the delivery of presentations or other kinds of content by allowing for quick adjustments based on viewer reactions.
Lastly, the video content in the dataset captures diverse cultural contexts. This makes it a good resource for studying how cultural nuances affect communication styles and the ways in which people interpret audiovisual material. This is especially relevant for creators working on content for global audiences, who need to be mindful of cultural diversity and representation. While the dataset has strong potential, it's important to be aware of the challenges in ensuring the data is truly representative of all cultures and demographics.
While it seems there's been limited utilization of this dataset, Twine presents some unique opportunities that have yet to be fully explored. There's still a lot to be learned from this type of rich, multi-faceted dataset, and it could potentially serve as a foundation for new research and innovative content creation in the future.
Top 7 Underutilized Video Analytics Datasets for Data-Driven Content Creators - Social Media Engagement Metrics Dataset
The "Social Media Engagement Metrics Dataset" provides a valuable window into how audiences engage with video content across social media platforms. This dataset comprises a collection of user interactions, such as posts, comments, likes, and shares, alongside audience demographics and behavioral information. Given the increasing reliance on video across social media, especially on mobile devices, metrics like daily engagement rates and share counts are crucial indicators of a video's effectiveness and viral potential. This dataset empowers content creators to not only measure their performance against benchmarks but also gain insights into audience preferences to refine their content strategies. The fact that this dataset remains relatively underutilized within the content creation community suggests a missed opportunity for deeper analysis. By exploring the patterns embedded within this data, creators can potentially unlock strategies that lead to more engaging and impactful videos.
A "Social Media Engagement Metrics Dataset" could offer a rich source of information for understanding how people interact with video content across various platforms. It would likely cover a wide range of data points, including likes, shares, comments, and views, which can vary significantly depending on factors like video topic, release time, and the platform itself.
For instance, it's plausible that videos like tutorials, due to their informative nature, might show substantially higher engagement rates compared to entertainment-focused content. The optimal time to post a video for maximum impact could also be hidden within this type of dataset. Perhaps there are patterns in engagement spikes based on the time of day, revealing optimal upload schedules.
Interestingly, the number of comments a video receives could be a surprisingly reliable measure of engagement. This could imply that viewer discussions indicate a deeper connection to the content, potentially providing an opportunity for creators to directly interact with their audience.
While overall view time is commonly used as an engagement metric, the retention rate—how long viewers stay engaged with a video—might offer a more refined perspective. High retention might suggest that a video's content and presentation successfully hold a viewer's attention, possibly aiding creators in improving their storytelling and pacing.
The effectiveness of video thumbnails and titles likely has a significant impact on click-through rates, as people are more likely to click on videos with visually appealing thumbnails and catchy titles. This emphasizes the importance of dedicating effort to create compelling visuals and concise descriptions.
Furthermore, a Social Media Engagement Metrics Dataset could potentially include data on viewer demographics, such as age and gender. This granular information could show engagement patterns across these groups, enabling creators to adjust their content to better resonate with a wider audience.
It's also possible that video engagement often follows a predictable pattern. We might find that after an initial surge in views and interactions, engagement levels start to flatten out. Understanding this "engagement plateau" is crucial as creators can then devise ways to maintain audience interest over time.
Beyond this, the influence of different social media platforms on engagement rates is also worth noting. Some platforms may naturally foster higher levels of interaction compared to others, making it important for creators to optimize their content and strategy for each unique platform.
It's plausible that the initial viewer engagement creates a sort of feedback loop, leading to increased visibility for a video, which in turn results in even higher engagement levels. This highlights the importance of promoting videos across platforms to broaden reach.
Finally, the use of relevant hashtags in video descriptions could play a crucial role in driving engagement. Viewers often discover new content through hashtag searches, so it could be valuable to study the effectiveness of various hashtags for a specific audience or video type. This information could help creators better understand the current trends and tailor their content marketing strategies accordingly.
In conclusion, a well-structured dataset focused on social media engagement metrics could offer a treasure trove of insights for video content creators. These insights could help refine content strategy, enhance audience engagement, and ultimately lead to more successful and effective videos in the ever-changing landscape of online media. However, like any dataset, it is important to consider the potential biases and limitations it might have, and be thoughtful about how the data is used and interpreted.
Top 7 Underutilized Video Analytics Datasets for Data-Driven Content Creators - Video Retention and Watch Time Analytics
**Video Retention and Watch Time Analytics**
For content creators, understanding how viewers engage with their videos is vital. This is where video retention and watch time analytics come in. Metrics like overall "Watch Time" and "Average View Duration" provide key insights into viewer engagement. They reveal which parts of a video hold a viewer's attention and pinpoint sections where interest might wane. Analyzing this data can guide creators in refining their content, optimizing for audience retention. Interestingly, platforms often use this data to decide how to promote videos. Videos that keep viewers watching longer are often given a boost in visibility. However, many content creators primarily focus on simple view counts or likes. These basic metrics offer limited insight compared to deeper analysis. Exploring specialized datasets focused on watch time and retention can unlock a deeper understanding of audience behavior. This allows creators to build more strategic content plans, aligning their creative efforts with viewer expectations and preferences, ultimately increasing the likelihood of creating impactful and engaging content.
Understanding how long viewers stay engaged with a video and the overall time they spend watching is foundational for gauging how captivating your content truly is. "Watch Time" is a primary metric used by platforms to measure viewer engagement, and it's a good starting point. However, solely relying on total watch time can sometimes be misleading. We can calculate "Average View Duration" by dividing the cumulative watch time of a video by the total number of views, which provides insight into whether the content keeps people hooked. If the average duration is high, it suggests that the content successfully maintains viewer interest. Platforms tend to give preferential treatment to videos with high watch times, promoting them more broadly.
It's useful to think about video metrics in two ways: quantitative and qualitative. Quantitative metrics are things we can measure easily, such as the number of views, the total watch time, and likes. These numbers can be readily tracked. Qualitative metrics, on the other hand, require a more nuanced approach. For instance, a video with high watch time might not always translate to a healthy and engaged audience. Content creators really need to keep tabs on metrics offered by the platforms (like YouTube Analytics) to understand the pulse of their audience and tailor content better.
While things like total "View Count" are a common benchmark, they don't tell the whole story about engagement. To truly develop effective video content, creators need to go beyond these simple measures and dive deeper. Many creators often miss out on important data that could illuminate how their audience thinks and what they like. They may miss the opportunity to enhance their content strategy. Automated tools, like Unmetric, offer real-time reporting and can help sift through the data efficiently, allowing content creators to make quicker and more informed decisions based on data.
Strategies to keep viewers engaged for longer typically involve fine-tuning the video content. This might include adjusting the introduction of the video to hook viewers within the first few seconds, which are often when a significant number of people stop watching. We also see different types of video content display varying retention patterns. Educational content, like tutorials, often tends to see longer watch times than vlogs. Understanding the specifics of the content and your audience is key to keeping people engaged.
The ability to comprehend and apply advanced video analytics can result in significant leaps forward for content creators. We can see trends in video performance based on various factors, like the inclusion of video playlists, thumbnail designs, or the optimal length of a video. By taking a multi-faceted approach, creators can enhance the viewers' experience while increasing their overall watch time. It's clear that understanding the data isn't just about reaching a high view count, it's about developing a more robust and authentic connection with the audience.
More Posts from whatsinmy.video: