7 Lesser-Known Public Dataset Sources for Video Analysis Research in 2024
7 Lesser-Known Public Dataset Sources for Video Analysis Research in 2024 - KITTI Vision Dataset Library For Autonomous Driving Research 2024
The KITTI Vision Dataset, a product of the Karlsruhe Institute of Technology and Toyota Technological Institute, remains a cornerstone for autonomous driving research in 2024. Its value lies in the diverse sensor data it provides, encompassing stereo cameras and LiDAR, which are vital for tackling a broad range of computer vision challenges within autonomous driving. These include tasks like identifying and tracking objects, understanding scenes, and estimating 3D object locations. Captured across various traffic conditions, the dataset offers over 7,000 annotated images for training object detection algorithms. It also incorporates a benchmark suite that allows researchers to assess their algorithms against real-world conditions, propelling progress in both mobile robotics and autonomous vehicle technologies. While extensively studied and referenced in the field, with over 70 research papers drawing upon its data, the dataset's future adaptability to the ever-evolving landscape of autonomous environments remains a question. While invaluable, the KITTI dataset might not always fully capture the complexity of contemporary autonomous driving challenges, highlighting the need for ongoing development of new datasets tailored to these emerging needs.
The KITTI Vision Dataset, a product of collaboration between the Karlsruhe Institute of Technology and the Toyota Technological Institute at Chicago, is specifically tailored for autonomous driving research. It's designed to provide a comprehensive understanding of the challenges faced in real-world driving environments. This is achieved through a diverse collection of sensor data, including stereo camera images and LiDAR scans. The dataset aims to address a wide range of computer vision problems like object detection (pedestrians, cars, cyclists), 3D scene reconstruction, and motion estimation (optical flow).
The KITTI dataset is built on a large collection of driving sequences captured over six hours, at various frame rates (10-100 Hz). This data includes a variety of sensor outputs such as color and grayscale images, captured using high-resolution stereo cameras, and depth information provided by a Velodyne LiDAR scanner. Additionally, a GPS unit provides location information, allowing researchers to study precise vehicle movement within the scene.
The dataset contains 7,481 annotated training images, each with precisely drawn bounding boxes for object detection tasks. It also provides a benchmark suite which is invaluable for assessing the performance of vision algorithms in the context of autonomous driving and mobile robotics. Notably, KITTI has been extensively utilized by the research community, having been referenced in over 70 publications, and it's become a standard benchmark for assessing algorithms in real driving scenarios. Its continued evolution and the benchmark tasks it promotes are influential in shaping the future trajectory of autonomous vehicle technology. However, some researchers have pointed out challenges in achieving truly robust performance in certain aspects of KITTI. For example, some object classes, like those that are frequently occluded or are of varying sizes, present difficulties for vision algorithms. This, in turn, drives the search for more robust methods for real-world autonomous driving scenarios. Despite this, the dataset remains a powerful tool in pushing forward the frontiers of research into autonomous systems.
7 Lesser-Known Public Dataset Sources for Video Analysis Research in 2024 - AVA Dataset 500k Labeled Human Actions In Movie Scenes
The AVA dataset is a collection of 500,000 human actions captured from 430 movie clips, each action labeled with one of 80 basic, "atomic" actions. It's a significant resource for studying human behavior because it contains a massive 162 million action labels, primarily focused on visually distinct movements. The dataset's construction, using clips extracted from longer segments of films, offers researchers a natural and diverse range of human activity to analyze. Annotations are made every 3 seconds, enabling precise temporal analysis for building video understanding models. While quite large, the dataset's primary focus is on individual actions, not complex chains of actions. This characteristic, while beneficial in some research, can limit the scope of investigations into more complicated human activity patterns.
The AVA Dataset provides a substantial collection of 500,000 labeled human actions extracted from movie clips, making it a valuable resource for training and testing action recognition models. It differs from many other datasets by focusing on diverse, realistic human behaviors found in movies, which often include more varied and complex actions compared to, say, sports or staged scenes.
The annotations within AVA are specifically designed to pinpoint individual actions, enabling researchers to accurately analyze the temporal aspects of human activities within a video. These actions are classified into over 80 different categories like "dancing" or "playing an instrument", providing a fine-grained understanding of the action being performed. This focus on individual, atomic actions, and their precise temporal location is particularly useful for understanding complicated events in video where multiple actions may occur at once. However, this level of detail does present challenges when it comes to employing typical object detection methods.
AVA is built from a massive amount of movie footage, over 2,800 hours, demonstrating the rich variety of human actions it encompasses. A key characteristic of this dataset is its emphasis on spatial-temporal labels. This means that each action isn't just identified, but also tagged with a specific start and end time. This approach facilitates a more in-depth analysis of how actions unfold within moving scenes.
There is evidence that combining the AVA Dataset with other datasets can significantly enhance the accuracy of human action recognition models. This finding underscores the importance of building hybrid learning models that can leverage diverse sources of information when dealing with complex video understanding tasks. However, some have questioned whether AVA's inherent bias, due to the nature of the movies it is built upon, might limit its applicability in truly generalizable real-world scenarios. It seems as if, while AVA is extremely valuable, researchers need to be mindful of how the characteristics of its data might impact the performance of their models when applied to new scenarios outside the scope of movie scenes. In short, AVA pushes the field of action recognition forward but also serves as a reminder of the limitations of using any specific dataset without careful consideration. This push to extend understanding of human actions in context through advanced AI techniques is what makes this dataset useful.
7 Lesser-Known Public Dataset Sources for Video Analysis Research in 2024 - NTU RGB D 120 Database For Human Movement Recognition Studies
The NTU RGB+D 120 database is a valuable resource for researchers working on human movement recognition. It provides a large collection of over 114,000 video samples, each containing both color and depth information, gathered from 106 different individuals. These videos showcase 120 distinct action categories, ranging from everyday activities to more specialized actions like those related to health or social interactions. The database offers over 8 million frames, making it a rich source for training and testing algorithms designed for understanding human motion in video.
One of the primary benefits of this database is its ability to address some limitations present in earlier benchmarks. For instance, it provides a significantly larger number of training samples and captures actions from more diverse angles than many previous datasets. The use of the Microsoft Kinect sensor during data collection adds further richness to the dataset. It captures not only RGB video and depth information but also skeleton data and infrared images, making it possible to study human movement in a multi-faceted way. This diverse collection of data is especially useful for exploring one-shot 3D activity recognition, where the goal is to understand and classify a single, unseen action effectively.
In essence, the NTU RGB+D 120 dataset offers researchers a strong foundation for building and evaluating new approaches to human movement recognition. It's a particularly relevant tool for studies focused on depth-based and RGB-D based action recognition research. Its sheer scale and the variety of data it contains promise to advance the field by enabling the development of more powerful and accurate video analysis techniques for human activity recognition. While it offers a powerful tool for researchers, it's important to remain mindful of the specific context in which the data was captured, as any dataset can have inherent biases that may influence research findings.
The NTU RGB+D 120 dataset is specifically crafted for delving into human movement recognition, encompassing a wide range of 120 distinct action classes. This makes it a valuable resource for researchers studying how humans move in various situations. It involves data from 106 individuals, providing a decent level of diversity for action recognition studies. These actions include everyday routines, social interactions, and even health-related activities.
The dataset contains over 114,000 RGB-D video clips, captured using Microsoft Kinect, offering a substantial amount of data for training and evaluating video analysis algorithms. This translates to more than 8 million frames across all these clips. The inclusion of RGB videos, depth information, skeleton data, and even infrared frames presents a rich set of data modalities. It's a definite step towards addressing some issues found in other datasets, like limited training data and a lack of different camera perspectives and action categories.
One of its unique strengths is facilitating research into "one-shot" 3D activity recognition. This could lead to more efficient ways to recognize human movements. By offering a large-scale dataset like this, it's also been a catalyst for exploring the effectiveness of 3D representations for action recognition, which is a significant contribution. The dataset's emphasis on realistic action labels and enhanced sample variety is considered a step forward in video analysis research.
It's an important advancement because it allows for more robust evaluation of methods and algorithms within the field. However, it's important to recognize that the environment in which the data was collected is quite controlled, and this can lead to questions about how well models trained on this data will generalize to less structured environments. Further exploration is needed to see how well these models perform under variations in lighting, occlusion, and other factors present in real-world scenarios. Regardless, it's clear the NTU RGB+D 120 dataset represents a significant resource in the field of human movement recognition studies, opening the door for future developments in the use of RGB-D based methods. The long-term implications could stretch into fields like virtual reality, human-computer interaction, and other areas where human activity recognition is a core aspect. It might be interesting to explore if future iterations of this dataset might encompass even greater diversity in actions and environmental contexts, which would push the frontiers of human movement recognition even further.
7 Lesser-Known Public Dataset Sources for Video Analysis Research in 2024 - VGGSOUND Dataset With 200k Audio Video Segments From Youtube
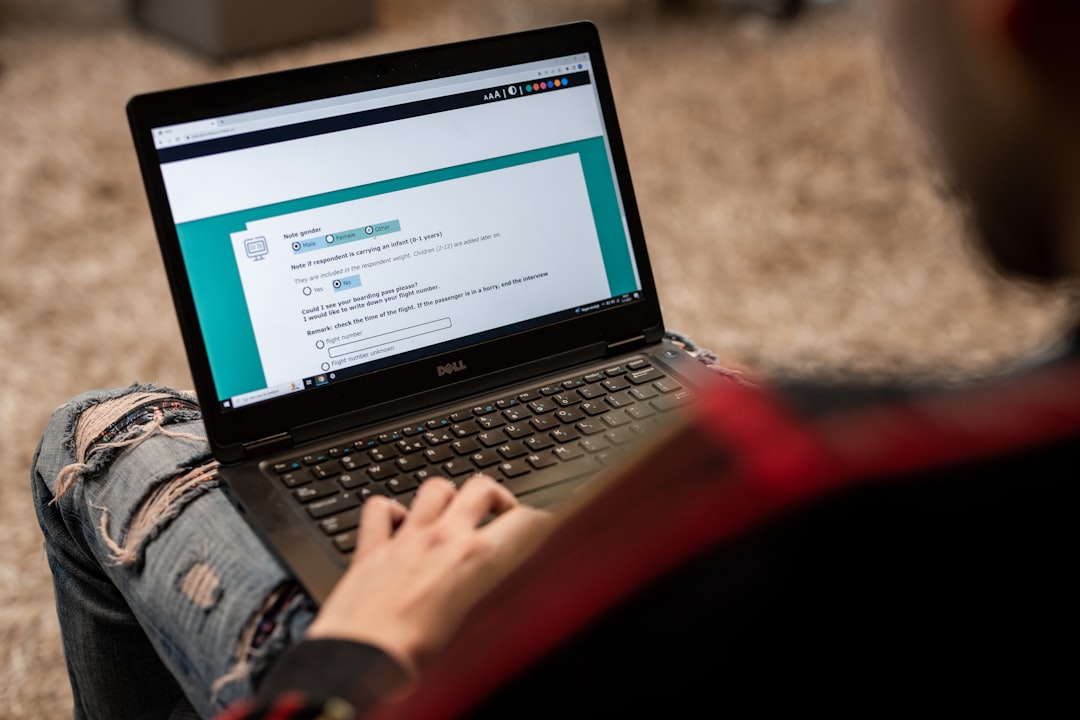
The VGGSOUND dataset offers a substantial collection of 200,000 audio-video segments sourced from YouTube, making it a valuable resource for video analysis research. It's organized into 310 different audio categories, providing a structured environment for developing and assessing audio recognition models. Each video segment is roughly 10 seconds long, carefully curated using computer vision techniques to align the visual content with the audio, effectively showcasing the source of the sound.
The dataset's strength lies in its relatively low level of labeling errors, making it a dependable option for audio classification tasks. The dataset's structure is user-friendly, with a CSV file providing information like YouTube URLs, timestamps, and audio labels to facilitate organization and access. Researchers working on audio recognition are given a head start with the inclusion of pretrained models and evaluation scripts. While the dataset provides a substantial set of data, it's always worth considering the potential biases introduced by relying on a single source like YouTube. The long-term implications of using this dataset are yet to be fully explored but hold significant promise for pushing the boundaries of audio understanding within video data.
The VGGSOUND dataset, a collection of over 200,000 audio-video clips gathered from YouTube, offers a sizable resource for research into audio-visual learning and recognition. It's structured around 310 distinct audio classes, which can be used to train and test audio recognition models. The creators utilized computer vision techniques to ensure that the video frames and audio samples are tightly linked, making it a strong dataset for multimodal tasks. Each segment, roughly 10 seconds long, presents a visual representation related to the sound it contains.
One aspect that simplifies using VGGSOUND is the CSV file included. This file provides a helpful organization system, with YouTube URLs, timestamps, audio labels, and different data splits. It's also readily available under a Creative Commons license, making it accessible for academic and research pursuits. It's been reported to have a fairly low level of label noise, which is valuable in building reliable audio classification models. The development and evaluation of the dataset is detailed in an ICASSP 2020 paper. Further aiding research, pretrained models and evaluation scripts are also available, which can streamline the process of audio recognition studies.
During dataset creation, they built a scalable pipeline, incorporating image classification algorithms. This helped to filter out irrelevant audio and improve the overall quality of the data collected. However, it's important to acknowledge that YouTube content inherently includes a wide range of audio quality and noise levels, which presents an ongoing challenge for research into robust audio-visual processing. While a valuable resource for understanding connections between audio and visual components of video, the inherent complexity and noise of real-world YouTube content can pose a hurdle for model training and evaluation. Nevertheless, VGGSOUND offers a chance to explore numerous real-world audio-visual tasks that might translate into future innovations in fields like content understanding and even robotics, where understanding both sight and sound is key.
7 Lesser-Known Public Dataset Sources for Video Analysis Research in 2024 - DAVIS Dataset For Object Segmentation In Unconstrained Videos
The DAVIS dataset is specifically designed for the challenging task of segmenting objects within unconstrained video sequences. It offers 50 video sequences, totaling over 3,400 densely annotated frames, presented in both 480p and 1080p resolutions. These sequences are carefully partitioned into training and validation sets, each featuring a variety of situations designed to test the efficacy of segmentation algorithms. The dataset's design allows researchers to evaluate object segmentation performance under circumstances like partial occlusions and object appearance changes, which are common in real-world videos.
The dataset gained prominence through the 2017 DAVIS Challenge, a competition that aimed to spur progress in video object segmentation. The competition itself, and the dataset it's built around, have been crucial in driving improvements to video segmentation algorithms, evidenced by numerous state-of-the-art models that leverage the dataset. Rigorous evaluation metrics accompany the dataset, ensuring a standard for comparing different approaches to segmentation. While the DAVIS dataset is valuable for driving research, it's important to consider that the complexity of object segmentation in real-world situations is quite nuanced, and the dataset may not encompass every possible variation in visual conditions. It's thus critical to approach interpretations of results obtained using this dataset with caution, considering the possibility of inherent limitations that could impact the generalization of findings to entirely new video situations.
The DAVIS dataset, focused on Discriminative Video Object Segmentation, offers a collection of over 5,000 video sequences showcasing a wide variety of objects meticulously segmented frame by frame. This makes it particularly useful for researchers tackling intricate video object segmentation challenges with a high degree of precision.
A key differentiator of the DAVIS dataset is its pixel-level annotations, which include details about both the object and its surrounding background. This level of granularity goes beyond the usual bounding box or region-level annotations found in many other datasets, enabling the development of more sophisticated segmentation models.
The video clips within the dataset are sourced from various real-world settings, including wildlife, sports, and urban scenes. This diverse range of scenarios makes it a valuable tool for evaluating how well models trained on the dataset generalize to different real-world environments beyond its curated content.
The DAVIS dataset tackles both single and multiple object segmentation challenges, allowing researchers to investigate how well different methods handle various levels of complexity. This dual approach to segmentation helps researchers develop more robust algorithms that can manage the intricate interplay of objects within dynamic video scenes.
The dataset also introduces evaluation metrics specifically tailored for video segmentation tasks. These metrics, including the J and F measures, are designed to overcome the unique difficulties encountered in dealing with video data. Consequently, they provide a more nuanced understanding of a model's performance across different frames.
Interestingly, the annotation process for the dataset combines manual segmentation with automated object tracking methods. This hybrid approach not only accelerates the annotation process but also ensures that segmentations are consistently high-quality across the dataset.
The DAVIS dataset has been instrumental in advancing the field of video object segmentation, helping to enhance several cutting-edge segmentation algorithms. Researchers have noted significant improvements in model performance when using DAVIS for training and validation purposes.
A noteworthy strength of DAVIS is its emphasis on temporal continuity. By meticulously capturing and preserving movement information over video sequences, it addresses a facet often overlooked in static datasets. This characteristic is critical for developing algorithms that leverage temporal context to understand how objects move over time.
While the dataset's quality and diversity are impressive, some researchers have pointed out limitations in the types of objects it features. More specifically, it could potentially benefit from including a wider range of occluded or overlapping objects, which are common challenges in real-world scenarios.
As of November 2024, the DAVIS dataset continues to evolve, with community-led initiatives seeking to expand its scope by incorporating new video clips and annotations from diverse sources. This ongoing development suggests the DAVIS dataset will continue to be a relevant resource as the applications of video analysis become increasingly diverse.
7 Lesser-Known Public Dataset Sources for Video Analysis Research in 2024 - Hollywood2 Dataset With Human Actions From Hollywood Movies
The Hollywood2 dataset is a valuable resource for evaluating how well algorithms can recognize human actions in realistic settings. It's built using video clips extracted from 69 different movies, covering a range of human actions like driving, eating, and hugging. Specifically, it contains 3,669 video clips classified into 12 action categories, offering a diverse set of human behaviors as observed in film. Importantly, each action is annotated, making it possible to rigorously study how well machines, compared to humans, can identify these actions.
Hollywood2 is part of a larger movement in video analysis research that relies on movie scenes to help create more robust algorithms for understanding human behavior. It acts as a complement to similar datasets like HMDB and UCF101, allowing for research into more sophisticated action categories. This is particularly helpful for developing machine learning models for recognizing and classifying actions within dynamic scenes.
While Hollywood2 provides a unique opportunity to investigate complex actions, it's crucial to remember that the dataset's origins in movies might make it less suitable for understanding completely natural human behavior. This is because movie scenes are often stylized and might not perfectly represent how people typically move or act in everyday situations. Nonetheless, Hollywood2 remains a useful tool for advancing the field of action recognition and further refining related machine learning techniques.
The Hollywood2 dataset, a collection of video clips from 69 movies, offers a unique perspective on human action recognition. It's interesting that it draws from the world of cinema, rather than just real-world recordings, suggesting that even stylized content can provide valuable data for studying how people move and interact. The dataset includes over 3,000 clips categorized into 12 action classes, spanning a broad range of behaviors like fighting, hugging, and running, making it useful for training models on a diverse set of actions.
One of the notable features is that the clips capture action in a temporal sequence. This means you're not just looking at a frozen moment, but the progression of an action over time, providing a valuable opportunity to analyze movement continuity and how actions unfold within a narrative structure. While this dynamic representation of actions is beneficial, it's important to remember that movie scenes are often staged or dramatized, which might introduce some bias. It remains to be seen how well models trained on this data generalize to more typical, everyday scenarios.
Another crucial aspect is the meticulous annotation provided. Actions are labeled every 3 seconds, allowing for a fine-grained analysis of action timing and sequence. This precision in temporal annotation is crucial for understanding how humans behave within dynamic environments. It's worth noting that the dataset is primarily focused on individual, atomic actions, rather than complex chains of events. This creates a challenge for researchers to build models capable of not only recognizing individual movements but also understanding them within a broader context.
Hollywood2's diversity extends beyond its range of actions. It includes a wide variety of actors, providing a rich environment to explore how individual characteristics, such as body language and movement style, might impact action recognition performance. Furthermore, the films represented come from various genres and cultures, presenting a potential for cross-cultural studies of human behavior. Researchers can examine how the same actions might be expressed differently across cultures and languages.
It's interesting to consider the potential applications of the Hollywood2 dataset beyond just refining computer vision algorithms. It might prove valuable for industries like gaming and virtual reality, as it provides a library of dynamic actions that could be used to create more realistic and responsive interactions within virtual environments. Moreover, combining Hollywood2 with other datasets could lead to significant improvements in model generalization across different contexts. It offers a rich, stylized window into human actions, potentially complementing more grounded datasets and helping models learn to identify actions across a broader spectrum.
Overall, the Hollywood2 dataset provides both opportunities and challenges for researchers interested in understanding human action recognition. Its unique approach of drawing from cinema provides a valuable, albeit somewhat biased, lens through which to study human movement. Recognizing both the strengths and potential limitations of the data is crucial for pushing forward in the field of video analysis.
7 Lesser-Known Public Dataset Sources for Video Analysis Research in 2024 - Epic Kitchens 100 Dataset For First Person Action Recognition
The Epic Kitchens 100 dataset is a substantial resource specifically designed for first-person action recognition within the field of egocentric vision. It expands upon the earlier Epic Kitchens 55 dataset, significantly increasing the volume of data available for research. Comprising 100 hours of footage, it captures over 90,000 actions across roughly 20 million frames, offering a wide variety of kitchen-related activities. This data was gathered by having 32 individuals wear head-mounted cameras in their own kitchens while undertaking typical daily tasks, providing a natural and unconstrained view of actions.
A noteworthy aspect of the dataset is the annotation process. The developers employed a unique method called "Pause-and-Talk," which involves participants narrating what they are doing during the recorded activities. This process yields richer contextual information alongside the action sequences. The dataset serves as a benchmark for several important tasks in action recognition, including tasks with full and weak supervision, as well as object detection, action anticipation, and caption-based video retrieval. Despite its considerable size and utility, some limitations remain. The diversity of real-world kitchen scenarios can still be limited, and scaling the dataset for increasingly complex models and algorithms remains a challenge. However, Epic Kitchens 100 plays a pivotal role in addressing the historical lack of large-scale, egocentric datasets. It's expected to contribute significantly to future advancements in video analysis and action recognition research.
The EPIC KITCHENS 100 dataset is an extension of the earlier EPIC KITCHENS 55 dataset, designed for understanding actions in first-person videos—also called egocentric vision. It's a substantial collection of around 100 hours of video, totaling roughly 20 million frames and encompassing approximately 90,000 actions recorded across 700 videos of varying lengths. These videos capture unscripted kitchen activities, recorded using head-mounted cameras worn by 32 different people in their own kitchens. This gives researchers a chance to analyze real-world cooking scenarios rather than overly controlled or staged ones.
The dataset uses a unique "Pause-and-Talk" narration interface for annotation, enriching the data with detailed information on the activities and objects involved in each scene. It supports six common benchmarks for action recognition research, ranging from fully supervised and weakly supervised action classification tasks to action detection, anticipation of actions, retrieving videos based on written descriptions (captions), and even adapting models to new video sources (domains). In addition to these action-centric tasks, it has potential for other types of analysis like object identification and how people interact with objects, which can help expand our understanding of how people use tools in various situations.
This dataset tackles some of the long-standing hurdles in analyzing first-person videos, including providing large-scale data for building robust action recognition systems. However, like other real-world datasets, it introduces challenges stemming from differences in lighting, camera angles, and occasional obstructions (occlusions). The variability of the human subjects themselves also raises questions about how well recognition models will perform across various body types, movement styles, and personal approaches to cooking. Some researchers have also noted the lack of complex social interaction in cooking—such as when multiple people are collaboratively preparing a meal—which may be a limitation in certain kinds of action recognition tasks.
Despite these complexities, EPIC KITCHENS 100 is publicly available, making it an accessible resource for the wider research community. Its potential for research extends beyond action recognition. It can be effectively paired with other video datasets which focus on aspects of human activity not strongly represented in this dataset. This combination could potentially lead to the development of hybrid approaches that may improve performance in the field of action recognition models. While it certainly addresses the need for more large-scale egocentric datasets, the challenges posed by its real-world nature remain a key research topic in improving the performance of video analysis systems. Since its initial release in 2018, it has grown in prominence as one of the largest and most widely used benchmarks for egocentric video analysis, pushing forward the development of more accurate and robust AI models for understanding and responding to human activities in videos.
More Posts from whatsinmy.video: