Analyze any video with AI. Uncover insights, transcripts, and more in seconds. (Get started for free)
7 Real-Time Image Transformations Reshaping Video Processing in 2024
7 Real-Time Image Transformations Reshaping Video Processing in 2024 - Neural HDR Video Processing Makes Low Light Footage High Quality
The ability to transform poorly lit video into high-quality footage is becoming increasingly important, and neural HDR video processing is a key technology driving this change. These systems use sophisticated deep learning techniques, including attention mechanisms, to refine details and suppress noise, leading to significantly better video in challenging lighting situations. This is especially crucial in areas like surveillance and autonomous driving, where clear, precise video is essential for safe and effective operation.
Traditional methods of improving low-light videos struggle to deliver acceptable results in real-time, particularly with the higher resolutions now common. This is where neural network approaches excel, offering the potential to handle high frame rates and resolution. Ongoing research into these methods is necessary as the field progresses, promising a future where capturing clear and detailed video, even in difficult lighting, becomes more commonplace.
Neural HDR video processing is an exciting development leveraging deep learning to transform low-light footage into a higher quality, more visually appealing format. It essentially involves training neural networks on a massive collection of images and videos across various lighting situations. This training process allows the networks to master the art of emphasizing details hidden within shadows while simultaneously maintaining the integrity of brightly lit areas. The result is a significant boost in visibility without the typical noise artifacts often associated with manipulating low-light images.
The approach often utilizes convolutional neural networks, which are adept at analyzing spatial information within video frames. By learning from the diverse training data, these networks can cleverly select the most relevant frames and time periods to reduce the appearance of motion blur, particularly advantageous for scenes filled with rapid movement. Some systems take this a step further with multi-frame processing, effectively combining data from consecutive frames to enrich detail and texture in a manner that mimics how the human eye processes visual information under challenging conditions.
But it’s not just about enhancing brightness. These techniques integrate color calibration methods to guarantee accurate color reproduction despite the limited light. Furthermore, by incorporating temporal filtering techniques, the latest developments are pushing the boundaries of neural HDR to minimize the introduction of artifacts caused by sudden shifts in light or subject motion. This contributes to a more seamless and enjoyable visual experience for viewers.
A fascinating implication of this technology is the ability to implement it in real-time broadcasting scenarios. This opens up possibilities for live event and sports coverage where environments may be poorly lit. Imagine the quality enhancement for night-time football games or dimly lit concerts! Besides enhanced visibility, the neural HDR process can subtly accentuate depth cues, helping the viewer better assess distances in scenes captured under challenging light conditions.
The increased accessibility of hardware acceleration, particularly with the emergence of AI-specific processors, is making real-time neural HDR processing a reality in consumer devices without the need for excessive computational power. This shift in accessibility is a key factor for wider adoption. However, it is crucial to be aware of the limitations. Ongoing training of the neural networks is essential to keep pace with new lighting conditions and environments, which can be computationally expensive and requires a continuous stream of high-quality data. This continuous adaptation remains a critical challenge moving forward.
7 Real-Time Image Transformations Reshaping Video Processing in 2024 - DeepStream Edge Computing Reduces Video Processing Time by 40%
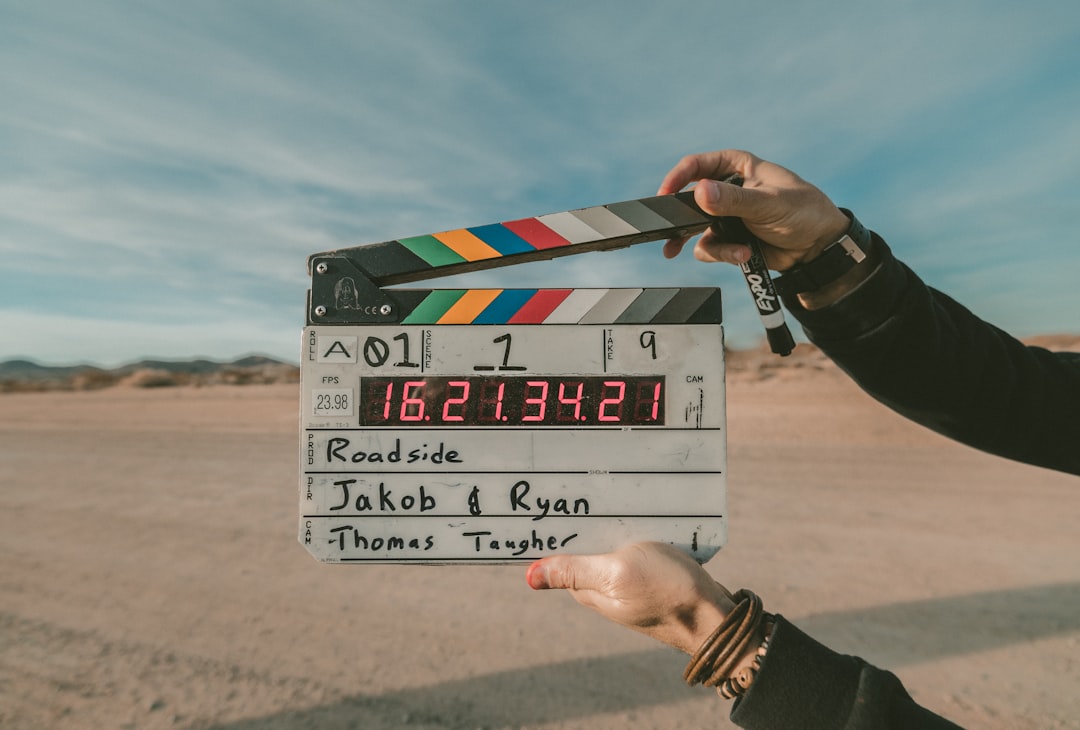
DeepStream edge computing offers a compelling solution for accelerating video processing, potentially reducing processing times by as much as 40%. This approach is particularly beneficial for real-time video analytics, allowing for swifter processing of data at its source rather than relying on centralized cloud systems. Methods like SplitStream, a distributed processing framework, demonstrate how edge computing can improve efficiency and device utilization. The closer proximity of edge computing to the data source itself helps meet the strict demands of real-time video analysis, a significant advantage over cloud-based processing that often introduces delays. Furthermore, it potentially opens doors for improved performance in future AI applications tailored for video processing. However, it's worth acknowledging that edge computing faces its own limitations in terms of available processing power, which necessitates careful consideration when deploying it for video analytics tasks.
DeepStream, a technology that pushes video processing to the edge of the network, offers a compelling approach to speeding up video analysis. Researchers have observed that using DeepStream can reduce the time it takes to process video by up to 40%. This is particularly important for tasks like real-time security monitoring and autonomous driving, where immediate insights from video data are critical.
One of the primary reasons for this improvement is the reduced need to send vast quantities of raw video data back to a central server for processing. Handling video processing at the edge means we can process data closer to where it is generated, which also minimizes bandwidth usage and can potentially lower operational costs related to data transfer.
Intriguingly, these edge devices using DeepStream can achieve processing power comparable to traditional server-based solutions while consuming less energy. In applications like industrial automation or remote sensor networks where power efficiency is a major factor, this can be a key advantage, allowing devices to operate for extended periods without needing frequent power replenishment.
DeepStream's ability to analyze multiple video streams simultaneously is another interesting development. It opens the door to more sophisticated surveillance systems, for example, where several cameras are used to monitor a larger area. The fact that it can achieve this without major performance degradation is noteworthy and points towards greater flexibility in system design.
While DeepStream has shown promise, some challenges remain. The need to continuously adapt to changing conditions and varying video quality presents ongoing research opportunities. It also utilizes GPU processing power, which while offering a significant speed boost, highlights the need to consider the specific computational resources required at the edge in order to be efficient. Further development and refinement are likely needed to fully explore the scope of what DeepStream and other edge computing approaches can offer.
7 Real-Time Image Transformations Reshaping Video Processing in 2024 - Real Time Style Transfer Adds Film Looks While Recording
Real-time style transfer is bringing new creative possibilities to video recording by allowing users to apply the look of classic film stocks while capturing footage. Powered by sophisticated neural networks, this method can now handle high resolutions and frame rates, ensuring that the artistic touches applied don't compromise the details in the original video. The technique intelligently transfers the style from one part of a video to another, helping maintain a consistent visual flow and avoid jarring shifts or flickering effects. This transfer can be controlled automatically or manually through user input, providing flexibility for a wider range of artistic choices. The ability to achieve these stylistic effects during recording is transforming video production across many genres, enabling creators to achieve a wider variety of artistic looks in their work. However, it's still early days, and the quality of results can vary and there are still some technical limitations that remain to be addressed. Overall, real-time style transfer is an emerging technology with potential to significantly change the creative process in video recording.
Real-time style transfer, using the power of deep neural networks, is emerging as a way to inject cinematic aesthetics into video recordings. These algorithms are trained on vast amounts of data capturing a range of film styles and artistic looks. They can then analyze and extract these visual styles, applying them dynamically to live footage while attempting to retain important details.
However, achieving this in real-time comes with a significant computational burden. The complexity of the process demands powerful hardware capable of handling the complex calculations with minimal delay. There's an inherent tension between the desired quality of the film look and the speed at which it can be processed, especially as video resolution increases. The neural network training itself is a major undertaking. It requires sophisticated learning techniques to handle the wide diversity of visual elements that might be encountered, and also ensure visual consistency across frames to prevent any flickering artifacts.
One notable characteristic is the ability to integrate artistic styles without sacrificing the fundamental content of the original video. Methods that adaptively adjust the style transfer based on the video content help to keep the viewer's attention focused on the subjects of the video, even when applying extreme visual changes. The impact on live streaming and online content creation is remarkable. It empowers creators with the ability to apply a unique visual signature to their live broadcasts, potentially enhancing audience engagement in a way that wasn't possible before.
Despite the impressive advancements, latency issues are still a hurdle to overcome. The speed of style transfer can still lag behind the live video feed, which is a particular concern in fast-paced scenarios such as live gaming or sports where instantaneous response is crucial.
To further enhance the user experience, some systems now allow individuals to customize their preferred cinematic styles by selecting from pre-built visual effects. While appealing, this feature requires the system to dynamically adapt to a wide array of stylistic choices in real-time, further increasing the computational challenge.
Moreover, researchers are working to improve the responsiveness of these algorithms so they can seamlessly handle changes in lighting, scene composition, and subject movement. Ideally, the style transfer would adapt dynamically without disrupting the viewing experience, creating a more fluid integration of aesthetic effects.
It's also encouraging that recent advancements in hardware acceleration, specifically AI-focused GPUs, are helping to address the heavy computational needs of real-time style transfer. These processors are designed to handle the specific types of calculations involved, relieving pressure on general-purpose CPUs and leading to smoother and more efficient performance.
Looking forward, researchers are exploring ways to expand the concept of style transfer beyond simple aesthetic changes. It's possible that future systems might incorporate a mix of different media types within a single video frame, blending live-action with animated elements. While this presents the possibility of incredibly creative storytelling techniques, it also brings into question how well we, as viewers, will adapt to and appreciate the melding of diverse visual styles.
7 Real-Time Image Transformations Reshaping Video Processing in 2024 - Motion Blur Reduction Through Frame Rate Optimization
Motion blur, a common issue in video, particularly when capturing fast-moving objects, is being tackled through intelligent frame rate optimization. Recent innovations allow for the reconstruction of videos from a single motion-blurred image by simply changing the desired frame rate. This dynamic adjustment, made possible by sophisticated neural networks, presents a novel approach to restoring clarity. The core idea is to treat the problem of recovering a clean sequence of images from a blurred one as an inverse problem, simultaneously figuring out the underlying movement within the scene. Deep learning techniques are rapidly improving in their ability to perform image restoration, significantly outperforming older methods. This allows for real-time enhancement of video content with much finer detail and sharper images than previously possible.
There are, of course, difficulties in developing these advanced systems. Computational cost is a critical hurdle, as these sophisticated algorithms can be very demanding on processing power. Finding the best balance between efficiency and the high-quality results we want in real-time video remains an ongoing challenge for researchers. As technology evolves, we can expect even better solutions to reduce motion blur, potentially reshaping the way we capture and experience video content.
Motion blur, a common visual artifact in videos, particularly during fast-paced action, is increasingly addressed by optimizing frame rates. Our eyes perceive motion differently depending on the number of frames shown per second, or FPS. A video with a higher FPS like 30, generally appears smoother than one at 24 FPS, even if the scene content is identical, showcasing how frame rate significantly impacts the perception of motion blur.
This smoother perception arises from persistence of vision, a quirk of human biology where our eyes retain an image for a short time after it's gone. By displaying more frames, we maintain the visual information for longer periods, leading to a more continuous, blur-free viewing experience. This aligns with temporal sampling theory which suggests that higher sampling rates capture a more accurate representation of movement, leading to sharper images, especially during rapid motion.
Interestingly, the effectiveness of frame rate optimization isn't uniform across individuals. The critical flicker fusion threshold, the point at which our eyes perceive a flickering light as a steady light, varies between about 50 and 90 Hz per person. This individual variation plays a role in how well motion blur is perceived and minimized with increased frame rates.
Adaptive frame rate control systems, designed to dynamically adjust frame rates based on the complexity of the scene and how much motion is occurring, show great potential for optimization. These systems aim to use processing power only when needed, avoiding unnecessary computation during less demanding parts of the video.
Even the choice between interlaced and progressive scanning significantly impacts motion blur. Progressive scanning offers a clearer image compared to interlaced, by updating the full screen at once, avoiding the potential artifacts associated with interlaced scan lines. However, achieving optimal frame rate benefits faces technical constraints. Consumer display technology is limited by physical factors impacting the speed at which it can refresh, limiting the effectiveness of these techniques. Displays with high refresh rates—120Hz and above—are needed to fully realize the benefits of high frame rates.
AI is also being leveraged in frame interpolation, a technique to predict and generate frames between existing ones, filling the gaps in motion. This holds great potential for reducing motion blur; however, these techniques can introduce their own artifacts if not handled carefully.
Frame rate optimization is crucial in areas like gaming, where responsiveness and clarity are essential for player performance and a quality experience. In fast-paced games, high frame rates are vital to maintaining a competitive edge and creating a seamless and immersive experience.
Besides visual clarity, frame rate optimization also interacts with psycho-visual effects in video processing. These effects influence how we perceive depth and reduce the discomfort we may feel when there are rapid motion changes, further shaping the overall viewing experience. While this field continues to evolve, optimizing frame rates holds tremendous promise in addressing a significant challenge in video processing.
7 Real-Time Image Transformations Reshaping Video Processing in 2024 - Object Detection Now Works on Moving Targets at 120 FPS
Object detection, the ability to identify objects within a video, is now capable of tracking moving targets with incredible speed and precision, achieving frame rates of 120 frames per second. This is a significant leap forward, particularly for applications demanding real-time insights, like autonomous driving or security systems. The speed increase is a result of advanced algorithms, with some like YOLOv7 leading the way in both accuracy and processing speed.
While impressive, the accuracy of these detection systems has been improved through the use of techniques such as data augmentation. These methods allow for the training of these models using more diverse and varied datasets, thereby improving their robustness in real-world conditions. This focus on accuracy is especially important when these object detection systems are used in applications that may have significant safety implications.
As the field of video processing develops, this increase in speed and accuracy of object detection holds significant potential. We can expect this capability to be further refined and integrated in areas like robotics and even urban planning where efficient object tracking is vital for managing complex systems and improving outcomes. While it remains to be seen exactly how far this technology will progress, it is clear that the future of object detection in video is rapidly evolving.
Object detection, a core component of computer vision, has seen impressive strides in recent years, particularly with the development of systems that can now achieve 120 frames per second (FPS) while still accurately identifying objects, even in motion. This is a big jump from earlier systems which typically struggled to reach a sustained 30 FPS. The ability to process images so rapidly opens doors for real-world applications in diverse fields like self-driving cars and robotic systems where swift, precise object identification is critical.
The key to hitting these high frame rates lies in a combination of optimization techniques applied to deep learning models. Quantization and pruning, for instance, are methods that streamline the model without sacrificing much in the way of accuracy. These clever optimizations are crucial for making high-FPS object detection feasible on less powerful hardware like those used in edge devices or embedded systems.
Moreover, motion compensation has become increasingly important for keeping up with moving targets. Sophisticated algorithms anticipate the paths of objects, allowing detection systems to stay on target without experiencing significant lag, a crucial requirement for applications like security cameras that need to track activity in real-time.
Furthermore, reducing latency is another crucial element in these new systems. Through specialized techniques, the time it takes for an image to be captured, processed, and a response returned is kept extremely low. This is vital for applications operating in dynamic environments where responses need to match the pace of motion as closely as possible.
The ability to run these computationally intensive models at such high frame rates relies significantly on the use of specialized hardware accelerators. Dedicated graphics processing units (GPUs) and tensor processing units (TPUs) excel at parallel processing, which is necessary for deep learning models. This enables us to achieve better performance while managing energy consumption, an important factor for battery-powered devices.
Interestingly, some advanced object detection systems dynamically adjust their frame rate based on the complexity of the scene. This adaptability allows them to conserve resources in simpler scenes or when less information needs to be processed, increasing efficiency and maximizing performance when it's needed.
The algorithms themselves have been enhanced with capabilities that incorporate more contextual awareness. These algorithms can now better understand the scenes and predict the movements of objects using previous frames, significantly improving overall reliability of object detection.
The rise of large and varied datasets, encompassing a wide range of real-world scenarios and lighting conditions, has been an essential ingredient in the training process. The greater variety in training data allows these models to better generalize when confronted with unseen situations or unique environments, essential for real-world use cases.
These advancements in object detection extend beyond simple object identification. Modern systems now possess the capability to track multiple objects simultaneously while maintaining their individual identities over time. This is incredibly useful in applications like surveillance or robotic navigation as it enables more robust tracking of objects within dynamic scenes.
In the end, all these improvements in object detection contribute to a far more seamless user experience in a variety of applications. Whether it's video games, augmented reality applications, or driver assistance systems, the ability to quickly and accurately identify objects without lag leads to more intuitive and compelling interactions with technology. This advancement promises to shape how we interact with the world around us in increasingly sophisticated ways.
7 Real-Time Image Transformations Reshaping Video Processing in 2024 - Depth Mapping Creates 3D From 2D Footage in Camera
Depth mapping is a technology that's transforming the way we experience video by taking 2D footage and converting it into a 3D format in real-time. Essentially, it generates a depth map, a sort of 2D representation of the 3D scene, that reveals how far away each part of the scene is from the camera. Tools like Apple's Depth Pro can produce highly detailed depth maps very quickly, even from single images. This allows for a much richer representation of the depth within a scene, enabling things like the integration of live-action actors into virtual worlds, and enhancing the immersive feel of video content.
Methods like SLAM (Simultaneous Localization and Mapping) further illustrate the potential of real-time depth mapping, allowing us to use a single camera to track our position in space and create a detailed 3D map of the surrounding environment. This opens up exciting possibilities for augmented reality and other immersive technologies.
However, while depth mapping technology shows promise, there are still obstacles to overcome. The computational demands of generating high-quality depth maps can be substantial, and the accuracy of these representations still needs improvement. As we move forward in 2024, the use of depth mapping is likely to increase in various applications, shaping the way we create and consume video content and potentially pushing the creative boundaries of video processing.
### Depth Mapping Creates 3D From 2D Footage in Camera
Depth mapping, a technique rooted in stereo vision, is transforming how we perceive 2D video footage. It fundamentally relies on the concept of disparity, using either dual lenses or structured light patterns to calculate the distance of objects from the camera. By essentially triangulating the scene, it creates a depth map—a 2D representation of a 3D scene—providing valuable spatial information for each point captured. This information is then used to construct 3D models, fundamentally converting standard 2D video into richer, more immersive 3D experiences.
We've seen a considerable leap in the capabilities of depth mapping with its ability to operate in real-time. This real-time processing is essential for applications such as AR and VR, where instantaneous depth information is critical for overlaying digital content onto the real world or immersing viewers in virtual environments. While not a new concept, the introduction of machine learning has further refined this technology. These algorithms are able to continuously improve the accuracy of the depth maps, allowing systems to adapt better to diverse lighting conditions and complex scenes. This is particularly useful in overcoming some of the classic challenges of depth mapping such as dealing with occluded objects or reflective surfaces which can confuse the algorithm.
Some systems employ a clever technique called structured light projection. It involves projecting a structured pattern onto a scene and then analyzing the deformation of this pattern as it interacts with objects. This approach enables precise 3D reconstruction without requiring complex multi-camera setups and has found use in a variety of applications. Furthermore, advanced algorithms can ensure temporal cohesion in the estimated depth, meaning that dynamic scenes, such as a sports broadcast, can have their 3D reconstruction updated in real-time to track moving subjects.
Interestingly, depth mapping is increasingly accessible in consumer electronics, like smartphones and standard cameras. This accessibility is rapidly changing the landscape of video creation, offering users a relatively easy way to produce 3D footage. It's truly democratizing the technology, allowing both professionals and hobbyists alike to explore new dimensions in their video productions. This easier accessibility has further fueled a rise in using this technology in visual effects in filmmaking. It enables precise control over the depth of field, helping filmmakers guide the viewer's attention to specific elements in the scene, far surpassing traditional depth-of-field controls.
While very promising, this technology is not without its challenges. Fine detail is sometimes lost in the transition from 2D to 3D, especially if the depth map estimation algorithm struggles with texture or proximity assessments, leading to a less than optimal viewing experience in certain cases. Another emerging area of research is the impact depth mapping has on video compression algorithms. Given the growing use of 3D content, efficient compression is increasingly important for streaming. If depth maps can be used to better guide the compression process, this could lead to smaller file sizes while maintaining high quality.
Finally, the marriage of depth mapping and AI, specifically within robotics, is incredibly exciting. With depth data, robots gain a much more intuitive sense of the physical world, allowing them to better navigate complex environments and interact with objects. This has significant implications for the fields of autonomous vehicles and industrial automation, showcasing how depth mapping can be so much more than just an aesthetic tool. It’s shaping our interactions with technology and leading to potentially disruptive advances across various disciplines.
7 Real-Time Image Transformations Reshaping Video Processing in 2024 - Smart Background Replacement Without Green Screens
The ability to seamlessly change video backgrounds without relying on cumbersome green screens is becoming increasingly accessible through smart background replacement technologies. These advancements, utilizing AI and other sophisticated algorithms, are making video editing and content creation more intuitive. Solutions like ChromaCam and XSplit Vcam highlight the potential for real-time background replacements, opening up new opportunities for live streams, video calls, and a range of other video-based applications.
Tools like AI Video Background Remover and BIGVU simplify the process of removing or substituting backgrounds, often with a single click, allowing users to focus on the main subject matter in their videos. While user-friendly interfaces are becoming more common, challenges remain in ensuring accurate background removal and ensuring the computational demands are low enough for real-time processing, particularly on less powerful devices.
Despite these obstacles, the rise of these smart background replacement tools demonstrates a promising trend in video production, offering a simpler and more accessible method for crafting compelling visuals across various formats. It's a technology poised to change the creative landscape for a broader spectrum of video creators.
The ability to seamlessly replace video backgrounds without the need for bulky green screens is becoming increasingly sophisticated thanks to recent advancements in AI and computer vision. These systems rely on a number of factors, pushing the boundaries of real-time image transformations.
One key development is the ability to handle variations in lighting in real-time. Algorithms can now estimate depth and analyze the overall scene to intelligently modify background lighting, ensuring it integrates seamlessly with the foreground subjects. This enhances realism in ways that were previously impossible without green screens.
Another significant factor is the accuracy of object segmentation. Deep learning-based methods are increasingly able to precisely define the boundaries of foreground subjects, even when the subjects have intricate outlines or are in complex backgrounds. This allows for a sharp and clean separation of the subject from the background. What's interesting is that some systems can even handle multiple individuals in a frame, creating independent image layers and allowing each individual's background to be modified without affecting others.
Facial recognition is also contributing to better quality. Systems are able to identify key facial features and expressions in real-time, assisting in maintaining correct scaling and positioning during background changes. This helps prevent a sense of detachment between the subject and the new background, contributing to a more natural overall appearance.
The emergence of edge AI is also playing a vital role in making these systems practical for use cases like live streaming. Moving the bulk of the processing from the cloud to local devices significantly reduces the latency associated with background replacement, crucial for applications where responsiveness is vital.
This technology is also being integrated into other visual effects, creating interesting combinations. Backgrounds can be dynamically manipulated based on the content and movements of the foreground subjects, opening up potential for more creative visual storytelling approaches. It’s also noteworthy that several of these systems allow users to fine-tune background replacement through user interfaces, empowering creators with more control over the final aesthetic.
Beyond this, we're starting to see the potential role of biometric data in refining background replacement further. These systems are learning to use things like body shape and movement patterns to create a more organic feel when integrating backgrounds. This may seem small, but these nuances are essential for a more realistic and visually appealing final result.
Interestingly, we're seeing the adoption of smart background replacement in video conferencing tools, a direct result of the increase in remote work. This eliminates the need for physical green screen setups for more professional-looking video calls, which is beneficial for improving workplace interactions and perceptions.
Finally, the training data used to train the AI models in these systems is constantly evolving. As models are exposed to increasingly varied data from a wider variety of environments and subjects, their ability to handle unfamiliar scenes during live use cases improves dramatically.
While we are still in the early stages of exploring the potential of these AI-driven background replacement methods, it is clear that it has the potential to become an essential tool for video editing, broadcasting, and communication in 2024. It will be interesting to see how this technology continues to develop and be integrated into our daily lives.
Analyze any video with AI. Uncover insights, transcripts, and more in seconds. (Get started for free)
More Posts from whatsinmy.video: