Analyze any video with AI. Uncover insights, transcripts, and more in seconds. (Get started for free)
Navigating the Starter Assessment Key Insights for Data Annotation Newcomers in 2024
Navigating the Starter Assessment Key Insights for Data Annotation Newcomers in 2024 - Understanding the Core Assessment Structure
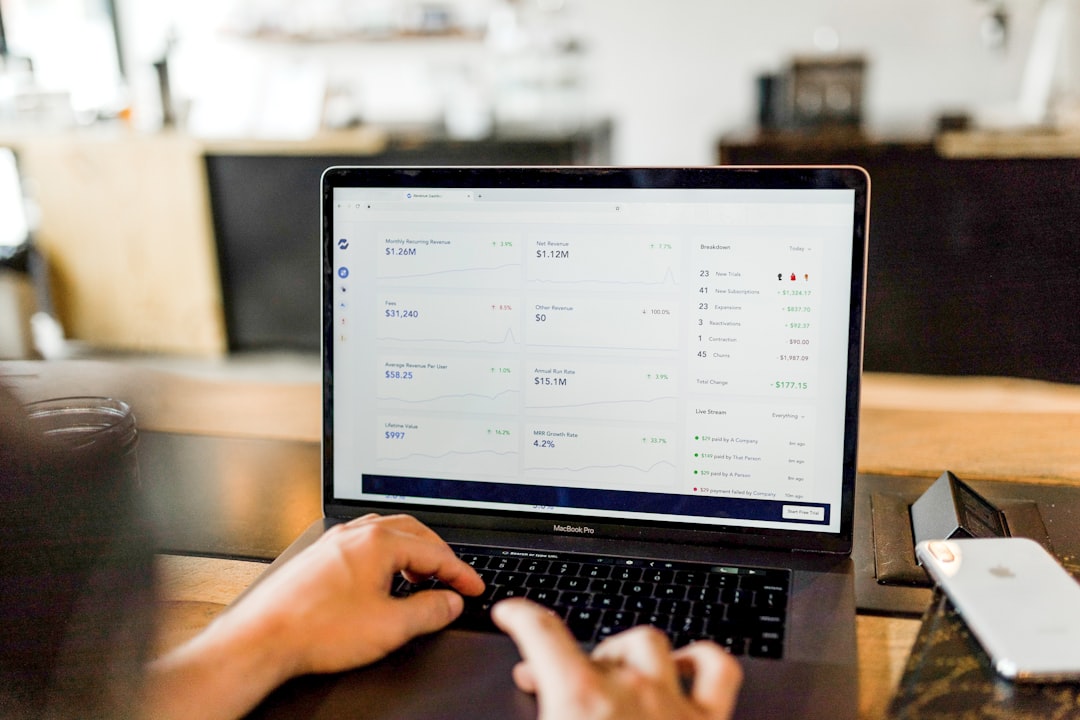
To succeed in the Data Annotation Starter Assessment, comprehending its structure is paramount. This initial evaluation focuses on your ability to accurately label diverse data types. This often involves tasks like identifying objects within images or categorizing sections of text, providing a clear snapshot of your data annotation skills. The assessment process generally involves reviewing prompts alongside sample responses, requiring you to judge their quality, appropriateness, and adherence to guidelines. You'll need to evaluate whether the responses are good, acceptable, or flawed and even determine if they present problematic content. These tasks aren't arbitrary—they reflect the vital role data annotation plays in training AI systems, which are increasingly impacting various sectors. By grasping the key principles behind the assessment structure, newcomers gain a crucial advantage in demonstrating their aptitude for this emerging field and subsequently securing relevant roles. It's worth noting that the criteria can be quite specific, emphasizing the need for attention to detail and adherence to instructions.
The core assessment for data annotation often involves looking at different types of data, like images and text, to get a full picture of how someone tackles annotation tasks. This multi-faceted approach, instead of focusing on just one type of data, aims for a deeper understanding, potentially leading to more accurate results in the assessment.
One common element is the use of pre-existing datasets as benchmarks. These serve as a yardstick, comparing the results of a candidate's annotations against a standard. This allows for a more objective way to assess how well a person is performing the task and provides a structured method for quantitative evaluation.
In crafting these assessments, a lot of consideration goes into the mental strain the tasks place on the annotator. This aligns with cognitive load theory where the goal is to find a sweet spot-tasks that are challenging enough to be engaging but not so hard they become overwhelming. Striking this balance can be tricky, but ideally enhances accuracy and effectiveness.
Evaluating performance can be done through a variety of measures. Precision, recall, and F1 score are commonly used statistical tools for this purpose. They provide a concrete way to gauge an annotator's performance and identify areas for improvement through targeted training.
Reducing bias is important in assessment. This often involves blinding the reviewers to aspects like the annotator's identity or any other potentially influencing factor. This “blinded” process intends to limit the impact of human subjectivity, ensuring that the annotation output is judged on its own merits.
A successful assessment is designed so different people judging the same data would give similar answers. This concept of inter-rater reliability is vital in data annotation as it helps ensure data quality consistency. Ideally, the variation between different annotators is minimal, giving us confidence in the annotation outcomes.
Increasingly, the assessments are incorporating real-time feedback. This immediate feedback allows annotators to understand their progress as they work and make improvements in the moment. This iterative process can lead to significant improvement in annotation skills over time.
By comparing the work of multiple annotators, we can see patterns emerge that might indicate biases or systemic errors in the approach. This can guide project managers or trainers in adjusting the training materials or assessment structure, refining the annotation workflow to address these potential issues.
A common feature of these assessments is the ability to predict future performance. This predictive element draws upon past annotation data and helps understand individual strengths and weaknesses before they are involved in large scale projects.
Using a graded system or a tiered evaluation is a valuable approach. This enables differentiating between less experienced and more skilled annotators, tailoring the assessment process to suit various levels of expertise. This not only helps identify suitable candidates, but also ensures that the evaluation process is constructive, promoting learning throughout the annotation journey.
Navigating the Starter Assessment Key Insights for Data Annotation Newcomers in 2024 - Preparing for Creative and Technical Evaluation Tasks
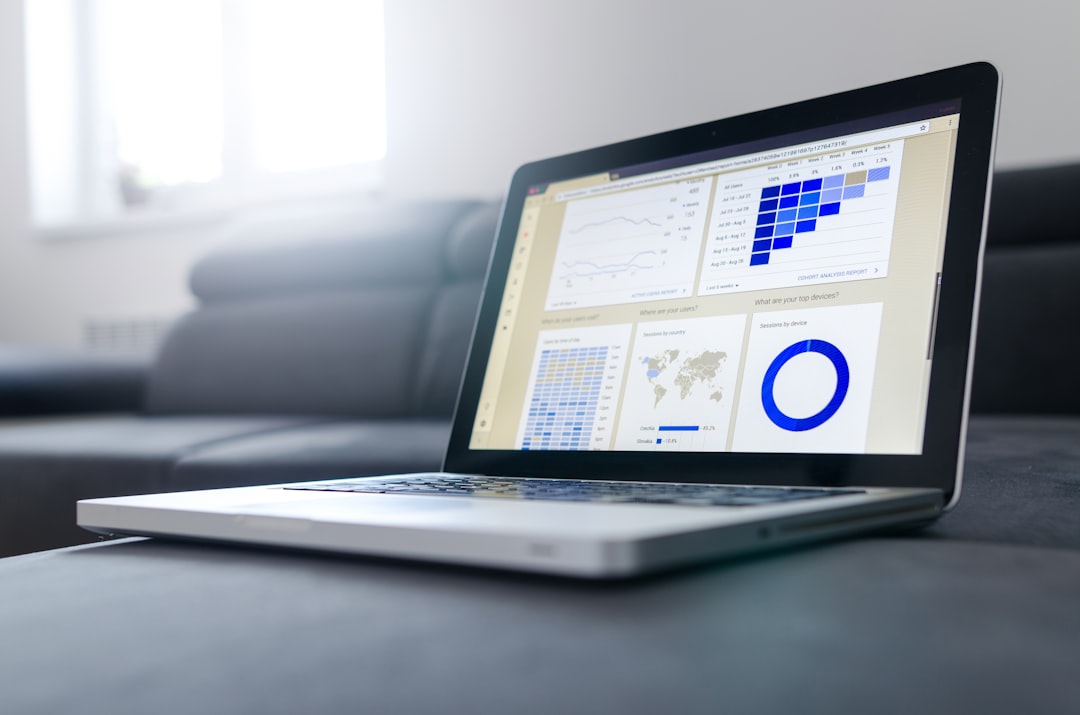
Successfully navigating the creative and technical evaluation tasks within the Data Annotation Starter Assessment requires a deep understanding of the assessment's criteria and a structured approach to evaluating information. You'll encounter various evaluation formats, such as prompts that require you to rate responses as good, acceptable, or flawed, and even to identify potentially inappropriate content. This evaluation process isn't merely about gauging the quality of annotations, but also about ensuring the responses adhere to provided guidelines. As the reliance on high-quality human-generated data annotation in AI applications continues to increase, possessing the technical skills to meet these rigorous evaluation standards becomes increasingly critical for anyone seeking a role in this field. Preparing for these tasks not only hones your abilities in evaluating data, but also contributes to the integrity of AI projects across various sectors that rely on accurate data annotation.
Preparing for these evaluation tasks involves understanding a few key aspects. First, the concept of cognitive load is crucial. Research suggests that designing tasks with an appropriate level of complexity can lead to better performance. Essentially, we want tasks that are stimulating enough to be engaging but not so difficult that they become overwhelming. This is a delicate balance but can be helpful in achieving more accurate and consistent results.
We've also noticed some recurring trends in how people tend to make mistakes during these evaluations. This highlights common hurdles in fully grasping annotation guidelines. If we can understand these recurring issues better, we can tailor training programs to address them more directly.
For data annotation to be truly useful, we need a high level of consistency between different individuals working on the same project. This inter-rater reliability depends on ensuring that everyone interprets guidelines in a similar fashion. Some minor adjustments to instructions or additional training can help reduce discrepancies significantly between annotators' outputs.
Implementing real-time feedback loops within assessments is proving to be quite beneficial. When annotators get immediate feedback on their performance, they tend to learn new skills much faster. This rapid response compared to those receiving feedback later suggests a powerful role for interactive learning within the assessment process.
However, we've seen that prolonged assessment tasks can lead to performance declines simply due to fatigue. This is a factor we must keep in mind as we design future evaluations. Breaking up tasks or including short breaks can help maintain annotators' focus and potentially improve the overall accuracy of their work.
Beyond immediate results, advanced statistical techniques are proving useful in predicting future performance from the initial assessments. This can guide skills development in a much more precise manner and contribute to more accurate project planning.
Using a diverse set of data types during assessment gives us a more comprehensive picture of an annotator's abilities. Individuals who can handle different formats and data types tend to adapt better when working on real-world annotation projects.
Blinding reviewers to factors like the annotators' background or identity helps combat bias and ensures the assessment environment is perceived as more fair. This objective approach leads to a more consistent evaluation process.
It's helpful to create different tiers within evaluation systems based on performance levels. This allows us to tailor training materials more precisely to specific needs. This approach not only helps us identify the best candidates but also supports continued learning throughout the journey of annotation.
Finally, it's essential to track individual growth over time. Regular assessments can provide insights into how skill sets evolve. This will allow us to refine training strategies to improve efficiency and effectiveness, potentially expanding these methodologies across larger teams.
Navigating the Starter Assessment Key Insights for Data Annotation Newcomers in 2024 - Mastering Image and Text Annotation Techniques
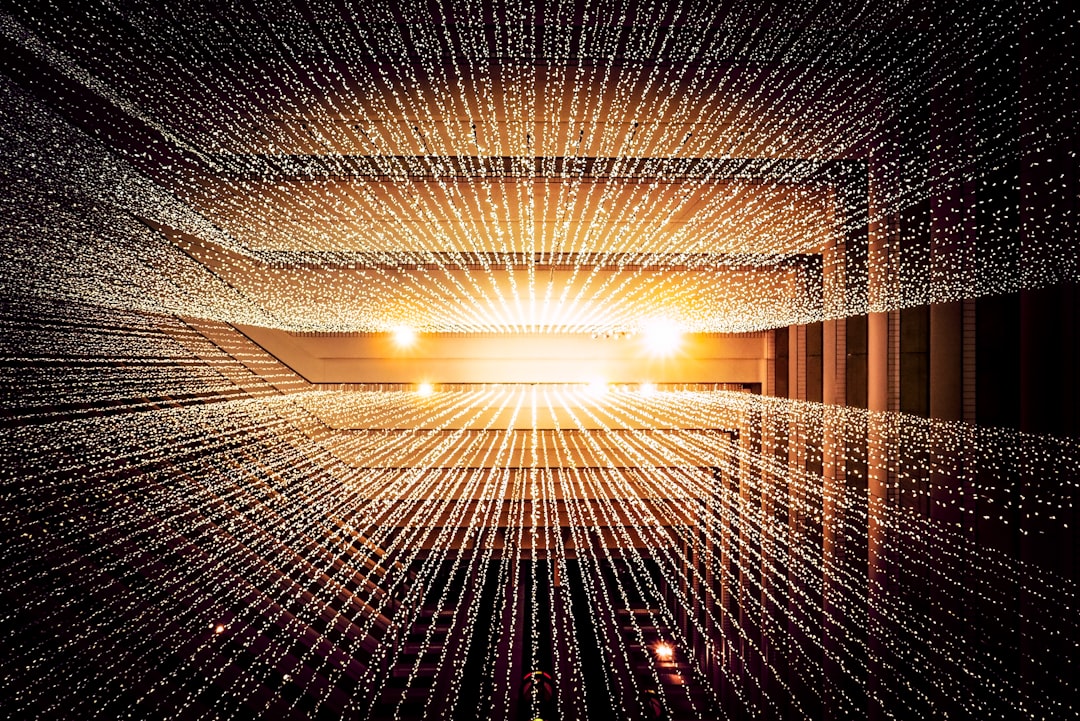
Within the evolving landscape of data annotation, mastering image and text annotation techniques is becoming increasingly vital, especially for those new to the field. Successfully annotating images involves utilizing a range of methods, like bounding boxes or segmentation, to pinpoint and label objects within the image. This skill is critical for the development of complex AI systems. Likewise, text annotation necessitates careful labeling of sentences and phrases, which is a key aspect of natural language processing applications. As the need for meticulously labelled datasets across diverse industries grows, newcomers must develop a strong understanding of industry best practices and quality control procedures. This will equip them to contribute effectively to AI development. Given the intricate nature of these tasks, understanding annotation methodologies and adhering to guidelines is essential to ensure the precision of machine learning models.
1. Image annotation isn't just about drawing boxes around objects. It also involves understanding the context and the relationships between those objects, which adds a significant layer of complexity, particularly when it comes to the mental effort required from the annotator.
2. Text annotation goes beyond identifying sentence structure. It needs to capture the subtleties of language, such as sentiment, sarcasm, and the broader context of the text. These aspects are essential for teaching advanced AI models to understand and respond to natural language more accurately.
3. Our brains are remarkably quick at recognizing patterns in images – some studies suggest we can do it in as little as 100 milliseconds. This raises interesting questions about whether manual annotation is the most efficient approach, particularly compared to how AI-driven annotation techniques might evolve in the future.
4. Cognitive load theory suggests that breaking down complex tasks into smaller, more manageable parts can help improve the accuracy of the work. This could mean that annotators may do a better job if they focus on a few key features within an image or text at a time instead of trying to process everything at once.
5. Vague instructions can be confusing for annotators, often leading to inconsistent results. It seems clear that the more specific and detailed the annotation guidelines are, the better. Research suggests that providing very precise guidelines can lead to a reduction of up to 30% in variability among the annotations.
6. Bias can creep into the annotation process in multiple ways, whether it's from the annotator's background or the specific dataset that's being used. To reduce this, it seems important to have a diverse group of individuals doing the annotation work.
7. It's surprising how much variation can occur between annotators when they're looking at the same data. Some studies have revealed discrepancies of up to 50%, highlighting the need for thorough training and clear guidelines to improve the reliability and consistency of annotation efforts.
8. Giving annotators real-time feedback as they work appears to be a highly effective learning strategy. Research has shown that immediate feedback can double the rate of performance improvement compared to more traditional approaches of delayed feedback.
9. A method called "think-aloud protocol" where annotators verbalize their thought processes as they work can shed light on the cognitive steps they're taking. This could help us understand where annotators might struggle or excel, leading to more effective training materials.
10. Recent research suggests that annotators who get exposed to a wider range of datasets tend to develop greater adaptability and skill retention. This potentially makes them better prepared to handle unforeseen challenges that might arise in future projects.
Navigating the Starter Assessment Key Insights for Data Annotation Newcomers in 2024 - Meeting the Rising Demand for Meticulously Labeled Datasets
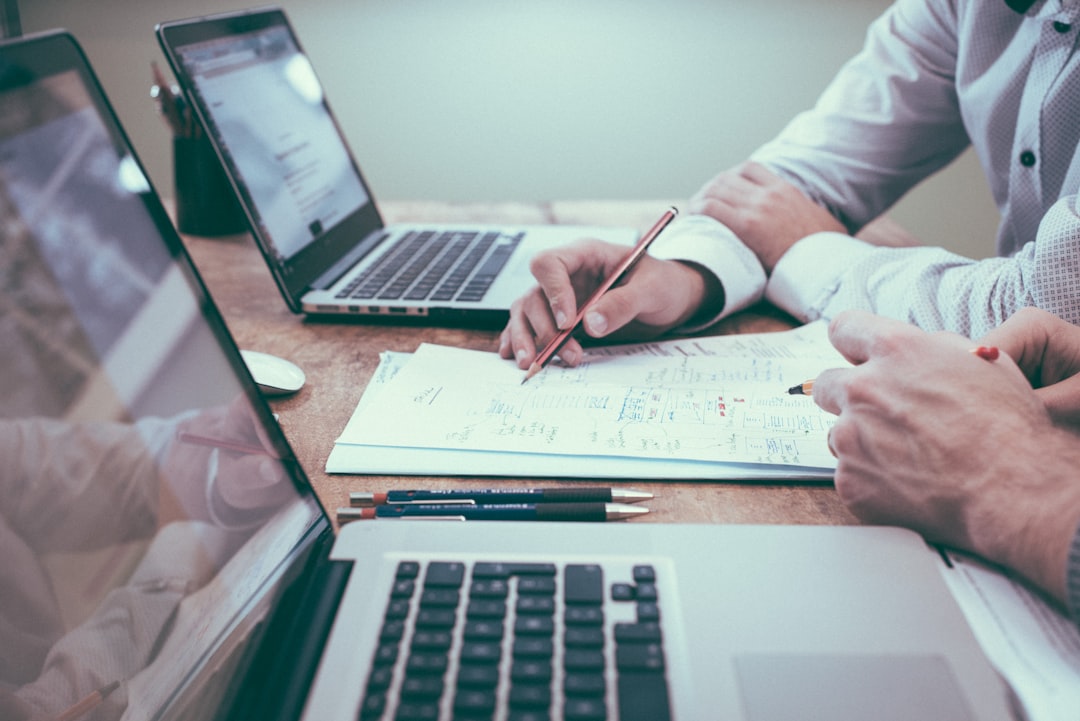
The surge in AI model complexity, particularly in fields like natural language understanding and computer vision, has fueled a dramatic increase in the need for meticulously labeled datasets. This isn't just a temporary trend; it signals a profound change in how organizations approach machine learning projects. Achieving accurate and reliable outcomes now hinges on the quality of annotated data. However, this increased demand reveals significant challenges, such as handling the transformation of unstructured data into structured formats for annotation. Additionally, concerns remain about consistency across annotators, the mental strain involved in the task, and the importance of having sufficient training and clear guidelines. Addressing these challenges and meeting the growing need for high-quality datasets will involve a combination of improving annotation workflows, leveraging automation where practical, and cultivating a diverse workforce of annotators. This multi-pronged approach is critical for safeguarding the quality and reliability of the data that underpins the development of powerful AI systems.
The increasing need for precisely labeled datasets is being fueled by the rise of crowd-sourced annotation, allowing for large-scale collaboration among annotators. However, this rapid expansion presents challenges in ensuring consistent quality and training across the workforce.
While automated annotation tools are becoming more prevalent, human annotators continue to play a critical role. Research indicates that human annotators often outperform automated systems in tasks requiring subtle pattern recognition and contextual understanding, especially in image-based applications.
Studies have revealed that prolonged annotation can induce cognitive fatigue, impacting accuracy. This highlights the importance of designing assessments that incorporate strategic breaks to maintain optimal performance and prevent decline in quality.
A primary source of annotation errors stems from unclear or ambiguous guidelines. Providing specific and detailed instructions can dramatically reduce inconsistencies, potentially leading to a 30% improvement in the consistency of annotations.
The principle of "inter-rater reliability," which seeks to ensure consistent results from multiple annotators, is facing challenges, with some studies finding discrepancies of up to 50% when different annotators work on the same dataset. This underscores the critical need for standardized training and consistent quality checks.
The presence of biases in datasets can lead to skewed results, as certain groups may interpret contexts differently. Incorporating a diverse group of annotators can help mitigate these biases and make annotation outputs more reflective of real-world scenarios.
Early stages of training often highlight a natural aptitude for certain tasks among annotators, such as image recognition, offering opportunities for tailored skill development and optimization.
Utilizing real-time feedback mechanisms during annotation has shown promising results, potentially doubling the pace of skill improvement. This dynamic feedback helps annotators refine their abilities more quickly during the assessment process.
Implementing "think-aloud" protocols—encouraging annotators to vocalize their decision-making process while working—provides valuable insights into their cognitive strategies. This allows us to refine training methods and address specific cognitive bottlenecks that hinder annotators.
The continuously evolving landscape of data annotation necessitates that professionals stay current with industry best practices and emerging techniques. As demands for specialized datasets increase, the field of data labeling will continue to evolve in response to new challenges, making it crucial for individuals in this field to remain adaptable and knowledgeable.
Navigating the Starter Assessment Key Insights for Data Annotation Newcomers in 2024 - Tackling the Challenge of Unstructured Data Transformation
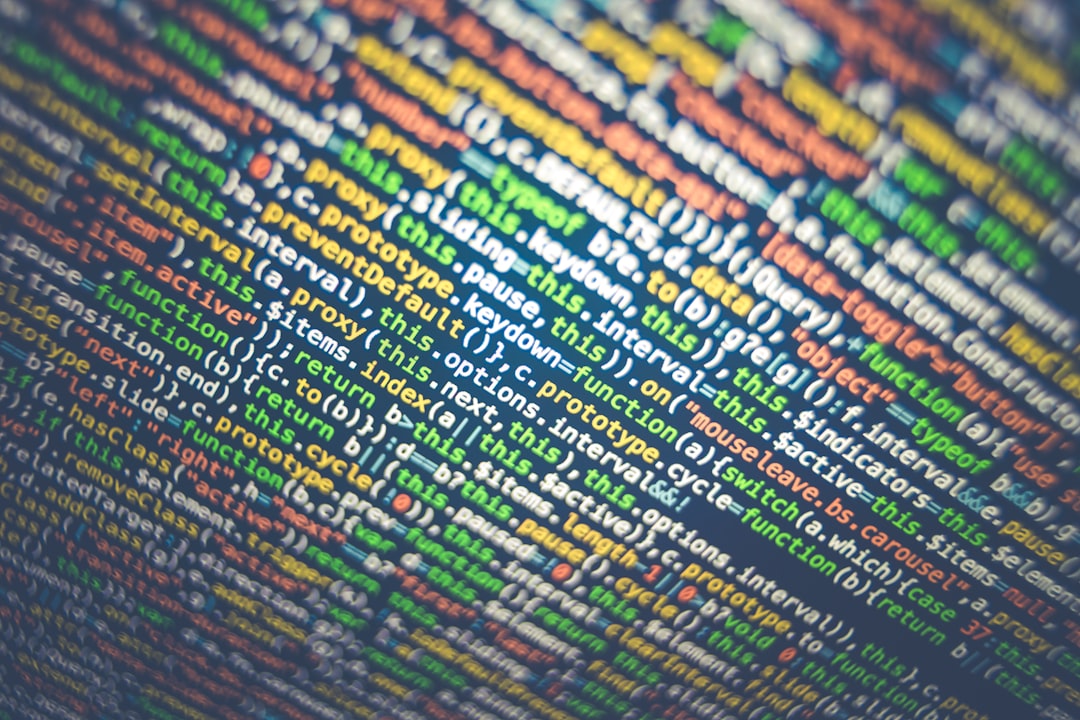
The increasing volume of unstructured data presents a significant challenge for organizations in 2024. A large majority of the data generated—potentially as much as 90%—falls into this category, encompassing a diverse range of formats including emails, images, and sensor readings. This type of data, unlike structured data that conforms to traditional database models, is difficult to manage and analyze. Specialized tools and methodologies are necessary to properly extract and utilize insights within unstructured data. Organizations are struggling to find effective ways to manage this, and many lack the expertise to transform it into a format usable by traditional data analysis methods. This necessitates a shift in how organizations approach data management, potentially incorporating more agile and innovative solutions. Focusing on the business questions that this data can help solve is crucial, and without a clear application, the data remains mostly dormant. Businesses must adopt innovative solutions to manage the growth of unstructured data and the complex challenges it presents. Failure to adapt can result in missed opportunities to glean critical insights from a vast pool of information. The future of data management is interwoven with the ability to leverage unstructured data, and the organizations that adapt their approach will likely have a significant advantage moving forward.
### Tackling the Challenge of Unstructured Data Transformation
The vast majority of data generated today, estimated at 80-90%, is unstructured. This includes a wide range of data formats, such as social media posts, sensor readings, and emails. Transforming this unstructured data into a format suitable for machine learning presents a substantial hurdle. It's not simply a matter of volume, but also a matter of complexity, making it challenging to derive insights efficiently.
Dealing with unstructured data places a heavier cognitive load on annotators compared to structured data. Studies indicate that unclear instructions or intricate tasks can lead to significant drops in performance, sometimes even by half. This underscores the need for meticulous and detailed guidance for those involved in the annotation process.
When we compare the effort needed for structured and unstructured data annotation, the difference can be stark. Researchers have noted that unstructured data annotation might take four to five times as long. This factor significantly influences project planning and resource allocation, as it demands more time, expertise, and possibly more manpower.
One interesting aspect of recent data annotation efforts has been the incorporation of adaptive algorithms. These algorithms allow for a dynamic annotation environment. As annotators input their labels, the system can fine-tune the guidelines in real-time, leading to a more refined process and a potential improvement in data quality. This feedback loop can help hone the annotation process and refine the datasets being created.
It's important to acknowledge that mistakes in the annotation of unstructured data can have a domino effect. Errors can ripple through the resulting machine learning models, decreasing accuracy potentially by up to 30%. This emphasizes the importance of implementing robust quality control measures at every stage of the annotation workflow.
The subjective nature of understanding and interpreting unstructured data leads to inconsistencies among annotators. Studies have indicated a wide variation in labels applied by different people to the same data, sometimes exceeding 70%. This variability raises questions about the objectivity of annotation tasks and how to standardize the processes.
Achieving high-quality annotation requires meticulous attention to quality control. However, this often adds a significant overhead cost, sometimes as high as half the total project budget. This emphasizes the necessity of prioritizing efficiency and planning the annotation workflow strategically to minimize these costs.
Automation is often touted as a solution for processing large datasets, but it frequently struggles with the subtle complexities of unstructured data. A significant proportion—potentially as high as 75%—still necessitates human intervention for proper annotation. This suggests that a purely automated solution isn't feasible at this stage and highlights the continued need for expert human input.
Interestingly, research indicates that more diverse annotation teams tend to produce better results. Diverse groups have been shown to create output that's up to 30% more accurate than teams composed of individuals with similar backgrounds. This is likely due to the increased perspectives and insights, mitigating potential biases present in individual interpretations.
Finally, it's essential to acknowledge the strain that extended sessions of unstructured data annotation can place on the human annotators. Fatigue and cognitive overload can significantly reduce accuracy, potentially leading to up to a 23% drop in the quality of outputs. Implementing strategies that promote focus and well-being, such as incorporating breaks or managing workload effectively, can help mitigate this negative effect.
Analyze any video with AI. Uncover insights, transcripts, and more in seconds. (Get started for free)
More Posts from whatsinmy.video: