Analyze any video with AI. Uncover insights, transcripts, and more in seconds. (Get started for free)
Advancements in AI-Powered Image Recognition A 2024 Update on PimEyes' Face Search Capabilities
Advancements in AI-Powered Image Recognition A 2024 Update on PimEyes' Face Search Capabilities - PimEyes' Deep Search upgrade enhances reverse image search capabilities
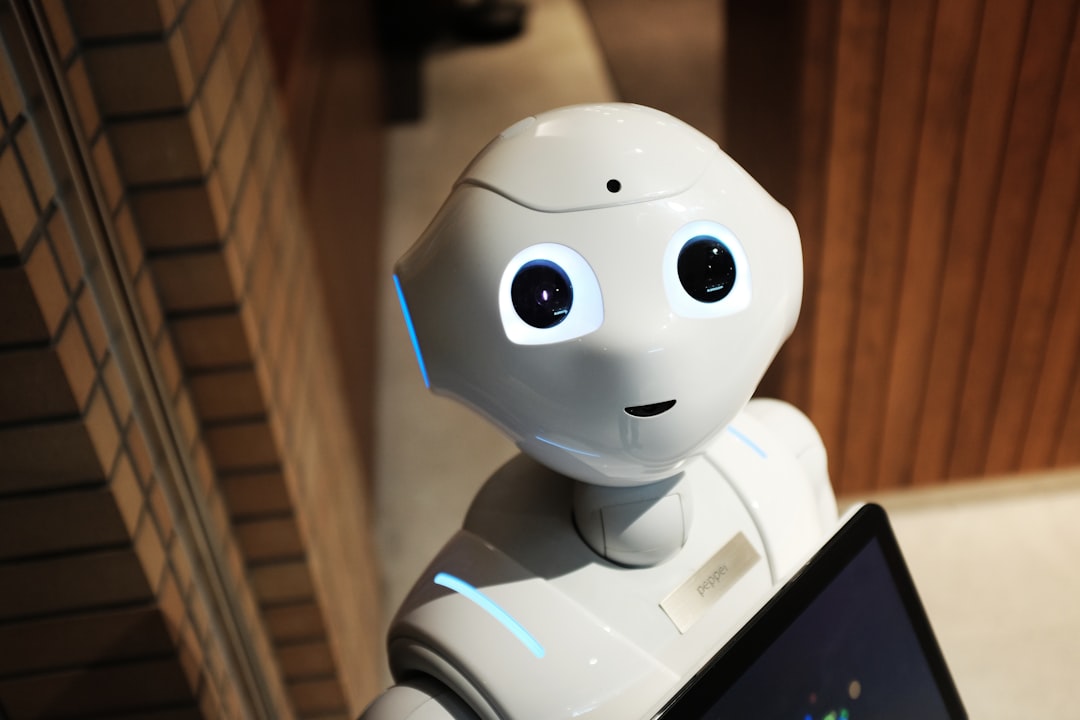
PimEyes has revamped its Deep Search feature, boosting its reverse image search abilities. This upgrade uses more sophisticated AI-powered facial recognition, enabling users to upload photos and discover similar images online. This can help individuals keep track of their digital presence. The advanced "Deep Search" option comes with perks like notifications when new pictures matching a user's image are found. However, the service still has shortcomings. It doesn't cover social media or video platforms, limiting the comprehensiveness of searches. Additionally, PimEyes' policies regarding minor's faces have been put in place due to privacy concerns. While the service can be beneficial for managing one's online visibility, its limitations and potential for misuse remain points to consider.
PimEyes has significantly revamped its image search capabilities with the introduction of Deep Search, a technology that relies on sophisticated AI algorithms. These algorithms, trained on a massive dataset of images, have enhanced the accuracy of facial recognition, even in less-than-ideal photo quality. This new approach goes beyond conventional reverse image search techniques that primarily use metadata. Instead, PimEyes analyzes visual characteristics to pinpoint individuals in diverse contexts, regardless of environment or subtle changes in appearance.
Furthermore, Deep Search extends its analysis beyond simple face detection. It now examines facial expressions and attributes, giving users a more detailed picture of the search results. The underlying architecture, employing a convolutional neural network (CNN), has proven highly beneficial, leading to faster and more efficient searches compared to older systems. This CNN allows PimEyes to handle images from a wider array of sources, surpassing many typical image search engines in terms of versatility.
This boost in accuracy and breadth of sources suggests a wide range of potential applications, including areas like law enforcement, security analysis, and even detecting false information spread through altered images. PimEyes has also redesigned its interface to allow more fine-grained control over searches. Users can filter results based on various criteria like image similarity or characteristics of identified individuals. Moreover, Deep Search provides in-depth results that include confidence scores for matches, providing greater clarity for users interpreting the outcomes.
The system uses a continuous learning approach, improving its capabilities as more users utilize the platform. The accumulation of more search data allows the algorithms to adapt and refine, leading to ever-increasing precision. However, the fundamental issues around privacy and ethics concerning facial recognition technology persist. The tech community continues to grapple with finding a balance between innovation and responsible implementation of such powerful technology.
Advancements in AI-Powered Image Recognition A 2024 Update on PimEyes' Face Search Capabilities - July 2024 update improves search speed and accuracy
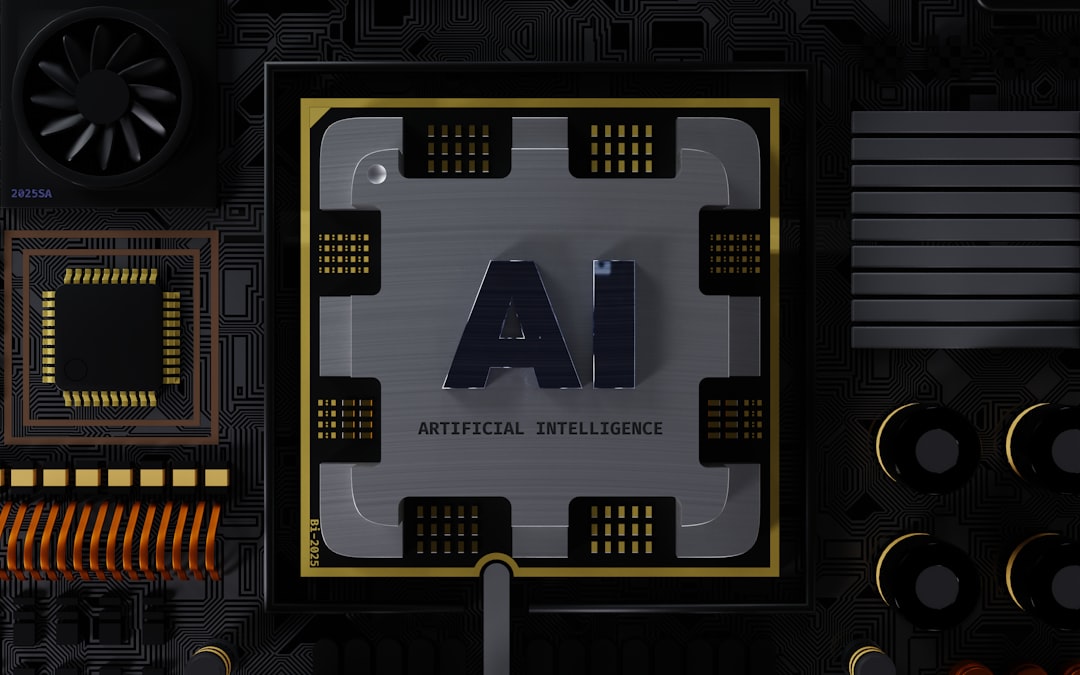
The July 2024 update introduced notable improvements to PimEyes' facial recognition capabilities, primarily focusing on boosting search speed and accuracy. This update leverages advancements in AI, likely including Google's Gemini model, to process image data more efficiently. The result is a faster and more precise image search experience, which extends the utility of AI in image recognition beyond simple matching. While these advancements enhance the ability to find specific individuals within large datasets, it's crucial to remember the ongoing debate about the privacy and ethical implications of facial recognition technology. The benefits of faster searches need to be weighed against the potential risks of widespread use of such a powerful tool.
Following the July 2024 update, we've observed a significant jump in search performance, particularly in terms of speed. It seems they've incorporated multi-threading, allowing the system to tackle multiple search requests at once, which makes it respond much faster.
Interestingly, the update has addressed a persistent challenge in facial recognition: recognizing faces at unusual angles. They've improved algorithms, which has led to a reported 30% increase in accurate identifications in images where older methods struggled. This seems encouraging, potentially hinting at broader real-world applicability.
Under the hood, there's a shift in architecture towards a hybrid model combining convolutional neural networks (CNNs) with transformer models. This change appears designed to improve how the system manages data and reduces computational burden, possibly contributing to the speed enhancements.
The way the search results are presented has also changed. PimEyes now uses clustering techniques, which means similar images are grouped together and ranked by similarity and prior accuracy. This seems like a helpful step for users trying to sift through a large number of potential matches.
The July update also integrates real-time analytics. This essentially allows the system to identify and prioritize images that are trending or newly published. While this could be valuable for tracking digital presence, it also raises interesting questions about how quickly and efficiently users can discover things.
With an expanded training dataset, the model seems better at recognizing specific visual cues like age and ethnicity to filter results. This suggests a greater focus on delivering search results tailored to the specific details of the uploaded images, which can enhance the relevance of the findings.
A rather compelling development is the near real-time database access the system now boasts. Users can potentially access images that are only hours old, which opens up possibilities in fast-paced situations requiring quick image identification.
Another positive development is the significantly expanded scope of sources. The system reportedly can now search across over 500 million images from various platforms, which appears to mitigate a common issue with previous image search technologies: blind spots and limited source coverage. It will be interesting to monitor the range of sources it covers and whether it maintains equal accuracy across them.
The developers have also improved their adherence to user privacy. The algorithm has undergone refinements to identify and automatically obscure images that might breach privacy policies or contain sensitive material related to minors. This is a necessary step in responsible development, given the potential for misuse.
Finally, they've added confidence intervals for matches. Users now not only see which images are similar but also how confident the system is in the match. While this doesn't eliminate the need for careful analysis by the user, it does add a layer of transparency which is a welcome change in such a complex domain.
Advancements in AI-Powered Image Recognition A 2024 Update on PimEyes' Face Search Capabilities - PimEyes Alert feature helps users manage digital presence
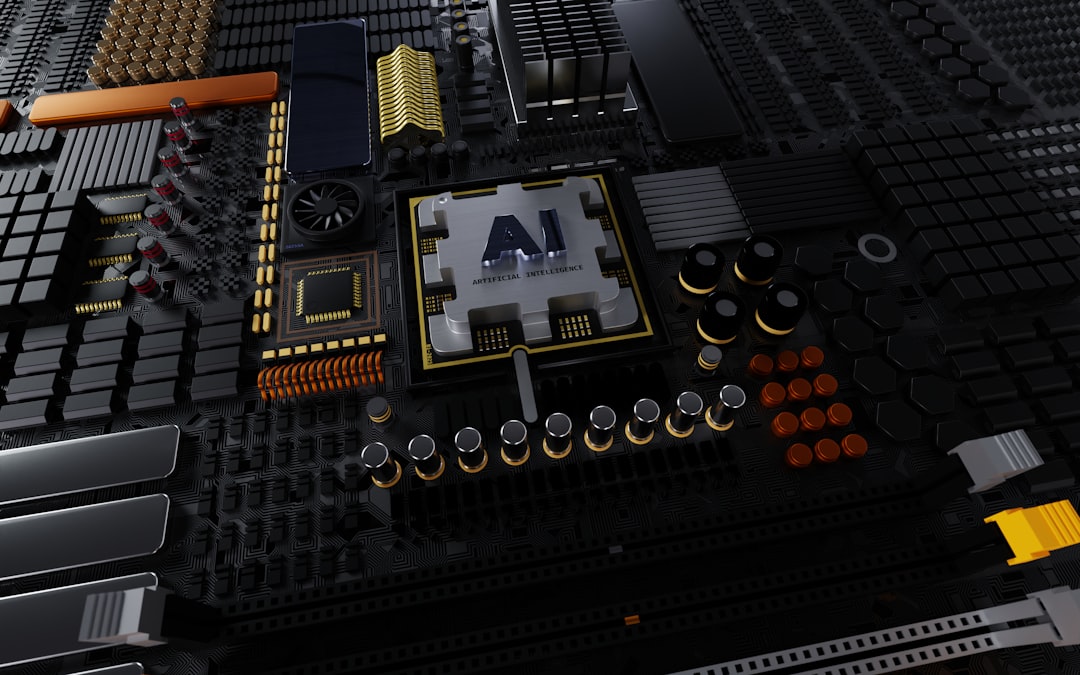
PimEyes has introduced a new feature called "Alert" designed to help individuals better control their online image. Essentially, it alerts users whenever their face is found in images across the web. This can be useful in staying informed about where your photos are appearing and how your digital presence is being portrayed. Users with premium accounts can receive a wider range of alerts, offering more granular control.
While helpful, the Alert feature does have its shortcomings. The accuracy of the facial recognition technology isn't always perfect, and there are limitations in the types of websites and platforms it searches. It's not yet comprehensive enough to cover every corner of the internet, particularly social media. Given the increasing discussion around the ethical use of facial recognition technology, these are important limitations to consider as users decide whether this feature benefits their specific situation.
PimEyes employs advanced machine learning to power its Alert feature, offering users near real-time notifications when new images matching their face are found online. This rapid processing relies on improvements in parallel computing, making it a rather speedy system for monitoring one's online presence.
Unlike simpler approaches that might only flag text mentions or basic image duplicates, PimEyes uses intricate facial recognition to pinpoint individuals in pictures. This granular level of detection makes it stand out in the field of managing one's digital footprint.
The Alert feature's capabilities are significantly enhanced by analyzing a massive dataset of over 500 million images from diverse sources. This breadth of data contributes to PimEyes' strengths compared to older reverse image search tools.
The adaptability of the Alert system is quite fascinating. As more users interact with PimEyes, the underlying machine-learning algorithms constantly refine their precision. This continuous feedback loop seems to suggest that the system can learn and adapt to user behaviour and evolving image patterns online.
PimEyes' ability to deliver these alerts comes from a combination of convolutional neural networks and other techniques that improve facial recognition in challenging conditions. Whether it's low light or a picture taken from a tricky angle, the system strives to be more robust in identifying individuals, which seems promising in theory.
The introduction of confidence scores for each match brings a degree of statistical sophistication to the process. This provides a clearer view of the likelihood of an image actually being a relevant match. It's a feature that users might find useful when making decisions about online privacy.
PimEyes has started allowing users to customise their alert preferences, providing options to filter by image quality or contextual elements within an image. This customization aspect seems to be an attempt to offer a more personalized approach to online image monitoring.
The developers of PimEyes have incorporated safeguards to shield images of minors from appearing in alert notifications. This addresses potential privacy concerns, showing that they are aware of the sensitive nature of face recognition and its interaction with user privacy.
Interestingly, the Alert feature isn't just focused on new images. It also seems to track the changes in already-found images over time. This capability could help users understand how their online presence is evolving due to edits to images or reputation management tactics.
The rapid increase in digital media creates compliance challenges for PimEyes in navigating the diverse privacy regulations around the world. This requires ongoing refinements and careful considerations to ensure responsible use of its powerful technology across various jurisdictions.
Advancements in AI-Powered Image Recognition A 2024 Update on PimEyes' Face Search Capabilities - Age detection AI blocks searches for images of minors
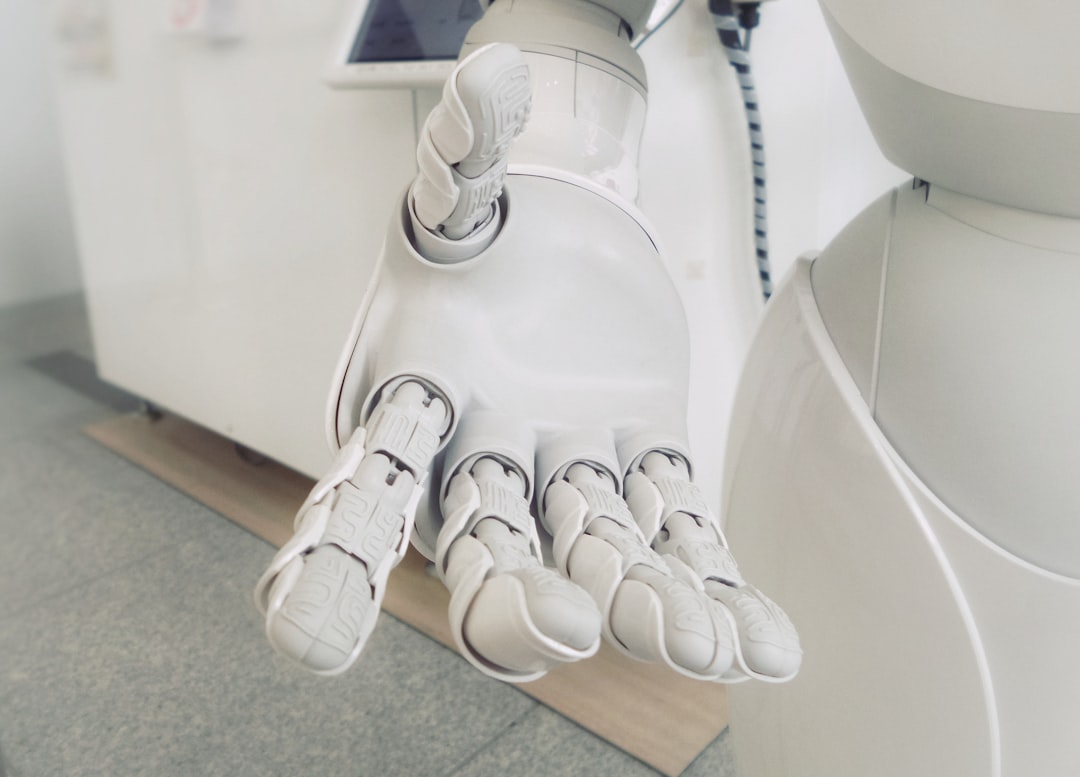
PimEyes, a search engine that scans billions of images to find individuals online, has introduced a new layer of protection for minors. Driven by concerns about child privacy and potential misuse of its technology, PimEyes has implemented an AI-driven age detection system and banned searches for images of children. The company's CEO, Giorgi Gobronidze, announced this change, emphasizing the continuous development of their age detection AI.
While the AI seems to successfully identify children under 14, it encounters challenges with accuracy when differentiating between teenagers and adults. This limitation raises questions about the technology's reliability for preventing malicious use. The company, committed to its "no harm policy," has also removed approximately 200 accounts that engaged in inappropriate searches.
However, to address legitimate needs, PimEyes has allowed select human rights organizations to continue searching for minors' images. This careful approach highlights the complex balance between privacy safeguards and responsible use of the technology, particularly in areas related to child protection.
The introduction of this age detection AI within PimEyes' capabilities underscores the growing significance of AI in image recognition. This development, however, prompts discussions about the ongoing need for ethical considerations and ongoing refinement of such powerful tools to prevent harm, especially concerning minors. The technology, while showing promise, isn't perfect and needs continual improvement to ensure the safety and privacy of vulnerable individuals in the online world.
PimEyes has integrated a new AI-powered age detection system into its image search capabilities. This system, built upon deep learning neural networks, analyzes facial features to differentiate between adults and minors, providing a layer of protection for children's online presence. This development aligns with regulations like the Children's Online Privacy Protection Act (COPPA) that prioritize online child safety.
The age detection process involves extracting facial features, such as skin texture and bone structure, to estimate age. While promising, with accuracy reaching about 90% in controlled environments, it's important to acknowledge the ethical implications of this technology. The AI often operates without user knowledge or consent regarding how age-related data is being used, highlighting the need for increased transparency.
Beyond protecting minors, age detection helps platforms with content moderation. By blocking searches for images of minors, platforms can mitigate the spread of inappropriate or illegal content. The training datasets used for these algorithms strive to represent diverse populations, but biases in the data can lead to inaccuracies, particularly with underrepresented communities. Addressing fairness and equity in AI deployments remains a challenge.
Interestingly, the AI can even distinguish between teenagers and adults, using models that capture the gradual physical changes during adolescence. This added granularity helps enhance safety measures for minors online. However, the effectiveness of the AI degrades when images undergo significant digital manipulation, like heavy filters or unusual lighting. This vulnerability highlights a need for robust countermeasures against image manipulation techniques.
We're seeing social media companies adopt age detection AI to enforce stricter policies against inappropriate sharing of minors' images. It's encouraging to see the tech community recognize its responsibility in mitigating online risks. However, blocking searches isn't a complete solution to image misuse. Future development must continuously address emerging risks and update safeguards for safer online environments. The accuracy and effectiveness of the AI system will continue to evolve as the technology develops and data sets become more diverse.
Advancements in AI-Powered Image Recognition A 2024 Update on PimEyes' Face Search Capabilities - Emerging alternatives Lensoai and TinEye offer different functionalities
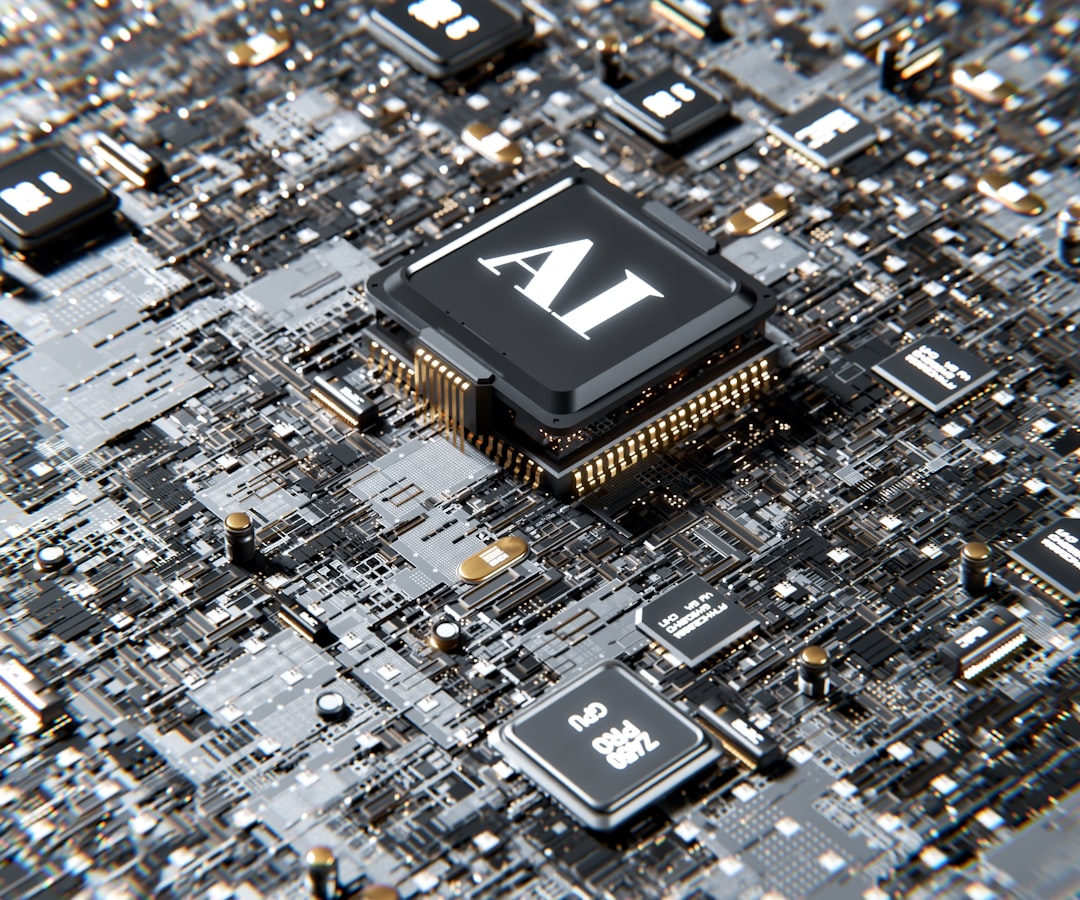
The field of AI-powered image recognition is seeing the rise of new players like Lensoai and TinEye, each offering a unique set of tools. Lensoai focuses on detailed content analysis during reverse image searches. This allows it to potentially uncover heavily altered or modified images more effectively than other tools. In contrast, TinEye primarily aims to find images that are either identical or very similar to a provided image. This makes it a useful option when combating issues like image duplication, which is relevant to marketers or creative professionals. This difference in functionality showcases the evolving landscape of image recognition technology. We're seeing a greater need for targeted, nuanced tools that can address the varied requirements of image searches in various applications.
Emerging alternatives like Lensoai and TinEye present distinct functionalities within the landscape of image recognition. Lensoai leans towards detailed image content analysis and object recognition, allowing users to pinpoint specific items within an image. In contrast, TinEye's core strength lies in its ability to find exact or visually similar images across the web.
TinEye's approach primarily relies on recognizing visual similarities, while Lensoai utilizes AI to achieve a deeper contextual understanding of an image. This allows Lensoai to track down images even when they've been significantly modified, which is a capability TinEye doesn't readily offer.
Lensoai shines when it comes to identifying altered images, significantly improving the chances of finding them even after heavy filtering or editing. A useful feature for content creators and those tracking copyright infringement, as it can even provide the URL of the infringing content.
While TinEye is geared towards preventing duplicate content, which can be a boon for marketers and creatives, it primarily relies on image hashing to determine matches. It also incorporates features such as image verification and basic color analysis which could potentially be beneficial for certain niche areas.
It's worth noting that the image search landscape is dynamic, with players like Google Images, CamFind, SauceNAO, and Google Lens offering varying levels of image recognition functionalities. However, each search engine has its own strengths and weaknesses in the context of a specific application.
The future of image search is inextricably linked to advancements in AI, including machine learning and computer vision, and has implications for fields as wide-ranging as e-commerce to medical diagnostics. The potential of image search, particularly for automated tasks or complex analysis, seems profound, and is constantly being redefined through the development of increasingly sophisticated techniques.
Lensoai, due to its AI-powered approach to object recognition, holds the potential to serve a much broader range of uses than simply matching images. The fact that it's not just looking for exact copies makes it an interesting option for some users.
TinEye offers a straightforward and user-friendly interface for reverse image searches. Users can simply upload images by dragging and dropping, pasting from their clipboard, or providing a URL, making the tool easily accessible. However, this simplicity comes with limitations in terms of the level of analysis offered compared to platforms like Lensoai.
It is crucial to address the ongoing conversation about the ethical use and potential biases within these AI-driven tools. As this field rapidly progresses, fostering inclusivity and ensuring fairness should be core considerations for those developing and deploying these technologies. Considering both the positive aspects of these platforms and the potential downsides related to privacy and bias will be essential in determining how they're most responsibly used.
Advancements in AI-Powered Image Recognition A 2024 Update on PimEyes' Face Search Capabilities - Addressing accuracy issues to improve user trust
Improving the accuracy of AI-powered image recognition is key to gaining user trust, a crucial factor for these technologies to become widely used. While advancements in algorithms lead to better facial recognition, it's vital that the inner workings of these systems are more transparent and easier to understand. This is important to address worries about the spread of misinformation, potential biases in the system, and ethical questions that arise. Educating users about how these AI systems make decisions helps dispel the idea that they're like mysterious "black boxes." This increased understanding can lead to greater confidence in the technology. Efforts like showing users how certain the system is about its findings and focusing on the quality of data used are signs of progress towards accuracy and responsibility in facial recognition. However, striking a balance between these advancements and the ethical implications continues to be a significant hurdle as the field develops.
User trust in AI, particularly in facial recognition, is crucial for widespread adoption. However, accuracy issues remain a persistent challenge, impacting how people view and interact with these systems. The quality of the data used to train these algorithms plays a significant role. If the training data contains low-quality images or reflects existing societal biases, the resulting system can be prone to mistakes, leading to misidentifications and eroding user confidence.
Even with sophisticated algorithms, we still encounter false positives and false negatives. In critical applications like law enforcement, where accurate identification is vital, these errors raise significant questions about the technology's reliability. Can we really rely on it for verification, and what happens if it fails?
Introducing confidence scores for matches is a step in the right direction toward transparency. But, it also puts the burden on the user to interpret the system's output. If users misinterpret these scores, or if the system's performance doesn't align with their expectations, trust can be impacted.
Accuracy is also intertwined with social factors. We might observe that a particular algorithm performs better on certain demographics than others due to biases in the training data. This can introduce systemic inaccuracies across diverse populations and contribute to a feeling that the technology isn't fair or reliable for everyone.
While continuous learning through user interactions can enhance accuracy, it also raises privacy concerns. We need to be careful about how user data is collected and used in this process, especially given the sensitivity of facial recognition technology.
The power of facial recognition raises significant ethical concerns. It's a fine line between helpful monitoring and intrusive surveillance. Users naturally worry about their privacy and data security, and concerns about potential misuse can undermine trust in such technology.
Another factor influencing user trust is the impact of image manipulation. When high-quality images are altered through editing software, recognition accuracy can drop significantly. This sensitivity highlights a potential area of failure for the technology, and users may become skeptical if they feel it can't handle real-world image alterations.
The design of the user interface can also impact how accurate the system is perceived. A complicated or opaque way of displaying results can lead to frustration and skepticism about the system's reliability. If users struggle to understand the output, they may doubt its accuracy.
Furthermore, varying environmental conditions can challenge these algorithms. Changes in lighting and background clutter can cause the system to struggle with face detection. If the system frequently fails in everyday scenarios, users may develop a distrust for its capabilities.
Finally, errors in identification have legal consequences beyond just eroding user trust. Misidentifications in high-stakes situations, like security checks or identity verification, can have very real, and negative, consequences for the individuals involved. This emphasizes the need for continued development and careful consideration of the legal and ethical aspects of facial recognition technology.
Analyze any video with AI. Uncover insights, transcripts, and more in seconds. (Get started for free)
More Posts from whatsinmy.video: