Analyze any video with AI. Uncover insights, transcripts, and more in seconds. (Get started for free)
Advances in Video Analysis AI Ethical Concerns and Real-World Limitations
Advances in Video Analysis AI Ethical Concerns and Real-World Limitations - Video Analysis AI Capabilities in 2024
The landscape of video analysis AI has shifted considerably in 2024, with notable improvements in the ability of these systems to understand visual information. New models, exemplified by Qwen2VL, demonstrate advanced comprehension of visual content within videos, allowing for more sophisticated analysis. Deep learning, a cornerstone of this progress, has honed the capacity of AI to identify trends and patterns within video data.
However, these advancements are not without their ethical challenges. The potential for misuse, particularly in creating convincingly realistic deepfakes, raises concerns about the impact on truth and trust. Further complicating matters is the increasing accessibility of these tools for users without a technical background, underscoring the necessity for clear guidelines on their deployment. The issue of privacy and the potential for spreading misinformation also loom large.
As we move forward, striking a balance between the drive for innovation and the need for responsible use is crucial. The potential benefits of video analysis AI are substantial, but without careful attention to the ethical and societal implications, the risks of misuse could overshadow the positive contributions of this technology. Addressing these complexities through the development of effective regulatory frameworks is essential to ensure a future where these technologies serve society in a positive and trustworthy manner.
The landscape of video analysis AI in 2024 is evolving rapidly, pushing the boundaries of what's possible. We're seeing systems capable of processing extremely high frame rates, allowing for the detection of subtle, rapid movements that were previously difficult to capture. This has huge potential, especially in areas like sports analytics, where fine details of athlete performance are critical, and security monitoring where quick responses are needed.
Beyond simply identifying objects, these systems are developing more sophisticated pattern recognition, enabling the classification of behaviors. This means algorithms are becoming better at distinguishing between different human actions, like friendly gestures versus aggressive movements. The implications for safety and security in public spaces are potentially quite significant.
Another interesting development is the increased use of multimodal data sources, allowing for a richer context in analysis. By incorporating audio along with the visual data, AI systems can gain a more nuanced understanding of situations, providing more comprehensive insights. This could lead to more accurate analyses and a better ability to understand the intentions behind actions.
Furthermore, adaptability is becoming more important. We're seeing video analysis AI systems that can tailor their algorithms based on specific user patterns or environments over time. This adaptability can greatly enhance the efficacy of long-term surveillance applications and behavioural studies, though it also raises questions about bias and fairness.
The shift towards edge computing is playing a crucial role in accelerating the video analysis pipeline. Edge processing reduces latency significantly, leading to faster response times in critical applications like autonomous vehicle navigation and security systems that require immediate action.
We are also seeing the emergence of AI models that can attempt to deduce emotional responses through facial recognition. While the accuracy and ethical implications of this capability are being debated, it shows the increasing sophistication of video analysis AI and offers potentially valuable insights in areas such as mental health assessments and improving customer service.
Efforts to reduce bias in these systems are ongoing. Researchers are working with more diverse datasets to improve the training of AI models and make sure they aren't perpetuating existing social biases in surveillance and law enforcement applications.
Interestingly, the quality of output from AI video analysis is improving through the use of generative techniques. Even from lower-quality source videos, AI can generate higher-resolution outputs, making the details and insights gained clearer.
Privacy and security are becoming increasingly important in the development of video analysis AI. Methods like differential privacy are being explored to maintain the efficacy of monitoring while also protecting individual identities and sensitive information.
Finally, there's a growing emphasis on transparency. Some systems are incorporating explainable AI features, making it easier for users to understand the rationale behind AI's decisions. This can improve trust in the system and help mitigate ethical concerns related to bias and transparency, ultimately leading to a more responsible use of this technology.
Advances in Video Analysis AI Ethical Concerns and Real-World Limitations - Privacy Concerns with Facial Recognition Technology
Facial recognition technology (FRT) has become a prominent tool in areas like security and public administration, leading to a surge in privacy concerns. Its growing use, especially within law enforcement, creates a complex ethical dilemma: balancing individual privacy against community safety. There's substantial worry regarding the nature of data gathered by these systems, along with the possibility of built-in biases in their analysis. These worries are amplified by the implications of widespread surveillance. Furthermore, FRT presents dangers like the loss of anonymity and data breaches, which can lead to severe privacy violations. The capacity of FRT to identify individuals in images also raises the specter of dystopian surveillance scenarios, potentially undermining civil liberties. Adding to the complexity, sophisticated algorithms underpinning FRT advancements both improve capabilities and complicate the privacy discussion. Given the rapid evolution of FRT, existing laws and regulations may not be sufficient to manage its widespread deployment. The need for thoughtful ethical discussions and possible interventions has become increasingly pressing.
Facial recognition technology (FRT) has seen incredible advancements, becoming a key component in areas like security and governance. However, these capabilities come with significant privacy concerns that warrant careful consideration. The sheer scale of some facial recognition databases, containing billions of images, is a source of worry. The speed at which retrieval and matching algorithms are improving only compounds this concern, raising the specter of unprecedented surveillance capabilities.
Research suggests that facial recognition systems can exhibit biases, particularly impacting women and individuals with darker skin tones. This is evident in higher error rates for these groups when compared to others. The potential for misuse in law enforcement or surveillance applications presents a clear ethical dilemma.
Furthermore, the legal landscape surrounding FRT is fragmented. Many jurisdictions lack comprehensive legislation to address the implications of this technology. This gap in regulation leaves citizens vulnerable to privacy violations with limited options for redress.
There's a growing unease about how FRT is being implemented in various contexts. Instances of its use for identifying protestors at rallies or monitoring marginalized communities raise uncomfortable questions about consent and the ethical implications of constant surveillance.
Adding another layer of complexity is the development of FRT systems that claim to detect emotions. While potentially valuable in fields like mental health or customer service, the accuracy and ethics of such technology are highly debated. The idea of mechanically interpreting human emotion raises questions about its validity and potential for misuse.
Many FRT systems operate with a "store and forget" approach. This practice involves indefinite data retention without explicit user consent. The continued storage of facial images, particularly within public and private spaces, raises serious risks for ongoing surveillance practices.
Another significant challenge is a lack of transparency regarding the algorithms that power FRT. Many developers do not publicly disclose how their systems function. This opacity creates distrust, fueling concerns about decision-making processes that are not subject to scrutiny or public understanding.
A major portion of the population isn't aware that FRT is being implemented in their daily routines. This lack of public awareness highlights the need for informed consent around the use of this pervasive technology and its implications. The ethical considerations surrounding the use of powerful surveillance systems demand a more transparent approach to public awareness.
Globally, there are significant discrepancies in the ways countries regulate and deploy FRT. This divergence leads to ethical dilemmas concerning international collaboration in law enforcement and the establishment of universally recognized privacy standards.
Despite advancements, FRT is not without its limitations. These systems are still affected by factors like lighting conditions, viewing angles, and occlusions, such as masks or hats. These challenges can result in a decrease in accuracy and reliability, making it difficult to ascertain how effective the technology is under real-world conditions. The gap between the promise and the actual application of FRT is an important area for continued study and development.
Advances in Video Analysis AI Ethical Concerns and Real-World Limitations - Algorithmic Bias in Video Content Analysis
The increasing sophistication of AI in video content analysis brings with it the concern of algorithmic bias. These biases, often stemming from limited and unrepresentative training data, can lead to skewed outcomes in how video content is analyzed and presented. This can manifest as the system favoring certain types of content or viewpoints over others, potentially reinforcing existing societal biases and limiting the diversity of information users encounter.
For example, AI-powered recommendation systems could inadvertently promote certain perspectives, effectively narrowing the range of information available to viewers over time. This can have significant consequences, particularly for marginalized groups who may already be underrepresented in existing data sets, thus further amplifying inequalities.
The ethical dimensions of algorithmic bias cannot be overlooked. Developers and researchers have a responsibility to mitigate these biases, ensuring algorithms are designed with fairness and inclusivity in mind. This includes using more representative data sets in the training process and regularly evaluating the impact of algorithms to ensure they are not producing discriminatory or harmful outcomes. Addressing these issues is crucial to ensure the responsible and equitable development of video analysis AI in the future.
The increasing reliance on artificial intelligence (AI) and machine learning (ML) in video content analysis has brought to light concerns about algorithmic bias, which can exacerbate existing societal inequalities and discrimination. A primary source of these biases often lies in the datasets used to train these algorithms. If the training data predominantly features specific demographics or behavioral patterns, the resulting algorithms may struggle to generalize accurately across diverse populations and contexts. This can lead to skewed outcomes and inadvertently reinforce harmful stereotypes.
For instance, facial recognition systems integrated into video analysis have shown higher error rates for marginalized groups, like women and people with darker skin tones. This can result in misidentification or disproportionate policing, magnifying social inequities. Algorithmic bias is not confined to individual identification, though. It can also influence broader behavioral assessments within video analysis. Systems designed to detect aggression or dissent might misinterpret cultural expressions or contextual nuances, leading to wrongful accusations or unfair profiling in public spaces.
Furthermore, the training processes for these AI systems often lack sufficient diversity. Many models overemphasize specific types of activity, like sports or scenes from urban environments, while overlooking less common or unconventional scenarios. This can hinder the accuracy and reliability of these systems in a wider range of real-world situations. Research has highlighted a phenomenon termed "contextual bias" in video content analysis algorithms. The circumstances under which a video is recorded – including lighting, environmental conditions, and location – can significantly impact an algorithm's interpretation, potentially leading to inconsistent results across seemingly similar scenarios.
The incorporation of feedback loops in machine learning for video analysis presents another potential pitfall. As these systems learn from their own output, errors and biases can become entrenched unless diligently reviewed and corrected through human oversight. This reinforces the need for robust quality control measures. The privacy implications of video analysis vary depending on the specific application. Practices that are viewed as acceptable in some cultures, like security cameras in public spaces, might be seen as intrusive in others. Consequently, there's a growing need for culturally sensitive approaches to algorithm development and implementation.
In certain public safety situations, misinterpretations by video analysis algorithms can lead to unnecessary intervention. For example, algorithms might mistakenly flag friendly interactions between people as potentially aggressive, resulting in unwarranted police involvement. Research suggests that many algorithms exhibit "confirmation bias," wherein they tend to favor information that supports pre-existing beliefs or assumptions, rather than providing objective analyses of video content. This inherent tendency to validate initial inputs can further contribute to biased outcomes.
Addressing algorithmic bias in video analysis is a complex challenge that necessitates a collaborative approach across disciplines. Bringing together experts from fields like sociology, ethics, and law can significantly enhance the design and deployment of these systems, ensuring they are not only technically proficient but also socially responsible and equitable. The increasing prevalence of AI in video content analysis underscores the critical importance of mitigating potential biases to ensure the ethical and equitable use of these powerful technologies.
Advances in Video Analysis AI Ethical Concerns and Real-World Limitations - Limitations of AI in Understanding Context and Nuance
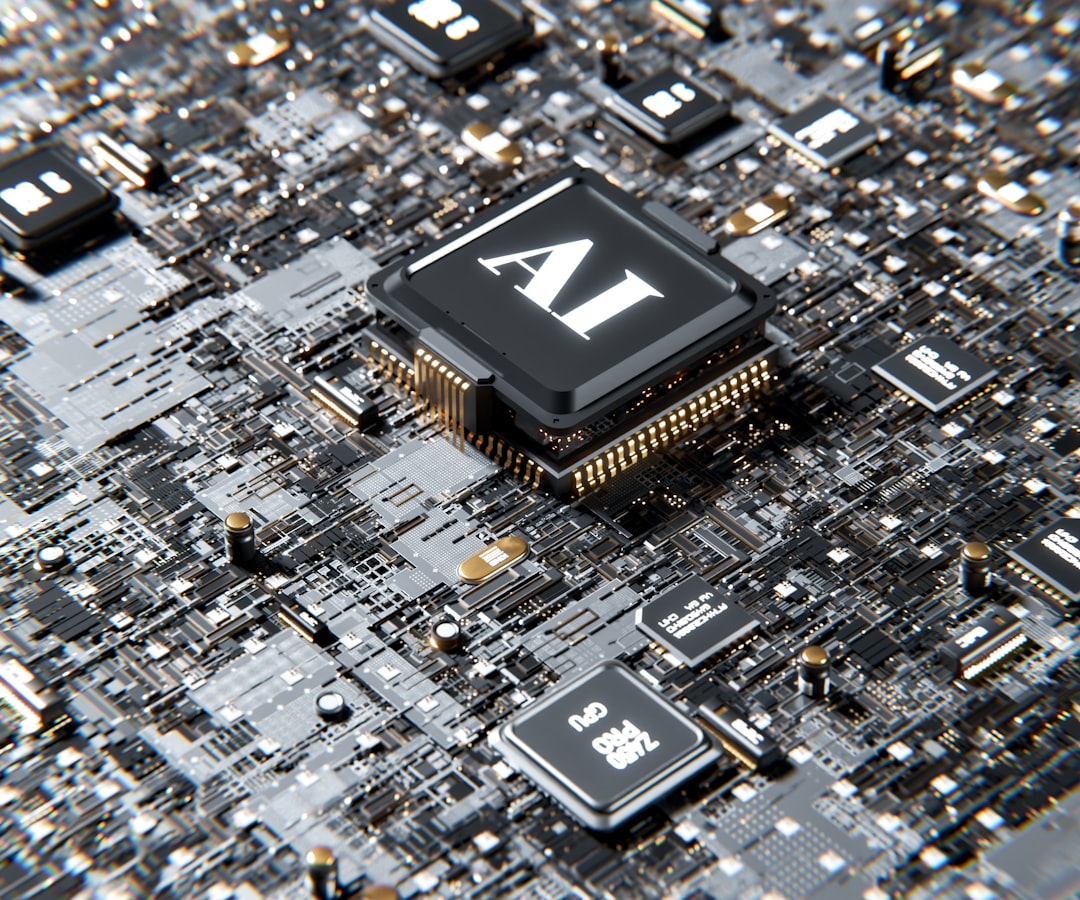
Despite the significant strides made in video analysis AI, a crucial limitation persists: its inability to fully grasp context and nuance. AI, in contrast to human intelligence, lacks the deep-seated conceptual and emotional understanding that allows us to interpret subtle language, cultural cues, and the complexities of human experience. This deficiency becomes particularly concerning when AI is tasked with ethical decision-making. AI systems are not equipped with the inherent human traits of intentionality or empathy that are fundamental to making thoughtful judgments. The difficulty AI faces in accurately deciphering the multifaceted aspects of human behavior raises serious questions about relying on it for critical tasks in areas like law enforcement or medical care. As the development of these AI systems progresses, it becomes increasingly urgent to acknowledge and address their inherent limitations to avoid unintended repercussions that might worsen existing biases or ethical dilemmas within society.
AI's capacity to understand context and nuance in video analysis, while improving, still faces significant hurdles. A core limitation is AI's reliance on pattern recognition rather than a deep, conceptual understanding of language and situations. This makes it prone to misinterpretations when dealing with subtle linguistic cues like sarcasm or irony, which rely heavily on tone and context that AI struggles to grasp.
Furthermore, AI models are often trained on specific datasets that may not fully capture the diversity of human experience. This can limit their ability to understand varied cultural contexts or non-verbal cues like body language, hindering their efficacy in diverse environments. Even when attempting to analyze emotions through facial expressions or tone of voice, AI lacks the emotional intelligence of humans, resulting in oversimplified and potentially misleading assessments.
The dynamic nature of context poses another challenge. AI algorithms may struggle to adapt to evolving situations, social norms, or behaviors over time. This “contextual drift” can lead to a system that is trained on a specific set of video scenarios but fails to analyze content that falls outside of that learned framework.
The "black box" nature of many AI models further compounds this problem. The lack of transparency in their decision-making processes makes it difficult to understand how they arrive at certain conclusions. Without clear insight into the internal logic, it's challenging to evaluate the reliability and fairness of the AI's outputs, especially in crucial applications like law enforcement.
AI's ability to handle conflicting information within video content also remains limited. Situations with multiple narratives or perspectives can confuse AI systems, leading to incorrect interpretations that could potentially worsen misunderstandings in critical contexts. Additionally, the length of the video can impact analysis. Excessively long or short clips may prevent AI from capturing important details that provide context, hindering the accuracy of its assessment.
Even with progress in recognizing objects and actions, a major gap remains in AI's comprehension of non-standard communication like gestures and body language. These nonverbal cues can carry rich meaning that is often overlooked by current AI models, resulting in a potentially incomplete understanding of the video's message.
Lastly, many existing AI models lack feedback mechanisms for learning from previous mistakes. Without the ability to adjust their contextual understanding based on errors, AI systems are more likely to repeat those mistakes in future analyses. This ongoing issue necessitates continuous improvement and research to bridge this crucial gap in AI’s capabilities.
Advances in Video Analysis AI Ethical Concerns and Real-World Limitations - Ethical Considerations for AI-Powered Surveillance Systems
AI-powered surveillance systems offer the potential to enhance security and efficiency across various sectors, yet their use brings about a complex set of ethical challenges. These systems can infringe on individual privacy in profound ways, raising concerns about misuse and the potential erosion of fundamental rights and freedoms. The use of AI in surveillance during events like the COVID-19 pandemic, while demonstrating the technology's potential for good, also showcased the ethical pitfalls, particularly regarding potential biases and discriminatory outcomes. Striking a balance between safeguarding public safety and upholding individual liberties remains a central challenge as these technologies advance. Transparency, accountability, and a commitment to responsible deployment are vital as we consider the broader societal impact of AI surveillance. The ongoing conversation emphasizes the critical need for careful consideration of how these systems are woven into the fabric of our lives, with a particular focus on establishing governance structures that protect individual rights in the face of ever-expanding technological abilities.
1. The lack of transparency in how AI-powered surveillance systems operate is a major ethical issue. These systems often act like "black boxes," making it hard for individuals and the public to understand how decisions are reached. This lack of clarity can easily lead to distrust in the technology and its various applications.
2. Many AI surveillance systems have a practice of storing data indefinitely without telling or getting consent from the people being recorded. This "store and forget" approach poses serious ethical questions about ongoing monitoring and the potential erosion of our privacy.
3. Being constantly monitored by AI can have a significant impact on individuals' psychology, causing increased anxiety and potentially affecting their behavior even when they've done nothing wrong. This constant awareness of surveillance can create a chilling effect on people's willingness to express themselves and act freely.
4. In urgent situations, like emergency responses, AI systems that don't fully grasp context can lead to mistakes. For instance, if an algorithm incorrectly identifies a friendly interaction as a conflict, it could result in unnecessary police intervention.
5. AI surveillance systems frequently rely on large amounts of data to make assessments. However, this aggregation of data can mask the specific circumstances of individuals. This can result in biased decision-making processes that don't take into account the unique context of a situation or a person, ultimately potentially harming those who are already disadvantaged.
6. The implementation of AI-powered surveillance disproportionately affects marginalized groups who may not be represented in the initial design and training stages of these systems. This can lead to policies that exacerbate existing societal inequities instead of trying to address them.
7. Surveillance systems that use machine learning can create a feedback loop where biased data leads to further biased conclusions. If a surveillance camera incorrectly identifies behavior due to biased training data, it can make the same errors in the future, potentially compounding unfairness over time.
8. The powerful surveillance capabilities of advanced AI present a risk that governments could overstep their authority. These systems could potentially be exploited to monitor dissent or intimidate opposition, which raises important ethical questions about the boundaries of government intervention in personal freedoms.
9. AI surveillance systems that are trained primarily on data from specific cultural contexts may not be able to accurately interpret behavior across different cultures. Misunderstandings in cultural expressions can easily lead to misinterpretations of behavior, which is especially concerning in diverse communities.
10. The rapid advancements in surveillance technology are often faster than the development of ethical guidelines and regulations. This creates a gap where the ethical considerations in the deployment and management of these systems may not be sufficient to adequately protect the rights and freedoms of individuals.
Advances in Video Analysis AI Ethical Concerns and Real-World Limitations - Real-World Challenges in Implementing Video Analysis AI
The integration of video analysis AI into real-world settings faces a number of hurdles that can hinder its efficacy. One major challenge is the phenomenon of "data drift," where changes in the nature of the data used to train the AI models can lead to a decline in their accuracy over time. This is a continuous issue that developers need to be mindful of. Furthermore, ensuring fairness and mitigating algorithmic bias remains a critical concern. The training datasets used to develop these systems often reflect and perpetuate existing societal inequalities, potentially resulting in discriminatory outcomes, particularly impacting marginalized communities. Adding to the difficulty is the inherent complexity of the real world, as AI systems frequently struggle to comprehend the nuanced and multifaceted nature of human behavior, including cultural customs and subtle social cues. Overcoming these limitations and ensuring responsible deployment is crucial for the future of video analysis AI. This calls for ongoing scrutiny of data quality, transparency in how AI algorithms function, and establishing robust ethical frameworks for their use.
The development and implementation of video analysis AI, while showing promise, faces a number of challenges related to the data used to train the systems. Limited and diverse training data often results in systems that struggle to accurately interpret real-world scenarios, potentially missing key details and misjudging complex human behaviors. This can be problematic when subtle cues need to be recognized, such as distinguishing between attempts at conflict resolution versus casual social interactions. The misinterpretation of such events by the AI could have negative consequences.
The integration of various data types like audio and video, while promising, is proving difficult to manage. Getting these AI systems to appropriately correlate information across different data formats remains a significant obstacle, leading to inconsistencies in outputs. Furthermore, the need to process information in real time, especially in scenarios involving high frame rates like sporting events, places immense strain on current video processing capabilities. Systems often compromise accuracy to handle the demanding processing load.
Another concern is the risk of overfitting during the machine learning training process, especially in the complex world of video analysis. The AI models can become overly specialized on the training data, leading to poor adaptation to novel situations. This lack of flexibility significantly weakens the effectiveness of these systems in diverse environments. Additionally, AI lacks a true grasp of social cues and historical context, leading to challenges with context-aware analysis. This limitation causes the AI to misinterpret events that may include culturally specific behaviors. This is especially problematic in law enforcement, where an accurate interpretation of events is crucial.
We also need to consider the impact of feedback loops within the algorithms. These can perpetuate existing biases. If an AI makes errors in recognizing behaviors, it is possible for those mistakes to repeat and amplify in subsequent analyses, potentially exacerbating issues of fairness and overall system reliability.
While improvements to AI continue, it is vital to be aware that this does not mean ethical considerations vanish. The pace of advancements in video analysis AI frequently outpaces the development of regulatory frameworks. The consequence is that existing laws are not equipped to manage the emerging applications of the systems, leaving a significant gap in legal and ethical guidelines.
Finally, a significant hurdle facing video analysis AI is that it doesn’t have the same level of judgment as humans. This lack of critical thinking presents ethical challenges when considering using AI to make important decisions. The systems simply cannot grasp the complex tapestry of nuance and context the way humans do. This ultimately raises concerns about relying on AI for tasks requiring human-like insight and a profound understanding of social situations.
Analyze any video with AI. Uncover insights, transcripts, and more in seconds. (Get started for free)
More Posts from whatsinmy.video: