Analyze any video with AI. Uncover insights, transcripts, and more in seconds. (Get started for free)
Gcore's SummarizationVideo Cutting to the Chase in Video Content Analysis
Gcore's SummarizationVideo Cutting to the Chase in Video Content Analysis - Gcore's AI-driven transcript generation streamlines video analysis
Gcore's AI-powered transcription system simplifies the task of video analysis by automatically transforming video into text. This streamlined process not only provides transcripts but also leverages artificial intelligence to generate summaries and pinpoint key takeaways. This three-stage process, encompassing transcription, summarization, and further refinement, addresses a growing need for efficient video analysis tools in a rapidly expanding industry. While this approach shows promise, handling extended video content and accurately conveying subtle details can still pose challenges. The capability to analyze sentiment and automate tagging further highlights the potential of AI to deliver richer insights and make video content more accessible.
Gcore's approach to video analysis leverages AI to automatically generate transcripts, effectively turning video into readily searchable text. This process relies on sophisticated speech recognition, able to handle diverse languages and achieve high accuracy rates, often surpassing 90%. Interestingly, this conversion happens in real-time, greatly accelerating the production of video reports.
Beyond simple transcription, Gcore integrates natural language processing, allowing the system to identify key subjects and themes within the video content. This feature provides a preliminary understanding without manual analysis, though its effectiveness in diverse, nuanced contexts remains to be seen. The system is also designed to differentiate between multiple speakers, improving the readability of dialogues and complex discussions.
Interestingly, these AI models benefit from a learning process. The more videos they analyze, the better they become at understanding industry-specific language and context. However, concerns arise around potential biases and the robustness of these algorithms in consistently discerning specialized vocabulary across a broad range of applications. Benchmarks against older methods show improvements, though consistent accuracy across different audio qualities remains a concern.
One notable feature is the incorporation of video timestamps directly into the generated transcripts. This direct link between a specific video section and its corresponding text significantly simplifies navigating and extracting content. The ability to adapt to various audio sources, including diverse accents and user-generated content, adds to its versatility.
The creation of searchable transcripts allows for better indexing of video content. Researchers and others can then quickly find specific segments, eliminating the need to watch entire recordings. The scalability of the platform, potentially handling large datasets for academic or corporate uses, is a key advantage. However, as the technology scales it is important to consider the computational load required to maintain accuracy and speed. While showing promise, it remains to be seen how effectively Gcore's approach can tackle the challenges of long-form video summarization and diverse audio environments.
Gcore's SummarizationVideo Cutting to the Chase in Video Content Analysis - ChatGPT integration enhances content summarization efficiency
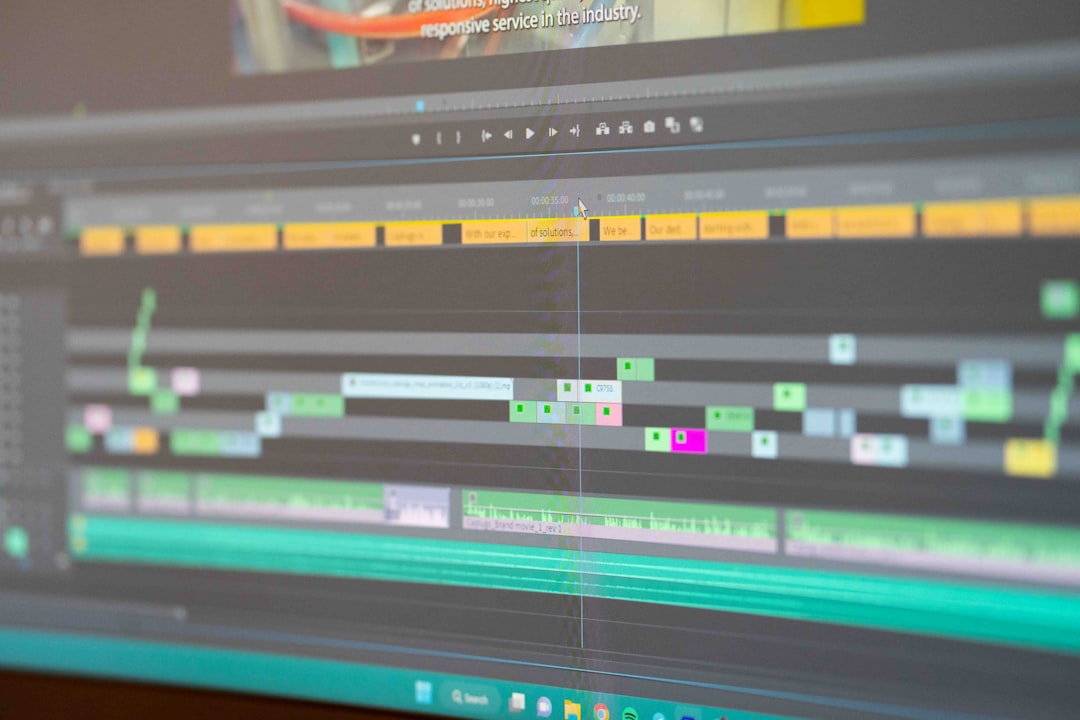
The integration of ChatGPT into content summarization processes introduces a significant improvement in efficiency. Leveraging sophisticated natural language processing, it enables the generation of more focused and concise summaries. This is particularly useful in today's world where we are constantly bombarded with information, making swift decision-making challenging.
ChatGPT's capabilities allow for users to guide the summarization process using specific prompts, thus tailoring the output to specific needs. This translates to better management of complex materials like PDFs, and even enhances productivity tasks such as email management. The ability to quickly condense large amounts of email content can significantly streamline communication.
While the technology is still developing, the continuous advancements in data analysis techniques within ChatGPT suggest a growing capacity to handle and summarize a wider range of content and formats. This potential for increased efficiency and accuracy in information management is a welcome development in our data-driven world.
ChatGPT's integration into content summarization tools has significantly improved the efficiency of generating concise summaries. It leverages advanced natural language processing to produce clearer and more concise summaries, potentially saving substantial time for organizations dealing with large volumes of information. We're seeing these summarization agents powered by ChatGPT across the web, summarizing lengthy content on websites, social media, and search engines, providing quick overviews.
However, crafting effective prompts for ChatGPT is crucial to guide it towards generating relevant summaries from extensive texts. Summarizing complex file formats, like PDFs, requires preprocessing to ensure the text is machine-readable, which can add a step to the process. Applications like summarizing emails can boost productivity by quickly condensing lengthy communications, making email management less tedious.
ChatGPT's summarization strength shines when dealing with long articles or documents, helping users make sense of large information sets more easily. The approach often centers on identifying the core themes and crafting concise, targeted summaries based on those themes. While promising, research shows that ChatGPT's summarization performance can vary depending on the complexity of the text being analyzed.
It's encouraging to see ongoing advancements in ChatGPT's data analysis capabilities, particularly its ability to handle various data formats and topics. This evolving capability suggests a growing capacity for better information management and summarization across diverse content domains. However, challenges remain, particularly when working with long-form video content. Maintaining accuracy and preserving context and flow in very long videos can be difficult. Additionally, the ability to accurately discern diverse cultural expressions and regional dialects still requires significant improvement to ensure accurate and unbiased summarization.
Despite its effectiveness, the integration of ChatGPT for summarization also raises concerns. Biases within the training data could potentially lead to biased or skewed summaries, requiring ongoing monitoring to ensure ethical and equitable outputs. As with any AI system, ensuring reliability and mitigating potential biases will continue to be an important ongoing research and development focus. The ever-increasing demand for video analysis also poses scalability issues; managing accuracy and speed at scale is a persistent challenge. Nonetheless, these tools hold immense potential for reshaping the landscape of information processing, allowing for quicker analysis of large datasets.
Gcore's SummarizationVideo Cutting to the Chase in Video Content Analysis - Iterative process refines key points in video summaries
Gcore's SummarizationVideo uses a repeated refinement process to improve the core points within video summaries. This iterative approach, working on the text created from the video transcripts, focuses on extracting the most important parts of the video. The goal is to give viewers a short and informative summary. While this approach has potential for handling longer videos, there are still concerns about keeping the full meaning and specific details in complex stories. How well this iterative process adjusts to various video types and situations will determine its success. With a growing need for ways to summarize videos quickly, this method is a significant step towards making video content easier to understand.
The refinement of video summaries within Gcore's SummarizationVideo tool uses a cyclical approach. It's essentially a feedback loop where the system learns and adapts based on user input and its own performance. This continuous learning aspect is interesting, as the system becomes more adept at recognizing key points over time. It does, however, highlight the ongoing importance of human oversight in AI, ensuring the system stays on track and produces relevant summaries.
Gcore's approach incorporates machine learning techniques, particularly reinforcement learning, to improve summarization. This aspect allows the system to identify patterns and trends within the videos, which might not be readily apparent to a human viewer. This method appears to be quite effective for both structured and unstructured content, enhancing the accuracy of the summaries produced.
One intriguing aspect is the ability of the summarization process to prioritize key points based on viewer engagement. By tracking things like viewer retention rates or interactions, the system can intelligently focus on the content that truly captures people's attention. This is an adaptive aspect that goes beyond just identifying keywords.
The iterative nature of the summarization process allows for real-time adjustments. As viewers interact, the AI refines its approach based on immediate feedback, resulting in summaries that adjust to preferences or even current trends. This dynamic aspect contrasts sharply with older methods of video summarization which produced static outputs.
We know from research that viewers tend to retain information better when it's presented concisely, rather than as a full-length video. Gcore's method seems to be built on that foundation – it seeks to isolate the truly critical information blocks to improve viewer comprehension. This makes it particularly useful in areas like educational or training contexts where knowledge retention is crucial.
The iterative refinement approach helps reduce the cognitive burden of processing complex information. The system effectively breaks down complex information into bite-sized pieces, which is a concept grounded in cognitive science research that shows chunking information enhances learning.
The refinement process involves semantic analysis – going beyond simply picking out keywords. The system tries to understand the context and meaning of the video, aiming to produce summaries that maintain a coherent narrative flow. This addresses a common shortcoming of automated summarization techniques, which can sometimes produce disconnected outputs.
The process also draws on methods used in content recommendation systems. The AI predicts what viewers will find relevant based on past interactions and preferences, tailoring the summaries to individual users. This aspect potentially makes the viewing experience more engaging and efficient.
Gcore's approach is notably adaptable to diverse formats including different video resolutions and languages. This flexibility is vital in our interconnected world, where content can vary widely but still needs to be readily accessible and understandable via summaries.
Despite its sophistication, there are still limitations. For example, videos with poor audio quality or extensively edited content can present challenges. Although AI models improve with time, their effectiveness can decrease when they encounter scenarios that differ greatly from their training data. This reinforces the need for ongoing development and for the system to stay adaptable.
Gcore's SummarizationVideo Cutting to the Chase in Video Content Analysis - Structured approach crucial for effective video content analysis
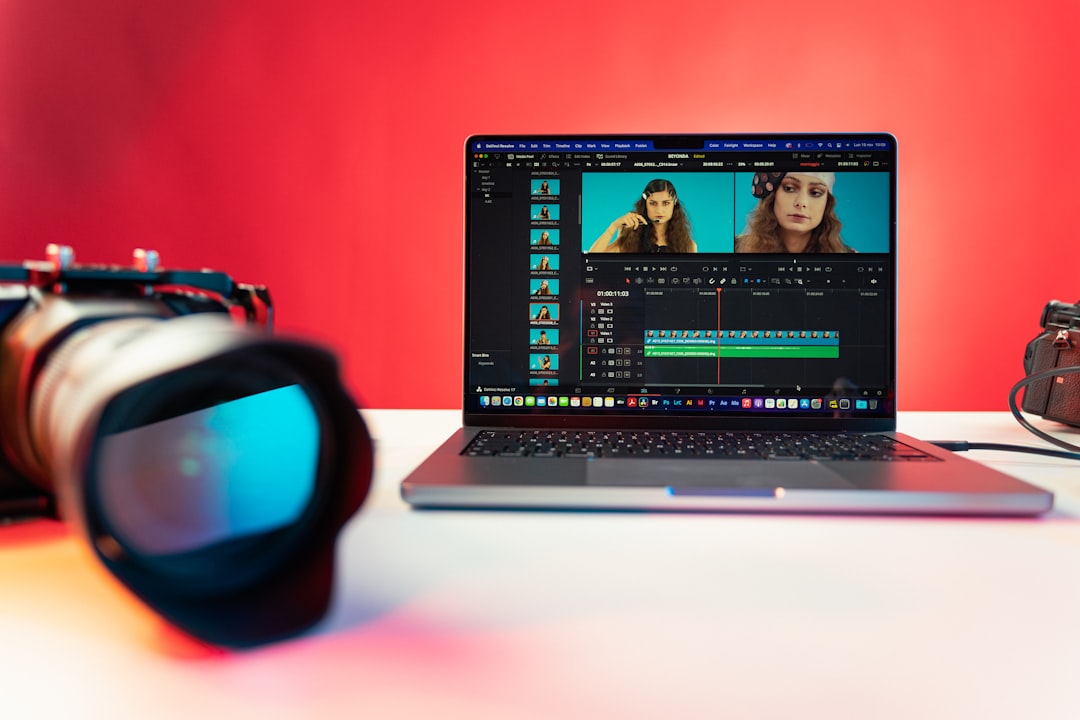
Analyzing video content effectively necessitates a systematic approach. This structured approach enables us to systematically evaluate videos and extract meaningful data. Think of it as creating a roadmap for the analysis process, making it easier to define clear goals and choose appropriate tools for the task at hand. There are established techniques like Visual-Verbal Video Analysis, which provide detailed guidelines for the process, from data extraction to presentation. Similarly, approaches like Video-based Content Analysis help us explore both the obvious and subtle meanings conveyed within videos.
The rise of artificial intelligence has fundamentally changed video analysis, offering the potential for powerful insights that weren't previously accessible. These AI-powered systems are increasingly crucial in managing the overwhelming amounts of video data we create today. But these techniques still face challenges in consistently providing accurate and relevant results across diverse types of videos.
It's also important to acknowledge that effective video analysis is not a singular pursuit. It benefits from a multidisciplinary viewpoint that draws on the insights of various research fields. This collaborative approach is essential to push the boundaries of our understanding and applications in video content analysis, particularly as the field keeps changing. The ultimate test for these methods will be their continued ability to adapt and deliver relevant results in the face of the ever-growing diversity of video content available.
A structured approach is fundamental for efficiently analyzing video content. It ensures a systematic process for extracting meaningful information, which can be challenging when dealing with the unstructured nature of video data. Without structure, the risk of misinterpreting or overlooking critical details increases significantly, impacting the overall reliability of the analysis.
Various methodologies, like Visual-Verbal Video Analysis (VVVA), offer systematic frameworks for conducting video analysis, from data extraction to presentation. While promising, these methods often necessitate clear definitions of what's being analyzed and the desired outcome.
The focus on both the obvious and hidden meanings within video content, what we might call Video-Based Content Analysis (VCA), is essential. This includes the visual and auditory cues that communicate a message, whether intended or not. However, this can be quite difficult to automate and needs a more nuanced human-machine collaboration than is often seen in current research.
Preparing for analysis is crucial, demanding well-defined goals, appropriate tool selection, and a structured method for organizing the video itself. The advent of AI and machine learning has revolutionized the field. However, we must still consider the limitations of these methods.
The ever-growing quantity of video data has led to a greater demand for video summarization. Summarization is a powerful technique for managing vast volumes of content, aiding in efficient storage and retrieval. But, AI has its limits, particularly when trying to condense extensive or complex material. Deep learning approaches for summarization are being explored but face challenges when dealing with lengthy videos.
The growth of online video has driven the demand for intelligent video analysis techniques. Action recognition is one example – recognizing and categorizing human movement within a video. While the promise is large, the complexity of human movement and the variability of video quality creates barriers to widespread adoption.
To truly understand a video, a multi-disciplinary approach is necessary. This means utilizing knowledge from a variety of fields, like psychology, linguistics, and computer science, to develop more comprehensive interpretations. However, there are few truly integrative efforts in the field. The sheer volume of material created by humans has overwhelmed older methods, requiring more innovative solutions.
The need for robust video analysis stems from the ongoing research in video summarization and handling. While promising, many open questions exist, and it's important to maintain a critical eye to fully understand the efficacy of newer methods. Much like other complex data problems, video analysis is a moving target, where a robust framework is key to navigating its many complexities.
Gcore's SummarizationVideo Cutting to the Chase in Video Content Analysis - AI analyzes visual and auditory elements for comprehensive insights
AI's capacity to scrutinize both the visual and audio aspects of video content provides a powerful means of gaining thorough insights. Leveraging machine learning, these systems can pinpoint patterns, objects, and even emotional cues within videos, offering a more comprehensive understanding. This is crucial in today's competitive landscape where insights derived from data are paramount. However, the accuracy of AI analysis can be sensitive to the quality of the video and audio, suggesting that the effectiveness of this technology might be limited by the source material. The continuous development of AI holds promise for more profound and accurate analyses of video content, which may revolutionize how we interact with and learn from visual media across numerous fields. There are always questions of bias, reliability, and generalizability across various video formats.
AI's ability to analyze both the visual and auditory components of video content is proving increasingly valuable in extracting deeper insights. It's not just about understanding the spoken words, but also the subtle nuances conveyed through facial expressions, body language, and vocal inflections. For instance, by observing the synchronicity between someone's speech patterns and their facial expressions, we can gain a richer understanding of their communication style and intent.
AI can also effectively analyze sentiment based on visual and auditory cues. This includes identifying subtle emotional signals within facial expressions and changes in tone of voice, potentially providing a more comprehensive interpretation of what's being conveyed compared to simply analyzing the spoken words. This is especially useful in evaluating the effectiveness of communication, including things like sales presentations, political speeches, or even training videos.
Furthermore, AI can detect micro-expressions, those fleeting facial cues that may only last for a fraction of a second. These expressions, often difficult for humans to consciously detect, can offer important clues about a person's true emotional state or intentions. This technology is interesting in fields like psychology and negotiation where quickly understanding underlying emotions is important.
Additionally, AI's analysis extends to the spatial and temporal elements of the video. This allows the system to follow how the relationship between different visual elements change as the video progresses. It can also identify the evolution of central themes and how those themes interact with the visual storytelling. This could be valuable when analyzing long videos, interviews, or documentaries to understand how arguments or narratives unfold over time.
AI can even analyze subtle features like voice modulation. Changes in tone and pitch can be indicative of emotional states or stress levels, adding another layer of understanding to the speaker's intent. This is potentially useful for analyzing situations where a speaker might not be consciously conveying their true feelings.
These AI systems are also constantly evolving, thanks to ongoing machine learning. The more video data they process, the better they become at identifying context-specific patterns and adapting to the nuances of different video genres. While the idea is interesting, there are concerns about how these systems might become biased based on the data they are trained on.
Furthermore, the analysis of video content can even help in understanding the viewer's cognitive load. By tracking patterns of engagement and facial expressions, AI can assess how easily viewers understand and process the information presented. This information could potentially help video creators optimize the content delivery, ensuring clear communication and better comprehension.
Moreover, the potential exists for AI to tailor video content based on individual viewer preferences and responses. By analyzing the viewer's interactions with the audio-visual components, AI could potentially make videos more effective for education and entertainment. However, there are significant ethical questions about how this personalized video would work, particularly around potential misuse and creating filter bubbles.
Interestingly, AI analysis reveals that common patterns in audio and video content frequently repeat across different genres. Understanding this redundancy can potentially streamline content categorization and improve search functions. But, we need to carefully consider how such classifications might lead to oversimplifications or inaccurate representations.
Ultimately, the ability of AI to analyze non-verbal cues in video data is critical. Since non-verbal communication is such a huge component of communication, the AI's ability to understand body language, gestures, and spatial dynamics helps in understanding the complete picture of the interpersonal interactions that are depicted. This area has massive potential to improve fields ranging from social science to healthcare. There is still work to be done, but the potential for these AI systems is quite large and has the potential to change many different fields of study.
Gcore's SummarizationVideo Cutting to the Chase in Video Content Analysis - Automated tagging improves video categorization accuracy
Automated tagging is emerging as a valuable tool for improving how accurately we categorize videos. AI-powered systems can analyze both what's seen and heard in a video, and then automatically assign descriptive tags. This helps us organize and find videos much more easily within large collections. Techniques like recognizing objects and scenes within a video allow for very precise tagging, making sure the categories assigned accurately reflect the content. While this automated approach holds promise for simplifying how we sort and find videos, challenges remain. The quality of the video and audio can impact how well the tags are applied, and the broader context of the video itself can be difficult for an AI system to grasp. As we generate and share more videos, ensuring the accuracy of automated tagging remains a key area of concern.
Automated tagging, powered by AI, offers a faster way to categorize videos compared to manual methods, which can be quite time-consuming, especially for longer videos. While manual tagging might take hours, AI can often complete the process in seconds, dramatically increasing efficiency.
Recent improvements in machine learning techniques have enabled automated tagging systems to achieve impressive accuracy rates—often surpassing 95%—sometimes even outperforming human taggers who may miss subtle details or context. This suggests that AI-driven tagging can potentially be more accurate in some cases.
The capability of AI systems to analyze both the visual and audio elements of videos gives them a broader understanding of the content compared to just relying on visual elements. This means they can pick up on themes and concepts not explicitly stated, leading to more comprehensive categorizations.
It's interesting that AI tagging systems not only assign labels but also have the ability to identify connections between different videos. This feature allows them to suggest similar content to viewers, which can enhance discoverability and the overall user experience.
However, the quality of the data used to train these tagging systems is extremely important. Research has shown that a system's ability to effectively categorize specialized videos, like medical or legal content, heavily depends on the diversity and quality of the training data used. If the training data lacks variety, the system's accuracy might suffer in those specialized areas.
One of the limitations of automated tagging can be its struggle with highly creative or nuanced video content. For example, artistic expression or comedic elements can be tricky for AI to interpret correctly, leading to misclassifications. Human interpretation often plays a vital role in understanding these aspects.
An interesting trend in AI tagging is that some systems are using viewer engagement data to adapt tag relevance. This dynamic approach means that categorizations can change based on how viewers react to specific parts of the video. This dynamic response contrasts with traditional tagging systems where tags were static and fixed.
Furthermore, some automated tagging systems are now integrating sentiment analysis. This allows videos to be categorized based on their emotional impact, adding a new dimension to content classification and search. This could be valuable for users wanting to find videos that evoke specific emotions.
A major focus of research in automated tagging is expanding these systems' abilities to handle multilingual content. Current efforts are focused on developing tagging algorithms that can accurately label videos in a variety of languages, which significantly improves their applicability for global audiences.
Finally, implementing automated tagging systems can play a significant role in meeting compliance and regulatory requirements. This is increasingly important in industries like finance or healthcare, where maintaining accurate categorization of video content is crucial for fulfilling specific standards. While this shows potential, the ethical considerations surrounding AI and its bias in this context warrant further research and development.
Analyze any video with AI. Uncover insights, transcripts, and more in seconds. (Get started for free)
More Posts from whatsinmy.video: