Analyze any video with AI. Uncover insights, transcripts, and more in seconds. (Get started for free)
How Different Data Annotation Platforms Handle Video Labeling Tasks A 7-Platform Analysis for 2024
How Different Data Annotation Platforms Handle Video Labeling Tasks A 7-Platform Analysis for 2024 - How Labellerr Cuts Video Labeling Time by 40 Percent Through Frame Interpolation
Labellerr's approach to video annotation focuses on significantly reducing the time it takes to label video data by utilizing frame interpolation. They claim a 40% reduction in labeling time through their method, which relies on model-assisted annotation to essentially "fill in the gaps" between video frames. This tackles a common pain point in video annotation: ensuring accurate labeling across frames, particularly when dealing with differing frame rates or synchronization issues. By interpolating annotations across frames, Labellerr aims to smooth out the labeling process for complex objects and scenarios. This makes their platform potentially attractive to industries that rely on large-scale video annotation projects, such as healthcare, automotive, and retail. Given the increasing trend toward automation in data annotation, Labellerr's technology could be a valuable contributor to a marketplace that's continually evolving to meet the demands for more efficient and cost-effective video labeling solutions.
Labellerr's approach to video labeling involves creating synthetic frames between existing ones, effectively increasing the temporal resolution without needing to capture new footage. This frame interpolation technique hinges on algorithms that predict how objects move within a video sequence. Essentially, it's about cleverly filling in the gaps between frames.
By lowering the number of frames that need manual annotation, Labellerr significantly reduces the workload for video labeling. Anecdotal evidence suggests that annotators spend a significant portion of their time dealing with individual frames, rather than analyzing the overall video flow. This means any technique that reduces the number of frames requiring individual attention has major implications for efficiency.
Labellerr incorporates sophisticated machine learning approaches to anticipate frame motion, and it's claimed this can decrease annotation time by up to 40%. The speed-up has implications for project management, enabling teams to finish projects faster.
This method also leads to smoother transitions within the annotated video, maintaining visual context during annotation. In complex scenes, these interpolated frames with their predicted motion can be helpful to improve the overall quality of training data.
Labellerr aims to retain visual consistency across interpolated frames, a crucial element when using these frames for machine learning. This approach is meant to enhance the quality of the labeling process and benefit downstream tasks like model training.
Focusing on key events rather than spending time on frames with minimal information is another potential advantage of frame interpolation. This prioritization potentially leads to a better-quality dataset for tasks like surveillance and autonomous driving.
Reduced labeling time directly translates into lower labor costs, especially with lengthy datasets. Given that video annotation can be a time-consuming and expensive process, even minor efficiencies can make a substantial difference in resource allocation.
The benefits of Labellerr's approach can be seen in various industries like healthcare, where fast analysis of medical videos could accelerate diagnostics and decision-making. Imagine the potential in patient monitoring or analyzing surgical footage.
However, frame interpolation isn't a perfect solution. Inaccurate motion estimation can produce anomalies that could confuse human annotators. It's crucial to manage these issues to prevent them from negatively impacting the data quality.
The continued improvement in frame interpolation technology may drive the development of more automated labeling tools. This raises interesting questions about the future of human involvement in video annotation. The ideal balance between automation and human oversight remains a point of discussion, directly affecting data quality and overall project effectiveness.
How Different Data Annotation Platforms Handle Video Labeling Tasks A 7-Platform Analysis for 2024 - Scale AI Machine Learning Pipeline Reduces Manual Video Annotation Steps from 8 to 3
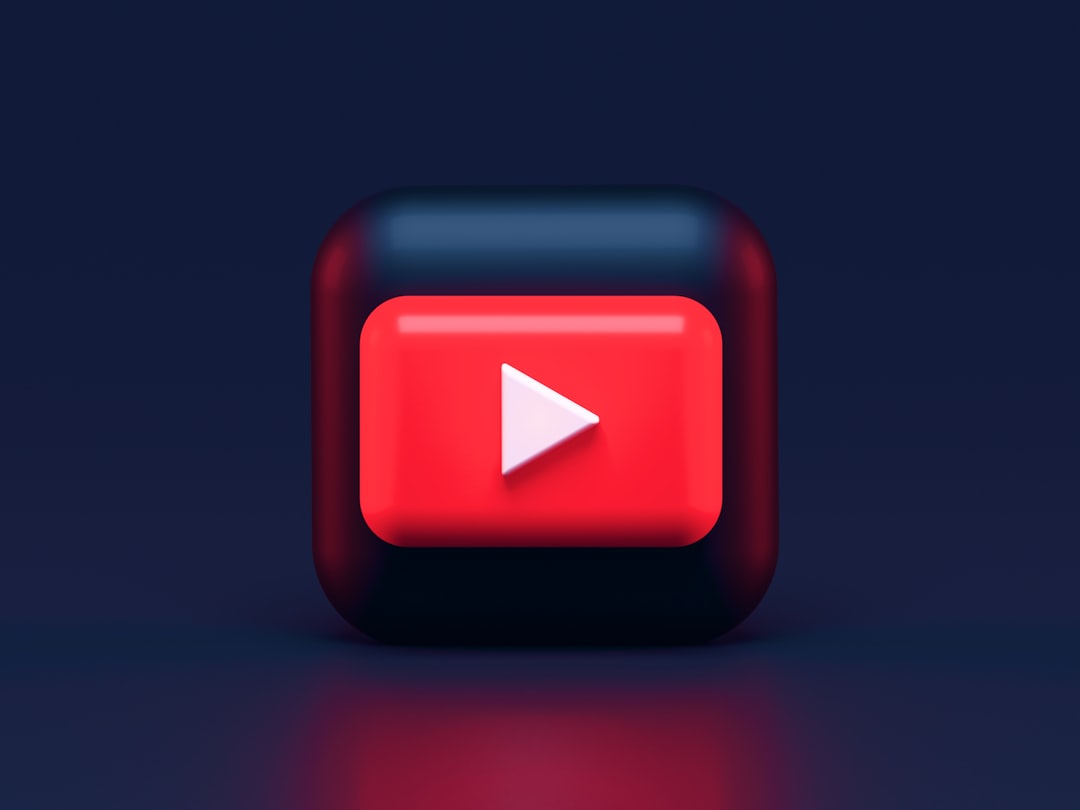
Scale AI has introduced a refined machine learning pipeline that streamlines the manual annotation process for videos. Instead of the typical eight steps, their system now requires only three, creating a more efficient workflow. This approach marries AI-powered techniques with human oversight, a combination designed to improve both the speed and quality of the annotations. Their ability to process a massive 10 million annotations each week highlights their capacity for handling large-scale video annotation projects. While they've incorporated automation into the process, the need for human intervention remains essential in maintaining the accuracy and consistency of the video annotations. The reduction to just three steps represents a potential shift in how video annotation is handled, which could impact industries relying heavily on video data for various tasks. It remains to be seen how widely this approach will be adopted, but it certainly offers a new direction for tackling the challenges of large-scale video annotation.
Scale AI's approach to video annotation hinges on a machine learning pipeline that streamlines the process, reducing the number of manual steps from a rather cumbersome eight down to just three. This reduction, achieved through a combination of AI and human expertise, appears to be driven by an attempt to optimize the workflow and likely minimize the chance for human errors creeping into the labeling process by lessening the need for repetitive tasks.
Interestingly, Scale AI appears to use techniques like active learning to guide annotators towards the most valuable parts of the video data, allowing them to focus on intricate sequences rather than wasting time on segments that are easily annotated. This idea suggests a smart way to allocate annotator time where it will be most impactful for the quality of the final annotated dataset.
They seem to use computer vision algorithms that help to predict movement within the videos and provide real-time suggestions for annotators, suggesting they are attempting to automate some parts of the annotation process without sacrificing precision. This potentially increases the speed of the entire process and offers a significant benefit when dealing with large volumes of data.
Reducing the manual involvement in the process not only speeds things up but arguably lessens the mental strain on the human annotators. This might translate to higher-quality annotations as annotators can focus better on the tasks at hand, avoiding some of the potential cognitive fatigue from tedious repetitive labeling.
Scale AI's platform is designed to work with various video formats and resolutions, suggesting it’s built to be flexible enough to serve a range of industries from movies and entertainment to fields like self-driving cars that typically have unique data characteristics.
The inclusion of automated quality control measures within their system appears to be a way to guarantee consistency in the quality of the data labeled. This potentially decreases the need for multiple human checks throughout the labeling process and streamlines the whole flow.
Their system's ability to manage large datasets quickly is noteworthy and could be a crucial advantage in sectors like security where rapidly analyzing surveillance videos might have implications for safety and security.
One aspect of their approach that stands out is the capacity to create detailed metadata along with the annotations. This is a clever way to increase the richness of the dataset and should improve performance for subsequent machine learning tasks using the annotated data.
The possibility of reducing the number of annotators needed without compromising the quantity and quality of the output is a compelling aspect of Scale AI's platform. This implies that achieving efficiency in the annotation process might lead to significant cost savings and potentially enables handling a greater volume of video projects. While this all seems quite promising, it's important to note that relying on automated solutions can still come with their own set of challenges and potential drawbacks.
How Different Data Annotation Platforms Handle Video Labeling Tasks A 7-Platform Analysis for 2024 - SuperAnnotate Multi Object Tracking System Handles 4K Videos at 60 FPS
SuperAnnotate's video annotation system is capable of handling high-resolution 4K videos at 60 frames per second, which is a significant feat in the field of video annotation. It provides the ability to track and identify multiple objects as they move across a sequence of frames, a key feature for many AI applications. SuperAnnotate's "Autotrack" function seeks to make the process more efficient by automating certain tasks, but the core annotation workflow still relies on identifying and labeling objects in each frame. While aspects like consistent object ID maintenance and object splitting during annotation show potential for a refined labeling experience, video annotation inherently requires more effort compared to still images because of the frame-by-frame analysis. However, there are reports that integrating SuperAnnotate with data processing platforms can lead to substantial reductions in annotation time, potentially making it a valuable tool for projects that require comprehensive video labeling.
SuperAnnotate's multi-object tracking system can handle 4K videos at 60 frames per second, which is quite impressive for maintaining visual clarity and detail. This capability is important when tracking multiple objects, especially in complex video scenes. They've built a system that can track and pinpoint objects across individual frames, making it useful for tasks that rely on understanding object movement within videos. The platform, being recognized as a top AI company, seems to have gained some industry credibility.
Their approach to video annotation is quite typical – they split videos into individual frames just like other platforms. While manual annotation is an option, they also offer automated features like "Autotrack" to assist with the often-tedious process of tracking objects across frames. This type of automation is a trend across these platforms, and SuperAnnotate follows suit with this common method.
One interesting feature is their focus on consistently maintaining unique object IDs across frames. This is useful for object detection and tracking applications, but it does add an extra layer of work for the annotation process. They also give annotators flexibility by allowing them to divide objects during the annotation process, enhancing precision and tailoring the labeling to specific requirements.
SuperAnnotate also emphasizes data security. It keeps user data on-premise and only allows read-only access. This is becoming a more important point for many companies when they use external annotation platforms. Video annotation, in general, is quite labor-intensive because of the need to look at each frame individually. However, the platform reportedly speeds things up in some use cases. Their combination with data processing platforms like Databricks has reportedly been shown to reduce annotation time by more than 60%. This is a significant improvement when compared to the slower speeds of manual annotation alone. It raises questions on the specific types of tasks that this platform is optimized for. How much of this 60% reduction is dependent on the data processing capabilities of Databricks?
How Different Data Annotation Platforms Handle Video Labeling Tasks A 7-Platform Analysis for 2024 - V7 Labs Automated Keyframe Detection Spots 89 Percent of Critical Frames
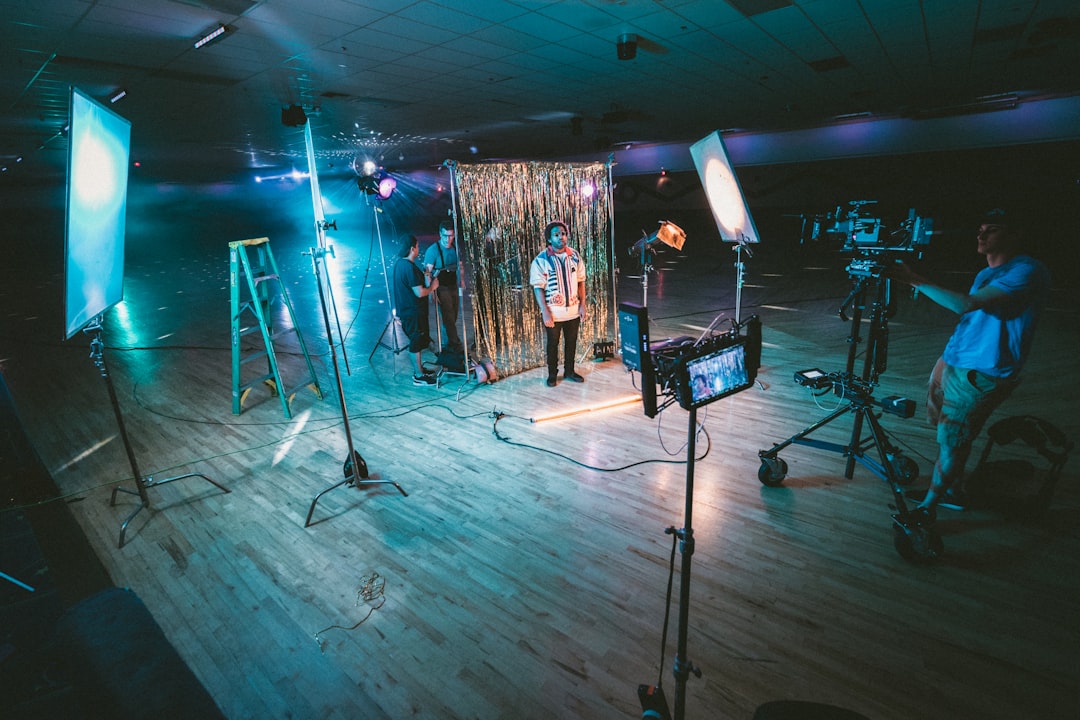
V7 Labs' automated keyframe detection system is noteworthy for its ability to identify a substantial portion of crucial frames in video data, reaching an 89% success rate. This achievement speaks to the platform's capability to efficiently navigate the complexities inherent in video analysis, where accuracy is essential. V7 offers a range of annotation tools, including bounding boxes and masks, that can accelerate the labeling process and enhance accuracy across diverse industries like healthcare and finance. Furthermore, features like Interpolate streamline the depiction of changes within videos, simplifying the annotation experience. While the reliance on automation offers potential for significant efficiency gains, it also necessitates a careful consideration of the balance between automation and human input to maintain the highest standards of data quality. There are natural questions about whether the platform might sacrifice accuracy at times due to automation and the need for human oversight remains.
V7 Labs' automated keyframe detection has demonstrated an impressive 89% success rate in identifying critical frames within video data. This high accuracy suggests their system effectively pinpoints the frames that contain the most important information, potentially minimizing the need for extensive manual review. This approach seems to leverage sophisticated computer vision techniques and machine learning algorithms to go beyond simply looking at differences between frames. It appears to understand the nuances within the video that make specific frames important, leading to more precise selection.
This capability allows V7 Labs' platform to differentiate between frames that hold essential information and those that are less informative. Consequently, video annotation workflows can be streamlined. Teams can focus their efforts on the most relevant parts of the video, potentially leading to more efficient use of time and resources when labeling videos. Furthermore, their ability to work with diverse video resolutions, including high-definition formats, allows it to handle a wider range of video datasets. This versatility suggests a potential application in numerous fields like entertainment, security, and sports analytics.
The V7 Labs approach stands out because it helps reduce manual annotation, a frequent bottleneck in video annotation workflows. By intelligently identifying the frames where changes are most likely to occur, it reduces the sheer volume of video that needs to be examined in detail. This can translate to faster project completion times, which is often a high priority for teams involved in video analysis. However, it's worth noting that the performance of the keyframe detection algorithm is impacted not just by data volume but also its quality. V7 Labs emphasizes the need for well-prepared training data, highlighting an important factor to consider when planning implementations of this type of technology.
While V7 Labs' system is designed to handle the majority of keyframes, it's important to recognize that it’s not perfect. They are transparent about the remaining 11% of frames that require human oversight. This necessary human intervention is critical to ensuring the overall quality and reliability of the annotated video data. Their system can process videos at considerable speeds, which is a key advantage when handling large datasets without compromising accuracy. This quick processing can be crucial in environments where timely insights are critical, such as sports analytics or crisis response settings.
Ultimately, V7 Labs' keyframe detection capability offers a potential pathway to creating more focused training datasets for machine learning models. By emphasizing those critical frames, this method can help curate more targeted datasets. This targeted approach might potentially improve the performance of models for tasks like object recognition and activity classification. Additionally, by lowering the labor required for manual reviews, this method could produce significant cost savings for video annotation projects. This economic advantage is likely to resonate with organizations focused on minimizing costs and speeding time-to-market.
How Different Data Annotation Platforms Handle Video Labeling Tasks A 7-Platform Analysis for 2024 - Appen Workforce Network Labels 1 Million Video Minutes Per Month Across 235 Languages
Appen's global workforce is responsible for labeling a substantial amount of video data—one million minutes each month, covering a remarkable 235 languages. They achieve this scale by utilizing a massive network of over a million skilled individuals situated in 70,000 locations across the globe. This vast and diverse group of contributors allows Appen to handle data annotation across a wide variety of formats, including video. While the breadth of Appen's capabilities in handling diverse languages and data types is noteworthy, the sheer volume of video data being processed could potentially pose challenges. Maintaining consistent and accurate annotation across such a large dataset and wide range of languages may prove difficult. Particularly when compared to more automated and efficiency-driven approaches emerging from other companies within the data annotation field. The balance between the scale of Appen's human network and the potential for quality control issues when dealing with complex video annotation tasks remains a point to consider.
Appen's workforce, spread across 170 countries and comprising over a million individuals, is capable of labeling a massive 1 million minutes of video data each month, covering a remarkable 235 languages. This sheer scale of operation speaks to their ability to handle enormous video annotation projects efficiently. While the ability to support such a wide range of languages is a major plus, it also raises questions about the consistency and accuracy across all of these languages.
Beyond simply labeling video content, Appen's platform caters to a diverse set of annotation needs, including tasks like object identification, sentiment analysis, and pinpoint event tagging. This adaptable approach allows it to address the annotation demands of a variety of industries, from entertainment to security, however it might imply a lack of specialization compared to other platforms tailored for specific industries.
Although Appen boasts an impressive AI-assisted platform, human annotation remains a cornerstone of their process. It's worth noting that around 90% of annotations undergo human review to ensure quality. While this helps maintain a high standard, it can also introduce potential bottlenecks in the annotation process when scaling to larger datasets.
Their use of active learning approaches suggests an attempt to iteratively improve annotation accuracy. By continuously incorporating past annotation data into their models, they are aiming to create a more robust and reliable labeling process. The effectiveness of this refinement remains a question that likely needs more extensive exploration.
The focus on data security is also important, especially as companies in sensitive industries use external annotation platforms. Appen's strict confidentiality policies offer a measure of reassurance when dealing with sensitive video data. However, maintaining security at this scale is a constant challenge, and any vulnerabilities could have substantial negative impacts.
There's a distinct emphasis on understanding the temporal context of the videos. This means their systems are being designed to identify crucial events and actions within a sequence of frames. This contextual awareness is valuable for applications like incident analysis or content moderation, though it raises questions about how well this contextualization works in practice for a wide variety of content types.
Given its scalable approach and comprehensive infrastructure, Appen positions itself as a cost-effective solution for companies seeking to reduce the expense of data annotation. This can be attractive for businesses looking to streamline data pipelines. However, it remains to be seen how they balance cost-effectiveness with the complexity and high-quality standards that some industries require.
The platform's ability to easily integrate with other systems adds to its appeal for companies aiming to integrate annotation processes into larger data workflows. This integration flexibility is a clear advantage in today's interconnected data landscapes. Yet, seamless integration across all systems isn't always guaranteed, and issues could arise with varying levels of platform compatibility.
Finally, Appen attempts to tailor their approach to specific industries. The implication is that they understand the diverse annotation needs across various sectors and can provide optimized services. This customized approach should lead to more effective model training for specialized applications. But one might question how deeply specialized these industry-specific adaptations are given their focus on being a general-purpose platform for a vast number of languages and use cases.
How Different Data Annotation Platforms Handle Video Labeling Tasks A 7-Platform Analysis for 2024 - Labelbox Active Learning Models Cut Video Processing Costs by 35 Percent
Labelbox's approach to video annotation incorporates active learning models to reduce processing costs by 35%. The core idea is to intelligently prioritize the most important data for labeling, so teams can focus on the parts of the data that will have the largest impact on model performance instead of simply labeling more data. This is a departure from the old way of thinking that simply labeling more data is always better. Labelbox's tools, including those for tracking objects and segmenting images, help make the annotation process more accurate and efficient, which is especially useful for video data. The built-in video editor also makes it faster and easier to find specific parts of a video and make edits. While this emphasis on efficiency and automated techniques can be useful, it also raises questions about the potential for mistakes to slip through. Maintaining quality control across a process that emphasizes speed and prioritization is a concern that teams using the Labelbox platform should keep in mind.
Labelbox's approach to video annotation leverages active learning models, resulting in a reported 35% reduction in video processing costs. This reduction in costs is a significant development, as it allows companies to redirect resources towards other vital areas, such as developing more advanced AI models. Active learning, in essence, is a strategy that focuses annotation efforts on the data points most likely to enhance the model's overall performance. This intelligent data selection process helps to prevent annotators from spending time on data that might not significantly improve the final model.
The emphasis on data selection helps to reduce errors often found in manual labeling, as annotators are presented with the most challenging or ambiguous data. This approach to intelligent annotation suggests a potential for scalability. Labelbox's system may be capable of handling increasingly large datasets without needing a corresponding increase in annotation expenses. Additionally, the active learning methods in Labelbox appear to be quite adaptable. As data is processed and labeled, the underlying active learning model can refine its approach to maximize annotation efficiency in real-time.
However, the move towards active learning involves a degree of upfront setup and configuration. Companies need to spend time setting up and training the model in their specific industry and application, which can be an initial hurdle. Despite this initial challenge, Labelbox's methods seem applicable across many industries, such as security, autonomous vehicle development, or entertainment. This versatility is advantageous, allowing the platform to adapt to a wide range of data types and annotation requirements. The 35% reduction in processing costs provides a tangible and compelling argument for companies considering Labelbox. When cost pressures are high, demonstrating these types of results can help to secure budgets and funding for newer data labeling approaches.
The Labelbox model provides an interesting example of human-AI collaboration. By using active learning, the role of the human annotator is refined and focused on the data that is most important for the project. The partnership between the human and the AI within the system could be an important factor in maintaining overall data quality, as well as reducing errors. By investing in systems like Labelbox that reduce operational costs, companies can potentially position themselves for increased profit margins on future projects. This forward-looking perspective on operational efficiency could be a deciding factor for companies who are focused on long-term returns.
How Different Data Annotation Platforms Handle Video Labeling Tasks A 7-Platform Analysis for 2024 - DataLoop AI Object Persistence Tracking Maintains 95 Percent Label Accuracy Across Frames
DataLoop AI's Object Persistence Tracking feature claims to maintain a high level of label accuracy—95%—across video frames. This is largely attributed to how it uses interpolation techniques to fill the gaps between keyframes, resulting in smoother object tracking that reduces the need for manual adjustments on every frame. Furthermore, DataLoop's approach involves integrating machine learning models to pre-annotate video data, potentially leading to a reduction in human error during the labeling process. While this integration of AI is intended to enhance efficiency and output, it also brings up the recurring concern in video annotation: the need to find the optimal balance between automation and human oversight to preserve annotation quality. The features DataLoop utilizes showcase both the potential upsides of AI in streamlining video annotation workflows and the ongoing difficulties in guaranteeing that the high level of efficiency doesn't come at the expense of accuracy. In the ongoing development of more efficient AI-based solutions for video annotation, DataLoop represents one of the platforms exploring this balance.
DataLoop AI's Object Persistence Tracking feature claims to achieve a 95% label accuracy across video frames. This level of accuracy suggests a robust system that is capable of maintaining reliable object tracking throughout a video sequence, potentially useful in applications needing precise object tracking like self-driving cars or security systems. They've built in methods that ensure object identities stay consistent from one frame to the next, which is crucial when a continuous understanding of object motion is important. This is especially helpful in complex scenes where numerous things are happening at once.
The platform's ability to track multiple objects simultaneously adds value for applications dealing with crowded scenes or situations with many moving parts. It can gather a richer dataset that is better suited for various machine learning applications. They've also focused on making the platform work well with other machine learning tools and data processing frameworks, which could be useful for researchers who are transitioning between the annotation and the model training steps in their workflows. The platform supports real-time object tracking, which is a necessity in fast-paced environments. This feature might be useful for applications such as video surveillance where immediate decisions are critical.
Additionally, it appears that DataLoop has incorporated tools to detect and fix errors during the tracking process, using the history of past annotations to make smarter predictions. This error detection process could be valuable for preventing problems as long-term projects proceed. The system is designed to be easy for users to learn and use, which could make it suitable for a wider range of individuals involved in video annotation. It can handle large amounts of data efficiently, a requirement for industries like self-driving cars or real-time sports analytics. The platform also leverages historical data to improve annotation accuracy and feedback mechanisms, potentially contributing to more consistent annotation over time. Finally, it offers collaborative features that can help multiple annotators work together on the same video project. This might be valuable in projects with strict time constraints.
While the claimed 95% accuracy is noteworthy, it's always important to validate these claims through independent testing to ensure that this accuracy is consistently achieved across various datasets and under diverse circumstances. The specifics of how they achieve this high accuracy and how it translates into real-world applications should be carefully evaluated. However, if these claims are backed by empirical evidence, DataLoop's platform could become an increasingly popular solution within the video annotation space.
Analyze any video with AI. Uncover insights, transcripts, and more in seconds. (Get started for free)
More Posts from whatsinmy.video: