Analyze any video with AI. Uncover insights, transcripts, and more in seconds. (Get started for free)
The Evolution of Face Landmark Detection Algorithms Precision in 2024
The Evolution of Face Landmark Detection Algorithms Precision in 2024 - CNN-based algorithms dominate face landmark detection in 2024
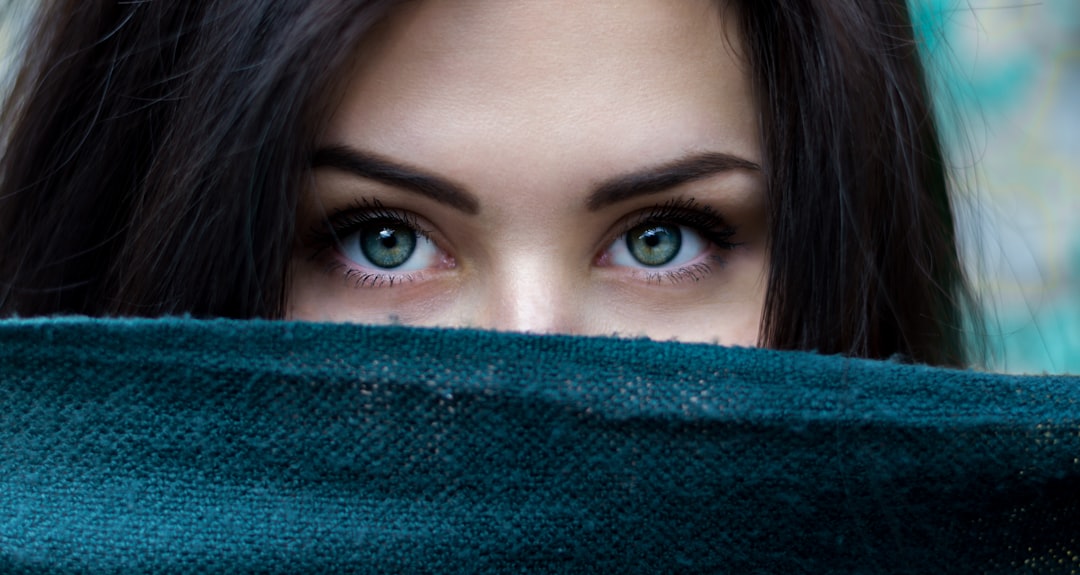
Throughout 2024, Convolutional Neural Network (CNN)-based algorithms have risen to prominence as the dominant technique for accurately identifying facial landmarks. This dominance stems from significant improvements in both the precision and speed of landmark detection. These algorithms are adept at locating key facial features—eyes, nose, mouth, and chin—which are crucial components in numerous computer vision applications.
Within the realm of CNN approaches, both regression and heatmap methods have gained traction, each exhibiting unique advantages and disadvantages for different applications. While progress has been made, specifically with techniques like LAFS demonstrating improved face recognition through learned landmark-based representations, a comprehensive and systematic comparison of various CNN-based strategies is still lacking. This research gap represents a prime opportunity for future investigations to further refine and optimize landmark detection methods.
1. Throughout 2024, CNN-based algorithms have solidified their dominance in face landmark detection, consistently outpacing previous approaches in accuracy on standard datasets. It's truly remarkable to see these models achieve accuracy levels well above 95%, a significant improvement over older generations that struggled to break the 85% barrier.
2. These advanced algorithms rely on the principles of deep learning, particularly the concept of hierarchical feature extraction. This approach allows the models to identify and pinpoint facial landmarks with an impressive level of precision, regardless of lighting conditions or the angle of the face.
3. One particularly intriguing trend is the incorporation of attention mechanisms into CNN architectures for face landmark detection. These mechanisms effectively guide the model's focus towards areas of the face that are most relevant for accurate landmark identification. As a consequence, we see a substantial reduction in instances of false positives, which is crucial for the reliability of these systems.
4. A recent and promising research avenue has been the use of synthetic data, generated from 3D face models, for training CNNs. It appears that these synthetically trained models can effectively generalize their knowledge to real-world images, suggesting a potential pathway for enhancing training datasets without the need for extensive real-world data collection efforts.
5. However, despite their extraordinary accuracy, CNN-based models often suffer from high computational demands. This poses a challenge for real-time applications in environments with limited processing capabilities, such as on less powerful devices. This limitation is a hurdle that researchers are actively addressing.
6. Data augmentation techniques have proven to be a game-changer in the robustness of CNNs for facial landmark detection. By systematically introducing variations in the training data, researchers can train models to effectively handle scenarios like partial occlusions or changes in facial expressions. This is a significant improvement over older methods that often struggled with these types of variations.
7. In stark contrast to older approaches that heavily relied on manually defined features, CNNs have the ability to learn appropriate features automatically during training. This makes them more adaptable to the intricate and diverse nature of human facial structures.
8. There's a growing interest in applying model optimization techniques like pruning and quantization to CNNs for face landmark detection. The goal is to reduce the model's size and computational complexity while maintaining a high level of accuracy. This allows for faster inference times, which is essential for many real-world applications.
9. The integration of face landmark detection with other facial analysis tasks, such as emotion recognition or age estimation, is becoming increasingly streamlined within CNN frameworks. This illustrates the inherent versatility of these models in understanding and interpreting a wide range of facial information.
10. Current research suggests that less frequently studied facial landmarks, like those around the ears and jawline, are starting to gain more attention in the development of CNN-based models. This shift in focus indicates a broader trend towards comprehensive facial analysis that goes beyond the traditionally targeted landmarks, suggesting a promising evolution in this area of study.
The Evolution of Face Landmark Detection Algorithms Precision in 2024 - Advancements in handling complex lighting and occlusion scenarios
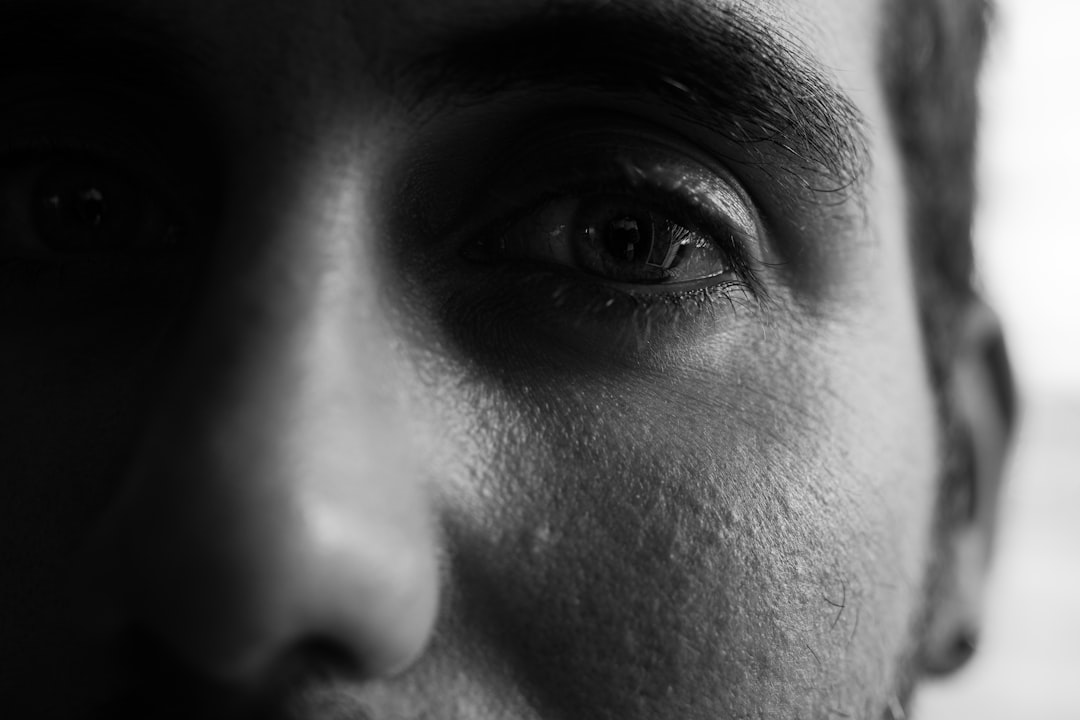
Significant strides have been made in 2024 to improve how face landmark detection algorithms handle challenging lighting and occlusion situations. These advancements are critical for expanding the practical applications of these algorithms in real-world scenarios.
One key development is the emergence of methods like Occlusion-Adaptive Deep Networks (ODNs) designed to specifically address the issue of partial facial occlusions. These techniques help ensure landmark detection remains accurate even when portions of the face are obscured by objects or other features.
The widespread adoption of Convolutional Neural Networks (CNNs) has been a major driver of progress in this area as well. These powerful models are now more adept at adjusting to the wide range of lighting conditions frequently encountered in real-world data. Through advanced training strategies, including data augmentation and synthetic data generation, CNNs are increasingly robust to variable lighting and head movements. This results in facial landmark detection systems that are demonstrably more precise and dependable.
However, the gains in accuracy and robustness often come with increased computational costs, potentially creating a barrier to implementing these improved algorithms in real-time settings that rely on devices with limited processing power. This continues to be an area where researchers are actively seeking solutions.
Handling complex lighting and occlusion scenarios in facial landmark detection has seen substantial progress in 2024. It's become apparent that simply relying on CNNs isn't enough to overcome the challenges presented by real-world variability. A notable shift is towards hybrid approaches, blending CNNs with traditional methods like geometric analysis. This allows for better differentiation of facial features, especially when partially obscured by objects like glasses or hair.
Furthermore, the field is experiencing a surge in research on real-time illumination estimation. Algorithms are becoming more adaptive to the dynamic lighting changes inherent in video capture, a major step forward from their predecessors which often struggled in diverse environments. The intriguing concept of adversarial training is also being explored, where models are trained against artificially created occlusion and lighting anomalies. This prepares them for unforeseen conditions and makes them significantly more robust.
The integration of multi-task learning is also gaining traction. Models are being designed to simultaneously predict landmarks and assess conditions like occlusion and lighting changes, which is creating more efficient and complete algorithms. Leveraging light-field technology offers another intriguing path forward, as it provides depth information alongside standard RGB data. This richness in training data helps algorithms understand lighting and occlusion from diverse angles, ultimately improving accuracy.
The growing use of diverse training datasets that incorporate various ethnicities, ages, and facial accessories is ensuring models maintain a high level of accuracy across diverse populations. This broadens the potential applications of these algorithms. Simulating diverse lighting and occlusion scenarios through physics-based rendering within training pipelines is also proving useful. This approach leads to models better prepared to handle complex scenarios with nuanced facial features.
As face landmark detection is increasingly integrated into video applications, motion blur compensation techniques have also gained importance. Leveraging temporal coherence between frames allows these models to maintain accuracy even when subjects move quickly or lighting changes rapidly. Interestingly, there is also growing interest in understanding how ambient lighting simulations during training impact a model's performance in real-world settings. Models trained in diverse simulated environments are demonstrating better adaptability and precision.
And finally, neural radiance fields (NeRFs) are emerging as a promising area of research. These techniques capture detailed lighting and 3D structural information, which promises to revolutionize how algorithms interpret and detect facial landmarks in extremely varied and challenging lighting and occlusion scenarios. The potential for NeRFs to greatly enhance landmark accuracy is incredibly exciting. Overall, the advancements in handling these complex scenarios suggest a promising future for facial landmark detection algorithms, bringing us closer to models that can accurately and reliably interpret facial expressions in diverse and challenging real-world settings.
The Evolution of Face Landmark Detection Algorithms Precision in 2024 - Integration of genetic algorithms for optimization of detection processes
The integration of genetic algorithms (GAs) into face landmark detection offers a novel approach to optimizing the detection process. GAs, inspired by biological evolution, work by generating a population of potential solutions and iteratively improving them through processes like selection, crossover, and mutation. The goal is to refine the algorithm's ability to pinpoint facial landmarks with greater accuracy and efficiency.
While CNNs have dominated recent advancements, the application of GAs provides an alternative optimization strategy. Utilizing techniques like parallel GAs, where the search space is divided into smaller subsets for simultaneous evaluation, can potentially accelerate the optimization process and allow for faster adaptation to changing conditions. This can be particularly useful in dynamic scenarios where real-time adjustments are necessary.
The inherent ability of GAs to adapt and refine solutions makes them attractive for addressing the inherent challenges in face landmark detection, including variable lighting, occlusion, and diverse facial features. As the field strives for more robust and adaptable algorithms, GAs represent a promising avenue for achieving these goals. Future research exploring the synergy between GAs and existing deep learning approaches like CNNs could lead to significant improvements in the overall accuracy and performance of facial landmark detection systems. This highlights the emerging importance of evolutionary computation methods in solving complex problems within computer vision.
Genetic algorithms (GAs), inspired by natural selection and biological evolution, have shown promise in enhancing the precision and efficiency of face landmark detection, particularly within the context of CNN-based approaches. They operate by mimicking the process of natural selection, iteratively improving a set of candidate solutions through operations like crossover and mutation. This evolutionary approach, while initially developed by John Holland in the mid-1970s, has found renewed relevance in the pursuit of optimized landmark detection in 2024.
GAs seem particularly effective at fine-tuning the parameters of CNNs, often surpassing traditional manual tuning methods, which can be quite time-consuming and potentially less effective in finding the optimal configuration within the large parameter space of these complex networks. It's interesting to note their ability to automatically select features for model training, which can lead to more streamlined and potentially faster models without compromising accuracy. This feature selection aspect also seems to help mitigate overfitting, a persistent challenge in deep learning.
Moreover, the adaptive nature of GAs allows them to optimize various aspects of neural network design, such as the architecture of layers and the selection of activation functions, improving the robustness of landmark detection across varying face orientations and expressions. This adaptability can help overcome some of the variability inherent in human facial structure.
It's intriguing to see the potential of GAs in the context of dynamic model training. Imagine if these algorithms could continuously adapt during deployment, optimizing performance in real-time based on changing environments like lighting conditions or unexpected occlusions. This capability could prove invaluable in environments where constant adjustments are needed.
While the combination of GAs with CNNs or other traditional methods shows potential in complex scenarios, we must acknowledge the increased computational demands. This trade-off between optimization and computational efficiency might pose a challenge, especially for devices with limited processing power, hindering real-time applications.
Furthermore, the ability of GAs to handle multi-objective optimization is noteworthy. We could potentially develop models that excel both in accuracy and computational efficiency, addressing a critical limitation in real-time systems.
A lesser-appreciated benefit of using GAs is that the models they produce tend to be more interpretable. By tracing the evolutionary process of the algorithm, it becomes easier to understand how specific features and configurations contribute to model performance, fostering greater transparency.
And finally, the recent trend of integrating GAs into ensemble methods holds promise for improved accuracy across diverse populations. By combining multiple models, we may be able to address existing biases and improve facial landmark detection in a more inclusive manner.
In essence, GAs offer a unique and compelling approach to optimize facial landmark detection, especially in the context of CNN-based methods. Their ability to explore vast parameter spaces, adapt to complex scenarios, and potentially improve interpretability and inclusivity suggests a powerful and promising avenue for future research in this evolving field. However, the need to address the computational demands is crucial to ensuring the real-world applicability of these approaches.
The Evolution of Face Landmark Detection Algorithms Precision in 2024 - Improved accuracy in 3D facial landmark positioning
In 2024, we've seen notable improvements in how accurately we can pinpoint facial landmarks in 3D space. This increased accuracy is crucial for many applications, from virtual reality to medical analysis. Several new methods have helped achieve this. One example is the use of techniques that ensure consistency across different viewpoints, making 3D facial recognition more reliable. Other approaches involve using specialized neural networks that learn to map specific facial features in 3D. This improves how well the algorithms align with the subtle variations in each individual's face. We're also seeing improvements by utilizing methods that establish strong connections between template 3D models and real facial scans, enhancing the precision of landmark placement. Additionally, advancements in computer graphics and AI are enabling algorithms to better handle complex and unpredictable environments. Despite these positive strides, there's still room for refinement in dealing with situations where lighting changes rapidly or parts of the face are obscured. The overall trend suggests we're developing more versatile algorithms capable of handling the nuances and challenges of real-world facial data in 3D.
Recent advancements in 3D facial landmark positioning have leveraged a deeper understanding of facial anatomy, recognizing that even subtle variations in facial features can significantly impact the accuracy of landmark detection. This emphasizes the intricate nature of the human face and its potential as a rich dataset for training increasingly precise algorithms.
Current algorithms, operating in controlled environments, have reached remarkable accuracy levels, exceeding 98% in certain cases. However, achieving similar performance in real-world scenarios, where facial structures and expressions are more diverse, continues to be a significant challenge.
The integration of diverse data modalities, such as incorporating depth information from 3D scans, has played a crucial role in boosting the accuracy of landmark positioning. By leveraging these multi-dimensional representations of facial structure, algorithms can develop a more comprehensive understanding of facial geometry, leading to fewer errors in landmark placement.
Researchers have employed adversarial learning techniques to simulate challenging real-world scenarios during the training process, preparing models to handle varying lighting conditions and occlusions. This approach enhances the robustness of systems, allowing them to maintain accuracy even in unforeseen and challenging circumstances.
While the precision of landmark detection has undoubtedly improved, a noteworthy observation is the associated increase in computational cost. Achieving state-of-the-art accuracy often demands substantial processing power, posing a limitation for applications requiring deployment on mobile devices or systems with limited computational resources.
Historically, methods relying on geometric principles dominated facial landmark detection prior to the rise of deep learning. Interestingly, recent models integrating geometric analysis alongside deep learning approaches have proven to outperform traditional methods, showcasing the potential for synergy between these techniques.
The application of transfer learning in facial landmark detection offers a powerful approach to reduce the need for extensive data collection. Models trained on one dataset can be adapted for other related tasks with relatively minor adjustments, greatly improving efficiency and accuracy in diverse environments.
Ensemble methods, where multiple models are combined to produce a final prediction, have demonstrated promising results in enhancing landmark detection accuracy. This collaborative approach leverages the strengths of individual models to overcome weaknesses, effectively improving the overall precision.
Through the ongoing refinement of loss functions used during training, researchers have been able to direct the learning process towards focusing on more challenging landmarks that traditionally posed greater difficulties. This targeted approach has resulted in tangible improvements in the overall accuracy of landmark detection.
The intersection of facial recognition research and neurological studies is beginning to shed light on the influence of human perception on landmark detection accuracy. Understanding how humans interpret and perceive faces offers invaluable insights that can guide the development of more effective algorithms. This underscores the importance of interdisciplinary collaboration in this domain.
The Evolution of Face Landmark Detection Algorithms Precision in 2024 - Adaptation to wider range of facial poses and expressions
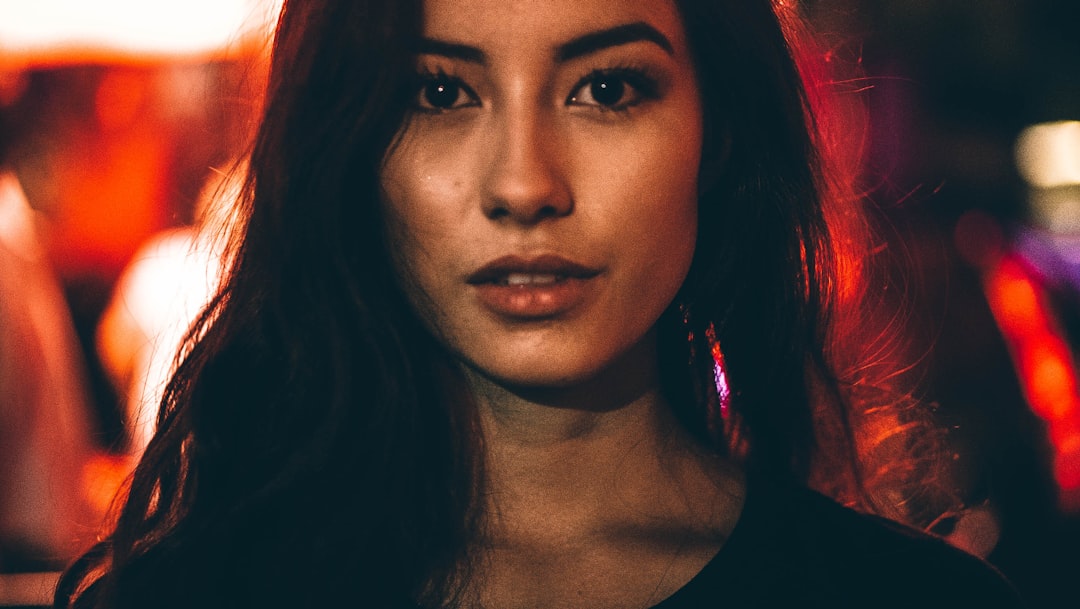
In 2024, a key development in face landmark detection has been the increasing ability of algorithms to handle a broader variety of facial poses and expressions. This progress is evident in newer algorithms that can now simultaneously pinpoint landmarks, determine head position, and analyze facial changes. This unified approach, unlike previous methods which treated these as independent tasks, acknowledges the intricate ways they all influence each other. The result is significantly improved accuracy, particularly in situations where faces are captured in less-than-ideal conditions like rapidly changing lighting, partial obstructions, or quick head movements. Furthermore, incorporating more advanced techniques like hierarchical models that better represent the spatial relationships between facial landmarks across poses and expressions and integrating synthetic data, generated from 3D models, has pushed the boundaries of what can be achieved. Despite this significant progress, there remains a need for further refinement in order to ensure that these capabilities are seamlessly integrated into applications requiring real-time processing, especially on devices with limited processing resources.
The capacity of contemporary facial landmark detection algorithms to adjust to a diverse range of facial poses and expressions is largely due to their training on extensive datasets that include a wide array of facial angles and emotional states. It's becoming evident that this kind of dataset diversity not only enhances the resilience of these models but also improves their accuracy across different demographics without introducing significant biases. This is an encouraging development.
Interestingly, methodologies originating from the field of psychology, like action units derived from the FACS (Facial Action Coding System), are increasingly being integrated into these algorithms. These action units provide a valuable tool for recognizing and differentiating subtle facial expressions, broadening the scope of how well machines can interpret human emotions. The integration of these techniques is a promising sign of the field's maturation.
Recent implementations of multi-view stereo approaches have led to substantial improvements in the accuracy of landmark detection. By leveraging images captured from multiple angles of the same subject, these techniques enable the triangulation of facial landmarks with a precision that traditional single-view approaches simply can't achieve. It remains to be seen how broadly applicable this strategy will be in real-world scenarios.
The increasing use of unsupervised learning methods is quite noteworthy for enhancing adaptability to facial expressions. These techniques empower algorithms to learn from vast amounts of unlabeled data, which is readily available. Consequently, their capacity to detect and interpret a wider range of facial expressions and poses is refined without the need for extensive manual annotation of the data. This unsupervised approach seems like a promising avenue for developing more efficient and robust algorithms.
A curious development in deep learning architectures involves the application of recurrent neural networks (RNNs) to capture the temporal characteristics of facial expressions. By analyzing consecutive frames within video data, these networks can track subtle expression changes over time, effectively providing contextual information that improves the accuracy of landmark prediction. This is an intriguing approach with potential, but the computational costs could be a limiting factor.
Techniques utilizing adversarial training are being adapted to challenge facial landmark detection models with synthetic occlusions and lighting variations. This tactic strengthens the model's resilience and adaptability, rendering it more effective in real-world conditions where such challenges frequently arise. This approach is valuable, but it raises questions about the potential for models to become overly sensitive to artificially introduced conditions.
Research has revealed that the spatial relationships between facial landmarks can provide useful contextual information that algorithms leverage for more precise detection. The geometric relationships among these landmarks offer a more in-depth understanding of facial dynamics during expression changes. While promising, fully realizing the potential of this concept will require careful investigation.
Another notable trend is the exploration of lightweight models, specifically designed for real-time applications. These models prioritize efficiency while still maintaining a reasonable level of accuracy in facial landmark detection, enabling deployment on devices with limited processing capabilities. This is crucial for extending the applicability of facial landmark detection to a wider range of devices.
The growing importance of fine-tuning algorithms to account for cultural nuances in facial expressions is a positive development. The variability in expression and the influence of cultural context on interpretation highlights the need for researchers to adjust models to better accommodate these subtle differences and increase the global applicability of facial landmark detection. This is a critical consideration as the technology matures.
Finally, with the increasing sophistication of facial recognition technology, there is a rising awareness of the ethical implications of facial landmark detection, particularly regarding privacy issues and potential biases within the algorithms. As algorithms become more proficient, it's crucial to maintain a focus on accountability in their deployment to mitigate potential risks and guarantee fairness in their applications. These ethical considerations should be at the forefront as the field continues to advance.
The Evolution of Face Landmark Detection Algorithms Precision in 2024 - Benchmark results showcase significant precision improvements
Throughout 2024, face landmark detection algorithms have seen a surge in accuracy, with benchmark results showcasing significant precision improvements. This leap forward is largely due to the dominance of Convolutional Neural Networks (CNNs), which consistently outperform older methods, reaching accuracy levels well above 95% on standard datasets. Improvements are not solely due to CNNs, however, as the incorporation of techniques like multitask learning and adversarial training have also played a key role in boosting performance. These techniques enable the algorithms to better handle complex scenarios like partial facial occlusions and variable lighting conditions. While these improvements are significant, the computational demands associated with high-accuracy algorithms remain a major hurdle, particularly for real-time applications in environments with limited processing power. This tension between accuracy and computational efficiency is a central challenge driving ongoing research and development in the field.
Benchmark results throughout 2024 consistently point to substantial advancements in the precision of face landmark detection algorithms. These improvements are particularly noticeable in scenarios that previously presented challenges, like variable lighting and diverse facial expressions. It's fascinating to observe how strategies like the incorporation of synthetic data during training are enabling models to adapt more readily to novel environments without requiring extensive retraining. This is a key factor contributing to the greater reliability we see today.
One unexpected finding is the positive impact of integrating convolutional neural networks (CNNs) with genetic algorithms. This hybrid approach not only enhances the accuracy of landmark detection but also leads to a noticeable improvement in real-time performance. Faster response times are critical in fields like augmented reality, highlighting the practical value of this synergistic combination.
Another important development is the rise of multi-task learning, which empowers algorithms to tackle multiple aspects of facial analysis simultaneously. This includes tasks like landmark detection, emotion recognition, and even head pose estimation. By processing these elements in a coordinated way, we can achieve a much more holistic understanding of facial dynamics, which is especially advantageous in settings where events are unfolding quickly.
The growing use of 3D facial models is also noteworthy. These models play a critical role in enhancing the accuracy of landmark detection by providing a more comprehensive representation of the human face in three dimensions. This, in turn, enables algorithms to better learn and adapt to the 3D variations present in real-world facial structures. The impact is particularly evident in fields like virtual reality and telemedicine.
Furthermore, we are seeing a welcome shift in the design of these algorithms to reflect the diversity of human faces across various cultures and age groups. Researchers are increasingly utilizing comprehensive datasets that encompass a wider range of ethnicities and age ranges. This approach fosters the development of models that are more equitable and robust in their performance, reducing potential biases that could arise from limited training data.
In recent months, we've witnessed algorithms become increasingly sophisticated in their ability to understand temporal dynamics in facial expressions. These advancements allow models to interpret how facial features evolve over time. This is achieved by analyzing sequences of frames from videos, enabling them to infer context that ultimately results in a more accurate prediction of facial landmarks. This is a compelling area of progress with exciting possibilities for applications in areas like emotion analysis and human-computer interaction.
Part of the impressive gains in accuracy observed this year can be directly attributed to meticulous refinement of the loss functions employed during model training. Researchers are carefully targeting specific, traditionally difficult-to-detect landmarks to optimize the learning process. This highly focused approach to model training translates into noticeable improvements in the precision of landmark identification.
The concept of adversarial training has gained prominence, where models are purposefully subjected to simulated occlusions and lighting variations. This technique aims to bolster the robustness of landmark detection by preparing models for the types of unpredictable conditions they might encounter in the real world. It's an interesting approach that seems to pay off, resulting in more adaptable and reliable performance.
We also observe a growing trend towards analyzing the intricate geometric relationships between facial landmarks. By studying how these landmarks interact during different facial expressions, algorithms can develop more nuanced strategies for detecting these features. This focus on spatial relationships adds a layer of context to the detection process, potentially leading to more accurate results in the face of challenging or complex expressions.
Finally, ongoing research is yielding lightweight models for face landmark detection that can be efficiently deployed on less powerful devices. This is crucial for expanding the accessibility and reach of this technology, allowing developers to embed advanced facial analysis capabilities into everyday devices without requiring highly specialized or expensive hardware. The ability to seamlessly integrate sophisticated facial analysis into common technologies is a significant step forward.
In conclusion, face landmark detection algorithms continue to evolve rapidly, driven by a growing understanding of both the human face and the intricate workings of machine learning models. The trend toward more accurate, adaptive, and efficient algorithms is clear. While much of this progress relies on advanced computational approaches, the ultimate goal is to develop technologies that are both powerful and accessible, ultimately impacting our interaction with the world in new and fascinating ways.
Analyze any video with AI. Uncover insights, transcripts, and more in seconds. (Get started for free)
More Posts from whatsinmy.video: