Analyze any video with AI. Uncover insights, transcripts, and more in seconds. (Get started for free)
The Current State of Content Moderation in AI Image Generation 7 Key Concerns for 2024
The Current State of Content Moderation in AI Image Generation 7 Key Concerns for 2024 - Deepfake Political Ads Expose Gaps in Current Detection Systems
The increasing use of deepfake technology in political advertising poses a significant challenge to existing content moderation systems. Examples from countries like Turkey and India show how these fabricated videos can muddle political discussions, hindering the public's ability to discern true from manipulated content. While advancements in machine learning and other detection techniques have emerged, significant gaps in identification capabilities remain, allowing certain deepfakes to bypass detection measures. Efforts to mitigate this issue through legislation, such as measures focused on protecting elections from AI-generated deception, are underway. However, the potential conflict with free speech protections presents a complex regulatory hurdle. As we near the 2024 elections, the widespread use of deepfake political ads underscores the critical need for more sophisticated detection systems and a robust legal framework to counter this evolving threat to political discourse.
The increasing sophistication of deepfake technology has led to its use in crafting highly realistic political advertisements, posing a significant threat to the integrity of political discourse. While initially developed for creative purposes, deepfakes now allow for the convincing imitation of real candidates, challenging the capabilities of current detection systems.
Studies have revealed a range of accuracy in deepfake detection systems, with some achieving only modest success rates, particularly against more advanced deepfakes. This suggests a potential vulnerability, as the technology behind creating these manipulated videos is becoming increasingly efficient. Some methods can generate deepfakes with remarkably limited data, enabling a quick and widespread distribution of deceptive content.
Furthermore, research indicates that deepfake political content might have a more profound impact on public opinion than traditional forms of misinformation. This suggests that audiences may be more susceptible to deepfakes, even if aware of their potential to be manipulated.
Deepfake detection often hinges upon the identification of minute visual or audio anomalies. However, the creators of deepfakes are countering this by incorporating techniques that obfuscate these telltale signs, making detection significantly more challenging. Coupled with the lack of widespread public awareness about deepfakes, this situation presents a serious threat. Surveys show a significant segment of the populace is either unaware of deepfakes or their implications for political campaigns, underscoring a crucial need for public education.
The ethical ramifications of using deepfakes in politics are considerable, particularly concerning the rights of individuals whose likeness may be utilized without their knowledge or consent. Legal and ethical ambiguities emerge regarding the accountability of those responsible for creating and distributing such content, especially in places where legal frameworks for synthetic media in politics are absent or weak.
The ongoing evolution of generative AI models, particularly GANs, fuels concerns about the growing sophistication of deepfake technology. The output of these models is becoming increasingly difficult to differentiate from genuine footage, even for individuals with experience in the field. As the technology continues to develop and spread, there is rising concern amongst policy makers and experts regarding its potential to undermine democratic processes and the public's faith in the integrity of elections.
The Current State of Content Moderation in AI Image Generation 7 Key Concerns for 2024 - AI Image Generators Still Unable to Block NSFW Content Creation
AI image generators, despite incorporating filters designed to prevent the creation of NSFW content, are still vulnerable to manipulation. Users have discovered ways to circumvent these safeguards by crafting cleverly worded prompts that trick the models into producing inappropriate imagery. This includes popular systems like DALLE 2, which can interpret unconventional inputs as requests for specific, potentially explicit, content. This issue is particularly concerning given the growing popularity of high-resolution image generators like Stable Diffusion, which boasts millions of users and has encountered difficulties in consistently blocking NSFW content. While developers are striving to enhance the effectiveness of safety measures, the limitations in current filtering technologies continue to raise concerns about the appropriate balance between user freedom and the necessity of protecting online users from potentially harmful content. Moving forward, achieving a harmonious blend of creative expression and content safety in the field of AI image generation will likely remain a persistent challenge.
Despite the ongoing development of sophisticated AI image generators, preventing the creation of NSFW content remains a persistent challenge. This is largely due to the inherent difficulty in accurately interpreting the context of user prompts. Distinguishing between artistic depictions and explicit content proves particularly complex for the algorithms currently employed.
Research indicates that users are finding ways to circumvent the filters designed to block NSFW material. By using obscure or nonsensical phrasing, they're able to manipulate the AI into generating images they might not otherwise create. This cat-and-mouse game between users and developers makes it difficult to establish robust content moderation.
The limitations of existing content filtering systems are further highlighted by their tendency to generate false positives. A benign image might be mistakenly flagged as NSFW, leading to a frustrating user experience and potentially discouraging the use of stricter content controls.
The quality of the training data used to develop these AI models has a significant impact on their ability to identify NSFW content. Many datasets used for training lack sufficient representation of diverse contexts related to human sexuality, leading to biased outcomes. This can manifest in a failure to identify explicit content or, conversely, in over-sensitivity, incorrectly flagging innocuous content.
Techniques like transfer learning, where models are repurposed for different tasks, haven't proven universally effective in eliminating NSFW content. The underlying challenge is in getting the model to reliably distinguish between explicit content and artistic expression.
There's a growing tension between the desire of users to employ AI for artistic endeavors and the need for safe online environments. Many artists and content creators are voicing frustration with the current limitations of moderation systems, particularly when dealing with subtle or artistic depictions. This conflict highlights a broader debate within the community concerning artistic freedom and responsible content creation.
Moreover, some AI image generators appear to exhibit biases that reflect societal stereotypes surrounding gender and sexuality. This introduces concerns about potential systemic issues in content moderation that can lead to skewed outputs.
Users continue to exploit loopholes in the current systems by creatively manipulating prompts. This ability to circumvent existing safety protocols underscores the challenges in effectively mitigating the generation of NSFW content.
As AI models continue to improve, so does the potential for misuse. The constant evolution of the technology necessitates an equally adaptive approach to content moderation. The ongoing struggle between those seeking to generate specific content and developers attempting to maintain safety standards continues to raise concerns about the future of online content.
Finally, legal frameworks surrounding AI-generated content are still evolving. The absence of clear guidelines regarding responsibility for NSFW outputs adds to the difficulty in implementing effective moderation practices. The lack of industry-wide standards makes it challenging to establish clear accountability, which ultimately hinders progress in developing comprehensive user protection measures.
The Current State of Content Moderation in AI Image Generation 7 Key Concerns for 2024 - Watermarking Solutions Fall Short as Fakes Flood Social Media
The surge of AI-generated content, particularly fakes, flooding social media platforms has exposed the limitations of current watermarking solutions as a means of content moderation. While initially presented as a promising approach to identify AI-created content, these watermarking techniques have proven susceptible to circumvention, leading to doubts among experts about their effectiveness. Major social media platforms haven't implemented strict enforcement of labeling for AI-generated or edited content, further hindering efforts to distinguish real content from fabricated images. Government initiatives, like the US government's push to adopt digital watermarking for combating misinformation, particularly during elections, acknowledge the growing concern. However, whether such measures will effectively address the issue remains uncertain. The ever-increasing sophistication of deepfake technology, coupled with the ethical and security challenges it poses, further complicates the situation and outpaces the current capabilities of watermarking to address them. The challenge of separating authentic from manipulated content in this rapidly evolving landscape appears to be a significant obstacle for online platforms and government agencies alike.
While watermarking has been proposed as a way to identify AI-generated content, its effectiveness is facing significant challenges in the current landscape of online content. Research indicates that traditional watermarking techniques are often easily bypassed by readily available image editing tools. Many content creators themselves express doubt about the effectiveness of watermarks, particularly against advanced techniques like deepfakes. This skepticism highlights a growing disconnect between the perceived protective capabilities of watermarks and their actual performance.
Furthermore, many watermarking methods introduce compromises, such as a noticeable reduction in image quality. This trade-off between content protection and aesthetic appeal creates a dilemma for content creators. The constant advancement of AI image generation tools further complicates the issue, as watermarks that were once effective can become easily bypassed with newer algorithms. This continuous arms race between watermarking techniques and image manipulation methods makes achieving reliable protection difficult.
The effectiveness of watermarking is also influenced by user behavior. Many individuals have learned how to effectively remove or replicate watermarks, which undermines their intended purpose. Coupled with a lack of awareness regarding content theft and the risks associated with sharing unwatermarked content, many creators unintentionally put their work at risk.
Current watermarking solutions also face challenges in standardization and interoperability across platforms. A watermark that is recognized on one social media platform may not be detected or enforced on others, leading to inconsistencies in protection. This fragmented approach diminishes the overall value of watermarking as a preventative measure.
The consequences of ineffective watermarking extend to the economic viability of creative endeavors. Studies have revealed significant financial losses for creators due to untracked reproductions of their work online. This issue has sparked interest in exploring alternative approaches, such as blockchain technology, which could potentially provide a more tamper-proof method of establishing ownership and tracking content provenance.
The ongoing evolution of AI image generation necessitates a more robust and adaptable approach to content protection. While watermarking offers a starting point, its limitations necessitate a deeper examination of complimentary methods to address the challenges posed by sophisticated deepfakes and AI-generated misinformation. The future of content protection likely relies on a multifaceted strategy that combines technical innovation with user education and a thoughtful consideration of the legal and ethical complexities of digital ownership.
The Current State of Content Moderation in AI Image Generation 7 Key Concerns for 2024 - Data Protection Laws Struggle with AI Generated Celebrity Images
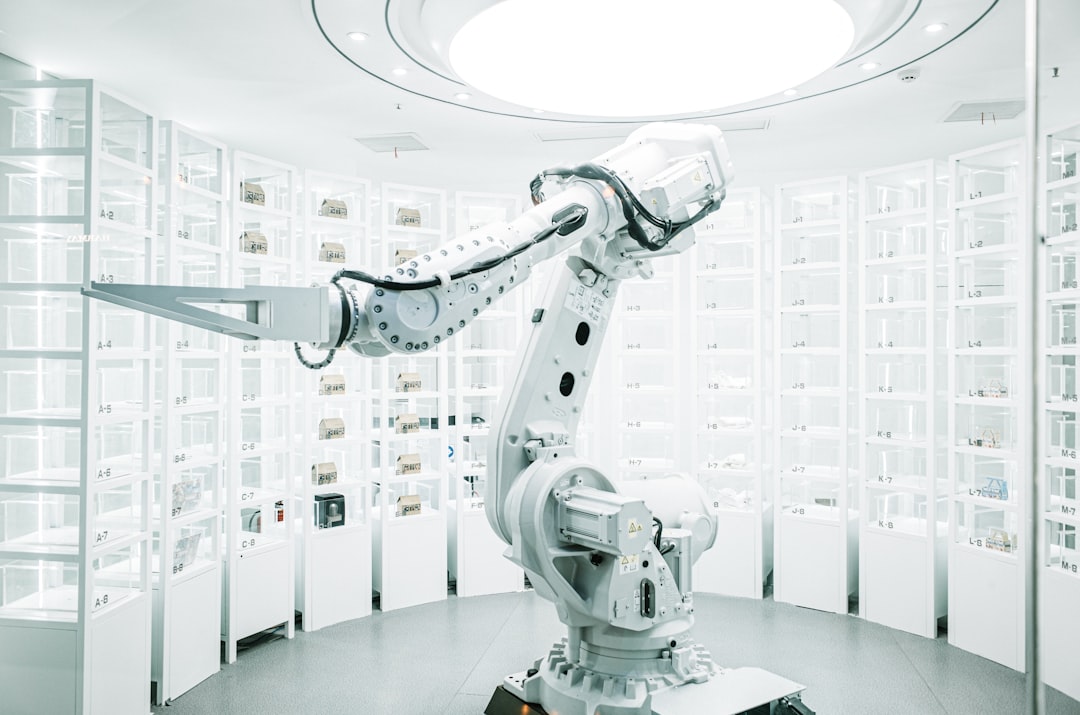
The rise of AI-generated images, especially those depicting celebrities, has thrust data protection laws into uncharted territory. Existing laws, both in the US and Europe, are struggling to adapt to the rapid advancements in AI technology. This is particularly evident in areas related to data ownership, individual privacy, and the ethical boundaries surrounding the unauthorized use of someone's likeness in AI-generated content.
Celebrities are facing a growing concern: the potential for widespread misuse of their images through AI-generated content. Legal frameworks are ill-equipped to address the unique challenges arising from the ability to create realistic, AI-generated images that mirror a person's appearance without their permission. The question of balancing innovation in the realm of AI with the protection of individual rights, especially regarding privacy and the control of one's image, is a complex one.
The current legal landscape often fails to provide a clear path for addressing these issues. This is leading to increased calls for re-examining and updating existing regulations to account for the unique characteristics of AI-generated content and the need to safeguard individual rights in the digital age. A robust and flexible legal structure will be critical for ensuring that the innovative potential of AI coexists with the necessary safeguards for people to protect their identity and image in the digital realm.
The rise of AI-generated images, especially those depicting celebrities, presents a significant challenge to existing data protection laws. The legal landscape surrounding the right of publicity, which governs the use of someone's likeness for commercial purposes, is uneven across different regions. While some places provide strong protections, others have less defined legal frameworks, leaving celebrities potentially vulnerable to the unauthorized use of their image.
Courts are starting to grapple with how consent applies to AI-generated images, considering not just the initial individual's permission but also the context and intentions behind the AI's creation. This evolving area could fuel increased lawsuits as various parties test the boundaries of copyright and privacy rights in this new digital domain.
Beyond the legal ramifications, AI-generated celebrity images can also impact individuals' mental and emotional well-being and public perception. The creation of unrealistic portrayals can lead to issues like anxiety and body image concerns amongst those who see them, especially among fans who may struggle to distinguish between reality and AI-generated content.
Many current data protection laws weren't built to handle the complex nature of AI-generated content, resulting in legal systems that struggle to adapt to the rapid pace of technological development. This disconnect often leads to uncertainty and challenges in enforcing rights associated with digital images.
From an ethical standpoint, there are significant concerns about the potential for misrepresentation of celebrities through AI. AI-generated images could damage a celebrity's reputation and impact their careers, leading to discussions about whether the AI creators should be held accountable for misleading portrayals.
Content moderation systems tasked with scrutinizing AI-generated celebrity images face a tough job. They need to identify likenesses while also understanding the difference between artistic interpretation and unauthorized duplication, creating a complex challenge for building reliable detection methods.
There's a worrying trend of AI tools amplifying the most sensational and often negative aspects of celebrity culture. This can inadvertently perpetuate harmful stereotypes and promote divisive narratives, which can unintentionally shape cultural perceptions in negative ways.
The explosion of AI-generated celebrity content on social media has made it challenging to differentiate between authentic and manufactured interactions. Research suggests that audiences frequently find it hard to distinguish between the two, which can skew public discussions surrounding a celebrity's actions and views.
The absence of a universally agreed-upon set of international standards for AI-generated content is causing concerns about potential jurisdictional clashes. A celebrity's rights may be upheld in one country but disregarded in another, complicating matters for the global nature of digital platforms.
As the legal battles surrounding AI-generated imagery become more frequent, new technologies for validating digital assets are gaining attention. Blockchain and similar technologies have the potential to improve the tracking of ownership and consent, representing a possible future solution to address the concerns around celebrity image exploitation.
The Current State of Content Moderation in AI Image Generation 7 Key Concerns for 2024 - Open Source Models Create New Content Moderation Challenges
The increasing availability of open-source AI image generation models presents a novel set of content moderation hurdles. These models, due to their open nature and adaptability, make it significantly harder to implement traditional content control measures. Users can now leverage these tools in increasingly creative ways, sometimes finding methods to bypass existing safety filters and generate content that might violate community guidelines or ethical standards. This puts developers in a difficult position, requiring them to consistently update and refine their approaches to content moderation. The ongoing back-and-forth between users finding loopholes and developers striving to close them highlights the evolving tension between creative freedom and the responsibility to ensure user safety. Therefore, navigating the complexities of content management in open-source environments while upholding ethical principles and safety protocols has become a prominent concern.
Open-source AI models, particularly those used for image generation, are increasingly challenging the landscape of content moderation. The rapid pace of development and the ease with which these models can be accessed and modified by anyone have created new avenues for generating harmful content, including misinformation and inappropriate imagery. This rapid proliferation poses a problem for moderation systems because they must adapt quickly to evolving methods of abuse, without the help of built-in safety features found in proprietary systems.
Another significant challenge is the lack of oversight in open source development. This means biases embedded within training data can easily be propagated through the generated content, potentially reinforcing existing stereotypes and harmful societal views. Furthermore, the culture of open source, which often emphasizes rapid innovation over meticulous testing, can lead to the release of models that haven't been adequately vetted for potential risks. Consequently, moderation systems may struggle to effectively address these evolving hazards.
The democratization of sophisticated image generation through open-source tools presents a fresh set of concerns about who is responsible for online content. Anyone with minimal technical expertise can now create complex content, which raises questions about the accountability for misuse or the creation of harmful content. Also unlike proprietary systems, many open-source models require users to set up their own safety protocols, leading to variations in how effectively content is screened. This lack of uniformity makes it harder for moderation efforts to be consistently effective.
Adding another layer of complexity is the emergence of decentralized platforms that support open-source models. Because no central authority controls these platforms or the tools, content moderation policies become significantly harder to enforce. A majority of users aren't even aware of how widely used these models are, and that makes it harder to identify AI generated content. This lack of familiarity with open-source models makes it challenging for users to discern real content from synthetic content, which may increase the spread of misinformation without anyone being aware.
While certain open-source models strive to include moderation capabilities, their open nature makes it relatively simple for users to disable or evade these safety measures. The moral implications of open source technology go beyond simple content moderation; discussions around the rights of content creators and the moral responsibility of developers are increasingly important, especially as these tools are used in ways that potentially violate privacy and misrepresent people. These are complex issues, and as these models become more commonplace it will become even more crucial to understand the wider implications of the technology.
The Current State of Content Moderation in AI Image Generation 7 Key Concerns for 2024 - Automated Filter Systems Show 47% False Positive Rate in Tests
Automated content filters, while intended to protect users, are showing a troubling 47% false positive rate during testing. This means that nearly half the time, these systems mistakenly flag harmless content as inappropriate or harmful. This high error rate is a major hurdle for developers striving to create effective content moderation. While there's increased interest in how AI language models can help moderate, research in this area is still underdeveloped, making it hard to fully grasp their potential or limitations. As the field of AI-generated content rapidly expands, the need for accurate and reliable content filters becomes increasingly urgent. This is further complicated by the constant ethical balancing act between user safety and protecting the freedom of expression. It's a challenging task for content moderation approaches to navigate this complex landscape effectively in the years to come.
1. **The Problem of Mistaken Identities:** Automated filtering systems designed to identify and block inappropriate content are surprisingly prone to error, mislabeling harmless images as offensive at a rate of 47% in recent tests. This high rate of "false positives" stems from the inherent limitations of the algorithms in accurately interpreting the nuances of visual content.
2. **User Frustration and Diminished Trust:** The constant barrage of false positives creates a frustrating user experience, constantly interrupting normal interactions with unwanted blocks. This can lead to a decline in trust for platforms that rely on these systems, impacting user engagement and potentially driving users to seek alternative platforms.
3. **Bias in the Machine:** Research suggests that automated moderation systems can exhibit bias, often mirroring the societal prejudices present in the data they were trained on. This can result in disproportionate flagging of certain types of content or demographics, highlighting potential issues of unfairness and discrimination.
4. **Limited Understanding of the World:** The datasets used to train these filters frequently lack the breadth and diversity needed to truly understand the subtle differences in human expression and cultural context. This gap in understanding can lead to a skewed perspective, with algorithms prone to misinterpreting certain types of images as inappropriate.
5. **The Clever User:** Users are continuously finding ways to outsmart these automated systems. By employing subtle variations in phrasing or cleverly worded prompts, they can manipulate the image generation process to produce content that bypasses filters intended to block it. This ongoing 'cat and mouse' game between users and developers underscores the challenges in creating robust content controls.
6. **The Cost of Accuracy:** Significantly reducing the false positive rate in these systems often demands significant investment in both sophisticated algorithms and high-quality training datasets. This creates a trade-off for developers, who must weigh the expense of improving accuracy against other priorities.
7. **Context is King, but the Algorithm is Confused:** These filters struggle to adapt to context. An image perfectly acceptable in one setting might be flagged as offensive in another, simply because the algorithm isn't capable of understanding the complexities of the situation. This highlights the need for systems that can more effectively analyze the surrounding context of an image.
8. **The Regulatory Shadow:** Persistent high rates of false positives could lead to increased pressure for greater regulatory oversight of content moderation systems. This regulatory scrutiny could inadvertently hinder the development and adoption of new technologies in content moderation, as companies face pressure to demonstrate the effectiveness of their algorithms.
9. **Beyond Still Images:** As content evolves beyond simple static images and incorporates more complex media like videos and interactive elements, automated filtering becomes considerably more challenging. The algorithms that perform well on static imagery may not generalize effectively to these more complex formats.
10. **When Rules Don't Match Reality:** High false positive rates may be an indication that the community guidelines these automated systems are designed to enforce aren't fully aligned with user expectations. Frequent misclassification can erode confidence in both the guidelines and the automated enforcement systems themselves, prompting questions about their legitimacy.
The Current State of Content Moderation in AI Image Generation 7 Key Concerns for 2024 - Content Authentication Methods Need Major Updates by Q2 2024
By the second quarter of 2024, the methods we use to verify the authenticity of content will need significant improvements. AI-generated content is rapidly becoming more realistic, making it harder to tell real from fake. Current methods of verifying content, like using encryption or having people check it, just aren't good enough at stopping the spread of false information and disinformation, especially with the rise of sophisticated deepfakes. Governments are starting to take action, trying to create rules and best practices for spotting AI-generated content. However, the constant evolution of this technology and the difficulty of keeping up with it means that we need stronger tools to ensure we know where content comes from and who created it. Without these updates, we risk losing trust in online media and the ability to discern what's true and what isn't.
By the second quarter of 2024, we're likely to see significant shifts in how we authenticate content. The current methods, particularly watermarking, haven't been as effective as initially hoped. Clever users are able to find ways around them, especially with the rise of readily available open-source tools. This means we need new strategies if we want to reliably pinpoint AI-generated content.
It's also become increasingly clear that our automated content filters have a lot of blind spots. They're prone to mistakenly flagging innocent content as harmful, which can be frustrating and erode people's trust. This high rate of "false positives", sometimes as high as 47%, reveals fundamental limitations in how these systems understand the intricacies of visuals and their context.
Another troubling trend is how existing biases in training datasets creep into the filtering process. This can result in some content or groups of people being disproportionately targeted, raising serious questions about fairness and potentially amplifying existing societal biases.
People are also quick to learn the tricks to manipulate systems, whether by cleverly wording their requests to image generators or by finding loopholes in the filters. This constant game of cat and mouse makes maintaining effective moderation a real challenge.
And the legal landscape is still far behind the technology. We need to better define the rules around using AI-generated content, especially when it involves someone's likeness without their consent. This is particularly urgent given the rise of AI-generated celebrity images. Current data protection laws are often inconsistent or outdated, especially across different countries, so we're seeing a fragmented approach to protecting people's rights.
Beyond legal hurdles, there's also the matter of economic damage to content creators. Inefficient moderation systems are leading to financial losses due to people copying and sharing work without permission. We desperately need more sophisticated ways to track and control content ownership.
Adding to the complexity is the rise of decentralized platforms that leverage open-source tools. There isn't a central authority to oversee things, which makes it incredibly difficult to establish consistent content moderation practices. The fact that many users aren't fully aware of just how widely these open-source models are used further complicates the situation.
All these concerns show that we urgently need to re-evaluate our content moderation approaches. The landscape of AI-generated content is shifting rapidly, and we need flexible and adaptable solutions to navigate the ethical and practical challenges that come with it. It's a tough balance between protecting people and preserving the freedom of creative expression, and it will continue to be a challenge in the coming years.
Analyze any video with AI. Uncover insights, transcripts, and more in seconds. (Get started for free)
More Posts from whatsinmy.video: