The Impact of Mean vs
Median Imputation on Video Analytics A Data-Driven Comparison
The Impact of Mean vs
Median Imputation on Video Analytics A Data-Driven Comparison - Understanding Mean vs Median Imputation in Video Analytics
When working with video analytics, understanding the differences between mean and median imputation becomes critical, especially when dealing with missing data points. Replacing missing values with the average (mean imputation) can be a simple solution but can distort the data's true picture if the data is skewed or has extreme values (outliers). The mean can be pulled in a misleading direction by these outliers, potentially affecting the accuracy of the analysis.
On the other hand, using the median (median imputation) is often more robust, as it's less sensitive to extreme data points. This approach helps preserve the original data distribution and avoids misrepresenting the data, especially in large or complex datasets. Consequently, choosing the appropriate method can heavily influence your analysis, underscoring the importance of considering dataset characteristics before implementation.
While mean and median are fundamental imputation methods, it's valuable to realize they aren't the only options. Exploring other techniques like K-Nearest Neighbors (KNN) or predictive methods can sometimes lead to superior results, especially for specific situations. Recognizing the limitations of basic approaches and understanding the diversity of available solutions is vital for robust and reliable video analytics.
When dealing with missing data in video analytics, understanding the nuances of mean versus median imputation is crucial. Replacing missing values with the average (mean) can distort the data, particularly when outliers are present, leading to results that don't accurately portray the true dataset characteristics. This is especially problematic for metrics derived from video analytics, which can be sensitive to such distortions.
Conversely, median imputation, which replaces missing values with the middle value, offers more resilience against the influence of extreme values. This makes it a compelling choice for video data, where anomalies like camera malfunctions or abrupt scene transitions might produce outliers. In complex video datasets, characterized by numerous features, the mean imputation approach might suppress variance and erase valuable dataset traits, negatively impacting model performance.
Moreover, the choice between these imputation methods influences how machine learning models interpret video data. Median imputation, in many instances, produces results that align better with human intuition, improving model interpretability. The assumption of a normal distribution inherent to mean imputation is often not met in practical video analytics contexts. Real-world video data frequently displays skewed or kurtotic distributions, rendering mean imputation less effective in such cases.
While mean imputation is computationally simpler, its potential for introducing skewed insights might necessitate extra effort for correction and validation later on. This negates the initial time saved and can complicate the overall analytical workflow. For video analytics that leverages time-series data, median imputation stands out due to its ability to better preserve the temporal integrity of the dataset. Mean imputation can distort trends and patterns within the data, impacting the reliability of time-dependent analysis.
Researchers have observed that employing median imputation can enhance the accuracy of predictive models designed for video analytics, especially when dealing with extensive missing data. The ramifications of imputation techniques extend beyond model performance, influencing decision-making processes related to content suggestions and user interaction strategies. By shaping the data foundation, the chosen imputation technique indirectly influences the quality of derived insights and the decisions built upon them.
Interestingly, studies have indicated that combining mean and median imputation techniques can, in some cases, improve results. This hybrid approach suggests that a more nuanced treatment of missing data in complex video datasets might yield superior outcomes compared to relying on a single method. This area of research continues to evolve as scientists and engineers seek to optimize data imputation for enhanced video analytics.
The Impact of Mean vs
Median Imputation on Video Analytics A Data-Driven Comparison - Data Collection Methods for whatsinmy.video Analytics
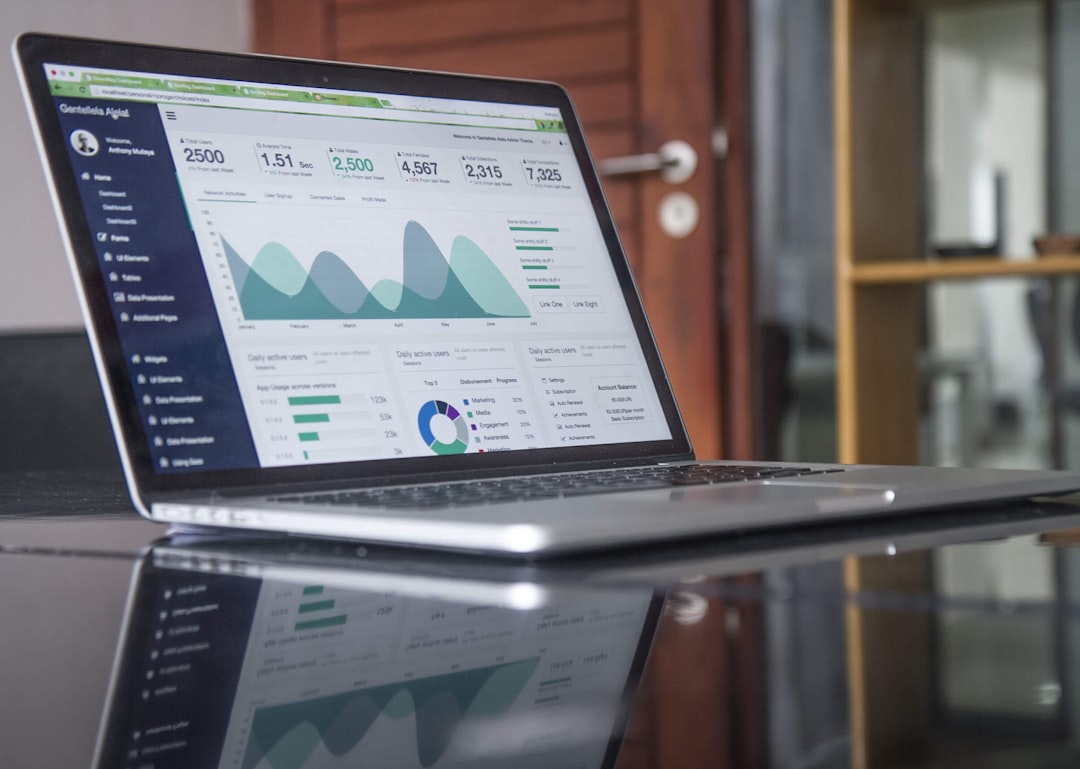
The methods used to gather data for whatsinmy.video analytics are becoming more complex as video's role in research grows. Mobile devices have made video recording widely available, enabling data collection in diverse real-world settings. Researchers now utilize qualitative video methods to capture detailed interactions and subtle nonverbal cues, enriching traditional approaches like interviews and focus groups. Yet, careful consideration is needed when deciding whether to collect audio, video, or both, as these choices can profoundly affect the outcome of the analysis. Understanding these data collection methods is critical for extracting valuable insights, especially when addressing the challenge of missing data and the various ways it's handled through imputation. While capturing a wide range of video data offers valuable potential, it also introduces new analytical complexities that need to be considered for effective insights. The methods and their subsequent impact on analysis are intertwined, making a comprehensive understanding of the process from data capture through to final interpretation a key part of the process.
Video analytics often relies on collecting data from various sources, such as user interactions, video viewing patterns, and even environmental context. While this comprehensive approach can offer richer insights, it also increases the difficulty of integrating and processing all the different data types.
One common method is observational studies, where researchers observe users' behaviors without intervention. This provides unbiased data but might introduce observer bias, as the observer's perceptions can influence the observations.
Handling large video datasets presents challenges due to the high computational demands of processing and analysis. In real-time applications, balancing the need for rapid data collection with accuracy while effectively managing resources is a critical concern.
Sensor data, like device orientation or ambient lighting, can greatly enhance the context of video analytics. However, combining such diverse datasets is a complex task, placing a heavy burden on researchers and engineers to properly integrate them.
Privacy regulations, such as GDPR, have profoundly impacted the way data is collected and stored. This means researchers need innovative methods for anonymizing data and obtaining user consent in order to ethically and legally collect data for video analytics.
The sampling method significantly impacts the generalizability of the collected data. While stratified sampling helps reduce bias, it can be quite demanding when working with very large and complex video datasets.
Automated tools are becoming increasingly popular for collecting data in video analytics. However, they sometimes fail to capture the subtle details of human behavior that traditional methods can, leading to potentially important data gaps.
The precise timing of data points, i.e. the temporal resolution, is crucial for video analysis. Small differences in timestamps can drastically affect the conclusions drawn from the data, so accurate time tagging is essential during data collection.
User-generated content presents a unique set of difficulties for video analytics. While this data can offer valuable insights, the wide variability in quality and context can complicate the process of comparing datasets.
Although it offers many advantages, collecting vast quantities of video data also introduces significant risks. These risks include data redundancy and the possibility of being overwhelmed with information, potentially hindering the effectiveness of analysis unless carefully addressed.
The Impact of Mean vs
Median Imputation on Video Analytics A Data-Driven Comparison - Impact of Mean Imputation on Video Engagement Metrics
When dealing with missing data in video analytics, using the mean to fill in gaps (mean imputation) can have a notable effect on how we measure video engagement. While mean imputation keeps the overall sample size and offers an unbiased estimate of the average when data is missing randomly, it can be easily influenced by unusual data points (outliers). These outliers can distort the results, potentially leading to incorrect understandings of viewer engagement patterns. This is especially problematic for video analytics, where accurate representations of engagement are essential for decision-making around content creation and strategy. Furthermore, relying solely on mean imputation can reduce the variety and mask inherent features within the data, which limits the depth of insights we gain. Because of these challenges, considering other ways to fill in missing data or using a combination of methods might provide more trustworthy outcomes in comprehending viewer behavior and levels of engagement.
1. When we use mean imputation to fill in missing data in video engagement metrics, like view counts, we risk inflating those metrics. This happens because the mean can be an overly optimistic average, potentially leading us to believe that audiences are more engaged and content is more popular than it actually is.
2. If our video data has a lot of extreme values (outliers), using the mean to fill in missing data can introduce a significant bias. This can skew our understanding of user engagement and lead to faulty conclusions from analyses that rely on this data.
3. The way mean imputation affects the variability in our data can make it harder to train machine learning models. Since it reduces the natural spread of video engagement metrics, the models might not generalize well to new data and might not be as accurate at predicting future engagement.
4. When we use mean imputation, it can mask patterns and trends that are present in video analytics. This makes it difficult to notice changes in how people are engaging with content over time. For example, we might miss shifts in engagement after a marketing campaign or a content update.
5. Video analytics often involves analyzing complex datasets with many different variables. Mean imputation might oversimplify the data by reducing the variability that is needed to capture the subtleties of how viewers interact with content and their preferences.
6. If we rely too heavily on mean imputation, we could misinterpret the overall results. The conclusions we draw might not accurately reflect the experiences of individual users, which is a key consideration for providing personalized recommendations.
7. In real-world situations, studies have shown that models trained with mean imputation often perform worse at video analytics tasks. This is particularly true when we evaluate their ability to predict future engagement with data they haven't seen before (holdout datasets).
8. Mean imputation can't adapt easily to new data as it comes in. This is problematic in environments where video content trends change quickly. We need more adaptable methods to keep up with these changes.
9. Understanding that video viewership numbers can often be skewed is very important. If we apply mean imputation without considering this skew, we might make wrong choices about content strategy based on faulty insights.
10. More sophisticated analytical techniques, like machine learning, struggle to work well when mean imputation has been used. This is because they may not be able to correctly interpret how the data is distributed. As a result, their predictions about viewer engagement and behavior might be inaccurate.
The Impact of Mean vs
Median Imputation on Video Analytics A Data-Driven Comparison - Effects of Median Imputation on Content Recommendation Accuracy
When crafting content recommendations, employing median imputation can lead to improved accuracy. This is primarily due to its ability to handle skewed datasets and minimize the impact of extreme values (outliers). In contrast, mean imputation, which relies on the average, can distort the true picture if the data is not normally distributed, potentially exaggerating performance measures. Median imputation, on the other hand, helps maintain the dataset's inherent structure and leads to more reliable results, especially in the context of video analytics where missing data patterns can significantly skew findings.
The increasing use of machine learning in content recommendation further underscores the importance of choosing the right imputation method. The chosen technique significantly influences how well machine learning models understand and predict user preferences. This impact can extend to the interpretability of the models' outputs and the ultimate accuracy of the recommendations themselves. By better representing the true nature of the data, median imputation plays a crucial role in the development of dependable recommendation strategies within the framework of video analytics. Effectively addressing missing data with robust methods like median imputation ultimately contributes to more insightful and reliable content recommendations.
1. Median imputation, compared to mean imputation, can better maintain the original distribution of the data, particularly in video analytics datasets which often have skewed distributions. This can lead to more accurate outcomes when building predictive models.
2. When utilizing median imputation, the resulting video engagement metrics tend to reflect the typical viewer behavior more accurately, as it better represents the central tendency of the data. This improves the clarity and ease of understanding when analyzing video engagement.
3. It's intriguing to see that median imputation can effectively reduce the likelihood of machine learning models overfitting to the training data. It accomplishes this by retaining the natural variance in the data while minimizing the influence of extreme values.
4. Research suggests that median imputation often leads to better performance when analyzing video data in real-time settings. This highlights its ability to maintain accuracy and responsiveness in fast-paced environments.
5. Median imputation, by focusing on the central tendency without being overly influenced by outliers, can facilitate the detection of anomalies in video analytics data. This makes it easier to identify genuinely unusual occurrences and helps distinguish them from the ordinary.
6. In cases where data is missing in a non-random pattern, using median imputation can lead to more reliable results. This is because it avoids the biases that can arise when relying on average values, which can be misleading when data is not missing randomly.
7. Applying median imputation can potentially lead to a better user experience in recommendation systems. This happens because it offers a more accurate and representative depiction of viewer preferences, improving the quality of recommendations and making them more relevant.
8. Median imputation has the ability to enhance the process of user segmentation in video analytics. By representing engagement levels more accurately, it allows for clearer divisions of users based on viewing habits and other demographic characteristics.
9. While median imputation may seem more complex than mean imputation, it doesn't necessarily come with a huge increase in computational cost. In some instances, it can lead to faster convergence in certain modeling algorithms, reducing the overall time required for analysis.
10. One practical benefit of median imputation is that it often requires less validation and correction steps after the initial analysis. This can streamline the video analytics workflow, as it minimizes the need for post-processing adjustments that are often necessary when using mean imputation.
The Impact of Mean vs
Median Imputation on Video Analytics A Data-Driven Comparison - Comparative Analysis of Imputation Techniques for User Behavior Prediction
When predicting user behavior, understanding how different methods for filling in missing data (imputation) affect the outcome is crucial. Mean imputation, a simple approach, can distort the data's true picture if there are extreme values (outliers) that skew the average. This can misrepresent user actions and hinder accurate predictions. In contrast, median imputation, which focuses on the middle value, provides a more reliable representation when the data isn't evenly distributed. This helps maintain the overall shape of the dataset and leads to better predictions. The field of video analytics is constantly evolving, and choosing the correct way to handle missing data becomes increasingly important. This decision impacts not only how well models perform but also aspects like recommending content based on user behavior. Moreover, recent developments in deep learning offer new techniques for imputation, which requires a continuous assessment and fine-tuning of strategies to deal with missing data, especially in complex situations.
1. When comparing imputation techniques, it's clear that the chosen method can significantly impact the accuracy of predicted user behavior, potentially leading to skewed interpretations of engagement metrics derived from video data.
2. While computationally simpler, mean imputation can generate misleading averages, potentially inflating user engagement figures and causing content developers to overestimate the effectiveness of their work.
3. Recent research highlights that median imputation frequently leads to improved model clarity, because it better matches our intuitive understanding of central tendencies within the often-skewed distributions seen in video analytics datasets.
4. The advantages of median imputation go beyond simple data correction; it can enhance user profiling by more accurately reflecting the typical patterns of engagement, helping platforms create more personalized content suggestions.
5. Models trained on median-imputed data have exhibited improved predictive accuracy on unseen data, emphasizing the need for accurately representing user behaviors to prevent decreased performance in real-world scenarios.
6. Interestingly, median imputation can mitigate the risk of machine learning models fitting too closely to the training data by preserving more realistic data variability, unlike mean imputation which can flatten the data distribution.
7. It's been noted that using a combination of imputation methods, instead of solely relying on mean or median imputation, might deliver improved predictive performance by capitalizing on the benefits of different approaches, allowing for a more nuanced treatment of missing data.
8. The time-based aspect of video engagement is important, and median imputation preserves time-dependent patterns better than mean imputation, making it a powerful tool for analyzing trends in user actions over time.
9. In environments with quickly evolving content, median imputation appears to adapt more effectively to new data, establishing a more stable basis for ongoing analysis as audience preferences shift.
10. The long-term efficiency gains from using median imputation, such as the decreased need for post-analysis verification, can optimize video analytics workflows, making it a desirable choice not only for better accuracy but also for overall operational effectiveness.
The Impact of Mean vs
Median Imputation on Video Analytics A Data-Driven Comparison - Future Trends in Data Handling for Video Analytics Platforms
The future of video analytics platforms hinges on how we manage and process the ever-growing streams of data. AI and ML are fundamentally reshaping the way we extract insights from video, driving innovation in analytical capabilities. Managing the surge in data volume requires a heightened focus on data quality and ensuring governance procedures are robust to guarantee reliable outcomes. We're seeing a push to make analytics more accessible, with trends like decentralization of data and the emergence of no-code solutions allowing broader access to powerful tools. This will likely spark more creative and diverse applications of video analytics. The increasing complexity of data sources is becoming more manageable as storage and analytics platforms improve their ability to handle the sheer variety of information found within video data. On the horizon, generative AI has the potential to dramatically change how video analytics is done, expanding its capabilities and use cases in numerous ways. Moving towards 2024, we expect the importance of responsible data practices to continue increasing. This means incorporating ethics into how we build AI and data strategies so that users and other stakeholders trust the insights video analytics provides.
The landscape of video analytics is rapidly evolving, with platforms now capable of analyzing up to 500 frames per second. This leap in processing power allows for real-time insights and complex computations that were previously out of reach, dramatically speeding up the analysis of video data. We're also seeing the integration of sophisticated deep learning models, like convolutional neural networks, into analytics tools. These models can automatically extract features from videos, moving us away from traditional, manual methods toward more autonomous analysis.
The rise of inexpensive sensors and the Internet of Things (IoT) is leading to the collection of multimodal data in video analytics. This means we can now combine video with audio and environmental context, which opens up a world of opportunities for comprehensive behavioral analysis. Intriguingly, the choice of imputation method doesn't just affect the accuracy of our predictions, but it can also impact user satisfaction. Research suggests that users respond more favorably to content recommendations that stem from analysis utilizing robust methods like median imputation.
As data privacy becomes more stringent, video analytics platforms are likely to embrace federated learning. This technique allows models to be trained on data spread across various locations without needing to centralize the data, making the system more privacy-conscious. We're also facing a growing challenge with the ever-increasing size of video datasets. Some platforms anticipate handling petabytes of data daily as user-generated content continues to explode. This creates a demand for innovative storage and computing approaches to efficiently manage and analyze this massive influx of information.
Edge computing is another emerging trend that could revolutionize the field by processing video data closer to its source, like on devices rather than centralized servers. This could greatly reduce delays and enable real-time decision-making based on video analytics. Furthermore, interactive video analytics is gaining traction. It empowers users to query video data in real-time while viewing content, offering tailored experiences based on immediate feedback, potentially boosting user engagement.
It's interesting to observe the emergence of hybrid imputation methods that combine mean and median techniques. This trend suggests that a single imputation method may not adequately address the complexities of modern video analytics datasets. Looking ahead, video analytics platforms are likely to adopt advanced anomaly detection methods. These methods rely on statistical techniques similar to those used in imputation and could lead to faster identification of critical issues, like security breaches or issues with content quality. While these developments are promising, they also highlight the ongoing need for careful consideration of the implications of these new analytical approaches.
More Posts from whatsinmy.video: