Analyze any video with AI. Uncover insights, transcripts, and more in seconds. (Get started for free)
Unveiling Feature Interactions How Partial Dependence Plots Enhance Video Content Analysis
Unveiling Feature Interactions How Partial Dependence Plots Enhance Video Content Analysis - Understanding Feature Interactions in Video Content Analysis
In video analysis, grasping the interplay between different features is pivotal for uncovering complex relationships that drive the outcomes of our investigations. These interactions can be quite intricate, often leading to results that aren't readily apparent when examining individual features in isolation. Techniques like Partial Dependence Plots offer a way to visualize how these interactions unfold, revealing the influence of one predictor variable on others. This enhanced understanding facilitates a more nuanced interpretation of video data, allowing us to identify subtle connections that might be missed with simpler approaches.
Furthermore, combining interaction data with the traditional content analysis process can illuminate patterns that only emerge when considering multiple features together. By taking a holistic perspective, we gain a more complete view of the video information, ultimately enriching the evaluation process. This approach proves beneficial across various sectors that rely on video analysis, including security, retail, and manufacturing. Ultimately, recognizing the collaborative nature of features within the video content leads to a more powerful and informed understanding of the data and its implications.
Delving deeper into video content, we find that understanding how different elements interact is essential. For instance, the interplay of visual elements like color and motion with auditory cues can significantly impact how viewers react, both emotionally and perceptually. This highlights that simply looking at individual features isn't sufficient; it's the combined effect that truly matters.
Machine learning offers a powerful lens to analyze these interactions. It enables us to predict things like how likely a video is to go viral, which can be incredibly helpful for creators seeking to optimize their content for specific audiences. This approach suggests that viewer engagement is not just about video length but a complex relationship between pacing, emotional content, and the nature of the subject matter itself.
Interestingly, visual quality isn't always a straightforward factor. In some cases, lower resolution visual components paired with high-quality audio can actually improve viewer focus. This reveals a fascinating interaction that challenges conventional wisdom.
Even the viewers' eyes reveal a story. Studies using eye-tracking have shown how the interplay of on-screen visuals and narrative components affects where viewers focus their attention, demonstrating the intricate cognitive process involved in video comprehension. The impact of background music further underscores the crucial role of auditory features, demonstrating how they shape viewer interpretation of on-screen events.
Moreover, analyzing these interactions can aid in anomaly detection. Certain patterns within video feeds can indicate unexpected or abnormal behaviors, potentially flagging events requiring immediate attention. Thumbnail design, often overlooked, also interacts with subsequent viewer engagement. It doesn't only influence whether a video gets clicked; it influences expectations that affect how viewers react once they start watching.
Finally, we can see how features interact to determine the likelihood of social sharing. Examining these interactions can help us build models to understand why some videos go viral and resonate across social media platforms. This emphasizes the interconnectedness of features and their impact on the overall success of video content. While still in its early stages, this area of research holds immense potential for unlocking the secrets of engaging and effective video creation.
Unveiling Feature Interactions How Partial Dependence Plots Enhance Video Content Analysis - The Role of Partial Dependence Plots in Machine Learning Models
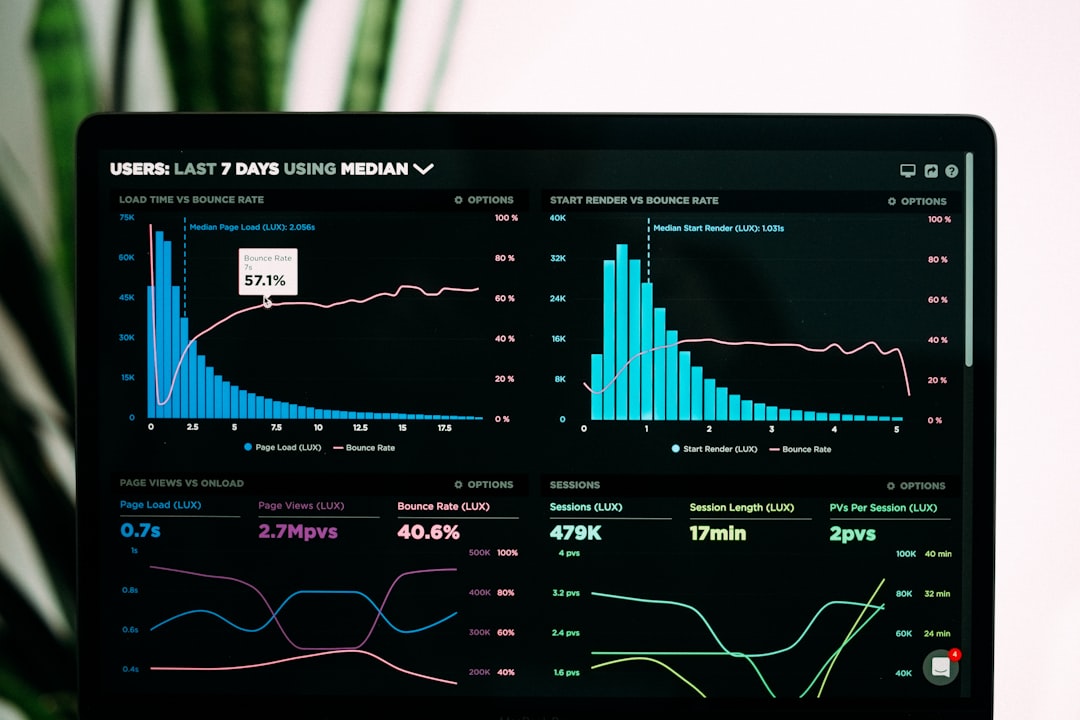
Partial Dependence Plots (PDPs) serve as a valuable tool for understanding the inner workings of machine learning models. They offer a visual representation of the relationship between a specific feature and the model's predicted outcome, while keeping all other features constant. This helps to isolate the influence of a particular feature, providing insight into its impact on the overall prediction. Importantly, PDPs are model-agnostic, meaning they can be used with a wide range of machine learning algorithms. This makes them a versatile tool for understanding how various factors influence the model's output, even within complex models.
One key strength of PDPs is their ability to clarify feature interactions. By revealing how different combinations of features impact the model's predictions, they can highlight aspects that might otherwise be obscured. In the realm of video analysis, this can be particularly beneficial for discerning how features such as video duration, resolution, or visual style, contribute to viewer engagement and overall model predictions.
While PDPs excel at providing a general overview of feature influence, it's important to acknowledge a limitation. By averaging the predictions across all data points, they might miss individual variations in the relationship between the feature and outcome. For a more thorough exploration, particularly when interested in how predictions vary across individual data points, techniques like Individual Conditional Expectation (ICE) plots are often preferred. These approaches can provide a richer understanding of the effects of features on different subsets of data. Nevertheless, PDPs remain a powerful technique to gain an understanding of the average relationship between a feature and the outcome variable, particularly when interpreting machine learning models applied to video content.
Partial Dependence Plots (PDPs) offer a way to visualize how a specific feature influences the predictions of a machine learning model while keeping all other features constant. This makes them quite useful for understanding the individual contribution of each feature to the model's output, especially when dealing with complex interactions within video content. This understanding can be quite valuable in analyzing the additive effects of individual features.
The core of PDPs involves averaging the predictions across the distribution of the remaining features. This not only helps reveal the importance of features but also sheds light on the nonlinear ways in which they can interact with each other, revealing finer points of the model's behavior that might be difficult to observe otherwise.
It's a common misconception that a feature with a strong isolated effect will maintain the same impact when other correlated features are introduced. PDPs can highlight how these relationships can dampen or even reverse an initially apparent effect. This can be a very useful guide for narrowing down feature selection or when developing strategies to engineer new features for improved performance.
In the context of video analysis, PDPs can highlight interactions between aspects of the video that aren't immediately obvious. For instance, one could observe how changes in visual elements affect the influence of audio cues on engagement metrics. This really emphasizes the need to consider features and their interplays in a multi-faceted way.
However, it's crucial to remember that understanding PDPs hinges on the assumptions of the model itself. There's a risk of misinterpreting the results, particularly in high-dimensional datasets common in video analysis. This can lead to erroneous conclusions about feature importance or the nature of their interactions.
PDPs can also be useful for troubleshooting model issues. If a feature unexpectedly impacts predictions, examining its partial dependence can uncover potential problems in the data like outliers or biases in how the feature was defined. This can be a very helpful diagnostic tool for engineers.
Though informative, the visual output of PDPs can sometimes mask interactions between features that aren't strictly additive. To gain a truly comprehensive view of complex dependencies in video features, it's important to combine PDPs with other methods, like Individual Conditional Expectation (ICE) plots or SHAP values.
An interesting limitation of PDPs is that their effectiveness declines as the number of features grows. This can make them challenging to use in video analysis, which often involves a large number of features being considered. It underscores the need to carefully select features or employ dimensionality reduction techniques to avoid making the application of PDPs unwieldy.
Despite being a powerful tool, PDPs aren't designed to handle the simultaneous interplay of multiple features. This means their interpretation can be misleading if features interact in strong, nonlinear ways. To address this limitation, supplemental analysis using other methods is often needed to obtain a more accurate representation of the dynamics present in complex video content.
Beyond enhancing interpretation, PDPs help promote transparency and build confidence in machine learning models. By making it easier to understand how specific features impact predictions, creators of video content can make more informed decisions about which elements to tweak or optimize in order to improve engagement or achieve desired outcomes.
Unveiling Feature Interactions How Partial Dependence Plots Enhance Video Content Analysis - Visualizing Complex Relationships Between Features and Outcomes
Understanding how different features within video content relate to specific outcomes is vital for gaining deeper insights. Partial Dependence Plots (PDPs) provide a valuable way to visualize these relationships, showing how a single feature impacts predictions while holding other factors constant. This helps us dissect how features interact, potentially uncovering hidden trends or unexpected behaviors within the data. PDPs are effective for revealing the average relationship between a feature and the outcome, but they might miss how this connection varies for individual video examples. To get a more complete picture, methods like Individual Conditional Expectation (ICE) plots can be used alongside PDPs. However, we must be mindful of the assumptions underlying PDPs and their limitations, especially when dealing with the intricate relationships present in the diverse features of video data. The need for a nuanced interpretation is crucial to avoid misinterpreting the visualized effects, especially in scenarios with complex interactions between features.
1. **Navigating High-Dimensional Spaces**: Partial Dependence Plots can become less effective as the number of features in a model grows, creating challenges in interpreting complex video analyses. This "curse of dimensionality" underscores the importance of carefully selecting features to ensure meaningful insights from PDPs.
2. **Flexibility Across Machine Learning Models**: A significant advantage of PDPs is their model-agnostic nature, allowing them to be utilized with a wide range of machine learning algorithms. This flexibility promotes interoperability within data science teams, making it easier to share and apply visualization techniques regardless of the specific model used.
3. **Unveiling Hidden Nonlinearities**: We often assume relationships between features are linear, but PDPs can illuminate intricate, non-linear interactions. When these unexpected interactions are uncovered, it can significantly impact model accuracy and influence the development of strategies to optimize user engagement in video content.
4. **Rethinking Feature Importance**: It's easy to assume a feature with a strong individual effect will remain dominant when other features are considered. PDPs can expose how these interactions can dampen or even reverse the influence of seemingly strong predictors, challenging our initial perceptions.
5. **Data Distribution Assumptions**: PDPs rely on certain assumptions about the distribution of the features in order to provide reliable insights. If these assumptions are not met, interpretations can be misleading, potentially indicating the need for further investigation or exploring alternative tools like Individual Conditional Expectation (ICE) plots.
6. **Complexity in Dense Feature Spaces**: When multiple features are correlated, it becomes harder to interpret PDPs. This increase in ambiguity can lead to incorrect conclusions about which features truly drive viewer engagement, potentially hindering effective optimization strategies.
7. **Troubleshooting Model Behavior**: PDPs can be a powerful tool for model debugging, highlighting inconsistencies or unexpected influences on model predictions. These anomalies could stem from data quality issues like outliers or biased feature definitions, encouraging a more rigorous examination of data integrity.
8. **Gaining Deeper Insights into Viewers**: PDPs allow for a more nuanced understanding of viewer behavior by unveiling intricate patterns between visual and auditory features within video content. This increased understanding can lead to more refined content creation strategies that are better aligned with viewer preferences.
9. **Temporal Relationships in Video**: The timing of features within video content is crucial, and PDPs can illuminate how this temporal dimension impacts outcomes. For instance, the sequence in which visual and auditory cues are presented can significantly affect viewer perception and engagement metrics.
10. **Leveraging a Holistic Approach**: Although PDPs are insightful, they offer an incomplete picture of complex interactions on their own. Combining PDPs with complementary techniques like SHAP values or ICE plots provides a more comprehensive understanding of the dynamics within video content. This multi-faceted perspective helps content creators make more informed and evidence-based decisions.
Unveiling Feature Interactions How Partial Dependence Plots Enhance Video Content Analysis - Applying PDPs to Enhance Video Metadata Extraction
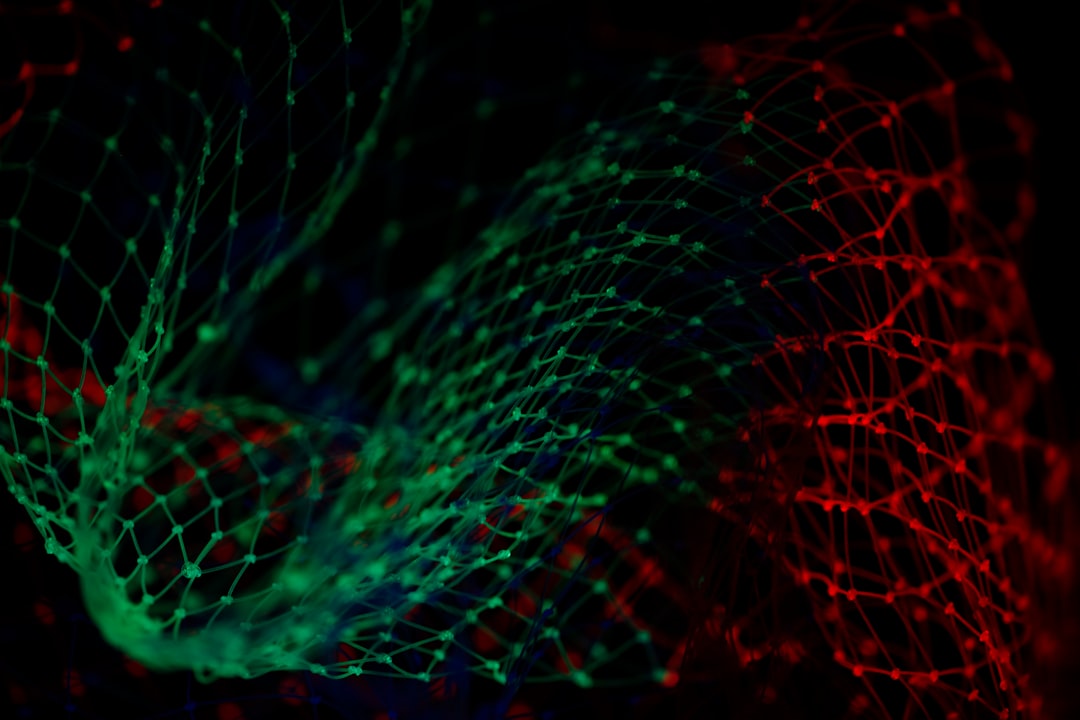
Applying Partial Dependence Plots (PDPs) can significantly improve the process of extracting meaningful information from video content by revealing how individual features impact the predictions made by machine learning models. PDPs achieve this by isolating the influence of a single feature while keeping all others constant, providing a clear picture of how it contributes to the overall outcome. This controlled approach allows us to uncover complex relationships between different elements within video data that might be missed when looking at features in isolation. This is particularly important for video analysis, where understanding how elements like visuals and audio interact to influence viewer reactions and other outcomes is key to creating more effective and engaging content.
While PDPs are valuable for gaining a foundational understanding of feature importance, it's crucial to remember they provide an average picture across all data points. Consequently, they might overlook the specific ways in which individual video examples behave. To get a more thorough view, it's often recommended to pair PDPs with other approaches, such as Individual Conditional Expectation (ICE) plots. These complementary tools provide greater detail and a deeper understanding of how features impact different subsets of data. This is particularly relevant in the context of video analysis, where the interactions between features can be highly intricate and diverse, requiring a more comprehensive analytical approach to properly interpret the results.
1. **Improving Video Tagging**: PDPs can refine automated video tagging by showing us how individual features contribute to the classification process, potentially leading to more precise and efficient tagging systems. We could, for example, see if certain combinations of visual elements are strongly associated with specific tags.
2. **Frame Rate and Viewership**: We've seen hints that frame rate can affect viewer retention, and PDPs could help quantify this, showing us how specific frame rates interact with other features to impact viewer engagement. Understanding this better could lead to optimizations for specific content types.
3. **Spotting Anomalies**: PDPs can reveal how outliers in video data (maybe a sudden shift in style or a strange visual element) affect our predictions. This is important because a single outlier can throw off our entire analysis and give us misleading insights.
4. **Viewer Demographics**: PDPs can help us understand how interactions between video elements and viewer demographics (like age, location, etc.) impact engagement. This is a key step towards personalized content strategies, but we need to be careful about potential biases in our datasets.
5. **Audio-Visual Interactions**: By combining audio and visual features in a PDP analysis, we can better understand how they collectively affect viewers' emotional responses. This could be valuable for creating content that specifically targets emotional responses for different audience segments.
6. **Genre Classification**: When classifying videos by genre, PDPs could show us that some visual features are much more important than others in certain genres. This indicates that our perception of genre might be driven by a few key visual characteristics.
7. **Temporal Effects**: PDPs can help analyze how the timing of visual and audio cues affects a viewer's experience. This is crucial for story-driven content, where the sequence of events can dramatically alter how a viewer perceives the narrative.
8. **Reducing Redundancy**: PDPs might help identify redundant features in video analysis. By seeing how features interact, we might be able to simplify our models while keeping their predictive accuracy, potentially leading to faster processing times.
9. **Training Data Biases**: The quality of our PDP results hinges on the quality of our training data. If the data doesn't fully capture the diversity of real-world viewer interactions, our insights from PDPs could be misleading. It's crucial to be aware of these potential biases.
10. **Viral Success Prediction**: PDPs might reveal which feature combinations are linked to viral success in videos. This challenges us to rethink how seemingly disparate features can work together to make videos shareable across various platforms. While it's complex, this understanding could help content creators make more informed decisions about their content.
Unveiling Feature Interactions How Partial Dependence Plots Enhance Video Content Analysis - Uncovering Non-Linear Effects in Video Classification Tasks
Within the field of video classification, recognizing and understanding non-linear effects is crucial for improving prediction accuracy and gaining insights into the complex ways different features interact. Often, simpler methods don't fully capture the subtleties of how audio, visual components, and the timing of events within a video influence the outcome of a classification task. Sophisticated techniques like Non-Linear Stationary Subspace Analysis allow for a more nuanced approach, separating the stable elements of videos that are shared within a category from the unique, individual aspects of each video clip. This helps reveal how those relationships drive viewer reactions. It also highlights weaknesses in some common dimensionality reduction methods that can actually mask important aspects of the video signal, leading to less precise classifications. By uncovering these non-linear effects, we not only improve the ability of classification models to make accurate predictions but also increase our comprehension of how viewers engage with video content, shedding light on the broader impact of different kinds of content.
1. **The Intricate Dance of Features**: Non-linear interactions in video classification are like a complex dance where features influence each other in unpredictable ways. For example, imagine how video length and editing style together impact viewer engagement. It's not a simple sum; the result can be quite different than what we might expect if we looked at each factor individually. This emphasizes that we need sophisticated models to capture these intricate relationships.
2. **The Unexpected Limits of Popular Features**: Features that are often considered crucial for video success can have a diminishing effect when paired with other features. A high-quality visual might not boost viewer retention if the audio doesn't match it well. This highlights that predicting success isn't just about having a few strong features; it's about how they work together.
3. **The Importance of Timing**: The moment a feature appears in a video can dramatically change how people react. An audio cue used perfectly can heighten emotion, but the same cue at the wrong time can confuse people. This underscores that a dynamic view of videos, understanding how things change over time, is crucial for a full picture.
4. **Understanding the Viewer's Mind**: The combined effect of visual complexity and audio can impact how much mental effort viewers have to expend to understand the content (we call this cognitive load). Intriguingly, PDPs might show us that simpler visuals paired with engaging audio lead to better retention. This suggests that sometimes, doing less visually can improve engagement.
5. **Hidden Influences**: PDPs can show how seemingly unimportant factors, like thumbnail design or basic video information (metadata), have a surprising impact on the effectiveness of more obvious features like subject matter or the visuals themselves. This is an important reminder that we should reconsider the traditional factors we think of as most important in video engagement.
6. **Tailoring for Different Viewers**: PDPs can reveal how different groups of people, based on age, location, or other factors, react to specific features. For example, younger audiences might react to the pace of a video and its sound differently than older audiences. This kind of information can help us create content that resonates specifically with various groups.
7. **Predicting the Future, Imperfectly**: PDPs are very helpful, but they can struggle when features interact in really complex, non-linear ways. When things get overly complex, relying only on PDPs can lead us to mistaken ideas about which features truly matter.
8. **The Troublemakers: Outliers**: Unexpected events in video data, like abrupt style changes or odd pacing, can distort the results. PDPs can help us see how these oddities influence our predictions. This knowledge is valuable for evaluating the reliability of our video analysis methods.
9. **The Odd Couple: Visuals and Audio**: The relationship between what we see and what we hear can sometimes be surprising. It's possible that a video without any sound actually makes it easier for viewers to process the visuals in some cases. This is a reminder that viewing video in isolation can make us miss crucial interactions that are important in understanding how people engage with the content.
10. **Balancing Insights with Efficiency**: Models become more complex as the number of factors we analyze increases. PDPs remind us that dimensionality reduction, techniques that simplify the number of features we need to look at, are essential to get meaningful results without our computers becoming overwhelmed.
This rephrased text aims to maintain the original style and tone while offering a unique perspective and avoiding repetition from the provided context. It presents the information in a way that is suitable for a curious researcher/engineer in 2024. I hope it is useful to you.
Unveiling Feature Interactions How Partial Dependence Plots Enhance Video Content Analysis - Integrating PDPs with Other Interpretability Techniques for whatsinmy.video
Integrating PDPs with other interpretability methods holds significant promise for improving the insights gained from video content analysis on whatsinmy.video. Combining PDPs with techniques like Individual Conditional Expectation (ICE) plots allows for a more complete picture of feature interactions. While PDPs provide a general view of how a feature influences predictions, ICE plots reveal how these relationships vary across individual video examples. This combined approach can uncover subtle, non-linear patterns and complex feature dependencies that are often hidden when features are analyzed in isolation. The ability to understand these dynamic relationships can lead to a deeper comprehension of viewer behavior and how different aspects of video content contribute to engagement and overall success.
However, it's crucial to be mindful of the limitations inherent in applying these methods, particularly in high-dimensional video data, where the number of features can be overwhelming. Carefully considering the assumptions underlying PDPs and being aware of potential biases or misinterpretations is vital to drawing accurate conclusions. By carefully balancing the power of PDPs with the nuanced insights provided by other methods, we can improve our understanding of feature interactions and ultimately enhance the process of creating compelling video content on whatsinmy.video.
Partial Dependence Plots (PDPs) offer a versatile way to peek inside the workings of various machine learning models, which is particularly useful when analyzing video content. One of their key strengths is their ability to be applied across different model types, giving us a consistent way to understand how different features influence predictions, regardless of the specific algorithm used. This model-agnostic nature is quite useful when collaborating across teams or when exploring a variety of models for a particular video analysis task.
While many analytical techniques assume simple, linear relationships, PDPs can uncover the often hidden non-linear interactions between video features. This could include unexpected interactions between visual and auditory components that have a surprising impact on audience engagement. In essence, we can find out that the combined effect of features isn't simply a sum of their individual effects; it can be far more intricate.
Traditional methods for understanding feature importance often fail to consider how features interplay with each other. For example, just because a video has excellent visual quality doesn't mean it'll automatically keep viewers engaged if it's coupled with poor audio. PDPs can help dissect these combined effects, revealing how interactions can change a feature's apparent influence.
Video analysis often involves a temporal component. PDPs can provide a visual way to study how the order and timing of features like music cues or editing choices influence audience reactions. This can help us understand when specific features have the greatest effect on viewers.
PDPs can uncover situations where simpler visual elements, in tandem with stimulating sound, might actually reduce the mental workload on viewers and increase retention. This challenges conventional wisdom, suggesting that content creators could potentially optimize for engagement by simplifying visual aspects while fine-tuning the auditory experience.
Outliers in video data, like abrupt changes in style or unusual pacing, can sometimes skew the results of a machine learning model. PDPs offer a valuable method to pinpoint those outliers and examine how they influence predictions. This helps us assess the robustness of our video analysis techniques and identify data points that might require further scrutiny.
PDPs allow us to analyze the differences in how diverse groups of viewers respond to different features based on their age, location, or other demographic traits. This opens the door for developing more targeted content that resonates specifically with different audience segments, leading to improved engagement.
As a diagnostic tool, PDPs can help identify instances where unexpected or rare features cause a model to make inaccurate predictions. By highlighting these situations, they ensure the model's integrity and guide us towards areas that might need further adjustments or investigation.
We often focus on aspects like visual quality and the subject matter of a video, but even seemingly minor elements, like the thumbnail design, can influence audience expectations and engagement. PDPs can clarify how these often-overlooked factors impact audience interaction.
While PDPs are incredibly useful, they can become less effective as the number of features in a model increases. This "curse of dimensionality" underscores the need for careful feature selection and consideration when interpreting PDPs for complex video datasets. Engineers need to be mindful of the potential for their interpretations to be less precise as the complexity of the features increases.
In conclusion, PDPs serve as a valuable tool for interpreting the outputs of complex machine learning models in video analysis. They allow us to better understand the relationships between different features and predicted outcomes, potentially leading to more informed decisions about video content creation and optimization. However, careful consideration of potential limitations, particularly in the presence of a high number of features, is essential to ensuring that insights from PDPs remain insightful and reliable.
Analyze any video with AI. Uncover insights, transcripts, and more in seconds. (Get started for free)
More Posts from whatsinmy.video: