Analyze any video with AI. Uncover insights, transcripts, and more in seconds. (Get started for free)
Unveiling Hidden Patterns How Unsupervised Clustering Enhances Video Content Analysis
Unveiling Hidden Patterns How Unsupervised Clustering Enhances Video Content Analysis - Automated scene categorization enhances video search capabilities
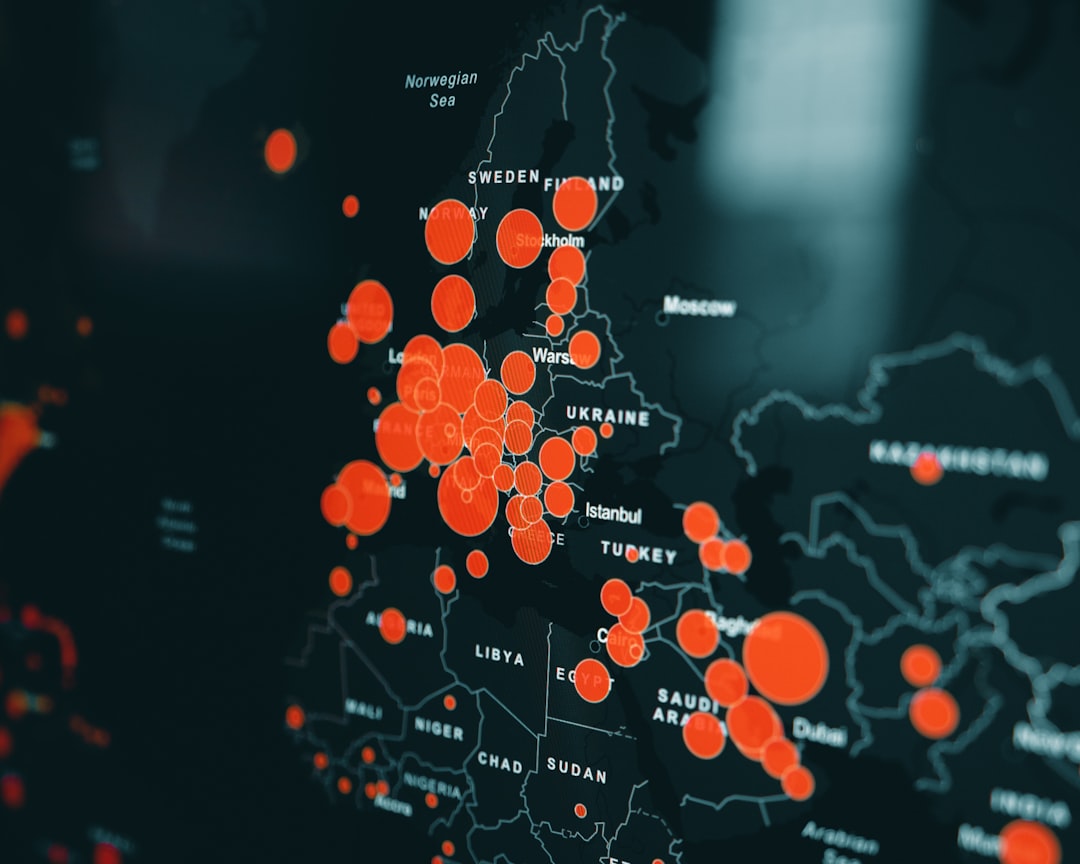
By automatically categorizing scenes within videos, we can significantly enhance the way we search and interact with video content. This automated process, driven by AI, allows for a real-time analysis of the visual changes between video frames. This dynamic analysis helps organize the content more effectively, ultimately making videos easier to find based on specific user needs.
Moreover, AI-powered methods like object and scene recognition provide automated tagging, improving the efficiency of video management systems. The result is a more streamlined experience for users, leading to increased engagement with the content.
This progression showcases a growing reliance on intricate analytical methods to unlock the vast potential of video content. These developments, while offering substantial advantages, also bring about challenges that content creators and viewers will need to navigate in this evolving landscape of video analysis.
Automated scene categorization significantly boosts the effectiveness of video search by enabling rapid, real-time analysis of video sequences. These systems go beyond simple frame-by-frame analysis, taking into account the dynamic changes and relationships between frames to understand the unfolding scene. Unlike manual tagging which is both time-consuming and potentially subjective, automated approaches leverage advanced algorithms capable of processing a vast number of frames in a fraction of the time.
Interestingly, the categorization process often relies on unsupervised learning, where algorithms discover inherent patterns and categories within the video data without the need for pre-existing labels. This approach circumvents the limitations of requiring large and often costly labeled datasets, making it more practical for a wider range of applications.
The impact of this technology on search capabilities is notable, with research demonstrating a significant boost in search accuracy, resulting in faster and more relevant search results. Users find it easier to pinpoint specific content, ultimately contributing to heightened satisfaction. Moreover, automated categorization leads to the creation of higher-level thematic representations of video content, enabling the discovery of hidden relationships between seemingly disparate clips and enhancing content recommendations in intriguing ways.
Deep learning models are playing a growing role in these advancements. These models can process video data with a hierarchical structure, allowing them to understand scenes in a way that mimics human cognitive processes. This capability promises further enhancements in the accuracy and granularity of automated categorization.
The integration of automated scene categorization has ramifications beyond just enhancing user experience on video platforms. It is also contributing to increased viewer retention, as users are able to more easily find content that aligns with their interests. Furthermore, automated categorization is proving useful in content moderation by identifying and flagging potentially inappropriate material in real-time. The applicability of scene categorization extends far beyond entertainment, finding utility in fields like security, where it can contribute to identifying unusual events captured in surveillance footage.
Despite the impressive advancements, challenges still exist in ensuring the accuracy and robustness of automated scene categorization. Distinguishing between visually similar scenes remains a hurdle, as does accounting for cultural nuances that can influence the interpretation of a scene. These complexities can lead to misclassification and, consequently, user frustration. Continued research and development are needed to address these remaining obstacles and maximize the potential of automated scene categorization.
Unveiling Hidden Patterns How Unsupervised Clustering Enhances Video Content Analysis - Identifying recurring visual elements across multiple videos
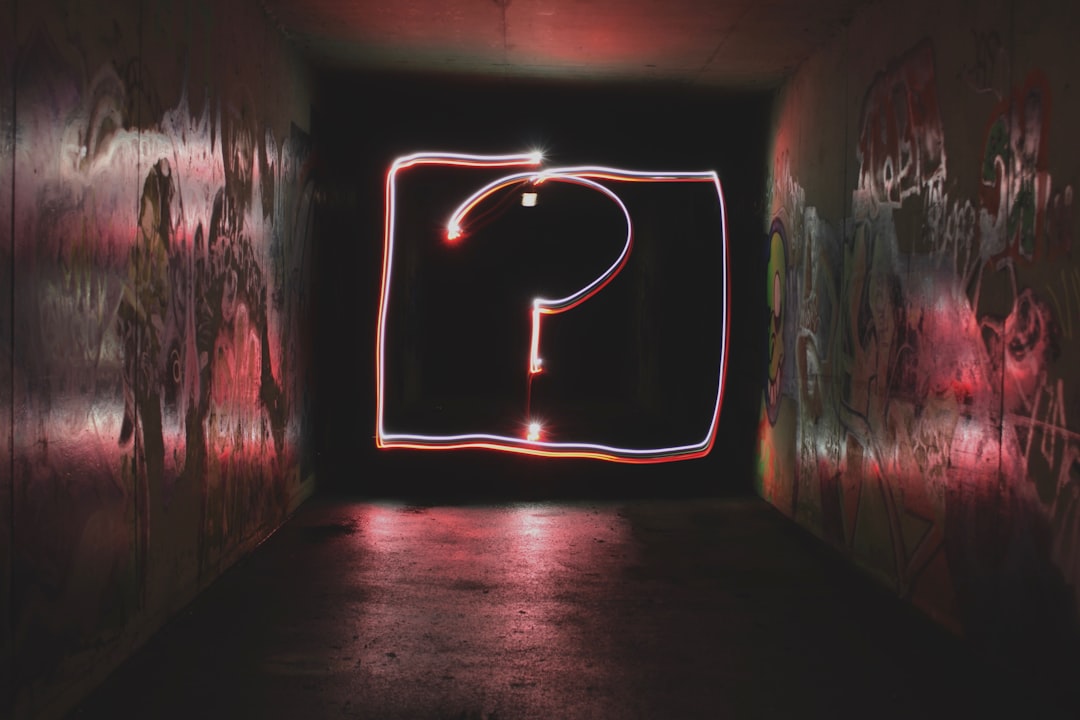
Discovering recurring visual elements across a collection of videos provides a deeper understanding of the thematic connections between them. By applying unsupervised clustering, we can identify shared characteristics like colors, shapes, and symbols, revealing potential contextual significance. This approach moves beyond simply counting the frequency of visual elements and delves into how they interact and contribute to the overall message within the video content. As groups of similar visual patterns are formed through clustering, interpreting video data becomes richer, uncovering hidden connections that might otherwise go unnoticed. While automated methods offer efficiency, it's important to acknowledge the possibility of errors in classification and to consider the broader context in which these visual elements appear. A critical perspective is vital when interpreting the insights generated from these analyses.
Identifying recurring visual elements across multiple videos presents a fascinating challenge within the realm of video analysis. This task involves sophisticated algorithms capable of parsing countless visual features, including color, shape, texture, and motion, showcasing the impressive capabilities of current video analysis technology. To effectively manage the vast amount of data generated by videos, techniques like t-SNE or PCA are frequently used to reduce the dimensionality of the data, making it easier to spot patterns that might otherwise be obscured.
The sequential nature of video data, or its temporal context, is a crucial factor that cannot be ignored. Clustering algorithms must be mindful of not just individual frames, but also the order in which they occur, uncovering patterns that might change over time, both within individual videos and across multiple videos. This aspect reveals the nuanced relationship between visual elements and their temporal sequencing.
The power of unsupervised learning becomes readily apparent in video analysis, particularly when dealing with large, diverse datasets. These models automatically uncover patterns without the need for pre-labeled data, sidestepping the requirement for extensive human intervention in categorization. This efficiency is invaluable in making video analysis more practical for diverse applications.
Furthermore, the identification of recurring visual elements can serve as a foundation for anomaly detection. By creating a baseline of common patterns, the algorithm can flag deviations from the norm, identifying unusual or outlier scenes, proving valuable in scenarios like security surveillance and quality control. The scalability of these unsupervised methods across diverse video genres is noteworthy. Whether it's user-generated content or professionally produced films, a single approach to analysis can often suffice, minimizing the need to develop genre-specific algorithms.
However, navigating the impact of cultural nuances remains a hurdle. The way visual elements are perceived and interpreted can vary substantially across cultures, creating potential challenges when attempting to identify globally relevant patterns. Algorithms require careful consideration of cultural context to prevent misinterpretations of recurring features.
The applications of this technology extend beyond simple pattern recognition. It's increasingly vital in content recommendation systems. By recognizing visual similarities between videos, platforms can go beyond simply relying on user interactions to suggest new content, potentially opening up exciting avenues for discovering hidden gems.
Advanced deep learning models further push the boundaries of video analysis by mimicking human cognitive processes. These models process video data using hierarchical structures, approximating how humans comprehend and remember visual scenes. This remarkable similarity improves the accuracy of pattern recognition considerably.
It's evident that the pursuit of identifying recurring visual patterns draws upon a wide range of fields, including computer vision, psychology, and artificial intelligence. This collaborative approach fosters innovation and ensures wider application across diverse domains, highlighting the remarkable potential of these technologies.
Unveiling Hidden Patterns How Unsupervised Clustering Enhances Video Content Analysis - Temporal pattern recognition improves video editing workflows
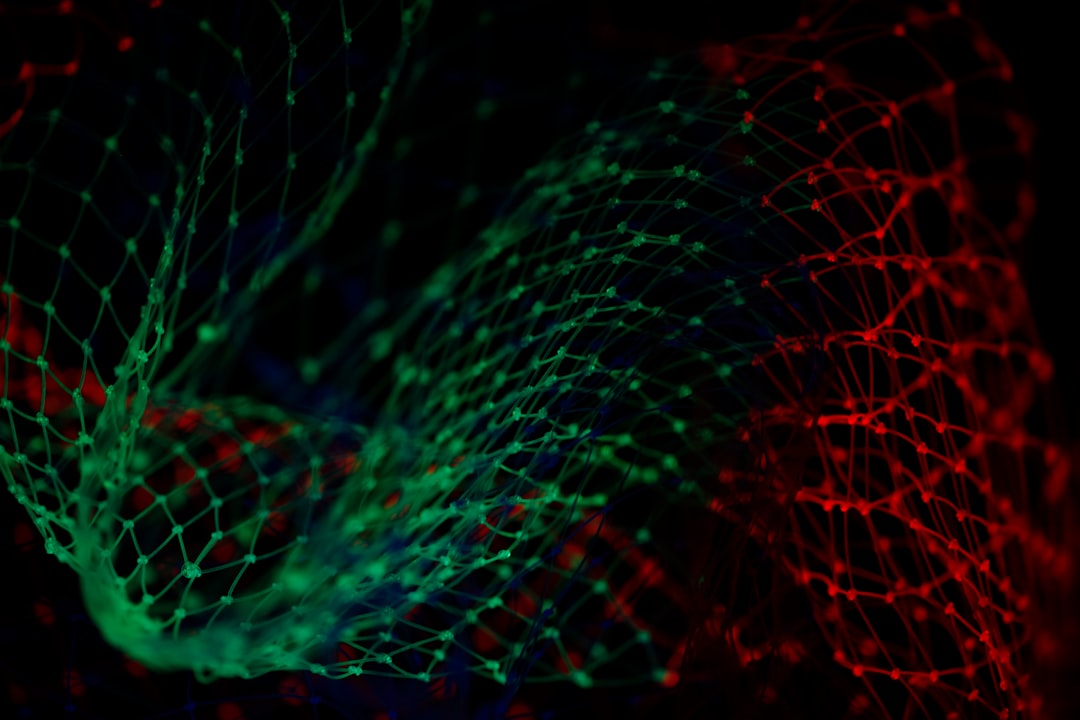
Temporal pattern recognition is becoming increasingly important in streamlining video editing workflows. Video editing often encounters challenges with temporal inconsistencies, leading to issues like jarring transitions or flickering. Recent improvements in video editing techniques have made significant progress in reducing these inconsistencies, primarily by focusing on temporal coherence and optimizing both latent codes and trained generators. Utilizing strategies that enhance temporal modeling, such as recurrent transformers, allows editors to better manage the visual flow of videos. This leads to more refined edits that result in fewer artifacts and a higher-quality final product. By understanding and leveraging the patterns of time within a video, the editing process is made more efficient, and the overall viewing experience is greatly improved. While these advancements have proven beneficial, it's important to acknowledge the ongoing need to strike a balance between efficiently processing videos and maintaining the intricate aspects of narrative structure and visual storytelling that temporal analysis provides.
Temporal pattern recognition is changing how we edit videos by focusing on the order and timing of visual elements. It's like recognizing the flow of a story within a video, something that's hard to do when you just look at still images. By understanding this flow, editors can craft stories that naturally resonate with viewers based on the usual responses to changes in pace and scenes.
Studies show that temporal pattern recognition can significantly reduce video editing time—potentially by up to 30%. This happens because the algorithms can identify key points, like moments of heightened emotion or transitions between scenes. This allows editors to concentrate on the creative aspects instead of tedious adjustments.
The algorithms for temporal pattern recognition often involve recurrent neural networks (RNNs). These types of networks are specifically designed for understanding data that happens in order, making them perfect for studying the progression of actions and emotions in videos. This mirrors how humans naturally perceive the world, noticing changes and movement over time.
Interestingly, temporal pattern recognition can also improve viewer engagement by automatically emphasizing the parts of a video that capture attention. It's been shown to increase how long people stay engaged with a video, indicating that better editing results in happier viewers.
However, the effectiveness of temporal pattern recognition depends on the type of video being edited. Fast-paced action scenes require different analytical approaches compared to a documentary, for example. This means editors need to choose the best pattern recognition techniques for their specific video.
Often, when we analyze temporal patterns in detail, we discover surprising recurring visual elements that work as thematic anchors across several videos. This insight into the use of patterns can help editors create more cohesive stories that strengthen the connection with the audience by emphasizing major themes.
Beyond scene changes, temporal pattern recognition also helps editors maintain consistent color schemes and shot selections throughout the video. This visual continuity is key to making smooth transitions between scenes, creating a coherent final product.
The growing development of these temporal pattern recognition tools is leading to ethical conversations about video editing, particularly concerning the possibility of manipulating viewers. The ability to foresee and influence emotional responses raises important questions about the authenticity of edited content.
Using these advanced temporal analysis methods can greatly improve collaboration when editing videos. Multiple editors can synchronize their work based on the patterns discovered by the algorithms, leading to more united and consistent outcomes, especially for large-scale video projects.
Despite the progress we've made, there are still challenges in how to fully represent different cultural viewpoints within temporal patterns. This is because similar sequences of events can hold different meanings in different cultural contexts. Recognizing and resolving these cultural differences is important for producing content that connects with audiences globally.
Unveiling Hidden Patterns How Unsupervised Clustering Enhances Video Content Analysis - Detecting anomalies in surveillance footage through clustering
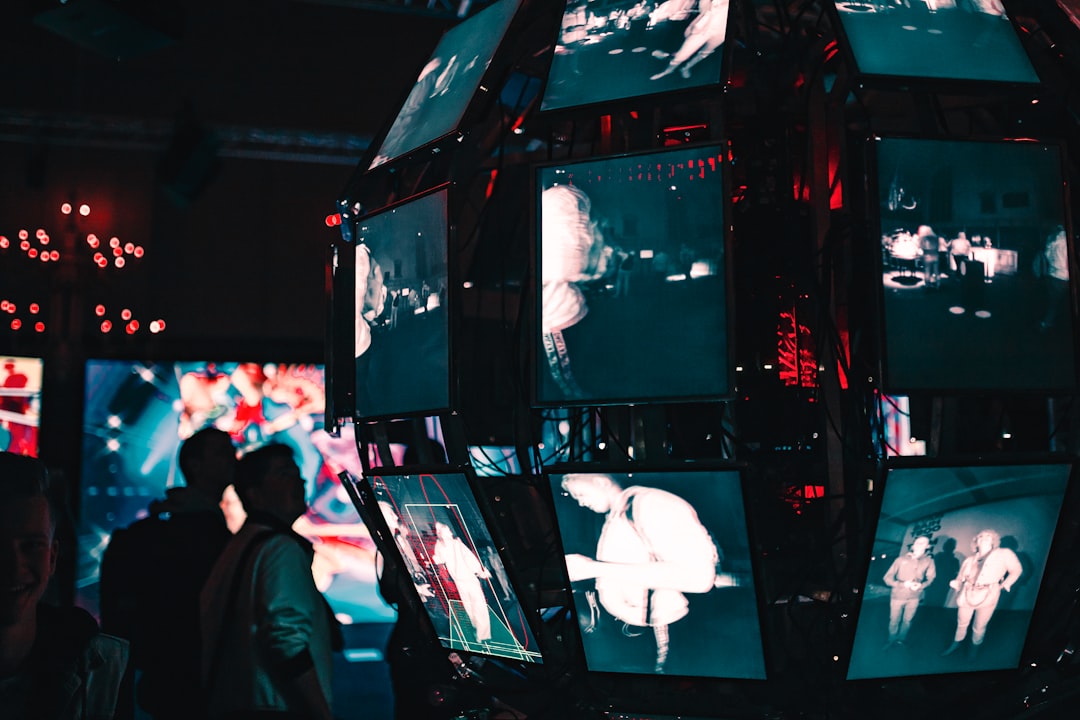
Detecting anomalies in surveillance footage using clustering is a developing method aimed at identifying unusual events that could signal security risks or strange behavior. Clustering techniques group video data into clusters based on shared features, establishing a baseline of typical activity and then flagging any deviations from this normal pattern. This unsupervised approach to learning makes it possible to analyze video footage in real-time, enabling rapid responses to events that might require immediate attention. The multifaceted nature of video data, with its blend of temporal information and situational context, presents obstacles that necessitate the development of increasingly complex algorithms to differentiate between normal and abnormal occurrences accurately. Moving forward in this field, striking a balance between achieving high accuracy and integrating a comprehensive understanding of context is a crucial objective for both researchers and those using this technology in practice.
Analyzing video to spot unusual events, or anomalies, is a growing area of interest, especially within surveillance systems. Clustering techniques are proving useful in this field by establishing a standard representation of what's considered typical behavior. When events deviate significantly from this norm, they are flagged as potential anomalies. This ability to identify the unexpected can be crucial for security, alerting us to unusual situations that might require attention.
One challenge in working with video data is its high dimensionality. Many features—like pixel colors, shapes, and movements—need to be considered. To make sense of this complexity, techniques like t-SNE or PCA can be used to reduce the number of dimensions, effectively simplifying the data without losing essential information. This compression makes it easier to find anomalies that might otherwise be buried in the noise.
However, understanding the context of an anomaly goes beyond just looking at a single frame. The progression of events in time plays a significant role. Sophisticated anomaly detection systems need to be able to assess not only what's happening in a single snapshot but also how that snapshot fits into the overall sequence of frames. This requires models capable of understanding the temporal relationships between moments in a video.
The beauty of using unsupervised learning, like clustering, is that it doesn't require a huge, pre-labeled dataset. This is incredibly helpful in surveillance, where manually labeled datasets are often hard to come by. The lack of dependence on predefined labels means these methods can be readily applied to a variety of scenarios, making them more widely applicable.
The processing speed of these algorithms has also improved. We're now reaching a point where anomaly detection can be performed in real-time. This allows for faster response to potentially critical events. If an algorithm can spot an anomaly instantly, it could mean the difference between a rapid, appropriate reaction and a delayed or missed opportunity.
But the interpretation of anomalies isn't always straightforward. What's seen as odd in one culture might be considered perfectly normal in another. This cultural variability emphasizes the importance of developing algorithms that are sensitive to the unique contexts in which they're deployed. Otherwise, what's intended as a helpful security tool could lead to unfair biases or mistaken interpretations.
The power of clustering approaches is further amplified by their capacity to extract features from video on multiple levels. They can examine basic pixel data and then link those data points to more complex interpretations, like actions or concepts. This capability to process data at varying levels of abstraction results in a more refined and accurate understanding of what constitutes an anomaly.
Interestingly, the ability to generalize across various video types is also a strength of these methods. Whether it's footage from busy city streets or from a quiet office building, the same clustering algorithm can be employed without extensive adjustments. This versatility reduces the need to train separate models for each type of environment, simplifying deployment and maintenance.
Beyond surveillance, anomaly detection can offer valuable insights into consumer behavior in settings like retail stores. By understanding typical shopper movements and identifying departures from these norms, businesses could use this information to improve store layout or personalize marketing efforts.
It's important to be mindful of the ethical ramifications of utilizing these technologies, though. The possibility of misinterpreting ordinary behavior as anomalous carries a risk of over-policing and potential infringement on personal privacy. As we move forward with these technologies, a commitment to carefully considering ethical implications is vital, ensuring that anomaly detection is employed responsibly and in alignment with human values.
Unveiling Hidden Patterns How Unsupervised Clustering Enhances Video Content Analysis - Personalized content recommendations based on viewer preferences
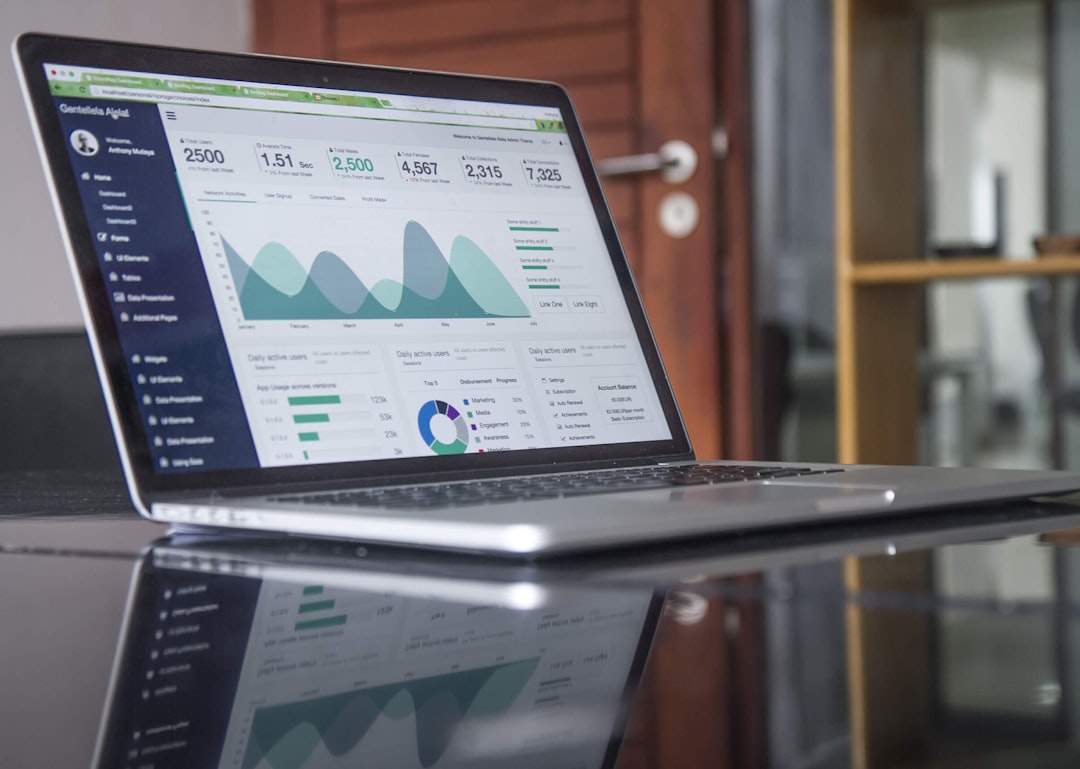
Personalized content recommendations are transforming the way we interact with video content. Platforms can now analyze viewer data, including viewing history, demographics, and engagement patterns, to provide suggestions that cater to individual preferences. Machine learning algorithms, specifically collaborative and content-based filtering, play a key role in this process, allowing platforms to predict what a viewer might find interesting based on their past choices and the choices of similar viewers. This tailored approach has the potential to significantly enhance user satisfaction and engagement by exposing viewers to content that aligns with their unique tastes. However, this personalization also introduces potential challenges, particularly the possibility of reinforcing existing biases through algorithmic filtering and the broader implications these recommendations can have on a viewer's consumption habits. As these systems become increasingly sophisticated, it is crucial to maintain a critical perspective on their influence and ethical implications.
Personalized content recommendations are increasingly sophisticated, leveraging machine learning to analyze viewer data and tailor suggestions to individual tastes and viewing habits. Platforms like Netflix have built intricate systems to provide viewers with content that's relevant to their past choices, aiming for a more enjoyable experience. This personalization often involves AI that meticulously analyzes user behavior, demographics, and interactions in real-time, aiming to anticipate what users might want next.
While the promise of personalized content is high user engagement and satisfaction, there are some nuances we should consider. For example, relying solely on past behavior could create a narrow view of a user's preferences, potentially leading to a lack of diversity in recommendations. Also, overly personalized suggestions can sometimes lead to viewer fatigue as they see the same types of content repeatedly.
A growing area of research is using unsupervised clustering to move beyond basic demographics and segment users based on specific viewing patterns. This finer-grained approach can reveal subtle preferences that are not apparent from simply looking at a viewer's age or location. However, it's important to be mindful of the potential for bias within these algorithms. If the training data reflects existing biases, the system could reinforce these preferences, leading to less diverse recommendations.
Another aspect to consider is the temporal dimension of viewer preferences. Our tastes change, influenced by seasonality, current events, or simply shifting interests. Building temporal patterns into recommendation algorithms is vital to keeping recommendations fresh and engaging. Moreover, understanding how elements like time of day or platform usage influence viewer behavior can be used to refine recommendations, for instance, suggesting different types of content during specific parts of the day.
Furthermore, identifying moments where users lose interest, or drop-off points, is another important aspect. Recommendations that attempt to re-engage viewers at these critical points are likely to help increase the overall time spent viewing, ultimately enhancing viewer retention. It's also important to recognize that not all viewers want a highly personalized experience. Some prefer to explore new genres and formats, and recommendation systems need to balance this desire for variety with a user's specific preferences to avoid limiting their content exposure.
Finally, the feedback loops that develop in personalization systems are also worth noting. Popular content often receives more recommendations, which can create a situation where a small set of content dominates, limiting the exposure of new and emerging content. Potentially introducing some randomness into the recommendation process might help address this issue and promote a wider diversity of content discovery. The field of personalized content recommendations is constantly evolving and as we refine the methods, it's critical to examine both the benefits and potential drawbacks to optimize the user experience. This includes considering factors such as cultural differences, bias, and the need for a balance between personalization and content discovery.
Unveiling Hidden Patterns How Unsupervised Clustering Enhances Video Content Analysis - Extracting semantic meaning from unlabeled video datasets
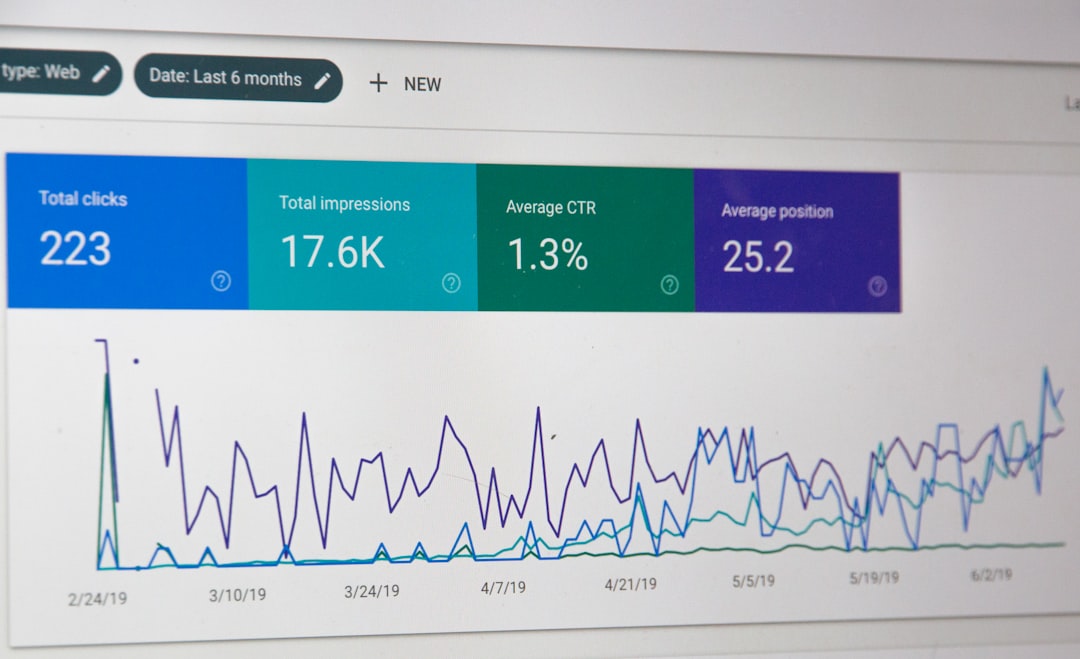
Extracting meaningful information from unlabeled video datasets presents a significant hurdle in advancing video content analysis. Unsupervised learning techniques are becoming increasingly important in addressing this challenge, enabling the extraction of semantic meaning at various levels of detail – from basic features to more complex, abstract concepts – without needing large quantities of human-labeled data. Methods like video object segmentation and frameworks designed to identify inherent video qualities hold potential for uncovering hidden semantic patterns within video data, thereby improving how video content is understood and retrieved.
However, while these approaches show promise, several hurdles remain. Efficiently processing massive video datasets is a major concern. Further, ensuring the reliability and accuracy of these semantic interpretations across different contexts is crucial for effective analysis and practical applications. As this field advances, it is essential to remain critically aware of the implications of these techniques and their potential for both positive and negative impacts. Navigating the complexity of automatically understanding video content requires a cautious and mindful approach.
1. **Unveiling Hidden Semantics**: Unsupervised clustering methods are increasingly vital in extracting semantic meaning from unlabeled video datasets. These algorithms, without human-provided labels, can independently pinpoint key features and underlying structures within the video data, representing a departure from traditional, label-dependent approaches.
2. **Time as a Dimension**: Video data's inherently sequential nature necessitates understanding how elements change over time. Clustering methods have begun to incorporate temporal factors, capturing the dynamic relationships between frames and enabling a more nuanced understanding of the video's semantic flow. This temporal sensitivity is crucial for accurate interpretation.
3. **Beyond the Pixel**: Researchers are exploring techniques that go beyond basic visual features, incorporating contextual cues within each frame. This enables algorithms to infer deeper meaning based on surrounding visuals, mirroring the way humans comprehend context. While promising, this contextual understanding remains an area with much potential for improvement.
4. **Simplifying Complexity**: Video data is inherently high-dimensional, making pattern recognition a daunting task. Dimensionality reduction techniques, such as t-SNE or PCA, become indispensable. By decreasing the data's complexity while preserving essential information, these methods help us see patterns that might otherwise be lost in the sheer volume of data.
5. **Multi-level Insights**: The strength of unsupervised clustering lies in its ability to analyze video at various levels. Algorithms can move beyond surface-level visual features and uncover deeper semantic meaning, recognizing relationships between different video sequences. This leads to a richer understanding of the overall video content.
6. **Security through Automation**: Anomaly detection in surveillance footage benefits greatly from unsupervised clustering. By creating a model of normal activity, algorithms can efficiently identify deviations from this baseline, potentially flagging security risks. This automated approach reduces the need for continuous human monitoring of video feeds.
7. **Cultural Variations and Challenges**: A major hurdle in semantic interpretation is the diverse range of cultural contexts that shape how humans perceive visual cues. Unsupervised algorithms struggle to account for these subtle differences, leading to the possibility of misclassification or incorrect interpretations of video content. More sophisticated models sensitive to cultural nuances are needed.
8. **Real-time Understanding**: A significant development is the ability of some clustering algorithms to perform analysis in real-time. This allows for immediate interpretations of video feeds, offering tremendous value in security and applications where timely awareness is crucial.
9. **The Impact on Viewer Engagement**: Understanding the inherent meaning of video content can have a significant impact on viewer engagement. Platforms are exploring how to utilize extracted semantic meaning to create more relevant content recommendations, potentially improving viewer retention.
10. **Navigating the Ethical Landscape**: The ability to extract hidden patterns from video data raises a variety of ethical concerns. Algorithms operating without proper oversight could potentially reinforce existing biases or misinterpret behaviors that are normal in one context but not in another. Careful consideration of ethical implications is necessary to ensure responsible deployment of these technologies.
Analyze any video with AI. Uncover insights, transcripts, and more in seconds. (Get started for free)
More Posts from whatsinmy.video: