Analyze any video with AI. Uncover insights, transcripts, and more in seconds. (Get started for free)
7 Essential Public Datasets for Training Video Classification Models in 2024
7 Essential Public Datasets for Training Video Classification Models in 2024 - Action Recognition with UCF101 Sports Dataset Containing 13,320 clips across 101 categories
The UCF101 dataset is a valuable resource for training action recognition models, containing a sizable collection of 13,320 video clips spread across 101 distinct action categories. Building upon the earlier UCF50 dataset, UCF101 expands the scope of action recognition with a diverse array of human activities, including sports, interactions, and body movements, spanning over 27 hours of video. This compilation, mainly sourced from YouTube, provides a rich variety of visual challenges, from diverse camera angles and object sizes to intricate backgrounds and lighting conditions. Researchers rely on UCF101 to rigorously evaluate and refine the performance of action recognition algorithms, thereby improving the capabilities of computer vision systems in understanding and interpreting video content. This dataset continues to be instrumental in driving advancements in automated video analysis, pushing the boundaries of what computers can achieve in this complex field.
The UCF101 Sports Dataset comprises a sizable collection of 13,320 video clips, each showcasing one of 101 distinct action categories. This makes it a valuable resource for developing models adept at recognizing specific actions within various sports settings. It's effectively a large-scale, curated library of sports action clips, ideal for training models that can understand subtle differences between sports actions.
Each video clip within the dataset has been meticulously labeled with the specific action it depicts, offering a strong foundation for supervised learning methods. This structured annotation, crucial for training models, provides a clear signal to the model about what's occurring in each clip.
While primarily sourced from YouTube, with contributions from other platforms, UCF101 highlights the possibility of leveraging publicly available videos for training action recognition models. It provides a case study of how readily available video content can be utilized to train algorithms that process and understand the dynamics of human actions.
The collection encompasses a diverse range of sports like basketball, soccer, and cricket, pushing models to differentiate between similar-looking but distinct actions. This diverse set of sports and actions makes it an excellent testbed for model development, as accuracy in recognizing diverse actions is essential for real-world applications.
One of the design choices in UCF101 was to incorporate a wide array of camera angles and shooting conditions. This deliberate diversity introduces complexity and reflects real-world scenarios, enhancing the ability of trained models to handle changes in viewpoints and environment. However, it also raises the need for models to be robust and capable of generalizing well across different visual conditions.
Interestingly, the dataset also exhibits some inherent overlap between certain action categories. It means certain actions can fall into multiple categories, posing a challenge for model discrimination. This overlap forces model developers to consider advanced techniques for learning feature representations that can accurately distinguish between similar or overlapping actions.
The UCF101 dataset provides temporal annotations for each clip. This enables researchers to study the effect of varying time scales and frame rates on model performance. Optimizing model design for action recognition across different temporal resolutions is crucial, especially when actions unfold at varying speeds.
UCF101 has been pivotal in encouraging innovation in action recognition techniques, including the development of two-stream networks. These networks process spatial and temporal information independently, demonstrating how UCF101 has shaped architectural design choices in the field of video understanding. This specific dataset, therefore, acts as a case study in the development of specific neural network architectures and helps researchers refine them for better performance on different datasets.
While the dataset is a valuable resource, it's not without limitations. There's potential for inconsistencies in action representation and, possibly, some level of 'noise' in labels. These factors could potentially limit the accuracy of models trained solely on UCF101 when applied to real-world scenarios. Therefore, relying on just one dataset can hinder robust model performance when faced with a more diverse or complex real-world application.
Due to its extensive use in research and the industry, UCF101 has fostered a substantial collection of benchmark results, offering insights into the state-of-the-art performance in action recognition. But solely focusing on UCF101 can narrow our perspective. It's crucial to explore and incorporate other datasets into training and evaluation to ensure that our models are robust and generalizable beyond this specific collection of actions. This underscores the point that broader training datasets lead to more adaptable and versatile action recognition models.
7 Essential Public Datasets for Training Video Classification Models in 2024 - Kinetics700 Dataset with 650,000 Untrimmed Youtube Videos
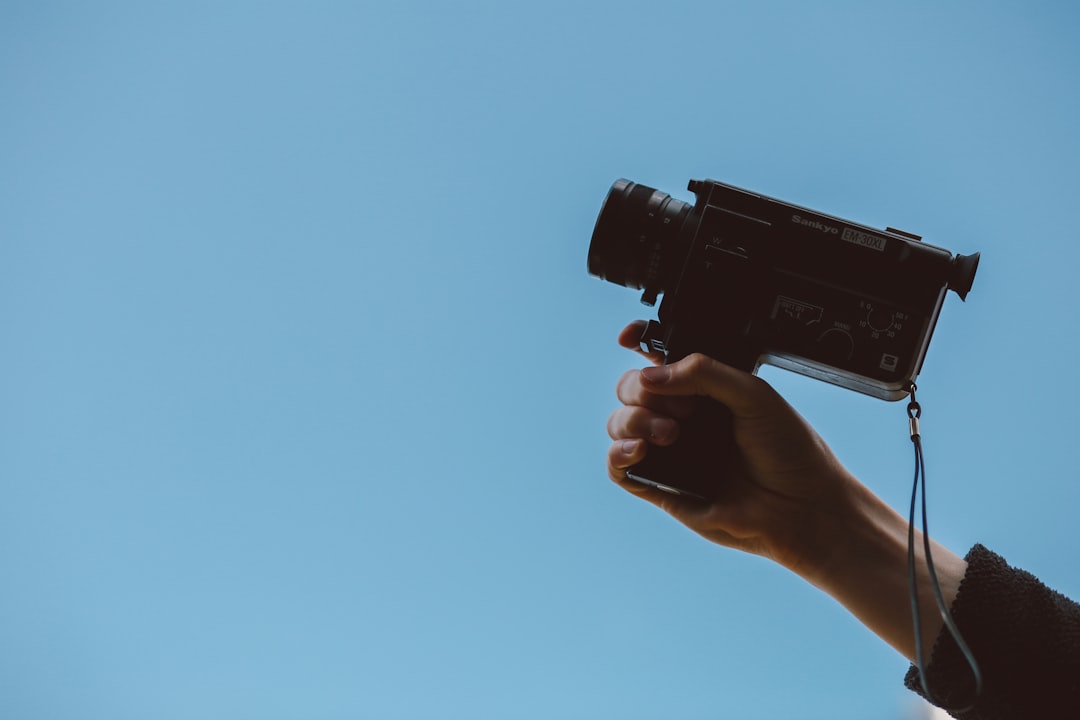
The Kinetics700 dataset is a substantial collection of 650,000 untrimmed YouTube videos, encompassing a wide range of 700 distinct human actions. Each video, lasting around 10 seconds, portrays diverse human interactions, including both object-related and person-to-person activities. This dataset is primarily designed to help improve video classification abilities in machine learning models, allowing them to better understand complex human actions.
Kinetics700 uses a WebDataset structure to help make loading data faster during training. This large dataset includes videos of various qualities and formats, giving it broad coverage of human actions. It's a natural progression from the earlier Kinetics400 and Kinetics600 datasets, and it expands the variety and quality of action classes that can be studied. Researchers can use Kinetics700 to further advance video analysis capabilities in 2024 and into the future. While useful, it's important to consider using it in conjunction with other datasets to achieve truly robust results in video understanding.
The Kinetics700 dataset, a collection of 650,000 untrimmed YouTube videos, stands out due to its impressive scale and the broad range of human actions it captures. Compared to earlier efforts like Kinetics400 and Kinetics600, it boasts a significantly larger number of action categories, making it particularly interesting for exploring finer-grained action recognition.
Firstly, with 700 distinct action classes, each represented by at least 700 video clips, the dataset provides a much richer landscape for classification than many prior datasets. This granular categorization allows us to train models capable of discerning between a wider array of human activities, fostering a deeper understanding of action dynamics.
Secondly, the inclusion of untrimmed videos is a notable feature. Unlike many datasets that focus on isolated, trimmed actions, Kinetics700 often presents videos with multiple actions occurring within the same clip. This brings a level of complexity that mimics real-world scenarios, forcing our models to handle more intricate and interconnected action sequences. We can study how temporal relationships between actions are learned by the model.
Further, the videos themselves exhibit the natural temporal unfolding of actions, allowing for analysis of action progression over time. This means that the models can be trained to recognize not just individual actions but also the sequence in which they occur. It helps us examine how models can learn the temporal dynamics and dependencies between actions within a video clip.
Since it's drawn from YouTube, the Kinetics700 dataset offers a diverse range of recording conditions, including variable lighting, camera angles, and overall video quality. This variability reflects the real-world challenges we'd expect models to encounter. It will be interesting to observe how models trained on this dataset adapt to such a wide variety of visual input.
Moreover, the sheer volume of 650,000 video clips offers a considerable advantage. The abundance of data can potentially lead to more robust and generalized models, particularly in dealing with the variation inherent in real-world videos. It also poses a significant computational challenge for processing and training.
Each video is labeled with its corresponding action, providing the basis for supervised learning. However, with such a massive dataset, the consistency and reliability of these labels across different annotators become critical factors to consider. It is possible that inaccuracies in labeling could introduce bias or noise into model training.
While predominantly visual, the presence of audio within these videos presents an opportunity to explore multimodal learning. This means we might be able to leverage both visual and auditory information to improve action recognition accuracy, potentially leading to more sophisticated and contextually aware models.
The diverse range of activities captured in the videos—from sports to everyday actions—indicates the dataset's potential for cross-domain applications. Models trained on Kinetics700 could potentially be adapted to scenarios far removed from the initial training data.
Finally, the dataset's large size and broad range of actions have already made it a standard benchmark for video classification. This has spurred the development of new model architectures and training techniques within the research community. As researchers grapple with the challenges presented by the dataset, we can expect further advancements in our understanding of video understanding.
However, the size of Kinetics700 is also a double-edged sword. It puts a tremendous computational burden on model training and evaluation. Efficient data loading strategies and hardware optimization will be critical to make full use of this valuable resource.
7 Essential Public Datasets for Training Video Classification Models in 2024 - Something Something V2 Dataset with 220,847 Hand Motion Video Clips
The Something Something V2 dataset is a collection of 220,847 video clips, each showing people performing simple actions with everyday objects. Its main purpose is to help train machine learning models to understand the nuances of human hand movements. With 174 distinct action categories, it's one of the largest datasets for evaluating models that recognize actions in videos. This version (V2) is a substantial expansion on the original (V1), which had only 108,499 clips.
Researchers can use it to build models capable of identifying a wide range of basic actions, like placing an item inside another, flipping objects over, or covering objects. This fine-grained understanding of actions is crucial for advancements in video understanding. While valuable, the dataset's immense size creates a computational hurdle when training models due to the millions of frames involved. This dataset is particularly useful for developing models that can interpret how people interact with objects through their hand movements, an increasingly important area of study in machine learning.
The Something Something V2 dataset is a collection of 220,847 short video clips, each capturing around 5 seconds of human hand actions with everyday objects. It's a valuable resource for researchers focused on the intricacies of hand gestures and their role in action recognition. The dataset breaks down these hand actions into 174 distinct categories, ranging from basic gestures to complex manipulations of objects. This level of detail allows for training models that don't just identify the action but also the context around it.
The dataset leans heavily on crowd-sourced content, primarily from YouTube, which gives it a diversity of recording styles and visual environments. However, this also means there's a wide range in video quality, lighting conditions, and backgrounds that models will need to learn to adapt to. Interestingly, the focus on hand actions sets it apart from other datasets that often capture full-body movements. It encourages researchers to create models specifically tailored to the nuances of hand gestures, a skill often overlooked in broader action recognition tasks.
Each clip in the dataset comes with meticulous annotations, providing details about the action itself, the involved object, and even the surrounding environment. This detailed labeling provides a solid foundation for supervised learning techniques. However, challenges remain within the dataset, like potential ambiguity in how certain hand motions are classified. This is because the same hand movement can be interpreted differently depending on the context. To mitigate this, researchers may need to experiment with sophisticated feature extraction techniques.
The Something Something V2 dataset is also useful for exploring multimodal learning and how hand gestures unfold over time. Models can be trained to learn these temporal patterns, which greatly improves their ability to comprehend dynamic actions. It's interesting to compare this dataset to others like Kinetics700 which focus on a much wider array of actions in less controlled settings. This comparison emphasizes how important the context and environment can be when training action recognition models.
One concern with the dataset is that its reliance on user-generated content could introduce biases in the types of hand actions represented. It might not capture the full spectrum of hand gestures across diverse cultures and interactions, something researchers should acknowledge and account for when developing models. In summary, the Something Something V2 dataset has become a significant benchmark for hand-centric action recognition. Its unique properties and challenges have pushed the development of new model architectures, particularly those focused on the delicate details of human hand interactions. This makes it a valuable asset for researchers seeking to understand the complexities of visual communication and human-object interactions through hand gestures.
7 Essential Public Datasets for Training Video Classification Models in 2024 - ActivityNet Dataset with 27,801 Untrimmed Videos for Daily Activities
The ActivityNet dataset stands out with its collection of 27,801 untrimmed videos depicting everyday activities, making it a valuable tool for training video classification models. It's organized into three separate subsets—training, validation, and testing—following a 2:1:1 ratio. ActivityNet covers a wide spectrum of 203 distinct activity types, with an average of 137 untrimmed videos for each category. Within these videos, an average of 141 activities are marked with their temporal boundaries, showcasing the intricate nature of real-world action sequences.
Beyond video classification, ActivityNet aids in understanding human activity patterns. To enhance the dataset, the ActivityNet Entities Challenge includes over 150,000 bounding box annotations connected to phrases describing the video content. Researchers can leverage this dataset for evaluating video understanding algorithms and comparing their performance. Conveniently, the dataset can be explored with the FiftyOne visualization tool, making it easier to access and analyze subsets of the data. While useful, it's important to remember that its focus on everyday actions might not fully represent the complexities seen in diverse real-world video data, possibly limiting its applicability to certain situations.
### Surprising Facts About the ActivityNet Dataset
1. **A Vast Collection of Daily Life**: ActivityNet boasts a truly impressive 27,801 untrimmed videos, focusing on the spectrum of everyday human activities. This scale presents a unique opportunity to explore a wide range of natural human behaviours beyond just isolated or pre-defined actions, potentially offering a more realistic view of how people move and interact.
2. **Precise Timing of Activities**: One of ActivityNet's distinguishing features is the detailed temporal annotations for each activity within the videos. This means that we have information about exactly when each activity starts and ends. This level of granularity is crucial for understanding how activities unfold over time and can help train models to recognise not just the activity itself but also its temporal dynamics.
3. **Real-World Videos**: The ActivityNet videos are largely pulled from sources like YouTube, offering a glimpse into real-world interactions and scenarios. This introduces a level of realism that's sometimes absent in datasets with more controlled, scripted content. This makes it a particularly good choice for researchers interested in understanding how models behave when dealing with messy, complex, and uncontrolled data, as is typical in real-world situations.
4. **A Diverse Range of Activities**: With over 200 unique activity categories, ranging from simple actions like eating to more complex ones like assembling furniture, ActivityNet is a diverse and comprehensive dataset. This is particularly important because it helps us shift away from a narrow focus on sports or very specific actions. Instead, we can explore how models can handle a much wider variety of human behaviours, paving the way for more versatile and generalisable video understanding systems.
5. **Untrimmed Videos Pose a Challenge**: The fact that the videos are untrimmed presents a unique challenge for action recognition models. They must learn to focus on the important parts of each video and filter out all the other unrelated things that happen within the same clip. This calls for more sophisticated model architectures that can effectively locate and extract the information they need within long, unconstrained videos.
6. **A Collaborative Effort**: ActivityNet benefits from ongoing contributions from the research community. This means the dataset isn't static; it evolves as researchers identify areas for improvement or new features to incorporate. This collaborative process ensures that the dataset remains relevant to the latest research questions and challenges.
7. **A Benchmark for Innovation**: ActivityNet has become a popular benchmark for evaluating action recognition models, which has helped researchers design and refine various approaches. It has been a catalyst for the development of specific techniques like temporal segment networks and two-stream networks, demonstrating its influence on the field.
8. **Beyond Visual Data**: Many of the videos in ActivityNet include audio alongside the visual content. This opens up possibilities for multi-modal learning, where models can learn to integrate information from both sources. This approach could potentially lead to models that are more robust and able to grasp a deeper understanding of activities within a video.
9. **Dataset Limitations**: Despite its advantages, researchers have highlighted some areas for caution. The diversity of video quality and potential for noise within some videos might introduce challenges for model training. This suggests that training solely on ActivityNet may not result in optimal performance when models are applied to more structured or controlled situations.
10. **Real-World Applications**: The emphasis on everyday human actions in ActivityNet has significant implications for a range of real-world applications. For example, its insights could be valuable for improving surveillance systems, assisting in elder care, and advancing human-computer interaction. This highlights the dataset's potential for designing systems that can better interact with humans in naturalistic settings.
7 Essential Public Datasets for Training Video Classification Models in 2024 - AVA Dataset with 437 15-minute Movie Sequences for Actor Analysis
The AVA dataset offers 437 fifteen-minute movie segments specifically designed to analyze actors and recognize their actions. It's notable for its annotations of 80 distinct, basic visual actions, meticulously placed within the clips both spatially and temporally. This results in a massive 159 million action labels, where multiple actions per person can be identified, highlighting common human actions. These clips are taken from uninterrupted segments of movies, making them valuable for studying how actions change over time. However, despite its detailed nature, results on the AVA dataset indicate a relatively low mean Average Precision (mAP) of only 15.8%, which suggests the task of achieving high accuracy is challenging. Focusing on individual actions can offer crucial details about human movement, yet the inherent intricacy of this dataset demands robust models to extract meaningful information.
The AVA dataset offers 437 fifteen-minute movie clips specifically tailored for analyzing actor actions and recognizing their movements within scenes. This focus on actor-centric analysis is unique, allowing researchers to build models that go beyond general action recognition and delve into understanding individual actor characteristics and behaviors within those actions.
Each of these 15-minute clips is meticulously annotated with detailed information, making it possible to train models that recognize subtle shifts in actor performance over time. This temporal granularity is a valuable feature, helping us explore how actions evolve and providing a more nuanced understanding of actor movements.
The dataset showcases a diverse range of contexts, pulling from movies with various genres and actors, resulting in a rich tapestry of visual and narrative situations. This broad representation is helpful for training models that can adapt to various settings, potentially enhancing their ability to accurately identify and interpret actions in real-world scenarios.
Furthermore, the annotation scheme employed by AVA is quite comprehensive. It not only documents the actions performed by the actors but also includes details about their interactions with others and the broader context of the scene. This detailed level of information creates a rich foundation for training models that can understand the relationships between actors and the broader context within a scene.
Each action within a clip is annotated with precise start and end times, providing valuable insights into how actions unfold over time. This allows for the exploration of how temporal patterns affect action recognition and aids in building models capable of temporal reasoning.
AVA primarily uses real movie content, which contributes to a degree of naturalism not always found in datasets with more controlled or staged content. While this offers a more realistic representation of action, it also presents challenges for model training. We need robust techniques that can adapt to the often-unpredictable nature of human behavior within real-world settings.
While primarily centered around visual content, the potential exists for incorporating audio (like dialogue or background sounds) into the analysis. This could encourage researchers to explore multimodal learning—using both visual and audio cues—and potentially lead to more insightful models that consider how sounds complement and enrich visual observations of actors.
AVA stands as a significant benchmark dataset for evaluating action recognition models. Its well-structured organization and diverse movie clips make it an ideal testbed for comparing various model architectures and algorithms, promoting innovation in the field.
One potential limitation of the dataset stems from the fact that the actions are labelled by human annotators, and consistency in labeling can be a challenge. This potential variability in perception could introduce 'noise' or inconsistencies into the data, potentially leading to model biases. Careful validation and robust training methodologies are critical to overcome this challenge.
Beyond its core function in actor analysis, the knowledge gained from training models on the AVA dataset has the potential to be applied in fields like security, sports analysis, and even human-computer interaction. This cross-domain applicability emphasizes its versatility in solving real-world problems that hinge on understanding and interpreting human action.
7 Essential Public Datasets for Training Video Classification Models in 2024 - Youtube8M Dataset with 8 Million Tagged Videos for Large Scale Training
YouTube8M is a massive dataset containing 8 million videos, each tagged with labels, designed specifically for training video classification models on a grand scale. It uses a vocabulary of 4,803 visual entities to describe the content within the videos. Human-verified annotations are provided for around 237,000 video segments across 1,000 different categories, focusing on identifying what's happening within specific segments of the videos. This large dataset is divided into training, validation, and test sets, with each category in the training set containing at least 100 videos, allowing for substantial training of machine learning models. To reduce the computing demands of working with so much video data, the dataset includes pre-calculated features from both the audio and video components of each clip. While it provides a unique opportunity to train models on a vast quantity of data, it's important to recognize that the sheer scale can also introduce its own complexities, such as the potential for annotation inconsistencies. It's often recommended that researchers supplement training with other datasets to ensure trained models perform well in a variety of conditions and situations.
YouTube8M is a vast video dataset containing 8 million videos, primarily collected from YouTube, making it a substantial resource for training video classification models. The videos are annotated with roughly 4,800 distinct visual concepts, covering a broad range of topics and themes. While the sheer size and scale of the dataset offer immense potential for training models, it also comes with a few caveats. One key aspect is its focus on user-generated content, which often leads to varying levels of quality and consistency in both the videos and their annotations. This means that models trained on this dataset might excel on similar platforms, but potentially struggle when applied to videos with higher production values or a more curated and scripted nature.
Despite this potential for noisy data, the dataset is a valuable tool for several reasons. Firstly, the breadth of visual concepts used for annotation makes it ideal for developing models that can identify and classify a wide variety of content. Secondly, while primarily built for video classification, the dataset includes information about individual segments within videos, allowing researchers to explore how activities unfold over time. This means researchers can create models that are not only sensitive to action recognition but also to the temporal context of the video.
Furthermore, the YouTube8M dataset can be customized by creating smaller, focused subsets tailored for particular tasks. This versatility addresses the needs of researchers interested in different areas of study within video classification, making it more adaptable to specific research questions. Being publicly available, the dataset encourages open collaboration and the sharing of advancements, accelerating the pace of innovation in the field. This open nature of the dataset is very valuable for the wider community and facilitates faster progress in computer vision and video understanding.
Additionally, YouTube8M offers intriguing avenues for research through its multimodal potential. The audio alongside video segments, and the possibility of associating text-based tags with the videos, allows researchers to create models that integrate multiple data modalities. This type of approach to modeling has the possibility to increase the overall accuracy and robustness of classification. Given its scale and comprehensive nature, YouTube8M has become a standard for evaluating different video classification algorithms, providing insights into the strengths and limitations of current model architectures.
It's worth noting that because of the vast quantity of videos and the ability to track them over time, the YouTube8M dataset could be used for conducting longitudinal research. Researchers could study how video content has changed over time or explore evolving trends in content creation and audience interests on YouTube. This could provide unique insights for understanding evolving media trends. Overall, YouTube8M is a compelling and complex dataset that provides a rich resource for video understanding research, presenting both immense opportunities and interesting challenges for researchers and engineers.
7 Essential Public Datasets for Training Video Classification Models in 2024 - THUMOS Dataset with 18,394 Sports Competition Videos and Timestamps
The THUMOS dataset, containing a large collection of 18,394 sports competition videos, presents a valuable resource for training video classification models, especially for action recognition. It features detailed timestamps that pinpoint specific events within the clips, providing a strong foundation for models to learn about the temporal progression of actions. The THUMOS14 subset, focusing on action recognition across 20 categories, is particularly useful for benchmarking and evaluating the performance of different models. This makes it a critical component in the ongoing advancement of research in this field.
Despite its contributions, the THUMOS dataset faces challenges, like the potential for inconsistencies in annotations and the inherent variation in performance across different models. These limitations emphasize the importance of adopting a broader approach to training and evaluation, incorporating a wider variety of datasets to develop robust and adaptable models. The THUMOS dataset, with its focus on action recognition within sports, remains an important asset for researchers looking to improve the capabilities of video analysis and computer vision systems.
The THUMOS dataset, comprising 18,394 sports competition videos with detailed timestamps, provides a unique resource for training video classification models focused on action recognition. It stands out from many datasets due to its emphasis on capturing actual sports events, which inherently involves a wider range of visual complexities compared to controlled or artificial environments.
A key feature of THUMOS is its temporal annotation – each video not only indicates the sport but also includes the precise start and end times for individual actions. This allows researchers to develop models capable of discerning dynamic action sequences, which is particularly crucial for real-time sports analytics and understanding the temporal progression of events.
Moreover, the THUMOS dataset includes a diversity of sports, ranging from individual athletics to team sports, each with its own unique set of actions. This rich collection pushes models to distinguish between subtle variations in movements, thus fostering their robustness in handling diverse action categories. Each video clip is meticulously annotated with up to 20 action classes, offering researchers the opportunity to investigate intricate actions within the context of sports, rather than relying on broad, generalized categories.
Unlike many datasets that use curated or controlled video content, THUMOS employs unedited footage from actual competitions. This introduces challenges like fluctuating lighting conditions, diverse camera angles, and varied backgrounds. This introduces realistic challenges that models need to overcome to maintain accuracy and reliability under less-than-ideal conditions. The inclusion of videos of various lengths, from short clips to extended sequences, encourages exploration of how actions evolve over time. This is particularly important in competitive sports, where understanding the temporal dynamics of actions is vital for making predictions and refining predictive models.
While THUMOS is a valuable resource, it's important to note that the large volume of videos and the process of labeling actions can introduce some inconsistency in annotation. There's a potential for subjective interpretation of actions to vary between annotators, which could impact the model's performance. To minimize these issues, thorough validation and rigorous testing strategies are crucial when employing the THUMOS dataset.
The THUMOS dataset has also played a vital role in establishing benchmarks for evaluating the performance of action recognition models. The intricate nature of actions within sports has spurred advancements in spatial and temporal modeling techniques, fostering progress within the broader field of video classification. While the dataset is primarily centered on visual content, the presence of audio commentary and spectator reactions presents an avenue for multimodal learning approaches. Incorporating audio cues alongside the visual information could potentially enhance the accuracy and contextual understanding of action recognition models.
Looking ahead, the comprehensive nature of THUMOS makes it a fertile ground for future research. Its focus on competitive actions could potentially lead to new advancements in sports analytics, performance evaluation, and perhaps even in innovative coaching applications that leverage automated analysis of visual content. The potential is there for expanding on standard machine learning applications and exploring how this unique dataset can further enhance our understanding of sports and competitive action.
Analyze any video with AI. Uncover insights, transcripts, and more in seconds. (Get started for free)
More Posts from whatsinmy.video: