7 Hidden Video Metadata Datasets That Machine Learning Researchers Actually Use in 2024
7 Hidden Video Metadata Datasets That Machine Learning Researchers Actually Use in 2024 - YouTube8M Video Dataset With Pre-Computed Features From 2M Labeled Videos
The YouTube8M dataset offers a vast collection of 8 million YouTube videos, each tagged with machine-generated annotations spanning 4,800 visual categories. This dataset is unique in its inclusion of pre-computed audio and visual features derived from a massive 19 billion frames and audio segments, making it easily manageable on a typical storage device. Notably, YouTube8M represents a significant leap forward compared to earlier datasets like Sports1M, showcasing a much larger scale and a wider variety of content. It's carefully structured into training, validation, and testing subsets, ensuring that each classification category contains at least 100 videos, leading to more reliable model evaluations. YouTube8M, with its comprehensive features and robust annotation process, has emerged as a standard benchmark for pushing the boundaries of video understanding and classification in machine learning research. While its sheer size is impressive, questions remain about the quality and consistency of the automatic annotation process which could impact model performance. Researchers will likely need to explore various pre-processing techniques to achieve optimum results depending on their specific research goals.
The YouTube8M dataset, released by Google, offers a massive collection of 8 million YouTube video IDs paired with pre-computed features derived from 2 million labeled videos. It's a significant resource for video understanding and classification research due to its sheer size and the diverse range of video content it encompasses. This size and diversity give researchers a wealth of audio and visual information to explore – a substantial jump from earlier datasets like Sports1M.
The pre-computed features extracted from billions of video frames and audio segments, designed to fit on a single storage device, make the data accessible and manageable, reducing the computational burden often associated with working with raw video data. Each video is linked to a variety of descriptive labels, which allows researchers to train models for multi-label classification, a task becoming more critical with the increasingly intricate nature of modern online video content.
A unique characteristic of YouTube8M is its detailed level of annotation: it offers not only typical features but also frame-level and video-segment level information, providing flexibility and depth for analysis. The interplay of these spatial and temporal aspects of video enables researchers to delve into how diverse video components affect the classification outcome.
It's important to acknowledge that a dataset this large, based on real-world YouTube content, carries potential biases, as popular video genres tend to be overrepresented. This can skew research results if models are trained relying on a balanced distribution of data across applications. However, this dataset also bridges the gap between research and industry applications, offering possibilities in areas such as video recommendations and automatic content tagging.
Despite its usefulness, limitations are inherent to the dataset. For example, some videos lack complete labels, requiring researchers to account for this during model training and evaluation. The open access nature of the dataset fosters collaboration but simultaneously prompts us to address potential reproducibility issues and the ethical considerations associated with propagating biases present in the original data. Researchers must remain mindful of these aspects when working with such a significant, but also imperfect, resource.
7 Hidden Video Metadata Datasets That Machine Learning Researchers Actually Use in 2024 - MIT Moments in Time Dataset For Action Recognition Across 339 Categories
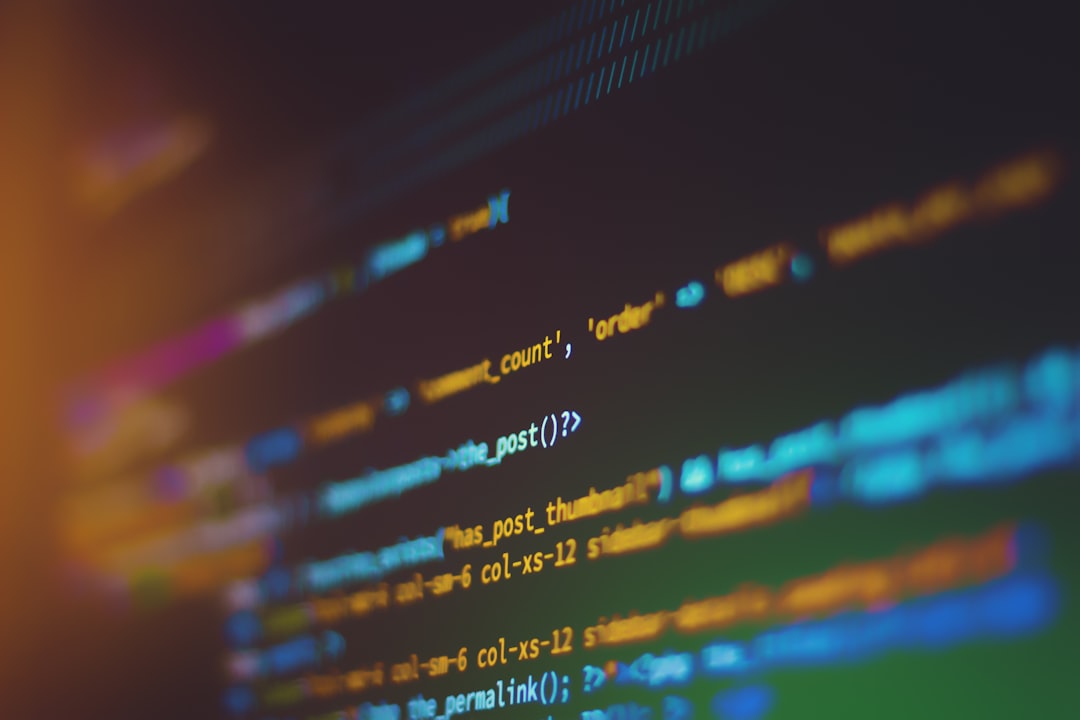
The MIT Moments in Time Dataset is designed to push the boundaries of action recognition in artificial intelligence. It comprises a substantial collection of over a million labeled, three-second video clips, each depicting a specific action. These videos cover a broad range of 339 distinct action categories, encompassing human actions, natural occurrences, animal behaviors, and objects, effectively providing a diverse representation of dynamic real-world events. While each video is tagged with a single dominant action, the presence of multiple actions within the same clip adds a layer of complexity to the task of recognizing and classifying actions.
One key feature of this dataset is its demonstration of significant variations within each action category, showcasing the inherent complexity and diversity of actions that AI systems need to learn to interpret. This complexity underscores the importance of robust machine learning models that can handle the spatial, audio, and temporal aspects of short videos. The dataset essentially aims to serve as a benchmark for developing advanced algorithms capable of abstract reasoning and understanding the intricate nature of real-world actions. By providing a large-scale, human-annotated dataset, Moments in Time emphasizes the critical role of such resources in advancing action recognition research within the machine learning field. It seeks to provide researchers with a robust dataset that goes beyond the scope of traditional datasets, aiming to drive progress in understanding and interpreting events from video data.
The MIT Moments in Time dataset offers a rich collection of over a million labeled videos, each spanning just three seconds, focusing on a diverse range of 339 action categories. This makes it a valuable resource for understanding how AI systems can recognize actions within short video clips. The compressed timeframe, however, presents a challenge in capturing the full temporal context of each action, requiring models to efficiently learn from limited information.
This dataset's strength lies in its ability to capture real-world events, including human-environment interactions and a diverse array of actions, from everyday occurrences like cooking to less frequent events, providing a realistic view of action diversity. This variety is key for developing models capable of generalizing beyond the training data and performing well on a broader range of scenarios.
The abundance of action categories opens up possibilities for transfer learning, where models trained on this dataset can be applied to other computer vision tasks. The sheer variety and size of the dataset potentially enables extracting powerful features that can translate to other related problems.
Unlike some datasets that focus on a single domain or category, Moments in Time presents a wider spectrum of actions and scenes, including natural events and object interactions. This variety helps to build more robust models capable of dealing with a broader range of real-world scenarios.
Human annotation ensures higher accuracy in the labeling process, a key advantage over automatically generated labels. This increased accuracy improves the quality of model training, as mislabeled data can hinder performance. But, there is a trade-off as human labeling is more time-consuming and can still introduce some inconsistencies.
However, the dataset's varied categories also raise the problem of class imbalance. Certain actions may be much more frequent than others, introducing biases if not properly addressed during model training. Addressing this requires specific strategies, such as data augmentation or using weighted loss functions.
One aspect that makes the dataset interesting is the varying levels of motion complexity seen across its video clips. From subtle movements to dynamic interactions, researchers can gain insight into how different motion patterns affect action classification.
Beyond human actions, the dataset also includes objects and their interactions, making it more complex and adding the potential for developing models using multimodal learning. These models learn to utilize visual and auditory features to better understand the actions.
The development of this dataset aligns with a trend in AI research focused on sequence understanding, encouraging development of models capable of recognizing actions based on the temporal flow of events within the videos, rather than relying solely on individual frames.
The wide variety of actions within the dataset presents unique opportunities for research into hierarchical action recognition. This allows models to recognize actions at different levels of abstraction – from general categories to very specific details, potentially leading to more refined understanding in machine vision systems. It's important to note, however, that this granularity may come at the cost of additional complexity in model architecture and training procedures.
7 Hidden Video Metadata Datasets That Machine Learning Researchers Actually Use in 2024 - Kinetics700 Human Action Video Dataset By DeepMind With 650k Clips
DeepMind's Kinetics700 dataset provides a substantial collection of 650,000 video clips, each depicting one of 700 different human actions. This makes it a valuable resource for researchers working on the challenging task of recognizing human actions in video data. Each action is represented by at least 700 video clips, ensuring a good variety and sufficient data for model training.
The clips, typically around 10 seconds long, originate from various YouTube videos, creating a rich and diverse range of human activities, encompassing interactions between people and interactions with objects. The dataset emphasizes actions centered around humans, providing a broad perspective on human behavior captured on video.
Researchers can leverage the dataset's public validation set, which includes pre-labeled clips, to easily assess the performance of their models. The Kinetics700 dataset, with its large size compared to earlier iterations, is a major step forward and has proven to be a very useful tool for the machine learning community. Its focus remains centered around human activities and it continues to play a vital role in advancing research on video analysis and understanding the nuances of human behavior. However, it's important to acknowledge that relying solely on YouTube videos for data can potentially introduce biases into the training process, something researchers will want to consider in their work.
DeepMind's Kinetics700 dataset offers a substantial collection of 650,000 video clips, each illustrating one of 700 distinct human actions. This makes it a valuable resource for exploring human action recognition within videos. Each clip, typically lasting about 10 seconds, captures a good balance between temporal context and manageable data size for training. The videos, mostly sourced from YouTube, showcase a variety of real-world settings and behaviors, offering a more naturalistic approach to action recognition compared to datasets that often rely on scripted scenarios.
The annotations, extending beyond simple action labels, delve into a diverse range of over 70,000 individual actions, offering a finer granularity for researchers aiming to build more comprehensive and accurate models. However, the dataset also has a significant challenge in terms of class imbalance, with some actions appearing far more frequently than others due to their popularity on platforms like YouTube. This poses a risk of models overfitting to the most common actions, potentially hindering their generalizability to less frequent scenarios.
Researchers can use Kinetics700 to assess their models against a standardized set of actions, allowing for better comparison across different model architectures and performance evaluation. Furthermore, isolating actions allows for investigation into hierarchical action recognition, where models can learn to break down complex actions into simpler steps, possibly leading to better understanding of intricate human behavior. The dataset offers a spectrum of action complexities, from simple movements to sophisticated coordinated actions, driving researchers to develop models that can handle this variability.
The dataset's focus on temporal continuity of actions also promotes research into algorithms that leverage sequences of video frames rather than just analyzing individual images to understand the context of the actions. However, since it utilizes real-world content, including YouTube videos, it's crucial to acknowledge inherent biases in the data regarding both the representation and frequency of actions. Researchers need to employ strategies to mitigate potential biases during model training and avoid creating models that are skewed towards the most frequently encountered actions in the dataset. This dataset's size and focus on real-world data make it a valuable yet complex resource for the study of action recognition, prompting researchers to explore new ways to train models for effective and unbiased analysis.
7 Hidden Video Metadata Datasets That Machine Learning Researchers Actually Use in 2024 - Sports1M Dataset With 1 Million Sports Videos And 487 Classes
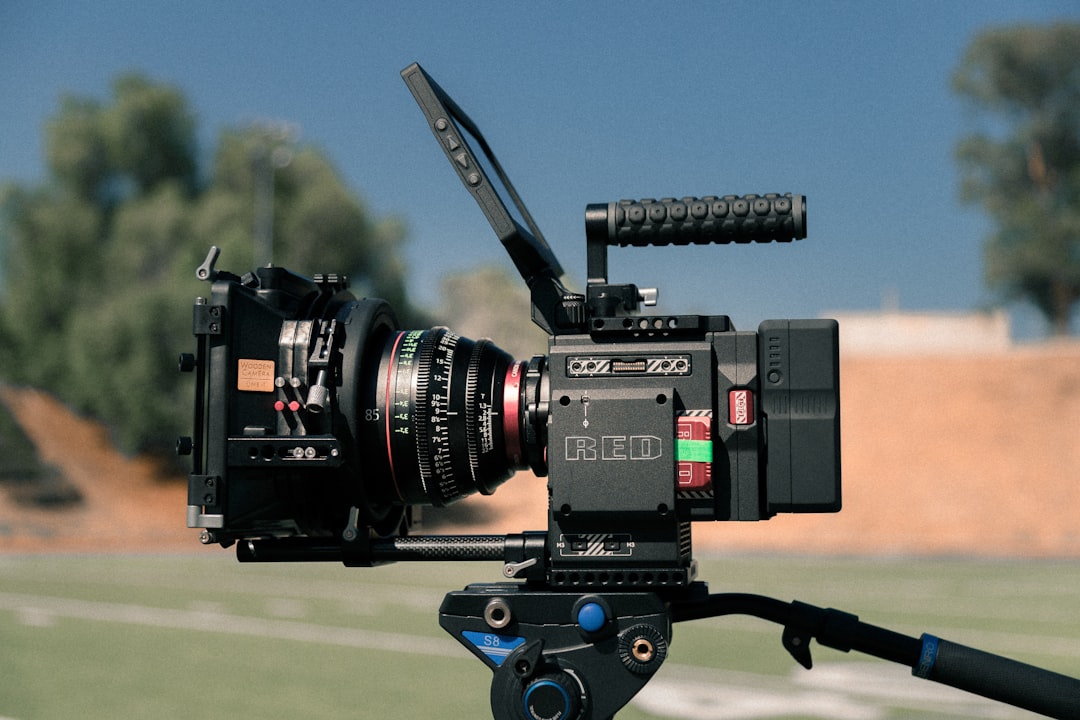
The Sports1M dataset offers a vast library of over 1 million sports videos, each tagged with one of 487 unique sport categories. This extensive dataset, largely sourced from YouTube, has proven instrumental in advancing research within the field of large-scale video classification. While approximately 7% of the original videos were removed by their uploader after the dataset was compiled, the remaining content remains a significant resource. The dataset's design includes specific preprocessing steps, including resizing to 92x128 pixels and then further adjusting the frame size with center-cropping to 92x121 pixels. These processing steps are critical for enabling the dataset's efficient use in machine learning tasks.
Researchers are particularly drawn to Sports1M's potential in refining video action recognition methods. Current benchmarks for performance on this dataset are being set by models like GBlend. The Sports1M dataset acts as a valuable benchmark for researchers in the computer vision space, offering a standardized collection for the testing and improvement of video classification algorithms. It's facilitated countless experiments and model evaluations, helping to push forward the boundaries of this domain. It remains a freely available resource, encouraging broader participation in video classification research.
The Sports1M dataset comprises a million sports video clips, each categorized into 487 different sports categories, providing a broad foundation for training and testing machine learning models designed for intricate video classification tasks.
Unlike simpler datasets that primarily use static images or audio labels, Sports1M videos capture the dynamism of sporting events. This dynamic nature exposes the algorithms to the temporal dimension which is crucial for comprehending motion and the context within a video.
Initially gathered from user-uploaded content on YouTube, Sports1M has faced some scrutiny due to potential copyright concerns. Relying on user-generated content can introduce biases, as certain sports or actions might be overrepresented due to their popularity.
This dataset covers a wide spectrum of sports, ranging from prominent leagues like basketball and soccer to more specialized sports like fencing and wrestling. This diversity allows for training models across a range of sports, although it makes the task of achieving consistent performance across the board more difficult.
Each video in Sports1M is around 5 seconds long, attempting to balance the need for adequate context with manageable file sizes. The short duration, however, could limit the capacity of models to capture important events leading up to and following actions within the videos.
Sports1M is a valuable standard for evaluating various video analysis challenges like action recognition and classifying videos with multiple labels. However, it’s size and complexity can generate significant computational requirements during training.
The Sports1M dataset displays class imbalance, a common challenge in datasets. Some sports might be represented more frequently than others, requiring careful model assessment to prevent biased evaluation and potentially affecting the overall accuracy of models.
Interestingly, Sports1M is frequently used to improve transfer learning capabilities. Models trained on it can be fine-tuned for other tasks related to sports and video analysis, showcasing its adaptability.
Human annotations were included in the original dataset design, leading to more accurate labels compared to datasets relying on entirely automated annotation. However, this increases the processing time and might introduce human bias, resulting in inconsistencies across the data.
The success of a model trained on Sports1M depends not just on its architecture, but also on effective techniques for dealing with common issues that are present in sports footage, such as noise and partial obstructions within the video.
7 Hidden Video Metadata Datasets That Machine Learning Researchers Actually Use in 2024 - ActivityNet200 Dataset With 200 Human Activity Classes And 849 Hours Of Video
The ActivityNet200 dataset offers a substantial collection of 849 hours of video data, making it a valuable resource for researchers working on human activity recognition. With 200 distinct activity categories, it covers a wide spectrum of everyday tasks, each represented by an average of 137 untrimmed videos. This focus on untrimmed videos is notable as it pushes researchers beyond the limitations of simpler, pre-defined video segments often used in benchmark datasets. The dataset promotes the development of more sophisticated temporal activity detection algorithms that can handle the complex and diverse nature of real-world activities.
The dataset's structure includes a taxonomy that utilizes parent-child relationships, which helps in organizing and classifying activities in a logical way. It also serves as a benchmark for evaluating different computer vision algorithms, providing a common set of challenges that can be used to compare various model performance. Ultimately, ActivityNet200 aims to drive advancements in machine learning and computer vision by encouraging the creation of more robust models capable of accurately understanding complex, ongoing activities within the context of longer video clips. While this dataset offers a unique opportunity for researchers, it's important to be aware that the diverse nature of the video content can create certain difficulties and may require careful model design to fully utilize.
ActivityNet200 offers a rich collection of 200 distinct human activity classes, which is significant because human behavior can be incredibly complex and varies widely depending on the context. It's a valuable resource for researchers who are trying to build AI models that can recognize and understand this intricate behavior.
With a massive 849 hours of video footage, ActivityNet is one of the largest datasets available for human activity recognition. This huge amount of data allows researchers to develop models that can learn from a wider array of real-world activities. Instead of being limited to controlled or pre-scripted environments, the dataset encourages researchers to focus on more natural and complex activities.
The dataset uses two-minute video clips for each activity class. This structure balances the need to capture detailed information about the activity with a manageable data size for processing. It allows the models to learn and understand more about how actions unfold over time.
ActivityNet200 stands out because it has a built-in mechanism for analyzing the timing and duration of activities in addition to the usual qualitative labels. This temporal grounding adds an extra layer of precision to video understanding, which is useful for tasks where it's important to know exactly when and for how long an action takes place.
The dataset is primarily structured for video-level annotations, meaning that each video can be associated with multiple action classes. This captures the fact that real-world human activities can be multi-faceted and sometimes overlap. It's a more realistic approach than some datasets that have a simpler one-to-one mapping between videos and actions.
While this dataset is substantial, there's a common problem in this kind of work, class imbalance. Some activities are naturally more represented in the dataset due to their prevalence on sources like YouTube. If not handled carefully, this can bias models, leading to a skewed understanding of activity frequencies and potential limitations in model performance on less common activities.
The activities included in ActivityNet200 are quite diverse, spanning many common everyday occurrences, such as cooking, working out, or other daily tasks. This makes it useful for training models that are capable of handling a variety of contexts and adapting to the real-world situations they may encounter during application.
The dataset was built using a mixture of human annotations and automated labeling processes. This hybrid approach allows for the large scale but also potentially introduces some biases or inconsistencies into the labeling process itself. These potential issues are something researchers should keep in mind when working with this resource.
One challenge that ActivityNet shares with many video datasets is recognizing activities that are partially occluded or change rapidly. These types of challenges require algorithms that are robust enough to deal with dynamic and less-than-perfect video conditions that are common in real-world footage. This presents a research opportunity to further improve the ability of algorithms to understand more complex and dynamic video scenes.
Similar to other video analytic work, the dataset can be used for a variety of tasks, from supervised learning (where the model learns with labeled examples) to unsupervised learning (where the model tries to discover patterns on its own). This ability to use ActivityNet for both types of learning techniques makes it flexible and fits into the evolving landscape of video data analysis and a greater variety of machine learning approaches for video data.
7 Hidden Video Metadata Datasets That Machine Learning Researchers Actually Use in 2024 - Something Something V2 Dataset With 220k Videos Of Basic Object Interactions
The Something Something V2 dataset consists of 220,000+ short video clips, all focused on the fundamental ways people interact with everyday objects. This dataset is particularly useful for training AI models to understand very detailed hand movements and actions, such as "putting something into something" or "turning something upside down." This focus on basic interactions helps bridge the gap between visual perception and understanding common sense reasoning about the physical world.
While many existing video datasets focus on broader, more general categories, Something Something V2 dives into a more nuanced level of human activity. This is important because it addresses limitations in current models that struggle to interpret the fine-grained details of physical actions. The videos are a useful training resource for building AI models capable of object classification and understanding intricate interactions within scenes, going beyond what was previously achievable with datasets like ImageNet. Its straightforward structure makes it a relatively easy starting point for researchers wanting to tackle these complex machine learning problems. Researchers looking to improve machine learning models in their ability to comprehend and predict human actions will find the Something Something V2 dataset a valuable tool.
The Something Something V2 dataset offers a sizable collection of 220,000 video clips, each showcasing simple interactions between people and everyday objects. This makes it a useful resource for developing machine learning models that can understand how objects are manipulated in dynamic scenarios. Interestingly, the dataset categorizes interactions based on both the objects and the context in which they're used. This leads to a much more granular understanding of object relationships across different situations, which can be helpful for developing more robust models.
One of the advantages of Something Something V2 is its meticulous human annotation, which contributes to a high level of labeling accuracy. This is a significant improvement compared to datasets with automatic labels and can make a real difference in the reliability of training and evaluating machine learning models. Because the video clips display object interactions as they occur over time, researchers can examine how actions evolve, a critical aspect for grasping complex behaviors in real-world situations.
This dataset presents a strong opportunity to explore multi-modal learning techniques. Since the videos might include audio alongside the visual data, researchers can potentially develop models that understand interactions in a more holistic manner, potentially improving model interpretation as audio may provide extra contextual information.
The diversity of actions captured in the dataset, ranging from simple actions like holding and passing to more elaborate multi-object interactions, provides a good test bed for training models that can generalize well across many different action types. The interactions captured are designed to mirror typical situations we encounter in everyday life. This aligns the training process more closely with actual real-world conditions, which could potentially lead to models that perform better in uncontrolled environments.
However, the dataset isn't without its challenges. The vast range of interactions may result in a class imbalance issue. Some interaction types will be far more common in the video clips, which can lead to biases in the trained models. If not handled carefully during training, this can impact how well the model recognizes less frequent interaction types.
This characteristic makes it potentially useful for transfer learning applications. Models trained on this dataset could serve as a starting point for fine-tuning on related tasks, using the knowledge of basic object interactions as a stepping stone to a broader video analysis goal. The fact that the dataset features partial object occlusions is another factor to consider. In a way, this makes it more realistic but also means that advanced recognition techniques will be needed to handle scenes where objects are only partially visible or change rapidly. This type of challenge can drive the development of more robust algorithms capable of interpreting dynamic scenes and understanding object relationships even when those relationships are not fully observable.
7 Hidden Video Metadata Datasets That Machine Learning Researchers Actually Use in 2024 - VGG Sound Dataset With Over 200k Audio Clips From 300 Categories
The VGG Sound dataset provides a large collection of over 200,000 audio clips spanning 300 distinct sound categories. Each clip is roughly 10 seconds long and comes with a corresponding video, ensuring the sound source is visually identifiable. This dataset encompasses a diverse range of challenging acoustic scenarios and noise types, making it relevant to real-world audio processing. The clips are primarily gathered from YouTube videos, with a focus on minimizing inaccuracies in the sound labels. With a combined total of around 550 hours of video and audio data, the VGG Sound dataset is primarily intended to support the development and testing of machine learning models specifically focused on audio classification. While valuable, it's important to note that the use of publicly available YouTube videos for sourcing the data could lead to biases in the data, a factor researchers should consider in their studies. It's a significant dataset for researchers interested in exploring and advancing machine learning and audio analysis techniques.
The VGG Sound dataset offers a collection of over 200,000 audio clips spanning 300 different sound categories, providing a diverse representation of the auditory world. This variety, encompassing everything from everyday sounds to more specific audio events, makes it a promising resource for training sound recognition models. It's noteworthy that it captures not just musical sounds, but also everyday occurrences like animal noises, human activities, and various environmental sounds, offering a broader scope than datasets focused purely on music.
While the source of the audio clips is diverse – including movies, documentaries, and YouTube videos – this variety introduces potential complexities for maintaining consistent labeling across different sound categories. There's a unique opportunity here to investigate the interaction of sound and visual context, since the dataset includes the accompanying video clips. Researchers could, for example, investigate how sound impacts the visual interpretation of the scene in multi-modal learning settings.
One can envision applying data augmentation techniques to expand the dataset's utility by introducing variations such as adjusting pitch, speed, or adding noise and reverberation. This could improve model robustness and enhance performance in a broader range of audio environments. However, dealing with a dataset of this size can introduce substantial computational challenges for researchers. It requires a thoughtful approach to data management and model training to optimize for efficiency.
The manual annotation process for labeling sound clips, while enhancing the accuracy of the classification compared to automatically-labeled datasets, could also introduce some human error. This means researchers must pay attention to potential inconsistencies and errors when curating data. We can imagine that the pre-trained models derived from VGG Sound could be used for transfer learning in specific audio recognition tasks. This could potentially enable new breakthroughs in creating real-world sound recognition applications.
An important consideration is the class imbalance that's likely present, with some sound categories far more abundant than others. This requires careful attention during model training to avoid biases towards the more frequently represented sounds. Moving forward, we can expect that machine learning advancements using the VGG Sound dataset will have impacts on fields like robotics and interactive systems. Understanding and responding to the audio environment is a crucial part of developing truly effective and user-friendly robotic and interactive applications.
More Posts from whatsinmy.video: