Analyze any video with AI. Uncover insights, transcripts, and more in seconds. (Get started for free)
Analyzing Goodfellow's Deep Learning Key Insights for Video Content Creators in 2024
Analyzing Goodfellow's Deep Learning Key Insights for Video Content Creators in 2024 - GAN Applications for Video Generation in 2024
The application of Generative Adversarial Networks (GANs) for crafting videos has become increasingly sophisticated in 2024. These models are now capable of generating videos with a remarkably human-like quality by drawing upon large collections of real-world video data to understand the intricacies of human-made content. However, some key issues persist. Maintaining consistent temporal relationships, especially synchronizing lip movements with audio, remains a difficult problem. The resulting videos sometimes exhibit unnatural or awkward postures, highlighting the need for further development in deep learning approaches to address this shortcoming.
Despite these limitations, recent progress in unsupervised deep generative models holds promise for improving the fidelity of generated videos. Researchers are exploring novel frameworks, such as Dynamic Generative Models, to tackle specific video generation tasks, like producing clearer and more nuanced sign language videos. It's worth noting that the creation of compelling video content using GANs depends heavily on the availability of extensive training data. Often, these datasets are repurposed from other areas like action recognition, which may limit the quality of the resulting videos. Ongoing research, such as exploring Recurrent GANs for producing videos without specific constraints, suggests that GANs will continue to play a crucial role in pushing the boundaries of video generation in the near future.
Generative Adversarial Networks (GANs) have shown impressive potential for video generation, particularly in 2024, pushing the boundaries of what's possible in automated content creation. While initially applied to image enhancement, they've evolved to generate entire videos from simple text descriptions, suggesting a strong leap forward in encoding visual information.
Recent developments in GAN architectures have resulted in significantly better temporal consistency, leading to smoother, more believable video output. This improvement tackles the issue of choppy or inconsistent movement that plagued earlier iterations, paving the way for more realistic-looking content. However, the ability to generate hyper-realistic videos does bring up important questions regarding the authenticity of digital media.
We're now seeing GANs implemented across a variety of fields. In filmmaking, for instance, they offer a powerful tool for pre-visualization, allowing directors to experiment with different scene compositions and visual effects prior to actual production. The possibility of manipulating scenes in digital environments could revolutionize the creative process, especially in reducing costs and maximizing creative exploration.
Beyond entertainment, GANs are finding a place in academic research. Researchers are leveraging them to generate synthetic training data for machine learning algorithms. This provides a safer and more efficient way to test algorithms in complex scenarios without relying solely on real-world datasets. This is particularly important in fields where real-world data can be difficult or dangerous to collect.
In the realm of video games, the use of GANs is growing. Game developers are exploring them for automated level generation and character animation, streamlining the development pipeline and enabling more dynamic in-game adjustments. This has the potential to revolutionize game design, allowing for much more flexible and customized game experiences.
Similarly, there's growing interest in personalized marketing applications of GANs. The possibility of tailoring video ads to individual viewers is appealing, though it also raises concerns about potential misuse of user data.
Despite these achievements, challenges still persist. Achieving consistent and realistic audio-visual synchronization remains a major hurdle, emphasizing the ongoing need for advancements in audio-video generation to create a truly immersive viewing experience. There is still much work to be done in this area. It will be interesting to see what innovative solutions emerge as research continues.
Analyzing Goodfellow's Deep Learning Key Insights for Video Content Creators in 2024 - Transfer Learning Techniques for Content Adaptation
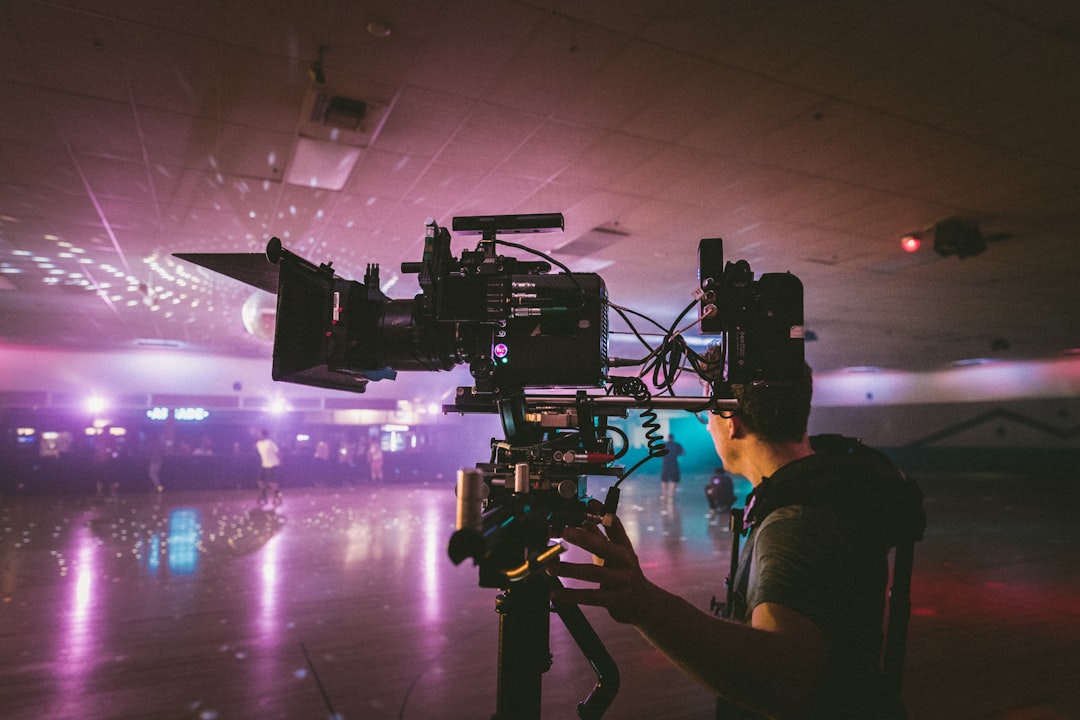
Transfer learning has emerged as a crucial tool for adapting content across different domains, particularly in scenarios where labeled data is scarce. By harnessing the knowledge acquired from pre-trained models, video creators can improve model performance and swiftly adapt to new content creation tasks, circumventing the need for extensive retraining from scratch. Techniques like fine-tuning, feature extraction, and data augmentation are instrumental in effectively transferring previously learned features to new contexts, allowing models to better generalize within the wide array of video content creation scenarios. The evolving field of deep domain adaptation, with its focus on applying prior knowledge, provides promising avenues for creators to improve their workflow and output quality, despite the inherent challenges of adapting to varying data sources. Furthermore, the incorporation of gradient-based meta-learning techniques indicates a trend towards more efficient model adaptation, potentially leading to even greater strides in content generation and tailored output for specific audiences. While promising, the efficacy of these techniques can vary depending on the specific application and the quality of the initial pre-trained model, making a nuanced understanding of transfer learning's strengths and limitations important for creators.
Transfer learning offers a compelling approach to reduce the computational burden and data requirements for training models, particularly valuable for content creators with limited resources. A well-trained model can often achieve impressive results with just a few labeled examples from a new task. This efficiency is a huge benefit, allowing creators to explore new content directions without needing to start from scratch each time.
Domain adaptation, a core concept in transfer learning, enables models initially trained on one type of content, say images, to be effectively adapted for another domain like video. This capability promotes efficiency by leveraging existing models to solve diverse content creation challenges, which can be useful in scenarios like content adaptation for various platforms.
Transfer learning empowers video content creators to react swiftly to evolving trends and audience tastes. Using pre-trained models, creators can seamlessly adapt content styles without the need to rebuild their entire model pipeline. This dynamic adaptation allows for greater flexibility and creativity in content development.
The process of fine-tuning within transfer learning involves more than simply tweaking parameters. It also offers the potential to incorporate layers that are task-specific, enhancing the model's performance for particular video generation goals. This might include adapting the content for a specific demographic or crafting a particular stylistic approach.
One interesting aspect of some transfer learning methods is the concept of "knowledge distillation." Here, a smaller, more efficient model learns to mimic the behavior of a larger, pre-trained model. This results in faster inference times without significantly compromising performance, potentially useful in real-time video processing applications.
However, the transfer learning process isn't without potential drawbacks. Negative transfer, a phenomenon where a pre-trained model performs worse after being fine-tuned on a new task, can occur if the source and target tasks are too different. This issue emphasizes the critical role of understanding the new task domain in order to minimize performance degradation.
In the context of video content adaptation, transfer learning strategies can leverage temporal patterns from one dataset to improve the handling of motion sequences in another. This reveals the critical importance of understanding how the passage of time impacts visual storytelling and how this understanding can be applied across different video datasets.
Transfer learning techniques can be particularly helpful in ensuring narrative consistency across multiple platforms. By intelligently adapting the content format while preserving the underlying message, these techniques can maximize reach while maintaining the core elements of the story.
While promising, it's crucial to recognize that transfer learning isn't a panacea for all content creation challenges. The success of these techniques is strongly linked to the quality and relevance of the data used to pre-train the model. This underscores the importance of thoughtful data curation when employing transfer learning techniques.
Current research in transfer learning for video content is exploring multimodal learning methods. These methods allow models to simultaneously consider visual and audio cues from diverse sources, ultimately leading to a more contextually rich and nuanced understanding of the content. This multimodal approach suggests a potential future direction for increasing the sophistication of video content generation using deep learning methods.
Analyzing Goodfellow's Deep Learning Key Insights for Video Content Creators in 2024 - Automated Video Editing Using Deep Learning Models
Automated video editing, powered by deep learning models, is transforming how content is created in 2024. Deep learning methods are being used to automate tasks previously handled by humans, like creating concise video summaries using neural networks to extract the most important parts of long videos. This addresses challenges related to the time-consuming nature of manually editing large amounts of footage. New models like Contextual and Multimodal Video Editing (CMVE) are exploring ways to incorporate both visual and text-based data to learn and apply various editing styles, improving the automated editing process. While AI-powered editing can be extremely efficient, it also introduces questions about its ability to truly capture the subtleties of emotional storytelling and artistic choices which are often a core part of the human creative process. As these models continue to develop and become more sophisticated, we can anticipate improvements in the speed and efficiency of video editing. However, it's important to also consider the broader implications for the nature of video creation and the role of human artistry in the process.
While deep learning has made significant strides in automating video editing, there are still challenges to overcome. For example, ensuring smooth and natural motion in edited videos remains a hurdle, even with models like Recurrent Neural Networks designed to handle temporal relationships. Maintaining consistent movement without introducing jarring artifacts is a continuous area of improvement.
The performance of these AI-powered editors is heavily tied to the diversity of the training data they are exposed to. If the training dataset is limited, the model might struggle to adapt to diverse video styles and editing scenarios, leading to less versatility and broader applicability. This reliance on high-quality, varied data is a crucial factor in optimizing performance.
Interestingly, researchers are exploring the use of reinforcement learning in some systems. These systems incorporate feedback loops that adjust the edits based on how viewers engage with the video, creating a dynamic interaction between model and audience. This approach suggests a potential shift in the way editors will interact with video editing workflows in the future.
Additionally, emotion recognition technology is being integrated into some video editing tools. By analyzing viewer emotional responses, these systems can fine-tune edits to maximize emotional impact. This capability highlights the evolving role of deep learning in crafting videos tailored for audience engagement.
However, the increasing complexity of these models presents a new set of challenges, namely increased computational demands. High-resolution video editing in real-time requires significant processing power, pushing the need for advancements in hardware and efficient algorithms. Meeting the demand for fast and responsive editing tools for high-definition content necessitates addressing these computational limitations.
One of the areas showing significant progress is the automation of transitions between different scenes. By leveraging narrative comprehension, models are learning how to create fluid transitions, potentially streamlining editing processes, particularly when dealing with various types of footage.
Deep learning models are increasingly capable of tailoring video edits to individual viewers' preferences. By analyzing user viewing habits, AI-powered systems can adjust video edits to better align with personal tastes. While offering great potential for personalized experiences, this raises critical questions about privacy and responsible data usage.
Another intriguing development involves leveraging generative models not only for selecting video segments but also for creating entirely new scenes. AI-generated segments can seamlessly integrate into existing edits, allowing creators to fill gaps or enhance scenes with visuals that match the overall style and mood of the video.
Models are becoming more sophisticated, moving beyond simple visual cues and incorporating a deeper semantic understanding of the video content. This semantic understanding allows AI systems to maintain narrative flow and enhance storytelling through video edits, adding a more nuanced layer to video creation.
The ever-increasing capabilities of automated video editing present ethical concerns related to content authenticity. The ease with which AI can manipulate videos raises questions about misinformation and deception. Establishing ethical guidelines for video content creation becomes more important as AI-powered editing becomes increasingly prevalent in digital media.
Analyzing Goodfellow's Deep Learning Key Insights for Video Content Creators in 2024 - Natural Language Processing for Script Generation
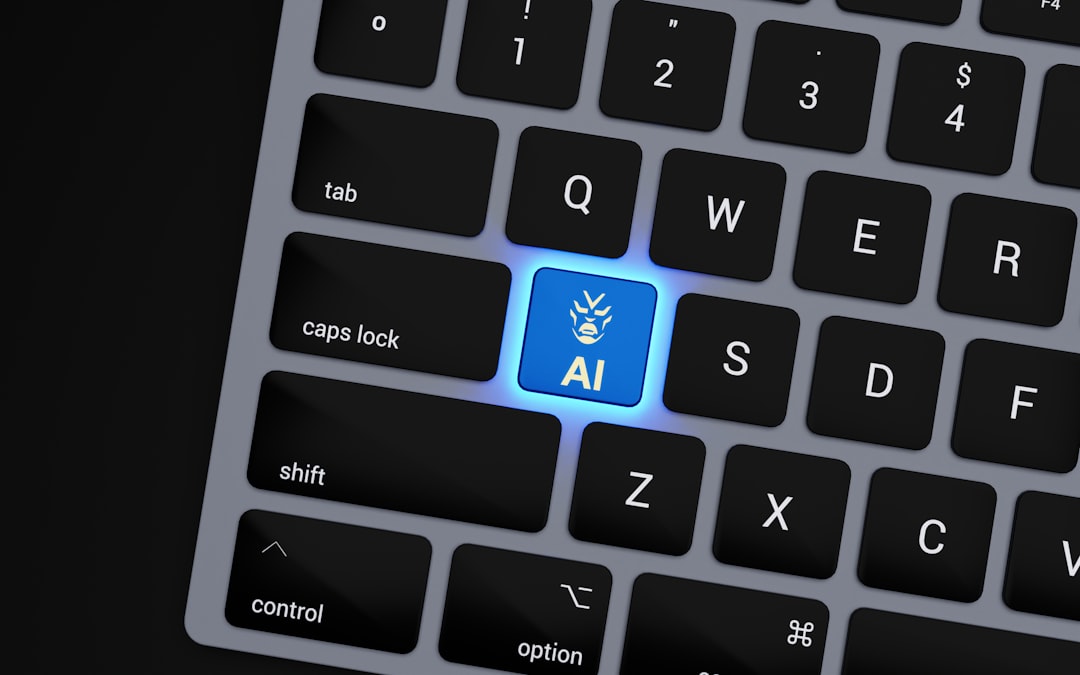
Natural Language Processing (NLP) is increasingly being used to generate scripts, taking advantage of deep learning's ability to create more logical and relevant storylines. Newer models, like BiLSTM and GPT-3, have shown significant progress in automating text creation, outperforming earlier methods in producing engaging scripts. As NLP advances, there's a growing concern regarding the balance between machine-generated content and the originality and creativity of human authors, particularly as these systems become more self-sufficient. While NLP can significantly speed up the script writing process, there are still complexities involved in crafting truly effective narratives that AI is still working to master.
### Natural Language Processing for Script Generation: Surprising Facts
Natural language processing (NLP) has become increasingly relevant in script generation for video content. While initially focused on basic text generation, it's now tackling the more nuanced aspects of storytelling. Maintaining a consistent narrative, especially in longer pieces, has been a challenge for automated systems. However, some newer NLP models are demonstrating surprising capabilities in this domain.
For example, character development is now being incorporated into the output of some NLP models. This means that they are starting to be able to create and sustain believable characters throughout an entire script, which was previously a major roadblock. Furthermore, we see progress in fine-tuning models on very specific genres like horror or comedy, which allows for more targeted and genre-specific outputs. This fine-tuning has brought to light the complexity of storytelling conventions, showcasing the level of detail that these models are starting to capture.
The ability to generate scripts of varying lengths is another advancement. Now, it's possible to create everything from short promotional videos to full-length feature films using the same NLP model. This adaptability is promising for content creators who need diverse forms of output for various platforms and target audiences.
In addition to narrative flow and genre, another intriguing aspect is the integration of emotional context into generated scripts. NLP systems are incorporating sentiment analysis, which enables them to gauge the emotional tone of scenes and characters. This potentially enhances viewer engagement because the resulting scripts can contain more impactful and realistic dialogue.
A further advancement is the use of multilingual datasets in NLP model training. This allows for scripts that can potentially resonate with a global audience. While opening doors to a wider range of viewers, this also introduces the challenging topic of dealing with cultural nuances and subtleties, especially in humor and expression.
The next frontier seems to be interactive scripts. Some researchers are creating NLP models that respond dynamically to audience choices or input, effectively allowing the audience to influence the direction of the story. This is a very promising avenue for video games, interactive content, and expanding audience engagement in general.
One of the interesting approaches being developed is the integration of visual context alongside text. This essentially merges NLP with computer vision, creating models that consider visual cues in tandem with dialogue. If successful, this approach could lead to scripts that create a more unified experience where dialogue seamlessly connects with onscreen actions, enhancing the overall coherence of the generated content.
Of course, with any technology, especially one related to creative processes, ethical considerations arise. Automated script generation raises questions about issues like copyright ownership and the risk of generating inaccurate or even harmful content. These are still open questions that require research and the creation of proper guidelines before these models see wider adoption.
However, it’s not necessarily about replacing human writers. Some researchers are developing NLP models that function as collaborative tools for writers. This means the models suggest dialogue, plot points, or possible story directions, essentially acting as "co-writers." This could be a way to maintain human ingenuity and intuition in the creative process while leveraging the efficiency and speed of NLP models, creating a novel workflow.
One factor that’s always important to remember is that NLP-generated scripts are highly dependent on the datasets used in training. If there are biases within the data, those biases will inevitably manifest in the generated outputs. This necessitates very careful curation of datasets to ensure the narratives are balanced and fair. This is an area of ongoing research.
Ultimately, the advancements in NLP for script generation are exciting. They highlight the potential for automated systems to assist in creative tasks like script writing. As these systems become more sophisticated, they will likely have a significant impact on the video content creation process.
Analyzing Goodfellow's Deep Learning Key Insights for Video Content Creators in 2024 - Computer Vision Advancements in Scene Analysis
Computer vision is experiencing a surge in advancements that are revolutionizing scene analysis, particularly in the realm of video. The shift is moving from simply analyzing individual images to deciphering the complex narratives and actions found within video sequences. Deep learning continues to be a primary driver in this progress, enabling a new generation of real-time applications, like automatically identifying pedestrians, monitoring vehicle movements, and enhancing security systems. These capabilities are becoming increasingly crucial for managing and understanding the dynamics of our modern environments.
Despite these positive developments, significant hurdles remain in accurately interpreting the dynamic elements of videos and the activities unfolding within them. This calls for more refined algorithms that can effectively handle the intricate nature of visual data in motion. Exciting new directions in computer vision involve multimodal learning and the application of deep learning approaches. These innovations are helping machines develop a more sophisticated, almost human-like understanding of the environment depicted within videos, including the objects present, the actions being performed, and the overall context of the scene.
The overarching objective is ambitious: to empower machines with a visual comprehension similar to that of humans. This desire to imbue machines with advanced cognitive abilities to decipher video is part of the continuing evolution within computer vision and is driving the search for new solutions to better analyze and understand the information hidden in video content.
Computer vision's progress in scene analysis has been quite remarkable, particularly in its ability to understand sequences of images rather than just single frames. Deep learning has undoubtedly been a major driver in this area, impacting not only computer vision but also other fields like natural language processing. Video scene analysis has become crucial in a wide range of applications such as keeping track of vehicles in real-time, detecting pedestrians for safety, surveillance systems, and even in the development of robots. While its importance is clear, video scene analysis is still a very challenging field, and researchers are continually striving to develop better algorithms and solutions.
One area of focus is the development of deep learning algorithms that can improve how well systems understand activities, interpret the scene itself, and automatically generate descriptive captions for videos. The ultimate goal in computer vision's pursuit of scene understanding is to develop machines that see the world similarly to humans, extracting the same level of information from images. We're seeing an increasing use of deep learning techniques in video processing, tackling problems like identifying anomalies, analyzing crowds, and recognizing activities. Deep learning is also being applied in medicine, with a particular emphasis on medical image analysis and video data from procedures.
Scene analysis itself involves interpreting the context and surroundings within an image, which involves tasks like identifying specific objects and figuring out actions or situations that are occurring. Computer vision is constantly evolving with newer techniques like deep multimodal learning emerging. The field is also seeing specific improvements in particular areas like being able to accurately locate and identify street signs from a variety of perspectives. While some of these advancements are showing promise, it's important to remain cautious about their limitations and potential shortcomings in complex and diverse real-world scenarios. Further research into their robustness and reliability is crucial before we can see their wide adoption in the real world.
Analyzing Goodfellow's Deep Learning Key Insights for Video Content Creators in 2024 - Reinforcement Learning for Personalized Content Delivery
Reinforcement learning (RL) is becoming increasingly important in tailoring content delivery to individual users, especially in areas like video streaming and online education. By utilizing user feedback and employing sophisticated deep reinforcement learning methods, content delivery platforms can intelligently deliver content that's more likely to resonate with individual viewers. This personalized approach improves the overall user experience and keeps viewers engaged. It moves away from the older model of delivering the same content to everyone, instead providing a more dynamic and adaptable experience that accounts for each user's unique interests and behaviors. Additionally, technologies like AI-generated content (AIGC) and methods that proactively cache content in advance further enhance the ability of these systems to anticipate and deliver what users want, improving efficiency and responsiveness. While RL presents exciting possibilities, it's crucial to think critically about the potential drawbacks, such as how user data is handled and the broader ethical considerations around personalized content delivery. The implications of these systems are complex, and they warrant careful attention from researchers, developers, and users.
Reinforcement learning (RL) has emerged as a promising approach to tailoring content delivery based on individual viewer preferences. The ability to dynamically adapt content based on immediate user feedback sets RL apart. This "on-the-fly" personalization fosters engagement by crafting a unique experience for each viewer.
RL excels at handling complex optimization scenarios. It can manage multiple objectives simultaneously, such as maximizing viewer satisfaction while also minimizing the data used for content delivery. This multifaceted approach presents opportunities that conventional methods often struggle to address.
Unlike simpler systems that primarily focus on immediate user feedback, RL takes a longer view, emphasizing sustained viewer engagement. The goal is to foster viewer loyalty by proactively providing content that keeps them interested over extended periods, which could prove challenging due to viewer's fickle nature and limited attention spans.
A core element of RL is the exploration-exploitation trade-off. RL models continuously weigh the benefits of showing new, potentially unproven content (exploration) against presenting content already known to align with user preferences (exploitation). Striking this balance effectively is vital for developing a high-performing recommendation engine.
Contextual bandits, a specific type of RL, are particularly effective in content delivery scenarios. These algorithms are able to leverage contextual information, such as time of day or a viewer's location, to make more tailored content recommendations. This type of contextual understanding is likely to become more and more important over time.
However, implementing RL for large-scale, personalized content delivery poses scalability challenges. As the number of viewers expands, the complexity of the RL model needed to maintain personalization for each individual grows considerably. Maintaining that complex dynamic across a large set of users poses an interesting challenge.
RL systems integrate viewer feedback into their decision-making processes, refining future content recommendations based on interactions. This continuous feedback loop forms a self-improving system that gradually aligns more closely with viewer tastes. Of course, achieving optimal results with these dynamic systems in a real-world environment is difficult due to various factors like user preferences and context.
One of RL's key advantages is the ability to learn patterns across multiple viewer sessions. This "cross-session" learning allows systems to retain knowledge of previous interactions and leverage that data to enhance future content delivery, enhancing viewer retention over time.
The development of RL-based systems for content delivery presents a variety of ethical questions. While these systems can indeed improve viewer engagement, there's a risk that they might inadvertently promote content that's sensational or misleading. Striking a balance between engagement and responsible curation remains a crucial concern.
Effective RL models need substantial viewer interaction data to learn from, requiring the collection of rich datasets. While beneficial, there is a need to address the tension between data collection and user privacy, and ensuring consent is obtained. It is likely that some people may opt-out of data gathering and this will need to be understood by content providers that wish to use reinforcement learning models in their systems.
In summary, reinforcement learning demonstrates a significant potential for achieving highly personalized content delivery, leading to enhanced viewer experiences. However, the challenges related to scalability, data collection, and ethical considerations require careful attention as RL techniques are developed and implemented for real-world use cases.
Analyze any video with AI. Uncover insights, transcripts, and more in seconds. (Get started for free)
More Posts from whatsinmy.video: