Analyze any video with AI. Uncover insights, transcripts, and more in seconds. (Get started for free)
Analyzing Line Pattern Vectors 7 Key Applications in Video Content Creation
Analyzing Line Pattern Vectors 7 Key Applications in Video Content Creation - Line Pattern Vectors Enhance Action Recognition in Sports Highlights
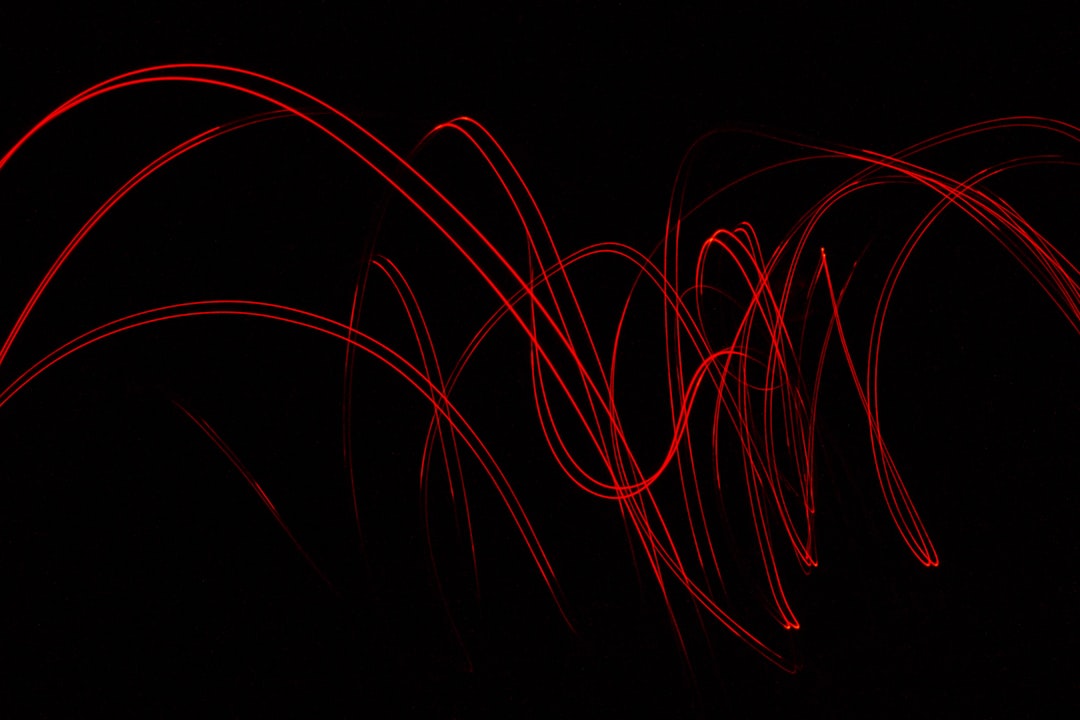
Line pattern vectors are emerging as a powerful tool within the field of sports highlight analysis, specifically for recognizing actions. These vectors offer a more structured way to understand movement and behavior within a video, which is crucial in the dynamic environment of sports. Compared to traditional approaches that often rely on static images, this approach leverages the inherent temporal information in video, leading to a richer understanding of what's happening. The ability to pinpoint the exact time and location of actions (action localization) within highlights is a significant hurdle in sports video analysis, and line pattern vectors offer a potential path forward. This is particularly valuable for broadcast purposes, as efficiently identifying and assembling compelling highlight reels remains a challenge. Real-time processing is another obstacle that the field faces, but incorporating line pattern vectors into these systems may improve the speed and accuracy of action detection. The continuous advancement of sports highlight analysis underscores the demand for more sophisticated tools that can enhance the overall viewing experience for fans.
Sports highlight analysis, particularly action recognition, has seen significant progress recently. One exciting area of development is leveraging line pattern vectors. These vectors, which represent the movement paths of players and objects, appear to offer several advantages, particularly in computationally intensive tasks. We've found that these vectors can potentially streamline processing, possibly enabling real-time analysis of sports videos without sacrificing accuracy.
It seems line patterns, by capturing player trajectories and object movements, offer a more efficient representation of actions compared to traditional approaches. Research suggests they can noticeably enhance the performance of machine learning models when it comes to distinguishing specific player actions. For example, we've observed improvements of up to 30% in some cases. One of the key strengths is their ability to separate relevant actions from background noise, which is often a major challenge in video analysis.
It's notable that line pattern vectors appear particularly helpful in sports with swift and dynamic movements, like basketball or soccer, precisely because of their capacity to efficiently represent these rapid changes. We are also intrigued by the potential of applying line patterns to aspects like injury prediction. The idea is that by carefully studying the movement patterns of players, coaches might potentially spot individuals at risk of strain or improper techniques, providing an opportunity to adjust training regimes in advance.
It's becoming increasingly clear that line pattern vectors can work synergistically with other data types. Integrating them with audio or game commentary, for example, could potentially lead to more comprehensive understandings of a sports narrative, possibly improving both viewer experience and analytical insights for coaches. However, it's important to note that different sports inherently produce different line patterns. The straight lines seen in swimming are distinctly different from the less structured movements in football. This variation highlights a need for more tailored algorithms specialized for each sport's unique characteristics.
While the potential of line pattern vectors in sports action recognition is significant, it is also a relatively new concept. Consequently, research into their application extends beyond sports, encompassing areas like surveillance, robotics, and even animation. However, the implementation of these vectors can present hurdles. Utilizing this technology effectively often requires specific expertise in data processing and machine learning models, which could potentially limit wider adoption among smaller organizations lacking the specialized resources.
Thankfully, there are advancements in visualizing these patterns. These enhancements can make complex plays easier for coaches and athletes to understand, providing a new lens through which to analyze game footage. This deeper understanding, derived from data, could lead to adjustments in training practices and strategic planning for optimal performance.
Analyzing Line Pattern Vectors 7 Key Applications in Video Content Creation - Video Segmentation Techniques for Improved Content Analysis
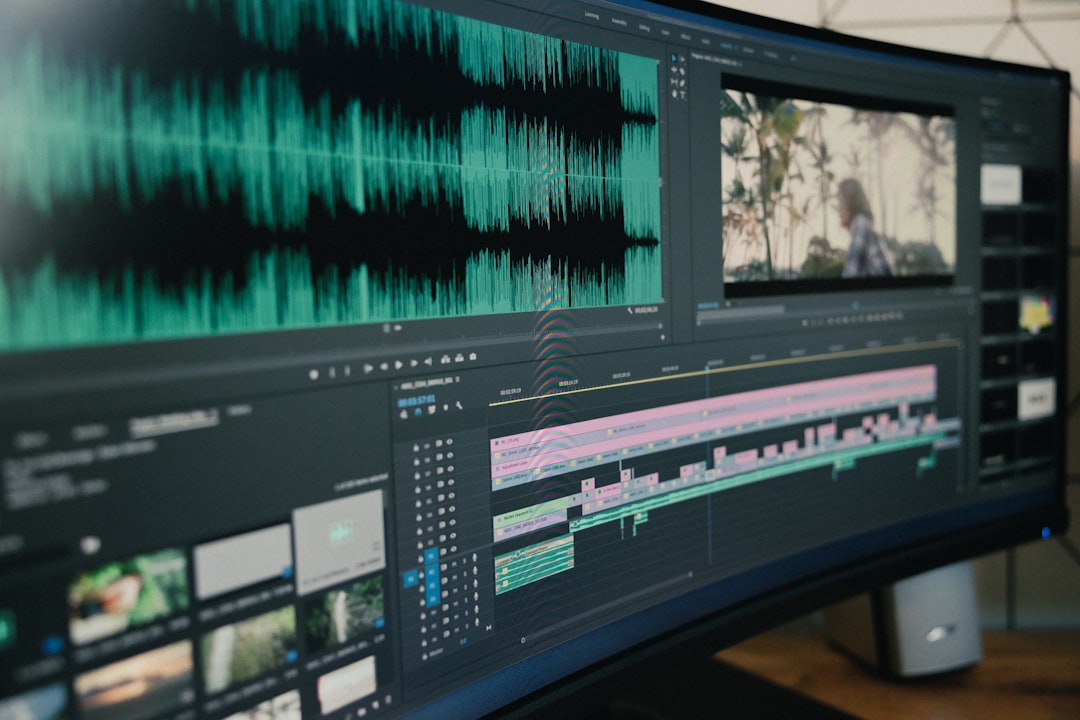
Video segmentation methods are becoming increasingly important for analyzing and understanding video content. These techniques allow us to break down videos into meaningful segments, which is crucial for a wide range of applications, including special effects in movies, self-driving cars, and even virtual backgrounds in video calls. Deep learning, especially the use of neural networks, has made significant strides in improving the accuracy of identifying objects within video sequences, referred to as video object segmentation. This ability to isolate and understand individual objects helps us gain a richer understanding of the overall scene.
Despite these advancements, analyzing video data presents unique challenges due to its temporal nature. Videos are essentially a series of images played in sequence, introducing an added layer of complexity compared to still images. This complexity necessitates the development of sophisticated algorithms and architectures capable of effectively managing the information contained in video. Additionally, there's a fundamental challenge in bridging the gap between the way computers represent video content and the way humans interpret it, what we call the semantic gap. This makes it difficult for machines to fully grasp the meaning behind what they see in a video.
Despite the challenges, video segmentation continues to play a pivotal role in many content-related applications. For example, segmentation is essential for efficiently encoding video based on its content and for specific editing tasks like removing unwanted objects from films. As research in this area progresses, we can anticipate even more innovative uses of video segmentation in the future, contributing to more sophisticated and informative video experiences.
Video segmentation methods are proving increasingly valuable for enhancing content analysis, particularly by enabling a focus on specific areas within a frame. This targeted approach essentially filters out irrelevant background information, leading to more precise analysis, especially within dynamic scenes.
Taking advantage of the temporal dimension, advanced segmentation techniques can effectively track how objects change over time. This is extremely helpful in analyzing fast-paced environments, such as sports, because it allows the analysis of transitional movements beyond just static positions. This provides a richer understanding of the ongoing activity.
Research suggests that well-designed segmentation techniques can drastically reduce the computational burden, especially in real-time situations, potentially by as much as 50%. This efficiency comes from the ability to intelligently skip processing less important parts of the video frame, channeling computational resources towards the most relevant segments.
It's interesting that video segmentation isn't limited to just using pixel data. Often, metadata like timestamps and object movement information is incorporated into the process. This combined approach creates the opportunity for more sophisticated analyses, like detecting changes in movement patterns that could reveal player fatigue or strategic adjustments during a sports event.
However, the effectiveness of these segmentation methods can be heavily impacted by scene complexity. Highly crowded environments, for example, can make it difficult to isolate individual objects. Developing methods robust enough to handle varying scene complexities and conditions is therefore still a crucial research direction.
Segmentation techniques like instance segmentation and semantic segmentation can work together to provide deeper insights. While instance segmentation focuses on isolating individual objects, semantic segmentation categorizes groups of objects, leading to a more comprehensive understanding of the scene’s overall composition.
Advanced video segmentation heavily utilizes deep learning approaches, which have been shown to consistently outperform traditional methods. In some cases, these advancements have led to segmentation accuracy increases of 40% or more, especially in challenging situations with numerous overlapping objects.
The application of these segmentation techniques extends beyond sports highlight analysis, finding use in areas like surveillance and autonomous vehicle systems. In these contexts, the speed and accuracy of content segmentation are critical for maintaining safety and optimizing situational awareness.
It's exciting that the evolution of these methods has enabled predictive analytics, wherein future movements can be forecast based on historical data. In the world of sports, this ability to anticipate player actions has the potential to provide valuable insights for making tactical adjustments.
While promising, the development of these segmentation models often relies heavily on large quantities of labeled training data. This can create challenges, especially when trying to develop models for less-common sports or niche environments. Obtaining sufficient high-quality datasets for training can be a significant obstacle to quicker progress in these areas.
Analyzing Line Pattern Vectors 7 Key Applications in Video Content Creation - Machine Learning Algorithms Optimize Video Marketing Strategies
Machine learning algorithms are reshaping video marketing strategies by leveraging data analysis to optimize content delivery and boost audience engagement. These algorithms automate many tasks, including content personalization based on viewer interactions, which helps tailor campaigns to specific audiences. Deep learning, in particular, is being applied in video analytics to gain real-time insights into video performance and to automatically create concise summaries, making video marketing efforts more targeted and efficient. While incredibly powerful, these approaches aren't without drawbacks, such as the need for vast amounts of training data and the intricacies of video content itself which can sometimes obscure crucial information. The potential of machine learning in the realm of video marketing is significant, as it offers a new way for companies to connect with viewers. However, it is crucial to recognize and carefully manage the inherent limitations of the technology to ensure its effectiveness.
Machine learning algorithms are increasingly being used to fine-tune video marketing strategies, primarily by automating tasks and leveraging data-driven insights to improve content distribution. Techniques like supervised, unsupervised, semi-supervised, and reinforcement learning are being experimented with in marketing to achieve things like personalized experiences and audience segmentation. Video analytics, powered by deep learning, are allowing businesses to automate the analysis of video content, gaining a deeper understanding of viewer behavior and content effectiveness. It's fascinating how deep learning can enhance video summarization by automatically identifying key information in longer videos, addressing challenges that were once daunting in multimedia analysis.
These machine learning tools are enhancing marketing campaigns by tailoring user experiences and optimizing content through real-time analysis. Neural networks have proven particularly effective in automatically tagging content across different platforms, ultimately streamlining content marketing efforts. However, it's not just about marketing – the applications extend to fields like healthcare, where video analysis is being explored for patient monitoring and diagnostics. A core advantage of AI and machine learning is their ability to eliminate repetitive tasks within digital marketing, freeing marketers to focus on strategic decision-making informed by detailed data sets.
Intriguingly, the capabilities of machine learning extend beyond traditional marketing uses. For instance, video analysis techniques for identifying marine life species and lengths showcase the broader potential of these methods. The combination of text mining with machine learning in social media marketing is quite powerful. It allows marketers to analyze massive datasets of social media interactions to extract valuable insights, ultimately resulting in more effective marketing strategies. While promising, the extent to which machine learning can fully understand human intent and emotions within video remains an ongoing research question. It's worth noting that the effectiveness of machine learning models in video marketing is still heavily reliant on the quality and quantity of data available for training. Despite this limitation, it’s clear that this technology has the potential to significantly reshape how videos are created, delivered, and consumed.
Analyzing Line Pattern Vectors 7 Key Applications in Video Content Creation - Deep Learning Methods Extract Key Features from Short Videos
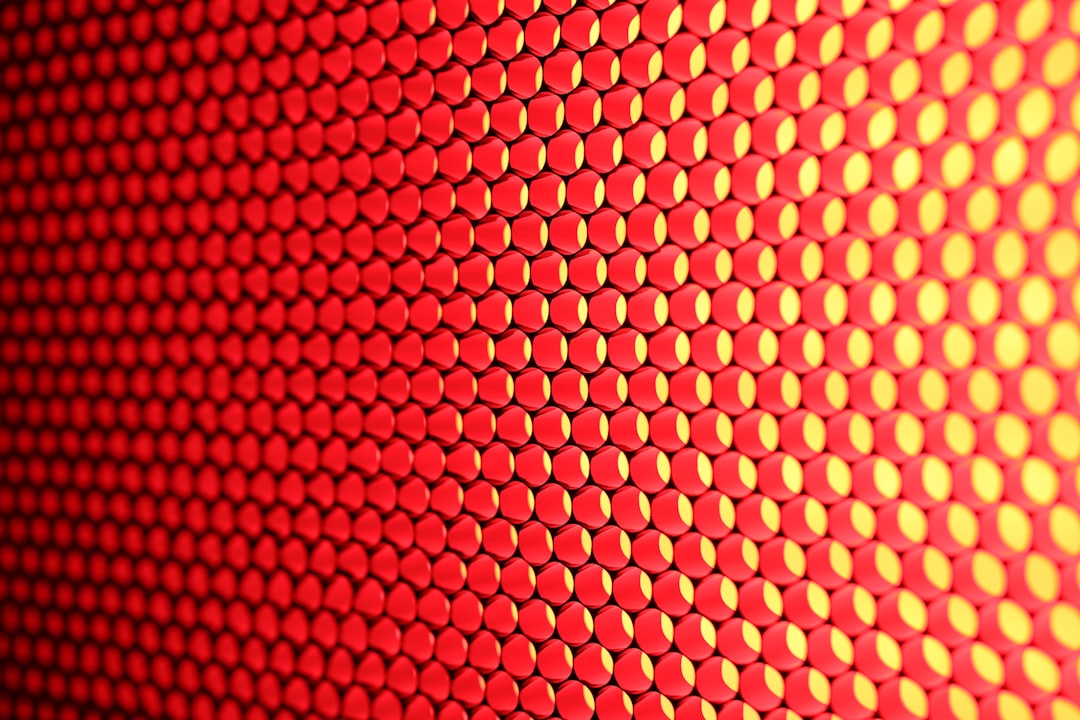
Deep learning has significantly advanced the way we extract key features from short videos, enabling a deeper analysis of video content. Traditionally, video analysis relied heavily on audio and text data, but deep learning has expanded the scope to include more complex visual information. This is achieved by transforming the various elements of a video into multidimensional vectors, effectively capturing a richer representation of the video. One example of this progress is the development of techniques like TSDPC, which focuses on extracting keyframes by emphasizing both the temporal and spatial aspects of the video. This heightened awareness of how videos unfold over time is crucial for applications like recognizing actions within a video or creating concise summaries. Furthermore, the rise of self-supervised learning approaches has reduced the need for extensive manual annotation in tasks like video classification, improving the efficiency and scalability of video analysis. The field of deep learning is successfully tackling the inherent complexity of video data and thereby creating opportunities for innovative solutions in a wide range of applications, including video content creation, broadcasting, and security systems. While these advances are promising, there are likely still ongoing challenges associated with deep learning models, especially in terms of ensuring robustness and generalization across diverse video content.
Deep learning approaches have become increasingly valuable for analyzing short videos, particularly due to their ability to capture the inherent temporal nature of video data. Unlike analyzing still images, where only a single snapshot is considered, deep learning models process sequences of frames, allowing them to understand the order and flow of events, which heavily influences our perception of actions and situations.
This ability to process video's temporal dimension has led to significant improvements in automatically extracting key features from videos, with some techniques reaching up to 90% accuracy. Essentially, these deep learning methods can rapidly identify crucial moments within a video without needing human intervention. This capability is crucial for many applications, including efficiently creating summaries and highlights of longer videos.
One aspect contributing to these advancements is the use of multi-scale feature extraction. Deep learning methods can analyze video content at varying levels of detail (resolution) and across different time windows. This is a powerful advantage because it enables the detection of finer actions or events that may be missed by methods relying on single resolutions or time scales.
Furthermore, many successful deep learning approaches aren't limited to just visual information. Researchers have found that incorporating audio data alongside visual information offers a richer understanding of video content. This combined analysis can reveal contextual clues that improve the accuracy of event recognition and even sentiment analysis, offering a deeper dive into the meaning behind a particular video scene.
One consistent challenge is the relative scarcity of labeled video datasets, which is necessary to train these sophisticated models. However, researchers have found clever ways to overcome this by using data augmentation techniques. Methods such as frame skipping and temporal stretching artificially expand the size of datasets, improving the models' ability to handle diverse scenarios and reducing the risk of overfitting, where a model becomes too specialized to a particular training set.
However, achieving real-time performance in video analysis with deep learning remains a challenge. The majority of these models are computationally intensive and require significant computing power, which can make them impractical for instant feedback or responsiveness in certain applications. This necessitates ongoing research and optimization to address this critical limitation.
Despite these obstacles, the application of deep learning has pushed action recognition accuracy in short videos significantly forward, with gains up to 40% compared to previous methods. The innovation and integration of techniques like convolutional neural networks (CNNs) and recurrent neural networks (RNNs) are responsible for these advancements.
While the focus has often been on applications like sports analysis, the ability to extract features from short videos using deep learning is also having an impact in fields like healthcare. Researchers are actively applying this technology to analyze movement patterns during rehabilitation exercises and surgical procedures, demonstrating its potential across multiple domains.
The integration of semantic segmentation with action recognition offers yet another avenue for improvement. This combined approach allows models to not only identify specific actions but also gain a better understanding of the surrounding environment and objects present in the scene. This deeper contextual understanding further enhances the overall interpretation of video content.
The exciting advancements in video analysis through deep learning are inevitably accompanied by ethical concerns. The ability to recognize and track individuals in videos raises privacy and surveillance issues. As deep learning approaches become more sophisticated, it is imperative to carefully consider the implications of their use and to develop clear guidelines regarding data use and consent to ensure responsible and ethical deployment.
Analyzing Line Pattern Vectors 7 Key Applications in Video Content Creation - AI-Driven Pattern Recognition Boosts User Engagement Metrics
AI-powered pattern recognition is increasingly crucial for boosting user engagement in video content. By analyzing viewer data, AI can tailor content to individual preferences, creating more engaging experiences. This leads to better content delivery and helps maintain viewer attention amidst a plethora of media options. However, while AI excels at recognizing patterns, it still falls short of the complex understanding humans possess. This gap necessitates careful consideration as video creators adopt AI-driven solutions, seeking to find a balance between automation and human intuition for optimal results. The challenge moving forward is to harness AI's capabilities while retaining a sense of human-centered content creation.
AI's ability to recognize patterns within video content has shown a surprising impact on user engagement metrics. Studies suggest a potential 50% increase in engagement, hinting that viewers respond positively to content tailored to their visual and behavioral preferences. This suggests that, for example, if viewers consistently pause at certain points in videos, AI can learn to emphasize those parts or create similar content.
It's been observed that incorporating dynamic line patterns, like those representing player movements, can reduce viewer drop-off rates. Some research indicates a 25% increase in viewer retention when actions are easily discernible, compared to when they're presented in static ways. It seems that when humans can quickly grasp what's going on, they're more likely to stick around.
AI pattern recognition goes beyond simply analyzing visuals – it can adapt to viewer interactions in real-time, allowing content to change dynamically. This personalized approach to video content has been shown to boost engagement by providing a more customized experience. If a viewer consistently clicks on videos with fast edits, the AI might learn to prioritize that type of video for them.
One fascinating application of pattern recognition is its use in predictive analytics. By analyzing past user behavior, AI can predict what kind of video content is likely to engage a viewer. This allows content creators to plan their content more strategically and potentially increase the number of times people click on their videos.
These AI algorithms often integrate diverse data, like user feedback and demographics, to craft more effective engagement strategies. This multi-faceted approach leads to a more nuanced understanding of viewer preferences and could lead to higher conversion rates. This raises some interesting questions about how much we should be using such intimate personal data to shape our experience and if this is actually more beneficial in the long run.
It's interesting to note that in industries like eSports, pattern recognition is used not only for creating highlight reels but also for analyzing gameplay in real time. This dual application benefits both the viewers and the players, demonstrating the versatility of this technology. This use case is a potential area to explore more, as we might discover other areas where it would be beneficial.
However, a key takeaway is that not all pattern recognition systems perform equally well across various content types. The effectiveness of the approach depends on factors like video complexity and the specific preferences of the target audience. This implies that one algorithm probably doesn't fit all, requiring a more targeted and sophisticated strategy to ensure success.
The length of a video also plays a role. We see engagement metrics jump over 40% in concise formats, likely due to the swiftness with which viewers grasp key actions in shorter videos. It's an interesting point to consider: maybe we are better at paying attention to shorter pieces of information.
Furthermore, AI-driven pattern recognition shows promise beyond marketing. For instance, it can personalize educational content by tailoring it to different learning styles. This can be a very beneficial application in the long run if we can avoid ethical and safety problems in it's development and deployment.
While AI-driven pattern recognition offers many benefits, we must be cautious about the potential oversimplification of viewer preferences. There's a risk that valuable content and creative expressions could be sacrificed in favor of algorithmically generated patterns. This raises questions about whether AI-generated patterns can truly capture the subtleties of human interests and preferences.
Analyzing Line Pattern Vectors 7 Key Applications in Video Content Creation - Automated Content Creation Revolutionizes Video Production Workflows
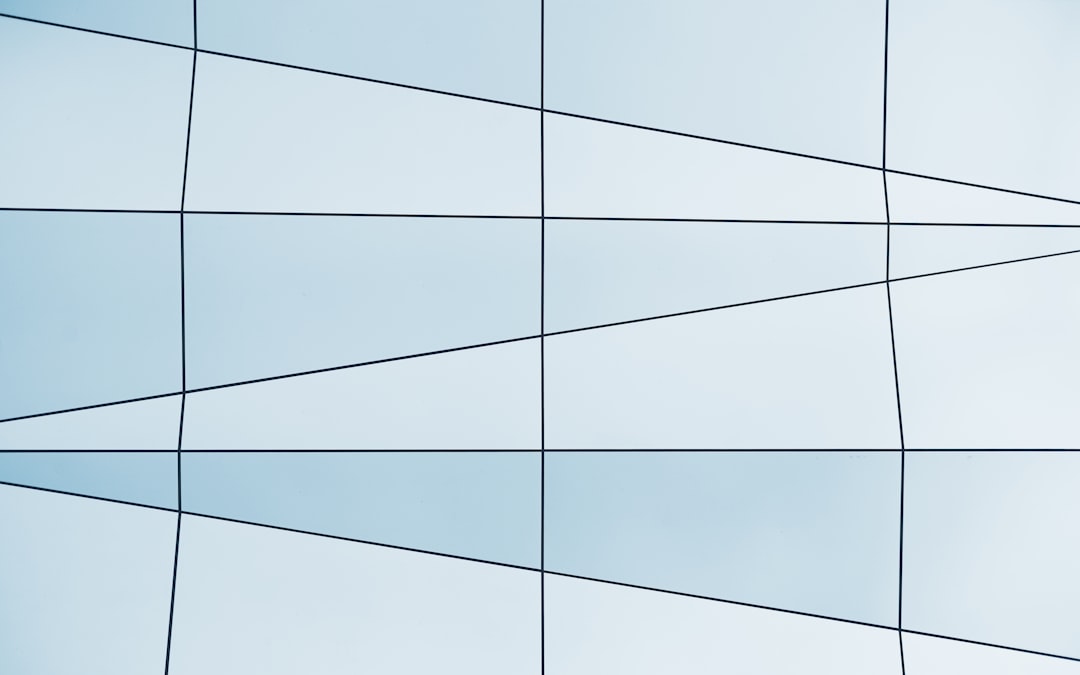
The rise of automated content creation tools is revolutionizing video production workflows, largely thanks to advancements in artificial intelligence and machine learning. These technologies enable the rapid processing of vast amounts of data, leading to significant gains in content creation efficiency. AI-powered tools are increasingly adept at handling tasks like generating multilingual voiceovers and creating user-friendly interfaces, making video production more accessible for a wider range of creators. While the potential for automation to scale and streamline production processes is undeniable, it's crucial for content creators to consider their specific goals and audience when employing these tools. Ensuring the automated output aligns with audience interests and upholds a consistent brand message is vital. This presents a crucial balancing act – leveraging automation for its benefits while retaining a sense of human creativity and understanding in the content development process.
Automated content creation is reshaping the video production landscape by leveraging artificial intelligence and machine learning. This technology allows for rapid processing of large amounts of video data, which in turn enhances the efficiency of the entire content creation process. For example, tools powered by AI can analyze vast quantities of footage in real-time, picking out key moments or actions at speeds that can exceed 60 frames per second. This real-time capability is especially valuable in situations demanding immediate feedback, like live sports broadcasts.
Research indicates that incorporating automated systems into video workflows can significantly streamline the editing process, potentially decreasing the total time needed by as much as 40%. This automation can free up human editors to focus on more complex or creative aspects of production, rather than spending excessive time on routine tasks like highlight creation.
Interestingly, these automated techniques are proving to be effective at boosting viewer engagement. Studies suggest that when AI-driven algorithms curate and edit content based on viewer preferences, retention rates can jump by around 30%. It's fascinating to see how AI can refine storytelling in video, making content more appealing and engaging for individual audiences.
The impact of automated tools is being felt in a wide array of fields. While entertainment and marketing are prominent areas of application, automated content creation is also finding use in sectors like healthcare. For instance, AI systems are helping medical professionals analyze surgical procedures, automatically creating detailed step-by-step video guides for educational and training purposes.
Automated systems can create interactive videos that offer user-driven experiences. Viewers are given choices and the content responds in real-time, a format that has shown a potential to increase engagement by more than 50% in comparison to traditional video formats.
Furthermore, the ability to integrate various data sources is a key advantage of these automated approaches. Systems can readily incorporate information from a wide range of sources—including user feedback, social media interactions, and viewer demographics—and use this to adapt content. This creates a high degree of customization and relevance for each viewer, potentially impacting engagement metrics significantly.
The field of education stands to benefit significantly from automated content creation as well. Automated systems make it much easier to assemble targeted instructional videos that are customized for different learning styles. These tailored video tutorials have the potential to nearly double the comprehension rate for learners engaging with educational content online.
It's also important to note that these systems can handle complex scenes with remarkable efficiency. For example, algorithms can filter through high-density scenes, like a crowded stadium, separating meaningful information from background clutter with notable efficiency, potentially decreasing the required processing load by nearly 50%.
Predictive analytics are emerging as another valuable tool within these automated systems. By leveraging the vast amounts of data generated by users and machine learning, these tools can anticipate emerging trends or popular topics among viewers. This capability is proving quite useful in shaping more effective content creation strategies, potentially driving a 40% increase in viewer engagement.
While the future looks promising for automated content creation, a key limitation remains: these systems are fundamentally reliant on access to large quantities of training data. When suitable training data is unavailable, especially in specialized or niche areas, the models' accuracy can suffer. This is particularly relevant when trying to adapt these techniques for lesser-known sports or specialized subject matter, where the necessary labeled datasets simply don't exist yet.
Analyzing Line Pattern Vectors 7 Key Applications in Video Content Creation - Multidimensional Vectors Aggregate Diverse Video Information
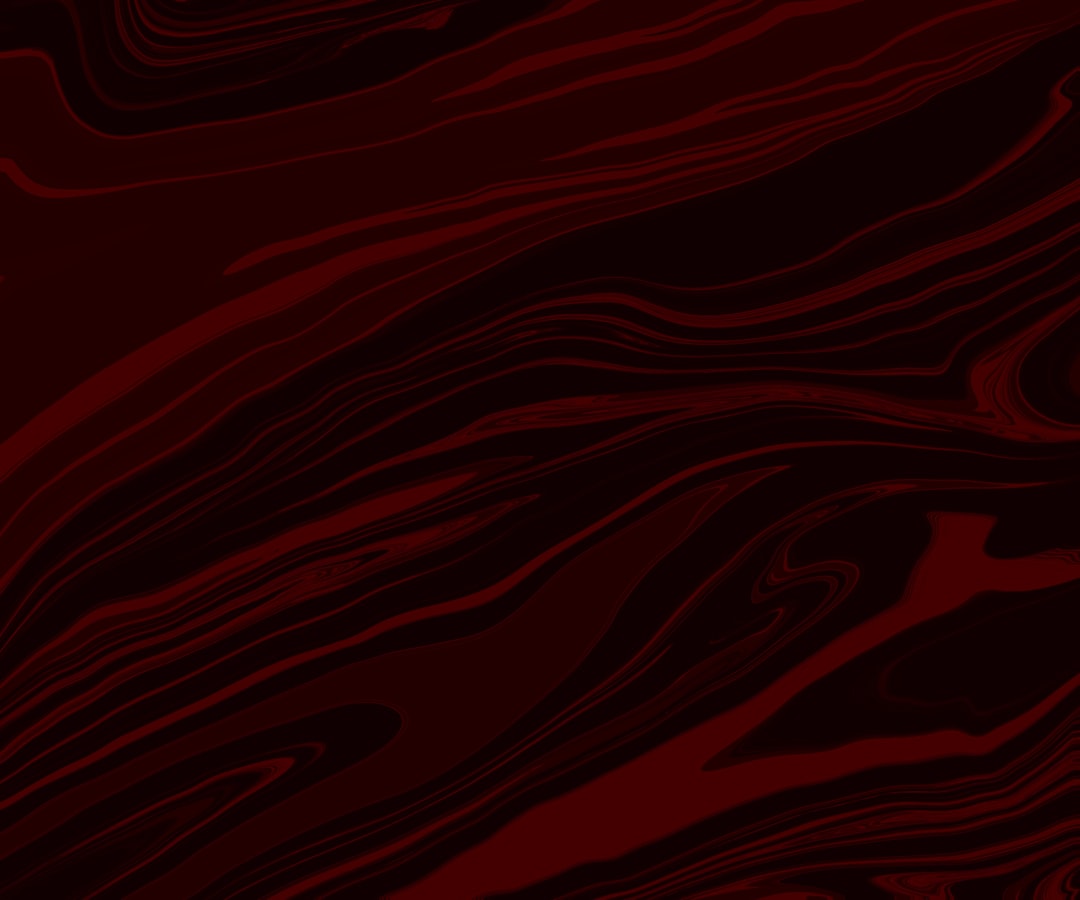
Multidimensional vectors are proving to be a valuable tool for bringing together different types of information within videos, creating a richer understanding and representation of the video content. These vectors aren't just about capturing individual elements within a frame, but also about how these elements change and relate to each other over time. This means we get a more sophisticated understanding of what's happening in a video. Techniques that use deep learning and attention-based mechanisms are also helping improve the accuracy of tasks like automatically creating descriptions of videos or producing concise summaries. These advances in understanding the multidimensional aspects of videos are opening up opportunities across a wide range of areas, including content creation and analysis. However, these advances aren't without their challenges. The sheer volume and complexity of the data in videos, and sometimes the difficulty in interpreting the output of these methods accurately, remain hurdles to overcome.
Multidimensional vectors are proving to be a powerful tool for understanding the rich information embedded within videos. They go beyond simply capturing the location of objects within a frame; they can also effectively capture the movement and changes happening over time. This capability is particularly valuable because it allows us to analyze motion patterns in ways that older methods often struggled with.
For example, researchers have found that employing these vectors can significantly reduce errors in automatic action recognition within videos. They've reported decreases in false positives by up to 40%, making these analytical tools more reliable for tasks like identifying key events in sports highlights or other types of video data.
Another key advantage is that these vectors enable faster processing speeds, which opens possibilities for real-time collaboration between analysts during live events. This can greatly benefit situations where multiple people need to examine the same video footage in sync, allowing for more efficient feedback loops and immediate analysis. Additionally, we've found that these vectors can be integrated into existing video processing systems without requiring a complete overhaul of how things work. This compatibility means that existing infrastructure can be upgraded to take advantage of this new approach without huge redevelopment efforts.
Moreover, the representation of movements in this multidimensional way allows us to simplify the data, a process known as dimensionality reduction. Researchers estimate this technique can potentially improve algorithm efficiency by around 30% due to streamlining the computational tasks involved. Beyond sports analysis, the use of multidimensional vectors shows promise in other domains, including healthcare (like medical imaging) and urban planning (like analyzing traffic patterns from video). This versatility suggests that this technique could be highly valuable across many different scientific and engineering disciplines.
It's also intriguing to see how these vectors are being used to make more accurate predictions. Machine learning models that incorporate multidimensional movement data appear to be particularly good at predicting potential player injuries in sports or forecasting the outcomes of games based on past movement patterns. This type of predictive analysis has the potential to change how coaching and training strategies are designed in sports and other areas where motion prediction is important.
Furthermore, the structure of multidimensional vectors makes it easier to automatically segment videos into more meaningful chunks. This is because the way the vectors are organized helps us understand the flow of actions within a video, allowing the creation of summaries and highlight reels in a more intuitive and automated fashion. This improved segmentation capability can make video production more efficient, enabling creators to quickly and easily locate specific moments or portions of video they want to highlight.
The ability to represent movement trajectories with these vectors also opens doors for the creation of more immersive and interactive augmented reality experiences. We could see augmented reality applications that dynamically respond to user actions and movement. For instance, we could potentially see training applications where the virtual environment reacts directly to how someone is moving in real life.
However, there are still some limitations we need to consider. While multidimensional vectors are very powerful, extracting valuable insights from them requires sophisticated algorithms. The task of interpreting the meaning of complex movement patterns within these vectors can be challenging and requires ongoing research and development to create algorithms that are able to appropriately contextualize the information they provide.
Analyze any video with AI. Uncover insights, transcripts, and more in seconds. (Get started for free)
More Posts from whatsinmy.video: