Analyze any video with AI. Uncover insights, transcripts, and more in seconds. (Get started for free)
Sora's 60-Second Video Generation Analyzing Text-to-Motion Capabilities in OpenAI's Latest AI Model
Sora's 60-Second Video Generation Analyzing Text-to-Motion Capabilities in OpenAI's Latest AI Model - Sora's 60-Second Video Generation Capability
OpenAI's Sora introduces a new level of video generation, particularly its ability to create up to 60-second clips. It's built on a diffusion transformer architecture, a technical approach that enables the model to craft remarkably lifelike and high-definition videos. This isn't just about turning text into basic animation. Sora can also seamlessly extend existing video footage and bring still images to life through textual cues. The potential for creative applications is vast; users can imagine and describe a wide array of scenes – from simple to complex – and Sora attempts to visually realize them. The resulting videos demonstrate a high degree of fidelity, able to render detailed environments and capture subtle human emotions. Although currently under research, Sora's capacity to synthesize compelling videos from text presents a pivotal moment in the evolution of media. It prompts us to reimagine how narratives unfold and how audiences connect with visual stories.
Sora's ability to generate videos up to 60 seconds long stems from a sophisticated algorithm that doesn't just translate text into motion, but into narratives. It accomplishes this by analyzing the nuances of the textual input, enabling a level of comprehension that surpasses traditional video generation methods.
This capability relies on powerful neural networks that forecast sequences of movement based on the text description, exceeding older approaches that were heavily reliant on manual interventions. Instead of relying on rigid pre-defined formats, Sora's design allows it to create original content by interpreting the intricate details of language, customizing outputs for specific scenarios.
Although generating videos with thousands of frames, Sora maintains impressive speed and resolution. Its algorithms efficiently map motion paths and transitions, ensuring that the swift generation doesn't come at the cost of quality. Moreover, Sora incorporates a feedback mechanism, which allows the model to learn from user input, refining its outputs and adapting to different styles and preferences over time.
Intriguingly, this technology allows Sora to go beyond basic animation, imbuing the generated content with emotional nuance. The model analyzes emotional cues within the text, effectively bridging the gap between human emotion and the digital realm. This capability extends even further by including sound design and accompanying audio, thus generating fully integrated audiovisual experiences.
Furthermore, Sora has the potential to adapt content on the fly. It can evaluate viewer reactions in real-time and adjust future content to enhance engagement. This real-time adaptation opens up possibilities for personalized and interactive content generation, demonstrating a fascinating intersection of AI and audience analytics. This technology's versatility is also noteworthy, as Sora can create a variety of video genres, from educational pieces to entertainment, showcasing adaptability that conventional editing software often struggles with.
However, despite the incredible potential, the ethical implications of Sora's capabilities warrant serious attention. The ability to automate content creation at this level raises concerns about the spread of misinformation and the responsible use of these tools, emphasizing the importance of thoughtful discussions and guidelines for their implementation.
Sora's 60-Second Video Generation Analyzing Text-to-Motion Capabilities in OpenAI's Latest AI Model - Advanced Scene Creation and Camera Motion Techniques
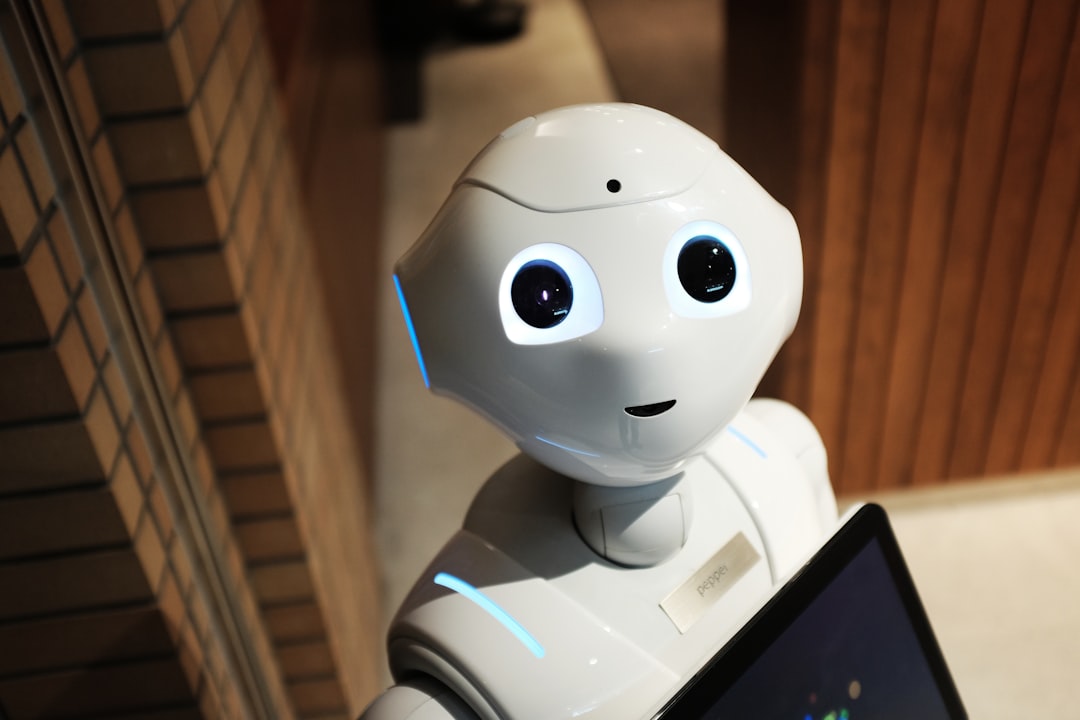
Sora's advancements in "Advanced Scene Creation and Camera Motion Techniques" represent a major leap forward in AI-driven video generation. Sora's strength isn't just about creating realistic images from text; it's about dynamically constructing complex scenes and seamlessly incorporating sophisticated camera movements that enrich the storytelling experience. This capability to craft truly cinematic scenes with nuanced camera angles and smooth transitions enhances viewer immersion by bringing narratives to life with exceptional visual detail and fluid motion.
Adding to the impact of these advanced techniques, Sora can intricately weave emotional cues and believable character interactions into the scenes, further deepening the viewer's connection to the story. By incorporating emotion into the visual narrative, content creators gain new abilities to elicit specific emotional responses from their audience, pushing the boundaries of how stories are communicated visually.
As the field of AI-driven video generation continues to evolve, the synergy of these enhanced scene creation and camera movement technologies will likely revolutionize the way visual narratives are crafted and experienced, prompting us to reconsider the very nature of visual storytelling.
Sora's impressive video generation capabilities extend beyond simply translating text into motion; it delves into advanced scene creation and camera work. It seems to dynamically adjust the frame rate based on the scene, allowing for smoother transitions and more believable movement. This implies that it doesn't just use a fixed frame rate, but adapts to the needs of the narrative – a useful feature for varied video styles.
Interestingly, the model incorporates a variety of camera motion algorithms that mimic techniques commonly used in film production. This includes things like smooth camera pans or zooming effects, adding another layer of realism to the output. These choices, if implemented correctly, should contribute to a more immersive and dynamic viewing experience.
Furthermore, its ability to simulate depth of field is quite intriguing. This is a common film technique to draw attention to certain elements while softening the background, which adds depth and perspective. How effectively it mimics this remains to be seen, but the potential is certainly there for more realistic visual compositions.
The model's approach to keyframe prediction is a departure from traditional animation techniques where these frames were manually set. Instead of that approach, it predicts key moments within a scene based on the text prompt. If successful, this could translate to more fluid and natural animations that match the intended story more closely.
It's noteworthy that Sora can integrate data from real-time motion capture systems, meaning it might be able to use motion capture data to create more realistic human movement in the videos. This could be a significant leap in creating convincing human-like characters within its generated scenes.
The system also seems to have a sophisticated understanding of 3D space, which should help to arrange objects in a scene in a way that is more natural, like how we perceive the world. This 3D awareness could potentially translate to more believable and immersive scenes where different elements interact appropriately.
Sora goes further with its lighting algorithms, which strive to emulate real-world lighting based on the scene itself. This means that it can generate more realistic shadows and reflections, contributing to the overall fidelity of the generated videos. There is definitely a lot of potential there if done well.
Beyond motion, Sora seems to be able to tie together visual effects and speech. It’s fascinating to consider that the system might be able to react and synchronize visuals to the audio content, creating a more dynamic and reactive storytelling experience. It's still early, but it highlights a potential for how AI models could learn and integrate different kinds of media in more sophisticated ways.
While a lot of these features may be automatically controlled, Sora gives users the possibility to adjust some aspects of the style. Think color palettes or the pace of the motion. Having this level of customization could be useful for adapting to different tastes or aesthetic choices.
And finally, Sora seems to have a self-training aspect where it learns from past successful outputs. This should refine the AI's understanding of diverse motion styles, pacing, and how it matches the tone and subject matter. This constant evolution is important for refining its skills, and it could lead to more impressive video outputs over time.
Despite the impressive possibilities, there are still a lot of unknown factors and challenges. We’ll need to see how successfully these techniques are applied in practice and how they impact the overall quality and realism of the videos. It's also worth considering how these features interact with one another and what types of potential failures or limitations could arise.
Sora's 60-Second Video Generation Analyzing Text-to-Motion Capabilities in OpenAI's Latest AI Model - Text-Based Instructions for Realistic Video Production
Sora's capacity for generating realistic videos based solely on text instructions is quite remarkable. It's not simply converting text to basic animation, but rather crafting detailed scenes with believable characters and nuanced interactions. Sora's strength lies in dynamically building elaborate scenes while using sophisticated camera techniques to enhance the narrative. It appears to grasp 3D space, adjust lighting in real-time, and predict keyframes smoothly, all contributing to visually compelling results. It also attempts to translate emotion from text into the visuals. These advancements are encouraging, but critical evaluation of the video quality and the broader ethical consequences of automated video production are crucial. As the technology progresses, it's important to acknowledge the potential issues while striving to use Sora responsibly and thoughtfully.
Sora's ability to translate text into realistic video relies on its sophisticated understanding of language and motion. It goes beyond simple animation, parsing context, tone, and even emotional nuances embedded in the text prompts to generate corresponding movement sequences. This level of comprehension is a significant step forward compared to earlier methods.
One notable aspect is its dynamic frame rate adjustment. Instead of a fixed frame rate, it seems to adjust it depending on the scene's needs, potentially leading to smoother and more natural transitions in the video. Whether this is truly seamless or just a clever illusion will require more examination.
Another interesting facet of Sora's video generation is its apparent understanding of three-dimensional space. It seems to arrange objects and characters in a scene in a way that respects how we perceive the physical world. This 3D awareness helps the videos feel more grounded and believable, although we still need to see how this plays out in more complex situations.
The model's integration with motion capture systems hints at a potential leap in creating more realistic human movements within the videos. If this data is handled properly, we could see more convincing characters with lifelike emotions and interactions, potentially changing how human characters are depicted in computer-generated scenarios.
Its lighting algorithms aim to mimic how light behaves in real-world environments. This focus on realistic shadows and reflections can significantly contribute to the overall realism of the output. It will be interesting to see how well it captures the subtleties of lighting in a diverse range of environments.
Sora's method for keyframe prediction is intriguing. Instead of relying on traditional, manually defined keyframes, it attempts to predict them based on the context of the text prompt. If this technique proves effective, it could pave the way for smoother and more natural animations that better match the intended narrative flow.
Further, Sora integrates emotional cues within the text. This enables the generation of scenes with subtle character interactions and emotional depth. How well this is achieved across various emotions and in complex scenarios remains to be seen.
Sora aims to offer an integrated audiovisual experience by incorporating sound design and synchronizing speech with the visual output. This creates a cohesive narrative, potentially blurring the lines between conventional video production and AI-generated content.
While the model handles many aspects automatically, it retains some user customization. Features like adjusting color palettes and motion pacing enable users to mold the output to better suit their artistic preferences.
Lastly, Sora's self-training ability helps it continuously learn and improve. This iterative process involves refining its understanding of motion styles and how to best align those with different video tones and subject matters. It suggests a potential path to producing increasingly complex and sophisticated videos over time.
Despite these exciting features, there are still unknowns and potential limitations. It will be crucial to see how these techniques perform in practice and the impact on video quality. Understanding how these different features interplay with each other is important, especially when considering potential failures or constraints. This field is still quite new, and these advanced methods will require further testing and refinement.
Sora's 60-Second Video Generation Analyzing Text-to-Motion Capabilities in OpenAI's Latest AI Model - Transformer Architecture Behind Sora's Video Generation
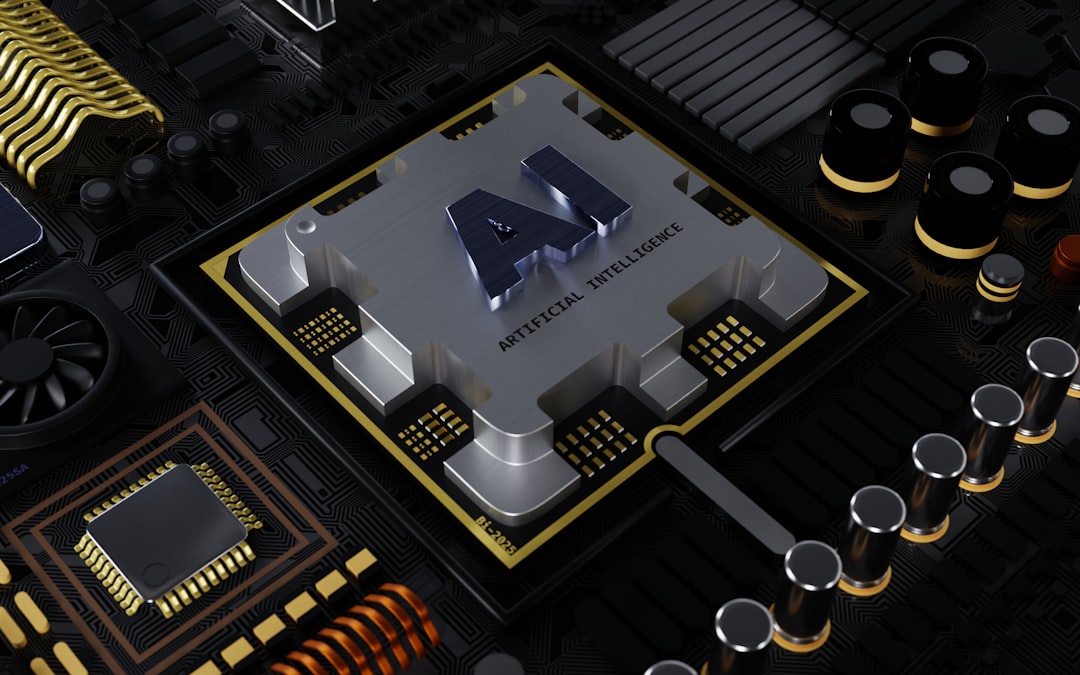
### Transformer Architecture Behind Sora's Video Generation
Sora's ability to create videos relies on a sophisticated approach called a diffusion transformer architecture. This architecture is designed to process both visual information and motion in a computationally efficient way, using "spacetime patches" to break down the video into manageable chunks. It's through these patches that Sora can take text descriptions and transform them into video, essentially translating words into moving images.
Features like cross-attention, which helps the model understand the relationships between different parts of the video, and adaptive layer normalization, which allows it to adjust its processing based on the specific video content, are crucial to this process. This detailed approach not only helps Sora to generate compelling and detailed videos but also gives it the ability to mimic real-world movement and interactions.
However, alongside its impressive capabilities, Sora's ability to generate realistic videos from text raises questions about how it will be used and its impact on content creation. There's a need to be aware of the potential for this technology to be misused, as the authenticity of generated videos may become harder to determine. Responsible development and careful consideration of its ethical implications are critical as this technology matures.
Sora's video generation relies on a transformer architecture, which is a departure from older sequential models. It uses self-attention mechanisms to understand the context of a text prompt better, leading to more nuanced video outputs. Essentially, it learns to weigh the importance of different words and phrases, capturing the subtleties of the input.
Furthermore, Sora incorporates cross-modal learning, allowing it to effectively blend text, images, and sound. This creates a more integrated narrative experience. This interweaving of different media types goes beyond what we've seen before and helps create more cohesive storylines.
Unlike a lot of other video generation models that prioritize image quality, Sora focuses on temporal resolution. This emphasis on the smooth flow of motion is vital for creating engaging and believable videos, making the movement appear natural instead of robotic.
Sora utilizes innovative motion prediction algorithms. Instead of rigid pre-programmed sequences, it dynamically adapts based on the text input's context. This leads to a more organic feel in the videos. Transitions and movements aren't mechanical, but rather respond to the scene and story in a way that feels much more lifelike.
The architecture includes a real-time feedback loop to continuously learn from user preferences. This means it isn't static; it constantly evolves by incorporating input from how people interact with the videos it generates. This dynamic adaptation is key to refining its capabilities over time.
Sora has an interesting ability to adapt its video style based on the narrative tone it extracts from the text prompt. It automatically adjusts things like pacing and the emotional intensity, helping it generate videos that align with various genres. It's almost like it has a basic sense of how to adapt its style for the content it's creating.
The model possesses an awareness of 3D spatial relationships. This understanding allows Sora to dynamically position objects and elements within a scene realistically. This is crucial for maintaining a believable world when the camera moves through the environment.
It's trained in an evolutionary way, where it tests various video styles against user preferences. It effectively discards styles that aren't favored and promotes those that resonate better with audiences. This ongoing refinement process, coupled with feedback loops, is vital for improving the AI's ability to produce desired results.
Sora's lighting algorithms are a strong aspect. They strive to mimic how real-world light works. This leads to a more accurate representation of shadows and reflections, improving the generated videos' depth and believability. It's impressive if they can get it truly correct.
Lastly, Sora can create synchronized audio and video outputs in real-time. This means that visual elements seamlessly respond to audio cues, creating a more engaging and comprehensive narrative experience. It's a significant step toward generating fully integrated multimedia content that is responsive to the different elements in the video.
While Sora shows a lot of promise, it's still early. How well these features translate into practical applications and their overall impact on video quality and the broader ethics of AI-generated content remain to be seen. There are many questions that need to be addressed before the capabilities are fully understood.
Sora's 60-Second Video Generation Analyzing Text-to-Motion Capabilities in OpenAI's Latest AI Model - Training Data Diversity for Improved Video Quality
The quality of videos generated by AI models like Sora is significantly impacted by the diversity of the data used to train them. A wide range of video examples, encompassing various styles, scenes, and human expressions, allows the model to learn and reproduce a broader spectrum of visual content. This diversity is crucial for creating videos that feel realistic and engaging, accurately capturing subtle emotions, complex camera movements, and intricate scene compositions that contribute to compelling narratives.
However, while this diverse training data fosters improved video generation, it also brings to light potential challenges related to biases and representation. Ensuring the training data accurately reflects the wide array of human experiences and cultural contexts is vital to prevent the model from generating biased or skewed results. The future development of Sora, and AI video generation in general, hinges on a careful balance between the richness and diversity of the training data and the need to address inherent biases that might arise. In essence, the caliber of the training data is essential to the quality and responsible implementation of these models.
OpenAI's focus on training data diversity in Sora is a crucial aspect of the model's development. The quality and variety of the video and text data used to train Sora directly impacts the realism and versatility of the generated videos. If the training data is limited or biased, it can lead to problems. For instance, generated characters might exhibit unrealistic behaviors or interactions, or the model may struggle to customize scenes based on individual preferences. This is because the model's ability to learn and adapt is dependent on the breadth and depth of its training data.
Sora's ability to understand and incorporate emotional cues from text is another aspect affected by training data. A lack of emotional diversity or cultural context in the training data can result in misinterpretations and a failure to generate outputs that connect with viewers in a meaningful way.
Beyond visual quality, the temporal aspects of video, such as motion and transitions, are significantly shaped by Sora's training. Diverse training data is needed to ensure that videos exhibit smooth transitions and realistic motion. Similarly, the model's understanding of depth of field relies on a diverse set of training examples. If the training data is insufficient or focused on a limited range of camera techniques, the rendered depth may appear unrealistic, leading to a poor viewing experience.
The model's ability to accurately position objects within scenes depends heavily on its understanding of 3D spatial relationships. If the training data lacks complexity in real-world spatial layouts, Sora might struggle to generate believable scenes, resulting in visually disjointed outputs.
Sora's capacity for real-time feedback and adaptive learning relies on diverse user interactions. Limited data on how people interact with the videos can hinder this adaptive process, preventing the model from efficiently refining its output style. Moreover, the quality of Sora's cross-modal integration—blending text, audio, and video—is affected by the diversity of the training data. If the training data is skewed towards a particular modality, it can lead to a less coherent and compelling storytelling experience.
Another key aspect of training data diversity is its relationship to ethical implications. If the training data contains biases or promotes stereotypes, the outputs can reinforce these issues, potentially leading to the spread of misinformation or harmful content.
Ultimately, the range of narratives that Sora can adapt to and the video styles it can create is influenced by the variety of scenarios present in its training data. A limited range of narratives in the training data can restrict its flexibility and make it less effective at producing engaging stories across different genres. Therefore, ongoing efforts to improve the diversity and quality of training data will likely remain important as the technology evolves.
Sora's 60-Second Video Generation Analyzing Text-to-Motion Capabilities in OpenAI's Latest AI Model - Real-World Applications and Motion Understanding
Sora's ability to translate text into intricate and realistic motion within videos represents a significant advance in artificial intelligence. The model's deep understanding of movement and its capacity to simulate how objects and characters interact within a 3D environment enables the creation of remarkably lifelike videos. This text-to-motion capability opens doors to a wide range of uses, from enriching educational content to crafting immersive entertainment experiences. The potential for Sora to adapt to audience preferences in real-time, modifying the generated content on the fly, introduces a new era of interactive video creation. This technology's flexibility could transform various industries, though ethical concerns about misinformation and bias within AI-generated content must be carefully considered and addressed. As AI video generation advances, Sora's influence on content creation prompts reflection on how we craft and interpret visual narratives.
Sora's approach to motion generation is a step beyond traditional methods. Instead of relying on rigid pre-programmed sequences, it uses advanced algorithms to predict and generate fluid movement based on the context within the text prompt. This approach helps to make the generated animations appear more natural and less robotic.
A key part of how Sora works is its use of "spacetime patches". By breaking down videos into these smaller segments, the model is able to manage the complexity of video creation more efficiently. This approach can help create smoother motion and increase the overall coherence of the scenes.
Furthermore, Sora demonstrates impressive abilities in building dynamic scenes. It can adapt lighting, arrange objects, and manage character interactions in real-time, potentially leading to a much richer and more engaging viewer experience.
While some AI models concentrate on producing exceptionally high-quality still images, Sora's primary focus is on temporal resolution. This means that the smoothness and fluidity of motion is a top priority. This emphasis on the natural flow of motion is crucial for creating truly immersive and engaging stories.
The model's developers have integrated a feedback mechanism that allows Sora to learn from user interactions. It can adapt its output based on preferences and choices. This continual learning and adaptation aspect is essential for improving both the quality of the videos and for potentially creating personalized styles over time.
Sora exhibits an understanding of 3D spatial relationships. This means that it can arrange objects and elements within a scene in a way that aligns with how we perceive the physical world. This aspect significantly enhances the realism of the visual output, particularly when there are complex camera movements involved.
One of Sora's more intriguing capabilities is its ability to interpret emotional cues from the text prompts. This enables it to create nuanced scenes that reflect the specific sentiments described in the prompt. If successful, this feature could lead to videos that connect with viewers on a deeper level.
Sora doesn't always rely on a fixed frame rate. Instead, it can adjust the frame rate based on the complexities of the scene. This dynamic frame rate adjustment has the potential to generate smoother transitions and a more natural visual flow that aligns better with the dynamics of the storytelling.
Sora's creators have designed it to leverage cross-modal learning. This means it blends text, images, and audio to create a cohesive narrative experience. By integrating these different media types, Sora aims to enhance audience engagement and comprehension of the stories it generates.
The model incorporates advanced lighting algorithms that strive to emulate real-world lighting conditions. This focus on creating authentic shadows and reflections can greatly enhance the overall visual quality and the depth of the generated scenes. It remains to be seen how well Sora will be able to accurately capture the nuances of different lighting conditions in a variety of scenes.
While these features appear promising, there are still uncertainties about how effective these methods will be in practice and what their broader implications might be for the quality of the videos and the ethical considerations surrounding AI-generated content. Further exploration is needed to fully assess Sora's potential and its place in the future of video content.
Analyze any video with AI. Uncover insights, transcripts, and more in seconds. (Get started for free)
More Posts from whatsinmy.video: