Understanding Pupillary Constriction Causes and Medical Implications in High-Resolution Video Diagnosis
Understanding Pupillary Constriction Causes and Medical Implications in High-Resolution Video Diagnosis - High Speed Digital Analysis of Pupil Behavior in Natural Light Conditions
The ability to analyze pupil behavior digitally at high speed, particularly under natural light, marks a substantial leap forward in our understanding of the pupillary light reflex. This approach, known as rapid pupillometry, allows us to capture subtle and fast changes in pupil size, providing a clearer connection between neural activity and optical responses within the eye. Researchers are increasingly employing machine learning techniques, particularly deep learning models, to predict how pupils react to various light conditions, highlighting the intricate interplay of light spectrum and time in influencing pupil diameter. Moreover, advanced pupillometer technologies, characterized by high resolution, enable precise measurement of pupil dynamics, enriching our comprehension of visual function and potentially providing valuable diagnostic tools in neuroscience. Computational methods are progressively becoming integrated into the analysis of pupil behavior, yet this progress also emphasizes the importance of recognizing and mitigating artifacts to derive valid and meaningful findings from the collected data.
1. The pupillary light reflex (PLR), a well-established phenomenon, is typically studied in controlled environments. However, the speed and complexity of pupil reactions, influenced by factors beyond just light, like cognitive processes and emotions, necessitates high-speed digital analysis to fully grasp these dynamic changes.
2. Traditional pupillometry often operates in controlled, artificial lighting, limiting its ability to capture natural pupil behavior. High-speed digital analysis can overcome these limitations by providing a more accurate representation of pupil dynamics in natural lighting, crucial for understanding how the pupil behaves in the real world.
3. Pupil dilation is not a simple reaction to brightness. Neurological factors like new stimuli and focused attention play a role, causing changes in pupil size. The capacity to capture this in high resolution video is vital for a more complete understanding of pupil behavior.
4. Reaching frame rates beyond 1000 frames per second, these systems allow researchers to analyze pupil behavior at a granular level. Details in the pupillary response that would be missed with the human eye or slower methods can be observed through this high-speed technology.
5. There is growing evidence that pupil size can act as a biological marker for various physical and mental states. This emphasizes the potential for high-speed digital analysis in clinical practice and treatment monitoring, aiding in objective assessment and diagnostic procedures.
6. The connection between natural lighting and pupil response isn't uniform across individuals. Age, health, and even daily biological cycles (circadian rhythms) impact the way pupils react to light, making it challenging to develop generalized baseline measurements.
7. Pupil size is intricately tied to the activity of neurotransmitters such as acetylcholine and norepinephrine. High-speed video analysis provides a means to study these biochemical interactions in real time, under different lighting conditions, potentially revealing more about the underlying mechanisms of pupil regulation.
8. Artificial intelligence is being used in high-speed pupil analysis, promising to predict behavioral and emotional responses based on pupil dynamics. This has wide-ranging implications, from psychology to security, where subtle changes in pupil response could potentially be utilized.
9. It's surprising how pupil behavior analysis can also improve user interfaces in technology. By understanding how pupils react to different visual information, user experiences could be enhanced. This highlights the potential of high-speed analysis extending beyond biomedical applications.
10. While the potential is significant, there are challenges in standardizing methods for high-speed pupil analysis across varying light conditions and subjects. Inconsistencies could influence the reliability and consistency of research results, especially in clinical trials and broader research efforts.
Understanding Pupillary Constriction Causes and Medical Implications in High-Resolution Video Diagnosis - Medical Applications of Dynamic Pupil Measurement in Stroke Assessment
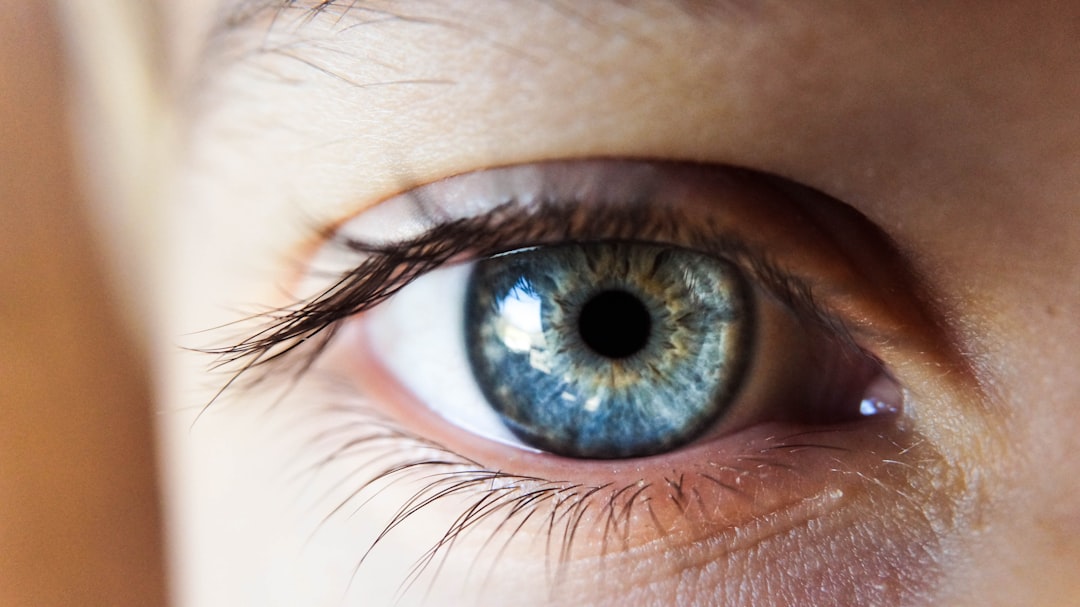
The application of dynamic pupil measurement, specifically through automated pupillometry, has emerged as a valuable tool in assessing stroke patients. This technique offers several advantages over traditional manual methods, primarily in its ability to provide more accurate and consistent measurements of pupil size, reactivity, and related features. Key metrics, such as the Neurological Pupil Index (NPi) and pupil constriction velocity (CV), are gaining recognition for their role in diagnosing and evaluating the severity of acute strokes. These metrics can act as independent markers for identifying neurological conditions and offer insights into the overall state of the patient's neurological function.
Moreover, high-resolution analysis enables the detection of subtle changes in the pupillary light reflex, which might otherwise be missed during manual examinations. This capability proves particularly useful in the context of acute brain injuries where early detection of such alterations can inform immediate treatment decisions and contribute to better prognosis. The integration of automated pupillometry into neurocritical care settings is fostering a shift towards a more precise and evidence-based approach to patient management, especially within the complexities of acute stroke scenarios.
While the clinical potential of automated pupillometry in stroke assessment is encouraging, it is essential to recognize that factors external to the neurological event can influence pupillary responses. Pain, certain medications, and elevated intracranial pressure can alter the expected reactions to light, underscoring the need for careful interpretation of the data. This highlights the importance of a comprehensive approach, integrating clinical context alongside the technological advances of automated pupillometry to optimize patient care.
Automated pupillometry, with its enhanced inter-rater reliability compared to manual methods, allows for more consistent measurements of pupil size, anisocoria (unequal pupil size), and light reactivity in stroke patients. This consistency is crucial, especially when evaluating subtle changes that might otherwise be missed by the human eye. Metrics like the Neurological Pupil Index (NPi) and pupil constriction velocity (CV) are becoming central to stroke assessment, as they provide objective measures of pupillary responses that can aid in diagnosis.
The pupillary light reflex (PLR), specifically the constriction aspect, serves as a powerful diagnostic and prognostic tool in acute brain injury scenarios, including stroke. Certain components of this reflex, especially how quickly the pupil constricts, act as independent predictors for stroke, suggesting a powerful link between pupillary behavior and stroke severity. Standardized pupillometry further improves the consistency of evaluations related to shifts in intracranial pressure (ICP) and other neurological conditions. However, factors like pain, opioid use, and increased ICP can alter the PLR, highlighting the need for careful consideration of these confounding elements.
Studies on acute stroke patients demonstrate that certain automated pupillometry parameters correlate significantly with the patient's outcome. This connection between pupillary dynamics and prognosis is promising but needs further research to refine the predictive value of pupillary metrics. High-resolution video diagnosis has identified abnormalities in pupil size and shape, linking them to changes in ICP and other neurological complications, adding further evidence to the importance of closely monitoring pupil behavior.
Interpreting the NPi is relatively straightforward: a score of 3 or higher indicates a healthy pupillary response, while lower scores signify reduced reactivity. The application of automated pupillometry in neurocritical care holds potential to enhance the accuracy of stroke diagnosis and improve patient management during acute episodes. However, achieving broader clinical acceptance will likely depend on standardizing methodologies to ensure consistent and reliable readings across different patients and settings. This is a particularly crucial area for future research, given the inherent variability in how individuals respond.
Understanding Pupillary Constriction Causes and Medical Implications in High-Resolution Video Diagnosis - Digital Recording Standards for Pupillary Light Reflex Testing
Digital recording standards are increasingly important in pupillary light reflex (PLR) testing, both in research and clinical settings. The PLR, a fundamental indicator of autonomic nervous system health, is now measured with greater precision through automated pupillometry. This allows for the detailed quantification of pupil constriction and dilation in response to light. Specialized systems, like PyPlr, and innovative imaging methods offer higher resolution and dynamic analysis of pupil behavior. This detailed analysis is vital for identifying and characterizing a range of neurological conditions and refining diagnostic practices.
However, a major challenge lies in the variability of pupillary responses. These reactions are not just influenced by light, but also a wide array of environmental and physiological factors. This variability underscores the urgent need for robust standards in digital recording techniques. Consistent methodologies are critical for ensuring the reliability and comparability of data across studies and individuals. The advancement of digital recording, coupled with more refined analyses, continues to expand the potential of pupillary assessment in neurodiagnostics and patient care, but standardization is paramount for maximizing the benefits.
The accuracy of digital recordings for pupillary light reflex (PLR) testing has reached a level where sub-millimeter changes in pupil size during light exposure can be detected. This high level of precision is important for neurodiagnostic work, especially when studying rapid changes in pupil size.
However, capturing high-speed video of the pupil comes with its own set of difficulties. Motion blur can easily distort the measurements, particularly when the eye or head moves. Using the right shutter speeds is vital for making sure the data collected under dynamic conditions is valid, highlighting the challenges of getting reliable pupillometry data.
Advanced computer processing methods used in high-resolution pupillometry can differentiate between the fast initial reaction of the pupil (phasic response) and the slower, longer-lasting adjustments to light (tonic response). This lets us better understand how the nervous system reacts to light both in the short term and the longer term, which can provide useful clues in diagnosing different medical problems.
The optimal frame rate for capturing pupillary responses is still being debated. Some researchers advocate for incredibly high frame rates (like 2000 frames per second) to catch every subtle aspect of pupil dynamics. But others warn that such high rates could lead to data overload and difficulties in analyzing the sheer volume of data.
Since individual eye anatomy varies significantly, it can introduce bias in PLR testing. Standardizing digital recording techniques can help build databases with average (normative) data for different populations. This is a crucial step for making sure that clinical assessments are precise and accurate.
It's also crucial to recognize that factors beyond just external light can influence PLR. Internal factors like someone's emotional state, fatigue level, and even the mental tasks they're engaged in can significantly change the pupil response. This highlights the need to incorporate a broad range of factors when using high-resolution pupillometry to analyze responses.
High-resolution video methods used to study pupil changes can be sensitive to environmental disturbances, such as reflections or unwanted glare. This means that detailed calibration procedures are essential to guarantee consistent and dependable results across different testing conditions.
Machine learning is changing the way we analyze PLR data. By using these techniques, we can move towards personalized medicine. This means being able to predict neurological assessments based on a person's individual responses, rather than relying on general population norms.
Studying pupillary responses across different ethnic groups presents a unique challenge. There can be variations in the basic size and responsiveness of the pupil, which means it's crucial to consider these demographic differences when developing accurate diagnostic approaches.
Even though digital recording methods are becoming more advanced, many clinical settings still use traditional methods for evaluating pupil responses. This gap emphasizes the ongoing challenge of effectively translating the newest research into everyday medical practice. This process requires careful adoption of these methods and appropriate training.
Understanding Pupillary Constriction Causes and Medical Implications in High-Resolution Video Diagnosis - Machine Learning Methods in Pupil Response Pattern Recognition
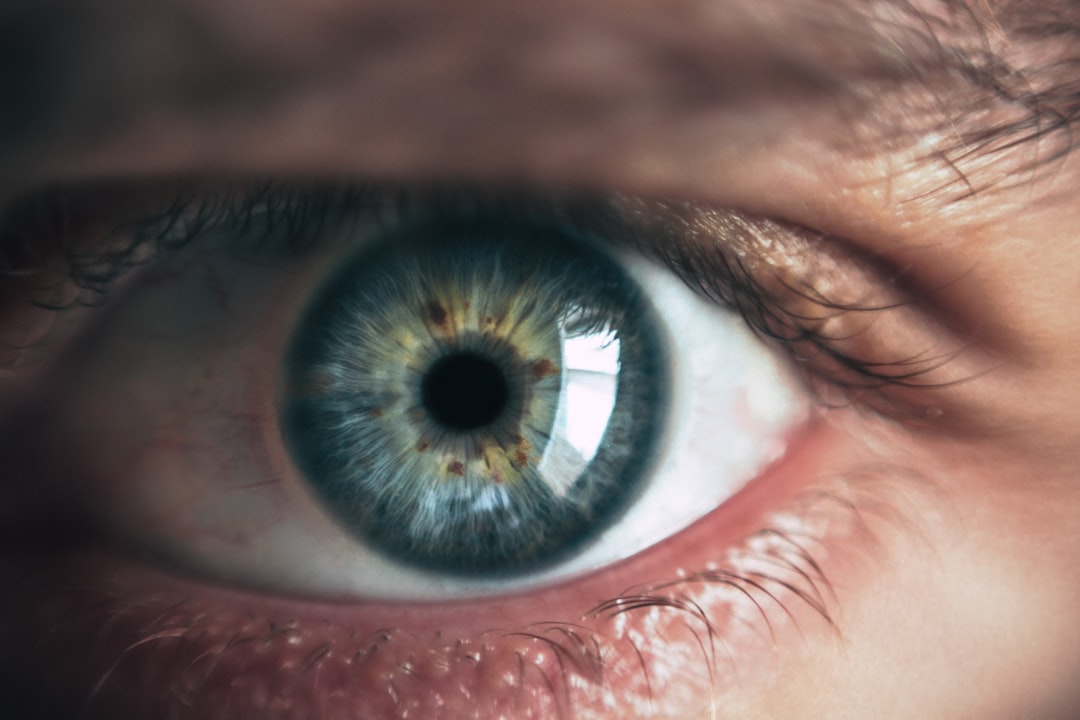
Machine learning is increasingly being used to decipher the intricate patterns within pupil responses, improving our understanding of the pupillary light reflex (PLR) and its significance in various medical contexts. These methods strive to capture the full range of pupil behavior, incorporating factors like light, time, and individual differences. By analyzing the complete sequence of pupillary responses, machine learning can help determine the expertise and skill of the operator doing the analysis. A primary goal is to create parameters that are less affected by variations in ambient light, ultimately making pupillary assessments more reliable and accurate, particularly when diagnosing conditions linked to the nervous system. The ability of high-resolution video to capture detailed pupil reactions, coupled with machine learning algorithms, offers the potential to uncover subtle variations in pupil behavior that might be missed during standard clinical assessments. However, the widespread adoption of these methods necessitates a push for standardized practices to ensure consistency and validity across different patient populations and healthcare settings. This standardization is crucial for maximizing the potential of these advanced tools in enhancing our diagnostic capabilities.
1. Machine learning algorithms, when trained on pupillary response data, can differentiate between automatic reactions triggered by light and those influenced by internal psychological factors. This highlights the nuanced and intricate nature of interpreting pupil behavior in real-time, showing that pupil responses are not always straightforward indicators of simple light reflexes.
2. The use of machine learning algorithms has been shown to enhance the detection of subtle shifts in pupillary response patterns, which conventional methods might miss. This increased sensitivity has the potential to lead to earlier identification of neurological conditions, offering the possibility of interventions that could improve outcomes.
3. Individual differences in pupil responsiveness are significant, with pupil behavior varying considerably depending on the person's cognitive load or mental demands. Machine learning can map these variations, potentially leading to more tailored neurocognitive evaluations, rather than relying on generic standards.
4. In machine learning, advanced data preprocessing methods are vital for removing unwanted noise and artifacts from high-resolution pupil tracking. Doing this helps to ensure that the generated data is reliable and comparable across different studies, improving the overall quality and rigor of research in this field.
5. Researchers have observed that machine learning can model the temporal dynamics of pupillary reactions quite well, capturing not only the peak dilation but also the recovery phases that follow. This ability to capture the full pupillary response sequence provides deeper insights into the workings of the autonomic nervous system.
6. The integration of machine learning into pupillary pattern analysis has opened up exciting possibilities for real-time monitoring systems. These systems could prove invaluable in acute clinical settings, enabling immediate feedback on a patient's condition and assisting with prompt medical decision making.
7. Interestingly, machine learning can be used to predict future pupillary responses based on historical patterns. This predictive capability could help identify individuals at risk for particular neurological conditions before symptoms appear, potentially allowing for earlier interventions.
8. Current limitations in machine learning applications for pupil analysis are linked to the availability of sufficiently diverse training datasets. This leads to the possibility of biases in the models, which could reduce the applicability of the findings across different populations. More representative training data is needed to overcome this limitation.
9. Machine learning's ability to analyze complex interactions between light and emotional states through pupil responses is driving advances in both clinical psychology and neuroscience. It illustrates the interdisciplinary benefits of this technology, expanding our understanding of how the brain and body interact with the environment.
10. While the potential of machine learning for pupil response analysis is clear, the field faces ongoing challenges. For example, we need continual refinement of algorithms to account for variations in eye anatomy and the wide range of response dynamics found in different demographic groups. Addressing these challenges is vital for realizing the full benefits of this technology in a diverse population.
Understanding Pupillary Constriction Causes and Medical Implications in High-Resolution Video Diagnosis - Video Frame Rates and Their Effect on Accurate Pupil Measurements
The accuracy of pupil measurements relies heavily on the video frame rate used during capture, given the rapid and constant changes in pupil size due to a variety of influences. To capture these dynamic changes, which include reactions to cognitive processes and emotional states, video systems need high sampling rates. Frame rates above 87 Hz are often necessary to accurately reflect the full range of pupillary responses. While higher frame rates can offer greater detail and insights, they also pose challenges in terms of data management and subsequent analysis. Furthermore, errors in measurements can be introduced when the pupil isn't consistently centered during recording, emphasizing the importance of meticulous technology and data capture techniques. Ultimately, careful consideration of the chosen frame rate is crucial to fully leverage high-resolution video analysis for studying pupil responses and for accurately applying this knowledge in medical diagnostics.
1. The speed at which video frames are captured, the frame rate, directly affects how accurately we can measure pupil size. For example, a standard 30 frames per second (fps) video might miss very fast changes in pupil diameter, while a 240 fps video could capture these fleeting shifts, potentially improving our diagnostic ability. This is particularly important when dealing with neurological conditions or situations where swift changes in pupil size are critical.
2. Evidence suggests that frame rates of 120 Hz or higher can significantly minimize blurring caused by motion. This is really important when trying to visually analyze how pupil size changes. Being able to get clear images of these changes is vital for understanding quick neurological responses and refining diagnostic methods.
3. Research shows that pupil diameter can fluctuate within mere milliseconds of a stimulus being presented. This reinforces the need for using high frame rate recording to capture these rapid changes, which can often be imperceptible at slower frame rates. Otherwise, we're likely missing a lot of important information.
4. It is a problem when the frame rates used in different devices are not consistent. It creates variability in pupil measurements and can make it tough to establish consistent, standardized testing protocols which are crucial for clinical evaluation and analysis. We need better standards across systems for the technology to be useful across research teams and clinics.
5. Just increasing the frame rate isn't a magic bullet. The quality of the lens and the lighting conditions are both big factors influencing the outcome of measurements. To optimize video recording for accurate pupil measurement, we need a holistic approach, not just focusing on frame rate.
6. Some more advanced recording methods, like variable frame rate, can change frame rate on the fly in response to the scene. This allows for adaptability and more robust data collection, especially for capturing a wider range of pupil behaviors that can vary drastically.
7. High-speed imaging systems that can exceed 1000 fps not only increase measurement precision but open up avenues for studying more complicated interactions between visual stimuli and how pupils react. This has huge potential for improving diagnosis in different clinical settings.
8. Even with recent advancements in technology, a lot of clinical settings still rely on standard video at around 30 fps. This makes it tough to effectively capture the fast pupillary changes linked to acute neurological conditions. To truly leverage new understanding of pupil behavior, we need faster capture methods to be more widely adopted in clinical settings.
9. The way a pupil responds can vary within the same person depending on the lighting. This underscores the need for high frame rates when taking into account those differences. Static measurements could misinterpret the patient's true neurological state if we don't understand the interplay between lighting and speed of reaction.
10. The connection between frame rate and pupil measurement brings to light an ongoing challenge in the field of engineering. We need to strike a balance between high-speed video capabilities and how efficiently we can analyze the data that comes out of it. This is critical both for immediate monitoring in a clinical environment, and for more detailed analysis done after data capture.
Understanding Pupillary Constriction Causes and Medical Implications in High-Resolution Video Diagnosis - Automated Screening Systems for Early Detection of Neurological Issues
Automated systems for early neurological issue detection are gaining attention because they provide objective measures of pupillary responses. These systems use automated pupillometry, a technique that precisely quantifies the pupillary light reflex (PLR) and related metrics like constriction speed and the Neurological Pupil Index (NPi). This real-time data offers the chance to pinpoint subtle changes in a person's neurological condition, potentially allowing for earlier detection of problems like acute strokes or brainstem damage, compared to traditional methods.
Despite their potential, it's important to remember that these automated systems need careful interpretation. The data from them should be considered alongside other patient information, since things like medication or the lighting conditions can change how the pupils respond. Finding a balance between the technology's capabilities and the natural variability in how pupils react is crucial. We need standardized ways to use these systems so we can get consistent and trustworthy results across different people and healthcare settings. Looking to the future, combining these high-resolution video analysis methods with machine learning and AI could improve our predictive abilities and further enhance our understanding of neurological health.
1. Automated systems for early neurological issue detection can capture pupillary responses in mere milliseconds, suggesting their potential for swift diagnosis in critical situations like stroke. This speed could be transformative for urgent medical assessments.
2. Studies have linked variations in pupil size to a variety of neurological conditions, including Alzheimer's and Parkinson's disease. This expands the possible uses of automated pupillometry in medical settings, potentially improving diagnostic capabilities.
3. Using high-resolution video, these automated systems can analyze pupil behavior with precision down to fractions of a millimeter. This is crucial for noticing small changes that might be important signs of neurological health, illustrating the fine detail now possible in diagnosis.
4. Recent developments in machine learning allow these automated systems to not just react to immediate pupil changes but also potentially predict long-term shifts in brain health. The hope is that this can lead to preventative healthcare approaches based on pupil behavior.
5. Because these automated screening tools continuously monitor, they can spot both long-term issues and temporary problems like fatigue or stress-related changes in pupil responses. This gives a more complete understanding of a patient's neurological state.
6. These systems can work in different lighting situations, making them helpful in various environments, from hospitals to field settings. This flexibility is essential in everyday situations where light levels change frequently.
7. Automated pupillometry systems can examine multiple patients concurrently, unlike traditional manual methods. This increases efficiency in busy medical environments and could lead to bigger research studies generating more reliable results.
8. Using automated systems to detect changes in pupil responses has been shown to make it easier to get consistent results across different clinicians. This addresses a common problem in manual assessments where individual judgment can affect how consistent the results are.
9. While promising, these automated systems can generate false positives, as things like medications or high anxiety can impact pupil reactions. This suggests a need for more sophisticated software to differentiate between real problems and normal variations.
10. A significant concern is the sheer amount of data generated by automated pupil analysis. The high-speed video generates so much information that it can become a challenge to interpret it in real-time for effective patient care if proper filtering and analysis aren't applied.
More Posts from whatsinmy.video: