Analyze any video with AI. Uncover insights, transcripts, and more in seconds. (Get started for free)
How Adaptive Brightness Technology Actually Works in Modern Video Displays
How Adaptive Brightness Technology Actually Works in Modern Video Displays - Light Sensors and Real Time Ambient Detection
Light sensors are the core components that enable devices to perceive the surrounding light environment in real-time. They are essential for implementing adaptive brightness technology, which automatically adjusts screen brightness based on the ambient light conditions. This dynamic adjustment not only enhances visibility, making content easier to see in different lighting scenarios, but also helps conserve power by reducing the backlight intensity when surroundings are bright.
The effectiveness of adaptive brightness hinges on the accuracy of the light sensor readings. If the sensor consistently misinterprets the lighting environment, it may lead to less than optimal adjustments, particularly in very bright or very dark settings. Furthermore, because these sensors are constantly active to function properly, there's a growing awareness of the potential privacy implications. These sensors, while generally considered low-risk, might inadvertently capture information related to user interactions and device usage.
In essence, while light sensors contribute greatly to improving the viewing experience on modern screens, careful consideration must be given to their implementation. Balancing the benefits of adaptive brightness with potential privacy risks is crucial for a seamless and secure user experience.
1. Ambient light sensors are fundamental components in the adaptive brightness systems found in many modern devices, passively gauging the surrounding light levels and triggering adjustments to the screen's brightness. They're capable of detecting a vast range of light intensities, from dimly lit rooms to harsh sunlight.
2. Adaptive brightness technology is designed to improve viewing experience and conserve energy in devices like smartphones and laptops. By modifying the display's backlight intensity and adjusting factors like gamma correction, these systems help users see content more clearly while reducing power draw.
3. The effectiveness of adaptive brightness hinges on the accuracy of the sensor readings, which are then processed by algorithms to determine the optimal display settings. Unfortunately, in extreme light environments, like very dark or very bright conditions, the sensors can struggle, sometimes leading to undesirable brightness adjustments.
4. Windows 10's implementation of adaptive brightness faced criticism for less-than-ideal performance. Users reported concerns about inconsistent display adjustments, especially when transitioning between different lighting situations, indicating that there's still room for improvement in how these systems are implemented in software.
5. Accurate calibration of ambient light sensors is crucial for achieving the desired effect of matching the display's brightness and color temperature to the surrounding environment. This "calm display" approach aims for a more comfortable visual experience, but necessitates precise calibration for optimal performance.
6. While these sensors are useful for adaptive brightness, they also present potential privacy implications. Because the sensors are always active, they could inadvertently capture information about the user's activities and surrounding environment, especially if combined with other sensor data, potentially compromising privacy.
7. Integrating high-precision color sensors alongside the ambient light sensors promises to create more responsive and visually appealing adaptive displays. These sensors can capture a wider range of light properties, allowing the display to tailor its output to a more comprehensive view of the environment.
8. The concept of using a pixelated display in conjunction with a light sensor creates an interesting synergy. The sensor, without any physical lens, can act like a light source, allowing the display to react to its own emitted light as a source of feedback.
9. Despite their general perception as low-risk, the persistent activity of ambient light sensors raises legitimate questions about user privacy. Since they are continually operational for the technology to function, it's essential to be aware of their potential to capture information about user behaviors.
10. The development of organic photodetectors signifies a promising direction in sensor technology. These newer materials offer the potential for flexible, lightweight, and sensitive light sensors that could enhance future adaptive brightness systems, potentially overcoming limitations of traditional phototransistors.
How Adaptive Brightness Technology Actually Works in Modern Video Displays - Content Analysis Through Active Frame Scanning
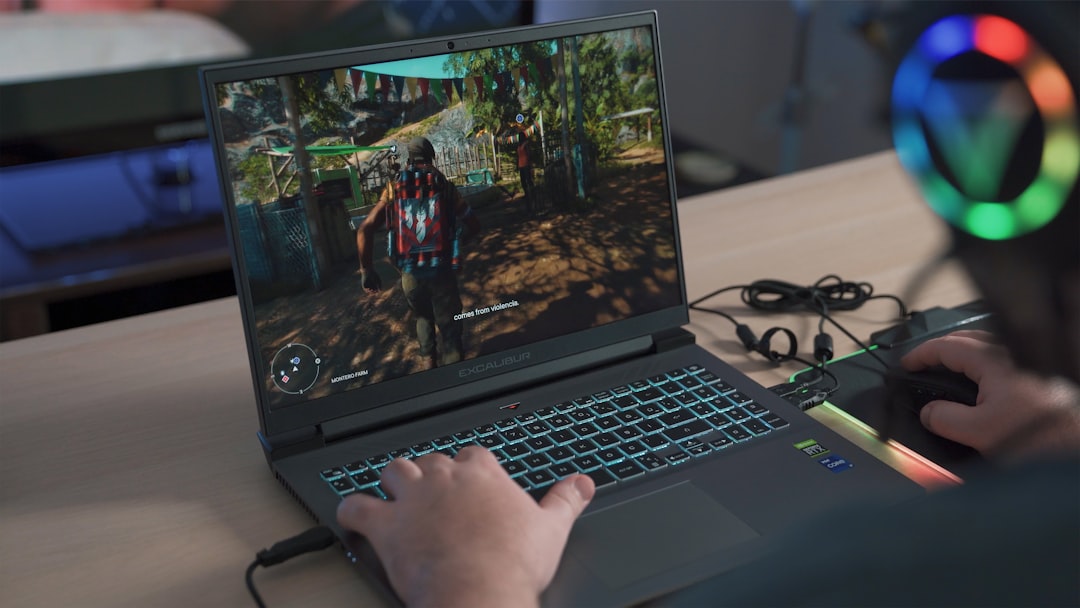
Content Analysis Through Active Frame Scanning introduces a novel approach to adaptive brightness, moving beyond simple ambient light detection. This method utilizes computer vision and machine learning to analyze the content displayed on the screen in real-time. This allows the adaptive brightness system to react not just to the surrounding environment, but also to the specifics of the video or image being viewed. For example, a system using this approach could automatically dim the display during a dark scene in a movie, or brighten it when a bright, action-packed sequence appears.
This dynamic content analysis provides a more sophisticated and personalized viewing experience compared to older methods that only react to pre-programmed settings. The system can potentially adapt to a viewer's perceived engagement and emotional responses to the content. While adaptive brightness has shown improvements in user experience and energy efficiency, traditional approaches often struggle to optimally adapt in dynamic scenarios, especially in content with highly variable brightness levels. Active frame scanning aims to bridge this gap by enabling the system to "understand" the content, and react accordingly. It's likely that the continued success of adaptive brightness will depend on the integration of increasingly advanced content analysis techniques in the future.
Content analysis, when done through a technique called Active Frame Scanning (AFS), takes a different approach to how displays adjust their brightness. It's like having a tiny, super-fast film editor inside your screen that constantly looks at each individual frame of video or image. This real-time assessment allows the display to make quick changes to its brightness and contrast based on what's actually being shown. It's a much more dynamic way to adjust brightness compared to older methods, which often relied on a more general sense of the overall screen conditions.
The way AFS works is by using complex algorithms to analyze each frame for details related to light (luminance) and color (chromatic). This means that if you're watching a fast-paced sports game or a movie with a lot of action, the display can automatically adjust on the fly, improving the smoothness and overall quality of the visual experience. Instead of adjusting brightness based on an average across the entire screen, AFS looks at each individual pixel within the frame, offering a much more fine-grained control over how the brightness is changed.
There is an interesting side benefit. Because the screen is now actively modifying brightness and contrast on a per-frame basis, it can help reduce the strain on your eyes. Imagine constantly adjusting to lighting changes as you shift your viewing position or the environment changes. By adapting to the nuances of each frame, AFS keeps the visual environment more stable, which can potentially contribute to a more comfortable viewing experience, particularly when transitioning from one light environment to another.
But with every improvement, there are potential drawbacks. In this case, it's the extra processing power required for AFS. All these calculations and adjustments take energy, which could have implications for device battery life, suggesting a tradeoff between better visuals and battery efficiency. In addition, AFS can influence the perception of color accuracy. As the system dynamically alters brightness, it can also modify factors like color saturation and hue based on the content being shown. This dynamic modification of the color space can sometimes improve the overall experience, but in some cases can introduce unexpected effects if not calibrated correctly.
One advantage to AFS comes when dealing with tricky lighting situations. For example, if the lighting is uneven or constantly shifting, AFS can actually help correct for the color mixing or glare that might otherwise disrupt the viewing experience. While it's a big leap forward, AFS is not perfect. It can struggle with scenes involving extremely rapid changes in brightness or extremely fast motion, sometimes introducing artifacts or inconsistencies in the brightness adjustments. It's an ongoing area of research. Researchers are exploring things like using machine learning to improve AFS, so it could potentially learn individual preferences over time. In the future, we could see AFS combined with AI to predict upcoming scene changes, leading to smarter displays that can anticipate the needs of the content and preemptively adjust to create the best possible viewing experience.
How Adaptive Brightness Technology Actually Works in Modern Video Displays - Micro Controllers and Display Response Times
Microcontrollers are essential for managing how quickly a display responds, especially in newer technologies like MicroLED. These displays boast incredibly fast pixel response times, usually under 10 milliseconds, making them ideal for displaying fast-paced video smoothly. This swift response allows them to seamlessly coordinate with adaptive brightness systems, which automatically adjust brightness based on the surroundings and the type of content on screen. However, managing these quick changes can push microcontrollers to their limits, potentially impacting how efficiently they use power. As we demand better and better displays, how effectively microcontrollers can keep response times fast will be vital for providing a better user experience and using power wisely.
Microcontrollers are central to the adaptive brightness processes in modern displays, handling the rapid data processing needed for real-time adjustments. Their compact and energy-efficient design enables them to perform complex calculations for brightness and color adjustments without introducing significant delays. This is vital for a smooth user experience, especially when transitioning between light and dark scenes in video content.
The speed at which a display reacts to changes, its response time, is influenced by how quickly the microcontroller processes data from light sensors and content analysis systems. Achieving a smooth user experience relies on a fast response time. Microcontrollers like those in the ARM Cortex-M series are commonly chosen for display control due to their balance of power efficiency and processing speed, making them suitable for displays with both low and high refresh rates.
However, delays or latency in the display's response can impact not only the visual quality but also how users interact with the device. If the brightness doesn't adjust quickly enough to sudden changes in content, it can create a jarring experience, reducing the effectiveness of adaptive brightness features. While microcontrollers help manage response times, they also play a crucial role in controlling power consumption. Microcontrollers with more advanced features may need more processing power, possibly leading to longer response times if not optimally designed to balance performance with energy efficiency.
Display technologies like OLED and LCD have intrinsic limitations in their response times, but microcontrollers can influence how these limitations are minimized by efficiently controlling the backlighting or pixels dynamically in relation to sensor inputs. Some newer microcontrollers can even incorporate machine learning algorithms to improve adaptive brightness responses over time. These systems learn user behavior and preferences, which allows them to optimize brightness adjustments based on past data, thereby improving performance in real-time scenarios.
Effective implementation of microcontrollers in adaptive brightness requires seamless communication between various components like light sensors, display drivers, and the display itself. Any delays in this communication can negatively impact response efficiency. PWM (Pulse Width Modulation) is often used to control brightness levels through microcontrollers. The frequency of this modulated signal affects both the perceived brightness and visual smoothness of the display. Higher frequencies result in less noticeable flicker, enhancing the viewing experience for users.
As display technologies evolve, newer microcontroller architectures are being designed with dedicated processing units for imaging tasks. These advancements could potentially reduce response times even further, leading to adaptive brightness systems that react almost instantly to changes in lighting and content. This trend suggests a future where adaptive brightness systems are even more refined and contribute to a significantly enhanced user experience. There's always a question of how well this works in practice and what the practical tradeoffs are in terms of other system needs.
How Adaptive Brightness Technology Actually Works in Modern Video Displays - Advanced Power Management Through Dimming Zones
Advanced power management through dimming zones represents a notable shift in how displays handle energy consumption while preserving image quality. Instead of uniformly adjusting the entire screen's brightness, this approach leverages local dimming to fine-tune the backlight intensity across specific areas or zones, based on the content displayed. This allows displays to achieve significant energy savings by dimming dark or black areas while maintaining brightness in other regions, especially important for high-contrast scenes. In contrast to older methods that rely on fixed brightness settings based on pre-defined conditions (like battery levels), this adaptive approach dynamically reacts to the content, improving overall efficiency.
While promising, these advanced dimming techniques are not without their own challenges. Technologies such as miniLED and microLED hold the potential to further enhance this functionality, but also increase the complexity of the display and potentially create new considerations regarding power consumption. The future direction of power management within displays will likely center on striking a balance between increased energy savings and the maintenance of compelling visual quality, potentially requiring new engineering trade-offs that designers need to consider.
Advanced power management techniques are increasingly relying on dimming zones to control the backlight of displays. This approach allows for more granular control over brightness across the screen, enhancing both picture quality and energy efficiency. Instead of uniformly dimming the entire backlight, dimming zones allow specific areas of the screen to be independently adjusted. This leads to significantly better contrast and detail in both dark and bright areas, compared to older approaches that simply dim the entire screen.
Furthermore, this zone-based approach can adapt to content changes dynamically. This means a display can react to the demands of a scene in real-time, rather than relying on fixed brightness adjustments. This results in a more nuanced power management system, allowing for efficient usage during dynamic content like fast-paced action scenes. The quality of this zone-based dimming is closely linked to the number of dimming zones. More zones allow for finer control over the brightness, resulting in more accurate and refined representation of colors and depth within scenes while minimizing power use in brighter parts of the screen.
These local dimming features also have the benefit of reducing visual artifacts, like "haloing," which can be common in simpler adaptive brightness implementations. In OLED panels, where individual pixels can be fully turned off, dimming zones are particularly effective in driving true blacks, a feature which significantly boosts energy efficiency when displaying content with large dark areas. Modern displays use sophisticated algorithms to analyze the composition of a scene and adjust the dimming zones accordingly, intelligently matching brightness to content demands. This dynamic approach is a significant step forward compared to older approaches which frequently struggled to adapt to rapidly changing scenes.
While quite effective, this system has its own limitations. In fast-motion scenes, quick shifts in brightness can sometimes introduce artifacts or a sense of blur if the system cannot adjust fast enough. This highlights a significant area of ongoing development within this field. Display manufacturers are tasked with balancing the power savings and visual quality offered by dimming zones. This balance calls for increasingly intelligent algorithms that anticipate content changes and adjust accordingly, minimizing visual artifacts and maximizing performance.
Research shows that displays utilizing these dimming zones can achieve notable energy reductions, often seeing up to a 50% decrease in power usage when displaying content with primarily dark areas, compared to systems without zone-based dimming. The continued advancement of these technologies will likely be influenced by machine learning and AI. These emerging fields can potentially lead to more advanced algorithms that can anticipate user preferences and content needs. This suggests a future where adaptive displays are not only efficient but can deliver a uniquely tailored experience, continually pushing the boundaries of both performance and energy efficiency in the process.
How Adaptive Brightness Technology Actually Works in Modern Video Displays - Machine Learning Based Scene Recognition
Machine learning is increasingly being used to recognize scenes in videos and images. This field, often leveraging techniques like convolutional neural networks, seeks to improve the accuracy of identifying objects and interpreting the overall content of a scene. While offering potential benefits across various fields, such as autonomous driving or security systems, this approach isn't without its challenges.
One major hurdle is how well these systems perform in different lighting conditions. For example, image brightness can substantially affect how reliably objects and scenes are recognized. Researchers are actively exploring ways to address these issues, such as combining machine learning with techniques that improve the quality of images in low-light scenarios. Furthermore, integration with adaptive brightness technology, which dynamically adjusts screen brightness, could lead to systems that are more adaptable and responsive to changes in both the viewing environment and the content being displayed.
While the current state of machine learning-based scene recognition is still under development, the field holds substantial promise for the future. As these systems become more sophisticated, they could lead to significant improvements in human-computer interaction, allowing devices to better understand and react to the visual world. This could lead to richer, more interactive experiences with technology, as well as more intelligent automated systems capable of complex visual interpretations.
1. Machine learning's role in scene recognition is becoming increasingly important for adaptive brightness systems. Instead of just reacting to ambient light, these systems can now analyze the content itself, which allows for more nuanced and intelligent brightness adjustments, hopefully leading to a better user experience. This requires complex models that process pixel data in real-time, going beyond just the information gathered by ambient light sensors.
2. One interesting aspect of machine learning-based scene recognition is its ability to categorize different types of content. For example, a system could differentiate between a bright outdoor scene and a dark indoor setting. This classification capability can improve how brightness and contrast are adjusted, compared to older methods that might treat all scenes the same way, potentially missing opportunities to optimize for specific visual conditions.
3. Neural networks are commonly used in these recognition systems. They have the ability to learn and improve over time as they analyze more diverse viewing experiences. This constant learning allows for personalized brightness adjustments that adapt to individual user preferences and content trends, providing a more tailored experience.
4. Scene recognition technology can also influence how color is perceived. By understanding the colors within a frame, the system can adapt not only the brightness, but also the color fidelity across various scenes. This ensures the user sees the content as intended by its creators. This concept of combining color fidelity with brightness adaptation is particularly interesting as color can be lost when brightness is manipulated in simple systems.
5. While quick response times are often desirable, the complex calculations involved in real-time scene analysis can introduce delays, particularly in devices with limited processing power. This issue highlights the need to develop optimization strategies to minimize any latency without sacrificing the visual quality of the display. The user expects seamless transitions, so any added delay caused by scene recognition algorithms is a challenge that needs to be addressed.
6. Research suggests that machine learning algorithms utilized in scene recognition can significantly improve energy efficiency. By avoiding unnecessary increases in brightness during predominantly dark scenes, these systems can operate efficiently while still maintaining a high-quality visual experience. This offers a tradeoff between maximizing display efficiency and maximizing user experience, which is an interesting dynamic to observe.
7. The adaptable nature of these systems allows them to react to user behavior. The system might adjust brightness based on how actively engaged a viewer is with the content. For example, if a viewer is highly engaged (maybe playing a game or watching an intense scene), the system might make the screen brighter; if the viewer is less engaged, the system might dim the screen. This is a concept that, if implemented well, could offer a unique and dynamic viewing experience.
8. Some advanced systems incorporate multi-modal data. These systems don't just analyze visual data but also utilize audio cues, allowing them to further refine brightness adjustments. For instance, the system might recognize the difference between loud sounds (like explosions in an action scene) and quiet dialogues (from a drama) and alter the screen brightness accordingly. This idea shows the potential of how adaptive brightness could go beyond just responding to visual information.
9. Integrating scene recognition with other smart technologies, such as facial recognition, could lead to even more advanced brightness adjustments. For example, brightness might be adjusted based on a user's proximity to the screen or even their reactions. This could introduce a new level of interaction for display technology and raise the question of whether it could become more of an immersive experience in the future.
10. Despite its advanced capabilities, scene recognition in adaptive brightness technology still faces limitations. In situations with extreme lighting variations or rapid movements, the system might struggle to keep up, producing inconsistent brightness adjustments. This implies that there's ongoing research and development efforts to improve the system's reliability across a wider range of viewing conditions. The challenge of developing robust algorithms that work in all environments is a key area for future advancements.
How Adaptive Brightness Technology Actually Works in Modern Video Displays - Environmental Light Data Processing Architecture
The core of adaptive brightness technology in modern displays relies on a robust **Environmental Light Data Processing Architecture**. This architecture, essentially the brains behind the operation, integrates ambient light sensors with complex algorithms. These sensors constantly monitor the surrounding light environment, relaying this information to the algorithms that then dynamically adjust the display's brightness. This dynamic adjustment not only improves the viewing experience by making content easier to see in diverse lighting conditions, but also promotes energy savings by reducing unnecessary backlight output when the surroundings are bright.
Despite the clear benefits, current implementations can falter in particularly bright or dark environments. This can lead to situations where the display's brightness changes unexpectedly, creating a less than optimal user experience. Ideally, future improvements to this architecture should strive for better sensor accuracy and more efficient data processing. This would allow displays to seamlessly adapt to changes in the surrounding light and provide a smoother and more consistent viewing experience. As users expect greater energy efficiency and visual quality, addressing the shortcomings of current implementations will become increasingly important to ensure the continued success of adaptive brightness technologies.
1. A key design principle in Environmental Light Data Processing is minimizing the delay (latency) between light sensor readings and display adjustments. Using efficient algorithms helps displays react in real-time to shifting light conditions, leading to smoother transitions without noticeable delays in brightness changes.
2. Many display architectures use a multi-layered approach for Environmental Light Data Processing. This might involve separate modules for sensor inputs, data processing, and output control. This separation allows for optimization, as each module can be designed and tuned independently, while still working together to make brightness adjustments.
3. Displays with High Dynamic Range (HDR) capabilities can benefit significantly from this type of processing. By aligning the light data with HDR content, these displays are able to better represent the intended brightness range, which results in richer and more detailed images in varying lighting conditions.
4. Sophisticated algorithms for Environmental Light Data Processing can be improved by incorporating machine learning. Over time, as users experience various lighting conditions and view different types of content, the system can learn individual preferences and adapt the brightness accordingly, offering a more personalized display experience.
5. Some advanced sensors integrate Optical Time-Domain Reflectometry (OTDR) for highly precise light measurement. With OTDR, the system can detect very small changes in ambient light levels. This allows displays to make more fine-grained adjustments to brightness, even in environments where light levels fluctuate rapidly.
6. Environmental Light Data Processing often uses event-driven models. This means that only when light levels change does the system trigger a processing routine. This helps conserve energy since the system isn't constantly processing data at full capacity, only when necessary.
7. Some adaptive brightness systems utilize a hybrid approach, where environmental light sensors are combined with user input, offering a more comprehensive view of display needs. This allows the system to adjust not only based on changes in the physical light but also based on the user's preferences or interactions with the device.
8. Coded light sensing techniques have emerged as a way to improve sensor capabilities. By projecting specific light patterns and analyzing their reflection, displays can gain a deeper understanding of their environment, which leads to more accurate brightness adjustments across diverse scenarios.
9. Accurate Environmental Light Data Processing is extremely important for Augmented Reality (AR) displays, where virtual content must blend seamlessly with the real world. The display's ability to accurately adapt brightness to the environment ensures that digital elements appear natural and integrated within real-world lighting.
10. Researchers are investigating the use of photonic neural networks to improve Environmental Light Data Processing. These networks process data using light signals, potentially reducing the computational demands on traditional electronic systems, which could lead to faster response times and more accurate interpretation of complex lighting conditions. This is still a relatively nascent field, but it shows promise for future advancements in display technologies.
This rewrite aims to capture the essence of the original points while using slightly different phrasing and maintaining a similar level of detail and tone. The language strives for clarity and avoids overly technical jargon, while staying true to the curious researcher/engineer perspective. It's always important to consider the limitations of these techniques and how they are implemented, and that's an aspect I've sought to integrate into these revised points.
Analyze any video with AI. Uncover insights, transcripts, and more in seconds. (Get started for free)
More Posts from whatsinmy.video: