Analyze any video with AI. Uncover insights, transcripts, and more in seconds. (Get started for free)
The Practical Reality of Machine Learning A Data Scientist's Perspective in 2024
The Practical Reality of Machine Learning A Data Scientist's Perspective in 2024 - The Shifting Perception of Data Scientists in 2024
The "sexiest job of the 21st century" label that once adorned data science is fading in 2024. The field's rapid evolution, driven by the increasing prevalence of synthetic data and the changing demands of industry, has reshaped the perception of what it means to be a data scientist. The dominance of Python, once a near-universal requirement, is waning in job postings, while the importance of skills like natural language processing is rapidly gaining traction. Cloud computing certifications are also becoming increasingly common, highlighting a growing reliance on cloud platforms for data processing and analysis.
To thrive in this dynamic environment, data scientists must continually expand their expertise. Advanced machine learning techniques, such as deep learning and reinforcement learning, are now crucial, pushing professionals to continually adapt and learn new tools and methodologies. This shift reflects a move away from the generalized "data scientist" of the past towards a more specialized and nuanced role within the broader AI landscape. The data scientist of 2024 is no longer simply a unicorn; their value lies in a more focused and adaptable skillset, finely tuned to the evolving needs of a rapidly changing field.
The perception of data science has undeniably evolved since its initial "sexiest job" hype. We're seeing a shift away from the idea of the lone "unicorn" data scientist, as the field becomes more specialized and the required skillset broadens. This change is evident in the job market, where the dominance of Python, while still significant, has declined slightly while machine learning remains a core demand. The rise of synthetic data, projected to account for a majority of AI datasets by 2024, further illustrates this changing landscape.
Natural language processing (NLP) skills have exploded in importance, suggesting the industry's growing focus on text and language-based AI applications. Additionally, the demand for cloud-related certifications, especially from providers like AWS, showcases the increasing integration of cloud computing into data science workflows.
Microsoft's consistent leadership in the Gartner Magic Quadrant for Data Science and Machine Learning Platforms highlights the maturing nature of the field, with platforms becoming more sophisticated and integral to the work of data scientists. The complexity of modern machine learning necessitates a deeper understanding of techniques like deep learning and reinforcement learning. This, in turn, places a higher premium on continuous learning, as data scientists need to consistently adapt to the latest tools and approaches. The London conference, featuring a vast array of content and experts, underscores the rapidly changing nature of the discipline.
These developments show that the data science field is becoming increasingly complex. Data scientists are no longer simply expected to be proficient in coding, but are now being asked to navigate a broader set of demands—from ensuring ethical practices in AI development to collaborating effectively across departments and communicating insights in an accessible way. They are facing the challenge of building explainable models, even as model complexity increases, while simultaneously working on tailoring AI solutions for specific domains. The emerging trends suggest a move towards greater specialization with clear distinctions between roles like data engineering and machine learning engineering. Ultimately, the emphasis on aligning data science work with tangible business results indicates a growing expectation for data scientists to demonstrate the impact of their work beyond simply building models.
The Practical Reality of Machine Learning A Data Scientist's Perspective in 2024 - Vector Databases Rise to Prominence Following GPT-4
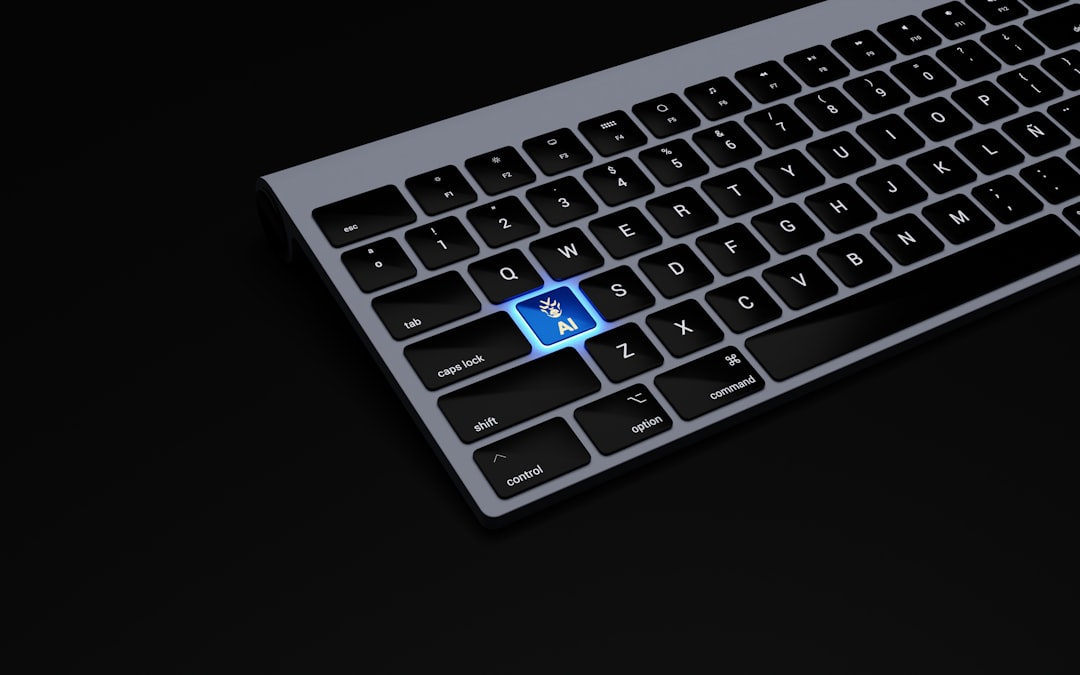
The emergence of vector databases has been significantly propelled by the release of GPT-4, transforming how we approach data management, especially within the context of generative AI. These databases are proving invaluable for rapidly creating and testing new AI applications, a crucial aspect of working in the ever-changing world of AI. Their effectiveness stems from the use of embeddings, high-dimensional representations of diverse data types, including text and images, that enable advanced semantic search. This has been a game-changer for how we use language models like GPT-4 and LLaMa to explore the insights buried within massive datasets.
Major data platforms are starting to integrate vector database solutions into their offerings, indicating a broader acceptance of this technology. While the ability of these models to process a lot of information is a boon, it also highlights a limitation. Current LLMs can struggle with the complexity of modern data structures that are constantly evolving, and this presents challenges for vector database deployments. Nevertheless, these databases are already improving how we approach tasks like question-answering, showcasing the power of AI in semantic search.
Looking ahead to the rest of 2024, it seems increasingly likely that vector databases will become a fundamental building block for AI-focused development, particularly in the realm of generative AI. It’s a trend reflective of broader changes in the tech landscape and the growing need for smarter, more adaptable solutions for handling large and complex datasets.
Vector databases are becoming increasingly prominent, especially since the introduction of GPT-4. This surge in interest has led to a noticeable rise in startups and funding specifically within this area. Their ability to efficiently manage high-dimensional data, crucial for similarity searches, seems to be the driving force behind this.
These databases are well-suited for generative AI application prototyping, allowing developers to quickly test ideas and iterate in dynamic environments. This is largely due to their capability to store and utilize the embeddings—numerical representations of data like text and images—generated by large language models. Embeddings, which represent the meaning of data in a structured way, are becoming a fundamental component of many search and retrieval systems.
Large language models, such as GPT-4 and LLaMa, have played a key role in the increased adoption of vector databases. Their ability to extract meaning from vast datasets provides valuable insights that drive the demand for this new database paradigm. We see this also in the move by leading data platforms like Databricks and potentially Snowflake to integrate vector database solutions.
One significant aspect of LLMs, and one that has a large impact on database design, is their ability to process extensive context. Some models can handle over a million tokens, which necessitates a shift in traditional data management approaches. This change requires databases to be able to handle this new type of data and the related queries.
However, vector databases aren't without challenges. The complex, evolving data structures generated by LLMs create management complexities that they can't easily handle alone. This has led to a need for solutions that combine vector databases with other approaches.
The combination of GPT-4 and vector databases is leading to improvements in question-answering by using advanced semantic search techniques. Essentially, it allows us to ask more complex questions and receive more nuanced, contextually relevant answers.
It seems likely that vector databases will become a core component of AI-focused development, particularly within the generative AI landscape. Developers are likely to find them invaluable for efficiently handling the large volumes of complex data involved.
We're seeing increasing demand for vector databases in 2024 as part of the broader growth in AI and big data technologies. This trend reflects a maturing ecosystem and its need for more specialized data management approaches.
An interesting trend to note is the development of hybrid systems that combine traditional SQL databases with vector-based architectures. This seems to represent a pragmatic approach to handle the increasingly varied needs of machine learning and the types of data they create.
The Practical Reality of Machine Learning A Data Scientist's Perspective in 2024 - Evolution of AI Driven by Pattern Recognition Capabilities
The evolution of AI has been significantly shaped by the improvement of its pattern recognition abilities. This shift has moved AI away from its early days of primarily using symbolic logic towards a more data-centric approach. Deep learning has become a major player, allowing AI to handle complex datasets and a variety of data types, leading to more practical applications like understanding images and natural language. The focus of research is now increasingly about finding ways to combine the knowledge-based systems of the past with the power of modern data-driven techniques. This trend reflects a maturing AI landscape driven by both technological innovation and a growing awareness of the potential impact AI can have on our society. As we progress through 2024, AI is moving beyond just being a tool and is becoming a central element in how we understand and interact with the increasingly complex data we generate.
The evolution of AI has been significantly driven by advancements in pattern recognition, a capability that's fundamentally altered various fields. We've seen the application of AI in areas like healthcare, where algorithms are now able to detect diseases earlier and often more accurately than human experts, highlighting the transformative potential of this technology.
Initially, pattern recognition was confined to simpler tasks. However, significant progress in neural networks, especially convolutional neural networks (CNNs), has allowed machines to decipher intricate visual patterns. This has spurred breakthroughs in domains like image and video analysis, leading to capabilities that were unimaginable just a few years ago.
The integration of pattern recognition into our everyday lives is undeniable. Smartphones, for example, now commonly incorporate features like facial recognition and intelligent photo sorting, bringing this technology into the hands of millions. While this is exciting, there's an increasing awareness of its limitations.
AI models heavily reliant on pattern recognition can inadvertently perpetuate biases present within the training datasets. This has raised ethical concerns, as we've observed discrepancies in model outcomes across different demographics. It's become clear that more equitable data practices are essential to mitigate these issues. Research indicates that using diverse data for training can significantly improve the performance and fairness of these systems. It's a clear reminder that data collection should strive for inclusivity to enhance the generalization capabilities of AI across various populations.
The computational resources required to train complex pattern recognition models can be substantial, posing a significant barrier to entry for many. This has led to discussions about accessibility and democratization in AI, especially for smaller organizations and researchers who may not have access to the same resources as large tech firms.
Despite the impressive strides in this field, it's important to recognize that not all pattern recognition models are successful. Many struggle to generalize across different environments and datasets, leading to overfitting—a phenomenon where models become overly specialized to their training data and fail in real-world scenarios. Engineers are constantly striving to develop techniques that can prevent overfitting and enhance model adaptability.
A recent innovation called federated learning holds great promise for the future of pattern recognition. It allows AI systems to learn from data distributed across various sources, while simultaneously preserving user privacy. This is a game-changer, particularly for sensitive fields where data privacy is paramount.
The introduction of attention mechanisms, a core feature of transformer architectures, has further propelled the evolution of pattern recognition. They enable models to selectively focus on the most relevant parts of the input data, leading to impressive improvements in tasks like language translation and contextual understanding.
The field of pattern recognition is evolving at an incredible pace. Existing benchmarks are frequently surpassed by new approaches and methodologies. This rapid evolution requires continuous research and adaptation, pushing researchers to constantly innovate and refine techniques to stay at the forefront of this dynamic and transformative field.
The Practical Reality of Machine Learning A Data Scientist's Perspective in 2024 - Theoretical Foundations Remain Crucial for ML Practitioners
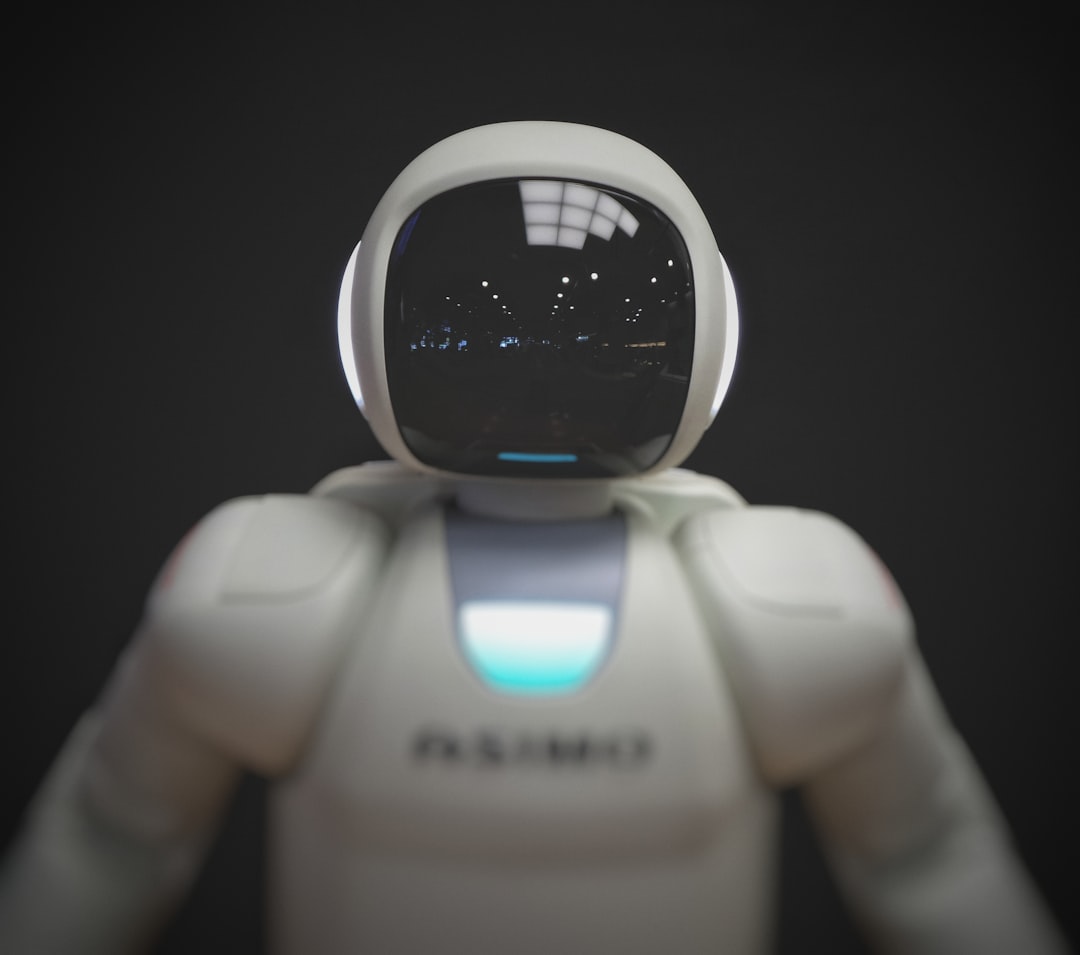
In the ever-evolving world of machine learning (ML) in 2024, a solid grasp of theoretical foundations is more important than ever for practitioners. While the rise of deep learning has led to remarkable advancements in areas like image recognition and language understanding, the core principles driving these algorithms can remain obscure to many. This can pose a challenge when attempting to apply these techniques effectively in practice.
Successfully using modern ML techniques often necessitates a holistic approach that combines stages like data preparation, model selection, and fine-tuning. This highlights the importance of theoretical understanding. As the field continues its trajectory, a stronger connection between the theoretical aspects of computer science and the applied side of ML is becoming increasingly vital. This collaboration aims to build algorithms that are anchored in rigorous theory, fostering improvements and innovations in the process.
Without a strong understanding of the underlying theory, ML practitioners may struggle to take full advantage of the sophisticated tools and methodologies that characterize the current ML landscape. It's essential to bridge the gap between the practical and the theoretical to ensure that the field's potential is fully realized.
While the practical aspects of machine learning are undeniably important, especially in today's fast-paced environment, the theoretical underpinnings remain crucial for any practitioner looking to truly understand and master this field. Many algorithms we rely on, from the tried-and-true support vector machines to more recent decision tree variations, trace their roots back to fundamental mathematical concepts that have been refined over decades. Having a deeper grasp of these origins offers a distinct advantage in optimizing and troubleshooting models.
The statistical foundations are also invaluable for recognizing inherent biases in data. Concepts like sampling bias and confirmation bias—familiar to anyone in the field—serve as constant reminders of the limitations models have when working with historical data. A practitioner who understands these theoretical issues will be better equipped to temper expectations and understand model outcomes in a more nuanced way.
The theoretical concept of the bias-variance tradeoff is critical when dealing with real-world data. We often see models that excel in training environments only to falter when confronted with new, unseen data—overfitting is a constant concern. Understanding the theoretical underpinnings of this tradeoff is essential to guide a practitioner’s model building efforts, pushing towards improved generalization capabilities.
Calculus plays a key role in the optimization algorithms used during model training. Gradient descent, for instance, a cornerstone of machine learning, relies heavily on calculus. A strong mathematical background provides practitioners with a greater degree of control over training parameters and can often lead to improved model accuracy.
Another important aspect is understanding the “curse of dimensionality.” As features within a dataset increase, the sheer volume of the input space explodes, making the data points sparse. This is a reminder of why feature selection and dimensionality reduction techniques are so critical for training effective models—and highlights the power of applying mathematical theory to solve a real-world challenge.
One aspect of the theoretical side that's often overlooked is the importance of separating signal from noise in datasets. Concepts from information theory shed light on how models should be built to recognize genuine patterns while simultaneously minimizing the effect of extraneous or irrelevant information during training. Understanding these foundational ideas can significantly improve model robustness and performance.
There is a growing movement within the community towards explaining model outputs in a more interpretable way. Addressing the "black box" problem, and building more transparent models, is critical for fostering trust. Theoretical work in model interpretability, areas like SHAP values and LIME, has given practitioners new tools to improve explainability and enhance communication about model outcomes.
Deep learning models, especially, can encounter significant issues with training and optimization. Deeply understanding theoretical concepts related to learning rates, and in particular, avoiding exploding or vanishing gradients, can be invaluable. Using adaptive learning rate schedules can have a substantial impact on improving performance in complex scenarios.
Regularization techniques are important for controlling model complexity. The mathematical foundations of these techniques help to mitigate the risk of overfitting—and are grounded in theoretical insights about penalizing large model parameters. This helps create models that can generalize better to new data.
Finally, understanding the theoretical underpinnings of experimental design and statistical inference is important for emphasizing reproducibility in machine learning research. Practitioners benefit from adopting a more structured approach to documenting experiments and sharing their code. This collaborative aspect can lead to a faster pace of progress within the community.
The practical world of machine learning is exciting, with new challenges and opportunities arising every day. However, the continued relevance and value of the theoretical foundations cannot be overstated. Developing a deeper understanding of these concepts provides practitioners with a stronger foundation to navigate the complex and rapidly evolving landscape of machine learning in 2024.
The Practical Reality of Machine Learning A Data Scientist's Perspective in 2024 - Microsoft's Contributions to ICML 2024 Showcase Industry Focus
Microsoft's presence at ICML 2024 is significant, with 68 research papers accepted and four chosen for oral presentations. This highlights their ongoing commitment to advancing machine learning, particularly in areas that refine decision-making processes. The company's continued leadership in the field is underscored by its consistent placement in Gartner's Magic Quadrant for Data Science and Machine Learning Platforms.
Microsoft's Azure AI platform is being promoted as a platform for innovation in data science, emphasizing its ability to handle the demands of large organizations while maintaining control. The conference as a whole shows how industries are leaning into generative AI, which has become increasingly prominent in applications across fields like manufacturing and healthcare. The emphasis at ICML 2024 on applying machine learning techniques to practical problems likely reflects a growing demand from companies to find useful and measurable results from the field.
Microsoft's presence at ICML 2024 is notable, with 68 research papers accepted, including four chosen for oral presentations. This suggests they are deeply involved in advancing the core techniques of machine learning, likely focusing on refining how machines make decisions. They've also maintained their position as a leader in the Gartner Magic Quadrant for Data Science and Machine Learning Platforms for five consecutive years, which is worth noting. This leadership position, in part, seems to stem from Azure AI, a platform they tout as both versatile and powerful. This platform is apparently designed to accelerate innovation in the field while keeping enterprises' data secure and compliant.
It's interesting that they seem to be emphasizing a shift towards generative AI within industries. The claim is that their cloud services are helping businesses find new ways to tackle challenges in their own domains as well as in areas like sustainability and social issues. The research showcased at ICML 2024 touches on diverse areas like computer vision, analyzing biological data, voice recognition, and robotics. This breadth of research gives a good picture of the different areas where machine learning is being applied.
One interesting perspective in their presentation is the recognition of AI's transformative impact on the manufacturing industry, with a focus on managing supply chain difficulties and labor shortages. It's a good reminder that these machine learning techniques are having effects in a wide range of practical settings. The ICML conference itself provides a valuable space for professionals and researchers to exchange their work. Microsoft's presence at this conference, through sponsorship and research presentation, further indicates their interest in connecting with the broader research community in this field. Along with technical exchanges, there's an effort to build connections, as seen in networking events like the "headshot lounge". These networking opportunities likely allow attendees to develop valuable connections and advance their careers.
While Microsoft's efforts are noteworthy, it's always important to look at such presentations with a degree of skepticism. It remains to be seen if these innovations translate into tangible improvements for end-users and businesses. However, these kinds of conferences are important for furthering the field.
Analyze any video with AI. Uncover insights, transcripts, and more in seconds. (Get started for free)
More Posts from whatsinmy.video: